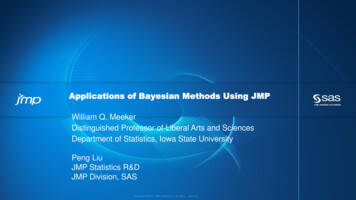
Transcription
Applications of Bayesian Methods Using JMPWilliam Q. MeekerDistinguished Professor of Liberal Arts and SciencesDepartment of Statistics, Iowa State UniversityPeng LiuJMP Statistics R&DJMP Division, SASCopyright 2013, SAS Institute Inc. All rightsreserved.
Bayesian Methods in JMPOverview Introduction to Bayesian statistical methods in JMP Reliability examples Bearing cage field-failure data analysis Rocket motor field data analysis An accelerated test to estimate telecommunications laser lifetime An accelerated test to estimate the life time of a new-technology ICprocessorConcluding remarksCopyright 2013, SAS Institute Inc. All rightsreserved.
Bayesian Methods in JMPReliability Probability that a system, vehicle, machine, device, and so on,will perform its intended function under encountered operatingconditions, for a specified period of time (Meeker and Escobar1998) Quality over time (Condra 1993) Failure avoidance A highly quantitative engineering discipline, often requiringcomplicated statistical and probabilistic analysesCopyright 2013, SAS Institute Inc. All rightsreserved.
Bayesian Methods in JMPBayesian Statistical Methods Over the past 30 years, there has been a revolution in theworld of statistical modeling and data analysis. Bayesianmethods are now widely used in many areas of application. Reasons for the revolution Rediscovery and further developments of Markov-chainMonte Carlo (MCMC) methods Spectacular improvements in computing power Development of relatively easy-to-use software (BUGS,Stan, SAS proc MCMC, and now JMP) Motivation for using Bayesian methods Provides a means combine prior information with limiteddata leading to improved inferences Modeling and analysis using Bayesian methods for somecomplicated models is easier than using classical methodslike maximum likelihood Downside? “You have to think.”Copyright 2013, SAS Institute Inc. All rightsreserved.
Bayesian Methods in JMPAircraft Engine Bearing CageField-Failure Data Data from the Weibull Handbook (1984) 1703 units had been introduced into the field over time;oldest unit at 2220 hours of operation. Design life specification was B10 8000 hours of operation 6 units had failed Do we have a serious problem? Re-design needed?
Bayesian Methods in JMPBearing Cage Field-Failure Data Event Plot
Bayesian Methods in JMPBearing Cage Weibull Maximum Likelihood Fitting
Bayesian Methods in JMPLikelihood InferenceModel forDataLikelihoodDataInference
Bayesian Methods in JMPBayesian InferenceModel emPosteriorDistributionInference
Bayesian Methods in JMPBearing Cage Field Data Joint Prior DistributionPrior for B10: Weakly informative B10 Lognormal 1000, 50,000 Prior for Weibull shape β: Informative: β Truncated Normal 1.5, 3 Note: JMP does not have TNORM, Normal 1.5, 3 is equivalent to TNORM 1.5, 3 here, with negligible differences.
Bayesian Methods in JMPBearing Cage Field DataComparison of Joint Prior and Posterior Distributions
Bayesian Methods in JMPBearing Cage Field DataComparison of ML and Bayesian InferencesMaximum LikelihoodBayesian with Informative Prior for β
Bayesian Methods in JMPLessons Learned With a small number of failures, not much can be said about reliability Engineers often have information about the Weibull shape parameter,based on knowledge of the failure mechanism Using the prior information will often lead to improved, more usefulinferences Bayesian methods provide a formal statistical method to combineinformation from different sourcesCopyright 2013, SAS Institute Inc. All rightsreserved.
Bayesian Methods in JMPRocket Motor Field Data Analysis Rocket motor is one of five critical missile components Approximately 20,000 missiles in inventory 1,940 firings over the life of the missile; catastrophic motor failuresfor three older missiles Failures thought to be due to thermal cycling, but only ageinformation is available Failure times not directly observed (1,937 right censored, 3 leftcensored observations) Concern about a possible wearout failure mode and thedistribution of remaining life of the stockpileCopyright 2013, SAS Institute Inc. All rightsreserved.
Bayesian Methods in JMPRocket Motor Lifetime Data
Bayesian Methods in JMPRocket Motor Life Data AnalysisEvent PlotWeibull Probability Plot
Bayesian Methods in JMPRocket Motor Weibull ML AnalysisWeibull shape parameter estimatewas 8.1 (very large)
Bayesian Methods in JMPRocket Motor Bayesian Weibull Analysis The ML estimate of beta 8.1 was much larger than expected. Estimate of fraction failing at 20 years was 0.46 LR Confidence Interval (0.023, 0.9999); not useful Prior distributions: Informative: β Truncated Normal 1, 5 Weakly informative: B10 Truncated Normal 5, 400 10,000 MCMC draws obtained from the joint posterior distributionCopyright 2013, SAS Institute Inc. All rightsreserved.
Bayesian Methods in JMPRocket Motor Comparison of the Likelihood Contours andSamples from the Prior and Posterior Distributions UsingPrior Information on βPrior SamplePosterior SampleCopyright 2013, SAS Institute Inc. All rightsreserved.
Bayesian Methods in JMPRocket Motor Comparison of ML Estimates and BayesianEstimates Using Prior Information on βMaximum LikelihoodBayesian
Bayesian Methods in JMPLessons Learned Even when no actual failure times are observed, there is still reliabilityinformation in the data. With very few failures, there is little information in the data The limited information can be supplemented by using knowledgeabout the failure mode and other engineering information JMP’s Bayesian analysis tools make Bayesian analyses easy.Copyright 2013, SAS Institute Inc. All rightsreserved.
Bayesian Methods in JMPAccelerated Testing In product design, engineers need to obtain reliabilityinformation quickly Test units at high levels of temperature, voltage, stress,or other “accelerating variable” to get reliabilityinformation quickly Use a physically-motivated model to extrapolate to useconditions Extrapolation is dangerous; assumed model may nothold outside the range of the dataCopyright 2013, SAS Institute Inc. All rightsreserved.
Bayesian Methods in JMPAccelerated Life Test of a Laser Data from Hooper and Amster (1990) Accelerated Life Test with temperature acceleration at 40 C, 60 C, and 80 C Units tested at use conditions 10 C, but none failed Test lasted 5,000 hours Interest is in estimating fraction failing at 30,000 hours ( 3.5 years) at 10 C.Copyright 2013, SAS Institute Inc. All rightsreserved.
Bayesian Methods in JMPSeparate distributionsFit Life by X Multiple Probability Plots toAssess Model AdequacySeparate distributions;common slopeArrhenius model
Bayesian Methods in JMPArrhenius Model Fit to the Laser DataNote: Arrhenius constant has been updated with more significant digits in JMP16.This would not change existing conclusions that used 110605.
Bayesian Methods in JMPLaser Lifetime Distribution Profilers to EstimateFraction Failing at 10 C 30,000 Hours
Bayesian Methods in JMPLaser Lifetime Bayesian Analysis
Bayesian Methods in JMPLaser Lifetime Comparison of Fraction Failing at10 C 30,000 Hours ML Estimates and BayesianEstimates Using Prior Information on β1Maximum LikelihoodBayesian
Bayesian Methods in JMPLessons Learned Accelerated life tests provide reliability information quickly Engineers often have information about the effectiveactivation energy that can be used to improve precision(or reduce cost through the use of smaller sample sizes). Bayesian methods provide an appropriate method tocombine the engineer’s information with the ALT data.Copyright 2013, SAS Institute Inc. All rightsreserved.
Bayesian Methods in JMPAnalysis of Interval-Censored ALT datafor a New-Technology IC Device An accelerated life test was run to evaluate the life time of a newprocessor IC device Tests run at 150, 175, 200, 250, and 300 degrees C Interval-censored data Failure only at 250 and 300 degrees C Developers interested in estimating the 0.01 Quantile of the lifedistribution at 100 degrees C
Bayesian Methods in JMP
Bayesian Methods in JMPML Estimation for the New-Technology IC DeviceFailures at 300C were caused by a different failure mechanism that would never be seen atuse conditions. Need to drop those data.
Bayesian Methods in JMPML Estimation for the New-Technology IC DeviceUsing only the 250 C DataWith only one temperature level, there is notenough information to fit the ALT regression model
Bayesian Methods in JMPBayesian Estimation Joint Posterior Distributions for the New-Technology IC DeviceUsing only the 250 C Data with Prior Information for the Activation Energy
Bayesian Methods in JMPBayesian Estimation for the New-Technology IC Device Using Onlythe 250 C Data with Prior Information for the Activation EnergyMaximum likelihood estimates with bad data
Bayesian Methods in JMPLessons Learned In some applications, interval censoring arises. Appropriate statisticalmethods exist for handling such data. Using excessive levels of an accelerating variable is likely to causefailures from mechanisms that will never be active in actual use Even with failures at only one level of temperature, we can estimatelife at the use conditions (and quantify statistical uncertainty) if wehave prior information about the effective activation energy (slope ofthe regression line) and use Bayesian methods.Copyright 2013, SAS Institute Inc. All rightsreserved.
Bayesian Methods in JMPConcluding Remarks Improvements in computing hardware and software have greatlyadvanced our ability to analyze reliability data. The use of Bayesian methods will continue to increase, allowing theeffective use of available engineering information to improve theprecision of reliability inferences and to reduce the costs in reliabilitytesting. JMP already has powerful tools for applying Bayesian methods in lifedata analysis (Life Distribution) and accelerated testing (Fit Life by X). Although these Bayesian capabilities are in the reliability part of JMP,they can certainly be used for non-reliability applications.Copyright 2013, SAS Institute Inc. All rightsreserved.
Bayesian Methods in JMPReferencesLi, M. and W.Q. Meeker (2014) Application of BayesianMethods in Reliability Data Analyses. The Journal of QualityTechnology, 46, 1–23.Meeker, W. Q., L. A. Escobar, and F. Pascual (2021),Statistical Methods for Reliability Data, Second Edition, JohnWiley and Sons, New York.Copyright 2013, SAS Institute Inc. All rightsreserved.
Bayesian Methods in JMPJMP ImplementationA two-stage algorithm:1. Simple Rejection AlgorithmTried-and-true; impractical if rejection rate is high.2. Random Walk Metropolis–Hastings AlgorithmEfficient; fail undetectably if the likelihood is “irregular”, e.g. flat. When there are few or no failures, the likelihood is relatively flat.Rejection rate is not bad, and simple rejection suffices. When there are more failures, the likelihood becomes more“regular”. Simple rejection becomes impractical, and random walkMH is more promising to succeed.Copyright 2013, SAS Institute Inc. All rightsreserved.
Bayesian Methods in JMPJMP ImplementationSpecify truncated normal prior in JMP.1. JMP does not have built-in truncated normal prior now.2. An equivalent normal prior can be used for Quantile, if the distribution belongs to log-location-scale family. 𝜎 or Weibull 𝛽Because negative values will be thrown out automatically.E.g.TNorm 5, 400 Norm -75, 399 Supplement script:tnorm config to norm config.jslCopyright 2013, SAS Institute Inc. All rightsreserved.
JMP already has powerful tools for applying Bayesian methods in life data analysis (Life Distribution) and accelerated testing (Fit Life by X). Although these Bayesian capabilities are in the reliability part of JMP, they can certainly be used for non-reliability applications. Bayesian Methods in JMP