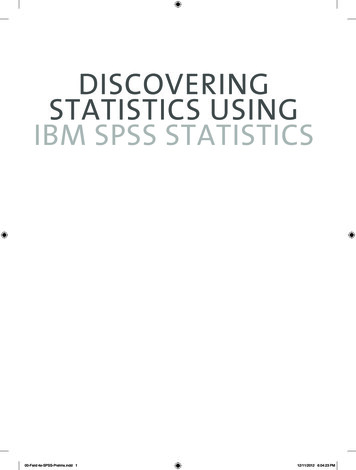
Transcription
DISCOVERINGSTATISTICS USINGIBM SPSS STATISTICS00-Field 4e-SPSS-Prelims.indd 112/11/2012 6:04:23 PM
C AT I S F I E D C U S T O M E R SDiego(Megan Chapman)Tonks(Erica Eckert)Cody(Andrea Luu)Sarah Dahl'sCatProf Fluffypants(Sanj Choudhury)Coltrane(Krista Soria)Sams(Claudia Pama)00-Field 4e-SPSS-Prelims.indd 2Nightshade(Dave Verkuijl)Kamaji & Choccy(Emily MargaretGay)12/11/2012 6:04:36 PM
DISCOVERINGSTATISTICS USINGIBM SPSS STATISTICSAND SEX AND DRUGS AND ROCK ’N’ ROLL4TH EDITIONANDY FIELD00-Field 4e-SPSS-Prelims.indd 312/11/2012 6:04:38 PM
SAGE Publications Ltd1 Oliver’s Yard55 City RoadLondon EC1Y 1SPSAGE Publications Inc.2455 Teller RoadThousand Oaks, California 91320SAGE Publications India Pvt LtdB 1/I 1 Mohan Cooperative Industrial AreaMathura RoadNew Delhi 110 044SAGE Publications Asia-Pacific Pte Ltd3 Church Street#10-04 Samsung HubSingapore 049483Editor: Michael CarmichaelDevelopment editor: Robin LuptonProduction editor: Ian AntcliffCopyeditor: Richard LeighProofreaders: Louise Harnby; Kate Harrison;Anna Gilding; Jennifer HinchliffeIndexer: David RudeforthMarketing manager: Ben Griffin-SherwoodCover design: Wendy ScottTypeset by:Printed by: Andy Field 2013First edition published November 2002Second edition published 2005Third edition published 2009Apart from any fair dealing for the purposes of research orprivate study, or criticism or review, as permitted under theCopyright, Designs and Patents Act, 1988, this publicationmay be reproduced, stored or transmitted in any form, or byany means, only with the prior permission in writing of thepublishers, or in the case of reprographic reproduction, inaccordance with the terms of licences issued by the CopyrightLicensing Agency. Enquiries concerning reproduction outsidethose terms should be sent to the publishers.Library of Congress Control Number: 2012944559British Library Cataloguing in Publication dataA catalogue record for this book is available fromthe British LibraryISBN 978-1-4462-4917-8ISBN 978-1-4462-4918-5 (pbk)00-Field 4e-SPSS-Prelims.indd 412/11/2012 6:04:38 PM
CONTENTSPrefacexixHow to use this bookxxvAcknowledgementsxxxDedicationxxxiiSymbols used in this bookxxxivSome maths revisionxxxvi1 Why is my evil lecturer forcing me to learn statistics?1.1. What will this chapter tell me? 1.2. What the hell am I doing here? I don’t belong here 1.2.1. The research process 1.3. Initial observation: finding something that needs explaining 1.4. Generating theories and testing them 1.5. Collect data to test your theory 1.5.1.1.5.2.1.5.3.1.5.4.1.5.5.1.5.6.Variables Measurement error Validity and reliability Correlational research methods Experimental research methods Randomization 1.6. Analysing data 1.6.1.1.6.2.1.6.3.1.6.4.1.6.5.Frequency distributions The centre of a distribution The dispersion in a distribution Using a frequency distribution to go beyond the data Fitting statistical models to the data 1.7. Reporting data 1.7.1. Dissemination of research 1.7.2. Knowing how to report data 1.7.3. Some initial guiding principles 1.8.1.9.1.10.1.11.1.12.Brian’s attempt to woo Jane What next? Key terms that I’ve discoveredSmart Alex’s tasksFurther 83839v00-Field 4e-SPSS-Prelims.indd 512/11/2012 6:04:39 PM
viD I S C O VE R I N G STAT I ST I C S US I N G S P SS2 Everything you never wanted to know about statistics2.1.2.2.2.3.2.4.What will this chapter tell me? Building statistical models Populations and samples Statistical models Reporting statistical models Brian’s attempt to woo Jane What next? Key terms that I’ve discoveredSmart Alex’s tasksFurther 83 The IBM SPSS Statistics environment892.4.1. The mean as a statistical model 2.4.2. Assessing the fit of a model: sums of squares and variance revisited 2.4.3. Estimating parameters 2.5. Going beyond the data 2.5.1. The standard error 2.5.2. Confidence intervals 2.6. Using statistical models to test research questions 2.6.1. Null hypothesis significance testing 2.6.2. Problems with NHST 2.7. Modern approaches to theory testing 2.7.1. Effect sizes 2.7.2. Meta-analysis at will this chapter tell me? Versions of IBM SPSS Statistics Windows versus MacOS Getting started The data editor 3.5.1. Entering data into the data editor 3.5.2. The variable view 3.5.3. Missing values .Importing data The SPSS viewer Exporting SPSS output The syntax editor Saving files Retrieving a file Brian’s attempt to woo Jane What next? Key terms that I’ve discoveredSmart Alex’s tasksFurther reading4 Exploring data with graphs4.1. What will this chapter tell me? 4.2. The art of presenting data 4.2.1. What makes a good graph? 4.2.2. Lies, damned lies, and erm graphs 00-Field 4e-SPSS-Prelims.indd 12012112112212212312/11/2012 6:04:39 PM
viiC ONTENTS4.3.4.4.4.5.4.6.The SPSS chart builder Histograms Boxplots (box–whisker diagrams) Graphing means: bar charts and error bars 4.6.1.4.6.2.4.6.3.4.6.4.4 4.6.5.Simple bar charts for independent means Clustered bar charts for independent means Simple bar charts for related means Clustered bar charts for related means Clustered bar charts for ‘mixed’ designs 4.7. Line charts 4.8. Graphing relationships: the scatterplot .4.12.4.13.4.14.Simple scatterplot Grouped scatterplot Simple and grouped 3-D scatterplots Matrix scatterplot Simple dot plot or density plot Drop-line graph Editing graphs Brian’s attempt to woo Jane What next? Key terms that I’ve discoveredSmart Alex’s tasksFurther reading5 The beast of bias5.1. What will this chapter tell me? 5.2. What is bias? 5.2.1.5.2.2.5.2.3.5.2.4.5.2.5.5.2.6.5.3Assumptions Outliers Additivity and linearity Normally distributed something or other Homoscedasticity/homogeneity of variance Independence Spotting bias 5.3.1. Spotting outliers 5.3.2. Spotting normality 5.3.3. Spotting linearity and heteroscedasticity/heterogeneityof variance 5.4. Reducing bias 5.4.1.5.4.2.5 5.4.3.5.4.4.5.5.5.6.5.7.5.8.5.9.Trimming the data Winsorizing Robust methods Transforming data Brian’s attempt to woo Jane What next? Key terms that I’ve discoveredSmart Alex’s tasksFurther reading6 Non-parametric models6.1. What will this chapter tell me? 6.2. When to use non-parametric tests 00-Field 4e-SPSS-Prelims.indd /11/2012 6:04:39 PM
viiiD I S C O VE R I N G STAT I ST I C S US I N G S P SS6.3. General procedure of non-parametric tests in SPSS 6.4. Comparing two independent conditions: the Wilcoxon rank-sum test andMann–Whitney test 6.4.1.6.4.2.6.4.3.6.4.4.6.4.5.6.4.6.Theory Inputting data and provisional analysis The Mann–Whitney test using SPSS Output from the Mann–Whitney test Calculating an effect size Writing the results 6.5. C omparing two related conditions:the Wilcoxon signed-rank test 6.5.1.6.5.2.6.5.3.6.5.4.6.5.5.6.5.6.Theory of the Wilcoxon signed-rank test Running the analysis Output for the ecstasy group Output for the alcohol group Calculating an effect size Writing the results 6.6. Differences between several independent groups: the Kruskal–Wallis test eory of the Kruskal–Wallis test Follow-up analysis Inputting data and provisional analysis Doing the Kruskal–Wallis test in SPSS Output from the Kruskal–Wallis test Testing for trends: the Jonckheere–Terpstra test Calculating an effect size Writing and interpreting the results 6.7. Differences between several related groups: Friedman’s ANOVA 6.10.6.11.6.12.Theory of Friedman’s ANOVA Inputting data and provisional analysis Doing Friedman’s ANOVA in SPSS Output from Friedman’s ANOVA Following-up Friedman’s ANOVA Calculating an effect size Writing and interpreting the results Brian’s attempt to woo Jane What next? Key terms that I’ve discoveredSmart Alex’s tasksFurther 2572582592592592617 Correlation 2627.1. What will this chapter tell me? 7.2. Modelling relationships 7.2.1.7.2.2.7.2.3.7.2.4.7.2.5.A detour into the murky world of covariance Standardization and the correlation coefficient The significance of the correlation coefficient Confidence intervals for r A word of warning about interpretation: causality 7.3. Data entry for correlation analysis using SPSS 7.4. Bivariate correlation 7.4.1. General procedure for running correlations in SPSS 7.4.2. Pearson’s correlation coefficient 7.4.3. Spearman’s correlation coefficient 00-Field 4e-SPSS-Prelims.indd 826226326426626826927027027127227427612/11/2012 6:04:40 PM
ixC ONTENTS7.4.4. Kendall’s tau (non-parametric) 7.4.5. Biserial and point-biserial correlations 7.5. Partial correlation 7.5.1. The theory behind part and partial correlation 7.5.2. Partial correlation in SPSS 7.5.3. Semi-partial (or part) correlations 7.6. Comparing correlations 7.6.1. Comparing independent rs 7.6.2. Comparing dependent rs 7.7.7.8.7.9.7.10.7.11.7.12.7.13.Calculating the effect size How to report correlation coefficients Brian’s attempt to woo Jane What next? Key terms that I’ve discoveredSmart Alex’s tasksFurther reading8 Regression8.1. What will this chapter tell me? 8.2. An introduction to regression 8.2.1.8.2.2.8.2.3.8.2.4.8.2.5.The simple linear model The linear model with several predictors Estimating the model Assessing the goodness of fit, sums of squares, R and R2 Assessing individual predictors 8.3. Bias in regression models? 8.3.1. Is the model biased by unusual cases? 8.3.2. Generalizing the model 8.3.3. Sample size in regression 8.4. Regression using SPSS: One Predictor 8.4.1.8.4.2.8.4.3.8.4.4.Regression: the general procedure Running a simple regression using SPSS Interpreting a simple regression Using the model 8.5. Multiple regression 8.5.1. Methods of regression 8.5.2. Comparing models 8.5.3. Multicollinearity 8.6. Regression with several predictors using SPSS 8.6.1.8.6.2.8.6.3.8.6.4.8.6.5.8.6.6.Main options Statistics Regression plots Saving regression diagnostics Further options Robust regression 8.7. Interpreting multiple regression 8.7.1.8.7.2.8.7.3.8.7.4.8.7.5.8.7.6.8.7.7.00-Field 4e-SPSS-Prelims.indd 9Descriptives Summary of model Model parameters Excluded variables Assessing multicollinearity Bias in the model: casewise diagnostics Bias in the model: assumptions 34534812/11/2012 6:04:40 PM
xD I S C O VE R I N G STAT I ST I C S US I N G S P SS8.8.8.9.8.10.8.11.8.12.8.13.8.14. hat if I violate an assumption? Robust regression WHow to report multiple regression Brian’s attempt to woo Jane What next? Key terms that I’ve discoveredSmart Alex’s tasksFurther reading9 Comparing two means9.1. What will this chapter tell me? 9.2. Looking at differences 9.2.1. An example: are invisible people mischievous? 9.2.2. Categorical predictors in the linear model 9.3. The t-test 9.3.1. Rationale for the t-test 9.3.2. The independent t-test equation explained 9.3.3. The matched-samples t-test equation explained 9.4. Assumptions of the t-test 9.5. The independent t-test using SPSS 9.5.1.9.5.2.9.5.3.9.5.4.9.5.5.9.5.6.The general procedure Exploring data and testing assumptions Compute the independent t-test Output from the independent t-test Calculating the effect size Reporting the independent t-test 9.6. Matched-samples t-test using SPSS 1.9.12.9.13.Entering data Exploring data and testing assumptions Computing the paired-samples t-test Calculating the effect size Reporting the paired-samples t-test Between groups or repeated measures? What if I violate the test assumptions? Brian’s attempt to woo Jane What next? Key terms that I’ve discoveredSmart Alex’s tasksFurther reading10 Moderation, mediation and more regression10.1. What will this chapter tell me? 10.2. Installing custom dialog boxes in SPSS 10.3. Moderation: interactions in regression 0.3.8.The conceptual model The statistical model Centring variables Creating interaction variables Following up an interaction effect Running the analysis Output from moderation analysis Reporting moderation analysis 10.4. Mediation 00-Field 4e-SPSS-Prelims.indd 0812/11/2012 6:04:40 PM
xiC he conceptual model The statistical model Effect sizes of mediation Running the analysis Output from mediation analysis Reporting mediation analysis 10.5. Categorical predictors in regression 10.5.1. Dummy coding 10.5.2. SPSS output for dummy variables 10.6. Brian’s attempt to woo Jane 10.7. What next? 10.8. Key terms that I’ve discovered10.9. Smart Alex’s tasks10.10. Further reading11 Comparing several means: ANOVA (GLM 1)11.1. What will this chapter tell me? 11.2. The theory behind ANOVA 1.2.8.Using a linear model to compare means Logic of the F-ratio Total sum of squares (SST) Model sum of squares (SSM) Residual sum of squares(SSR) Mean squares The F-ratio Interpreting F 11.3. Assumptions of ANOVA 11.3.1. Homogeneity of variance 11.3.2. Is ANOVA robust? 11.3.3. What to do when assumptions are violated 11.4. Planned contrasts 11.4.1.11.4.2.11.4.3.11.4.4.11.4.5.Choosing which contrasts to do Defining contrasts using weights Non-orthogonal comparisons Standard contrasts Polynomial contrasts: trend analysis 11.5. Post hoc procedures 11.5.1. Type I and Type II error rates for post hoc tests 11.5.2. Are post hoc procedures robust? 11.5.3. Summary of post hoc procedures 11.6. Running one-way ANOVA in SPSS 11.6.1.11.6.2.11.6.3.11.6.4.11.6.5.General procedure of one-way ANOVA Planned comparisons using SPSS Post hoc tests in SPSS Options Bootstrapping 11.7. Output from one-way ANOVA 11.7.1. Output for the main analysis 11.7.2. Output for planned comparisons 11.7.3. Output for post hoc tests 11.8. Calculating the effect size 11.9. Reporting results from one-way independent ANOVA 11.10.Key terms that I’ve discovered00-Field 4e-SPSS-Prelims.indd 7247447512/11/2012 6:04:40 PM
xiiD I S C O VE R I N G STAT I ST I C S US I N G S P SS11.11.Brian’s attempt to woo Jane 11.12.What next? 11.13.Smart Alex’s tasks11.14. Further reading12 Analysis of covariance, ANCOVA (GLM 2)12.1. What will this chapter tell me? 12.2. What is ANCOVA? 12.3. Assumptions and issues in ANCOVA 12.3.1. Independence of the covariate and treatment effect 12.3.2. Homogeneity of regression slopes 1 12.3.3. What to do when assumptions are violated 12.4. Conducting ANCOVA in SPSS eneral procedure Inputting data Testing the independence of the treatment variable and covariate The main analysis ContrastsOther options Bootstrapping and plots 12.5. Interpreting the output from ANCOVA 12.5.1.12.5.2.12.5.3.12.5.4.What happens when the covariate is excluded? The main analysis Contrasts Interpreting the covariate 12.6. Testing the assumption of homogeneity of regression slopes 12.7. Calculating the effect size 12.8. Reporting results 12.9. Brian’s attempt to woo Jane 12.10. What next? 12.11. Key terms that I’ve discovered12.12.Smart Alex’s tasks12.13.Further reading13 Factorial ANOVA (GLM 3)13.1. What will this chapter tell me? 13.2. Theory of factorial ANOVA (independent designs) actorial designs Guess what? Factorial ANOVA is a linear model Two-way ANOVA: behind the scenes Total sums of squares (SST) Model sum of squares, SSM The residual sum of squares, SSR The F-ratios 13.3. Assumptions of factorial ANOVA 13.4. Factorial ANOVA using SPSS 13.4.1.13.4.2.13.4.3.13.4.4.13.4.5.13.4.6.00-Field 4e-SPSS-Prelims.indd 12General procedure for factorial ANOVA Entering the data and accessing the main dialog box Graphing interactions Contrasts Post hoc tests Bootstrapping and other options 12/11/2012 6:04:41 PM
xiiiC ONTENTS13.5. Output from factorial ANOVA 13.5.1.13.5.2.13.5.3.13.5.4.13.5.5.Levene’s test The main ANOVA table Contrasts Simple effects analysis Post hoc analysis 13.6. Interpreting interaction graphs 13.7. Calculating effect sizes 13.8. Reporting the results of two-way ANOVA 13.9. Brian’s attempt to woo Jane 13.10.What next? 13.11.Key terms that I’ve discovered13.12.Smart Alex’s tasks13.13.Further reading14 Repeated-measures designs (GLM 4)14.1. What will this chapter tell me? 14.2. Introduction to repeated-measures designs 14.2.1.14.2.2.14.2.3.14.2.4.14.2.5.The assumption of sphericity How is sphericity measured? Assessing the severity of departures from sphericity What is the effect of violating the assumption of sphericity? What do you do if you violate sphericity? 14.3. Theory of one-way repeated-measures ANOVA 14.3.1. The total sum of squares, SST 14.3.2. The within-participant sum of squares, SSW 14.3.3. The model sum of squares, SSM 14.3.4. The residual sum of squares, SSR 14.3.5. The mean squares 14.3.6. The F-ratio 14.3.7. The between-participants sum of squares 14.4. Assumptions in repeated-measures ANOVA 14.5. One-way repeated-measures ANOVA using SPSS 14.5.1.14.5.2.14.5.3.14.5.4.Repeated-measures ANOVA: the general procedure The main analysis Defining contrasts for repeated measures Post hoc tests and additional options 14.6. Output for one-way repeated-measures ANOVA 14.6.1. Descriptives and other diagnostics 14.6.2. Assessing and correcting for sphericity: Mauchly’s test 14.6.3. The main ANOVA 14.6.4. Contrasts 14.6.5. Post hoc tests 14.7. Effect sizes for repeated-measures ANOVA 14.8. Reporting one-way repeated-measures ANOVA 14.9. Factorial repeated-measures designs 14.9.1.14.9.2.14.9.3.14.9.4.14.9.5.The main analysis Contrasts Simple effects analysis Graphing interactions Other options 14.10. Output for factorial repeated-measures ANOVA 00-Field 4e-SPSS-Prelims.indd 7457612/11/2012 6:04:41 PM
xivD I S C O VE R I N G STAT I ST I C S US I N G S P SS14.10.1. Descriptives and main analysis 14.10.2. Contrasts for repeated-measures variables 14.11.Effect sizes for factorial repeated-measures ANOVA 14.12.Reporting the results from factorial repeated-measures ANOVA 14.13.Brian’s attempt to woo Jane 14.14.What next? 14.15.Key terms that I’ve discovered14.16.Smart Alex’s tasks14.17.Further reading15 Mixed design ANOVA (GLM 5)15.115.2.15.3.15.4.15.5.What will this chapter tell me? Mixed designs Assumptions in mixed designs What do men and women look for in a partner? Mixed ANOVA in SPSS 15.5.1.15.5.2.15.5.3.15.5.4.Mixed ANOVA: the general procedure Entering data The main analysis Other options 15.6. Output for mixed factorial ANOVA 15.6.1. The main effect of gender 15.6.2. The main effect of looks 15.6.3. The main effect of charisma 15.6.4. The interaction between gender and looks 15.6.5. The interaction between gender and charisma 15.6.6. The interaction between attractiveness and charisma 15.6.7. The interaction between looks, charisma and gender 15.6.8. Conclusions 15.7. Calculating effect sizes 15.8. Reporting the results of mixed ANOVA 15.9. Brian’s attempt to woo Jane 15.10.What next? 15.11.Key terms that I’ve discovered15.12.Smart Alex’s tasks15.13.Further reading16 Multivariate analysis of variance (MANOVA)16.1. What will this chapter tell me? 16.2. When to use MANOVA 16.3. Introduction16.3.1. Similarities to and differences from ANOVA 16.3.2. Choosing outcomes 16.3.3. The example for this chapter 16.4. Theory of MANOVA 16.4.1.16.4.2.16.4.3.16.4.4.Introduction to matrices Some important matrices and their functions Calculating MANOVA by hand: a worked example Principle of the MANOVA test statistic 16.5. Practical issues when conducting MANOVA 16.5.1. Assumptions and how to check them 00-Field 4e-SPSS-Prelims.indd 2/11/2012 6:04:41 PM
xvC ONTENTS16.5.2. What to do when assumptions are violated 16.5.3. Choosing a test statistic 16.5.4. Follow-up analysis 16.6. MANOVA using SPSS 16.6.1.16.6.2.16.6.3.16.6.4.General procedure of one-way ANOVA The main analysis Multiple comparisons in MANOVA Additional options 16.7. Output from MANOVA 16.7.1.16.7.2.16.7.3.16.7.4.16.7.5.Preliminary analysis and testing assumptions MANOVA test statistics Univariate test statistics SSCP matrices Contrasts 16.8. Reporting results from MANOVA 16.9. Following up MANOVA with discriminant analysis 16.10.Output from the discriminant analysis 16.11.Reporting results from discriminant analysis 16.12.The final interpretation 16.13.Brian’s attempt to woo Jane 16.14.What next? 16.15.Key terms that I’ve discovered16.16.Smart Alex’s tasks16.17.Further reading17 Exploratory factor analysis17.1. What will this chapter tell me? 17.2. When to use factor analysis 17.3. Factors and components 17.3.1. Graphical representation 17.3.2. Mathematical representation 17.3.3. Factor scores 17.4. Discovering factors 17.4.1.17.4.2.17.4.3.17.4.4.17.4.5.17.4.6.Choosing a method Communality Factor analysis or PCA? Theory behind PCA Factor extraction: eigenvalues and the scree plot Improving interpretation: factor rotation 17.5. Research example 17.5.1. General procedure 17.5.2. Before you begin 17.6. Running the analysis 17.6.1.17.6.2.17.6.3.17.6.4.Factor extraction in SPSS Rotation Scores Options 17.7. Interpreting output from SPSS 17.7.1.17.7.2.17.7.3.17.7.4.17.7.5.00-Field 4e-SPSS-Prelims.indd 15Preliminary analysis Factor extraction Factor rotation Factor scores Summary 70370512/11/2012 6:04:41 PM
xviD I S C O VE R I N G STAT I ST I C S US I N G S P SS17.8. How to report factor analysis 17.9. Reliability analysis 17.9.1.17.9.2.17.9.3.17.9.4.Measures of reliability Interpreting Cronbach’s a (some cautionary tales) Reliability analysis in SPSS Reliability analysis output 17.10.How to report reliability analysis 17.11.Brian’s attempt to woo Jane 17.12.What next? 17.13.Key terms that I’ve discovered17.14.Smart Alex’s tasks17.15.Further reading18 Categorical data18.1. What will this chapter tell me? 18.2. Analysing categorical data 18.3. Theory of analysing categorical data ��s chi-square test Fisher’s exact test The likelihood ratio Yates’s correction Other measures of association Several categorical variables: loglinear analysis 18.4. Assumptions when analysing categorical data 18.4.1. Independence 18.4.2. Expected frequencies 18.4.3. More doom and gloom 18.5. Doing chi-square in SPSS 18.5.1. General procedure for analysing categorical outcomes 18.5.2. Entering data 18.5.3. Running the analysis 18.5.4. Output for the chi-square test 18.5.5. Breaking down a significant chi-square test with standardized residuals 18.5.6. Calculating an effect size 18.5.7. Reporting the results of chi-square 18.6. Loglinear analysis using SPSS 18.6.1.18.6.2.18.6.3.18.6.4.Initial considerations Running loglinear analysis Output from loglinear analysis Following up loglinear analysis 18.7. Effect sizes in loglinear analysis 18.8. Reporting the results of loglinear analysis 18.9. Brian’s attempt to woo Jane 18.10.What next? 18.11.Key terms that I’ve discovered18.12.Smart Alex’s tasks18.13.Further reading19 Logistic regression19.1. What will this chapter tell me? 19.2. Background to logistic regression 00-Field 4e-SPSS-Prelims.indd 2/11/2012 6:04:42 PM
xviiC ONTENTS19.3. What are the principles behind logistic regression? g the model: the log-likelihood statistic Assessing the model: the deviance statistic Assessing the model: R and R2 Assessing the contribution of predictors: the Wald statistic The odds ratio: exp(B) Model building and parsimony 19.4. Sources of bias and common problems 19.4.1. Assumptions 19.4.2. Incomplete information from the predictors 19.4.3. Complete separation 19.4.4. Overdispersion 19.5. Binary logistic regression: an example that will make you feel eel 19.5.1. Building a model 19.5.2. Logistic regression: the general procedure 19.5.3. Data entry 19.5.4. Building the models in SPSS 19.5.5. Method of regression 19.5.6. Categorical predictors 19.5.7. Comparing the models 19.5.8. Rerunning the model 19.5.9. Obtaining residuals 19.5.10. Further options 19.5.11. Bootstrapping 19.6. Interpreting logistic regression 19.6.1. Block 0 19.6.2.19.6.3.19.6.4.19.6.5.Model summary Listing predicted probabilities Interpreting residuals Calculating the effect size 19.7. How to report logistic regression 19.8. Testing assumptions: another example 19.8.1. Testing for linearity of the logit 19.8.2. Testing for multicollinearity 19.9. Predicting several categories: multinomial logistic regression 19.9.1.19.9.2.19.9.3.19.9.4.19.9.5.Running multinomial logistic regression in SPSS Statistics Other options Interpreting the multinomial logistic regression output Reporting the results 19.10.Brian’s attempt to woo Jane 19.11.What next? 19.12.Key terms that I’ve discovered19.13.Smart Alex’s tasks19.14.Further reading20 Multilevel linear models20.1. What will this chapter tell me? 20.2. Hierarchical data 20.2.1. The intraclass correlation 20.2.2. Benefits of multilevel models 00-Field 4e-SPSS-Prelims.indd 1812/11/2012 6:04:42 PM
xviiiD I S C O VE R I N G STAT I ST I C S US I N G S P SS20.3 Theory of multilevel linear models 20.3.1. An example 20.3.2. Fixed and random coefficients 20.4 The multilevel model 20.4.1. Assessing the fit and comparing multilevel models 20.4.2. Types of covariance structures 20.5 Some practical issues 20.5.1.20.5.2.20.5.3.20.5.4.Assumptions Robust multilevel models Sample size and power Centring predictors 20.6 Multilevel modelling using SPSS 20.6.1.20.6.2.20.6.3.20.6.4.20.6.5.20.6.6.Entering the data Ignoring the data structure: ANOVA Ignoring the data structure: ANCOVA Factoring in the data structure: random intercepts Factoring in the data structure: random intercepts and slopes Adding an interaction to the model 20.7. Growth models 20.7.1.20.7.2.20.7.3.20.7.4.20.7.5.Growth curves (polynomials) An example: the honeymoon period Restructuring the data Running a growth model on SPSS Further analysis 20.8. How to report a multilevel model 20.9. A message from the octopus of inescapable despair 20.10.Brian’s attempt to woo Jane 20.11.What next? 20.12.Key terms that I’ve discovered20.13.Smart Alex’s tasks20.14.Further reading00-Field 4e-SPSS-Prelims.indd 84184484985085185385486086286386486486586586621 Epilogue: life after discovering statistics86721.1. Nice emails21.2. Everybody thinks that I’m a statistician21.3. Craziness on a grand scale21.3.1. Catistics21.3.2. Cult of underlying numerical truths21.3.3. And then it got really ences899Index90812/11/2012 6:04:42 PM
P R E FA C EKarma Police, arrest this man, he talks in maths, he buzzes like a fridge, he’s likea detuned radioRadiohead, ‘Karma Police’, OK Computer (1997)IntroductionMany behavioural and social science students (and researchers for that matter) despisestatistics. Most of us have a non-mathematical background, which makes understandingcomplex statistical equations very difficult. Nevertheless, the evil goat-warriors of Satanforce our non-mathematical brains to apply themselves to what is the very complex taskof becoming a statistics expert. The end result, as you might expect, can be quite messy.The one weapon that we have is the computer, which allows us to neatly circumvent theconsiderable disability of not understanding mathematics. Computer programs such asIBM SPSS Statistics, SAS, R and the like provide an opportunity to teach statistics at aconceptual level without getting too bogged down in equations. The computer to a goatwarrior of Satan is like catnip to a cat: it makes them rub their heads along the groundand purr and dribble ceaselessly. The only downside of the computer is that it makes itreal
7.3. Data entry for correlation analysis using SPSS 270 7.4. Bivariate correlation 271 7.4.1. General procedure for running correlations in SPSS 272 7.4.2. Pearson’s correlation coefficient 274 7.4.3. Spearman’s correlation coefficie