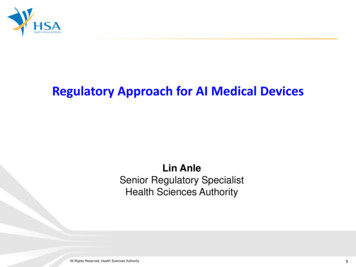
Transcription
Regulatory Approach for AI Medical DevicesLin AnleSenior Regulatory SpecialistHealth Sciences AuthorityAll Rights Reserved, Health Sciences Authority1
HSA’s Role inHealth Products RegulationOur Role Ensure that pharmaceuticals, biologics, medical devices and health-related productsin Singapore are wisely regulated to meet appropriate standards of safety, quality andefficacy throughout the product life cycle Ensure timely access to good quality & safe health products Support the health and biomedical sciences industry and facilitating its developmentOur Regulatory Philosophy1Benefits outweigh foreseeable risks2Risk-based approach453Confidence-based approachAll Rights Reserved, Health Sciences AuthorityAdoption and judicious adaption ofinternational standards & bestpracticesForging strategic partnership bothregionallyinASEANandinternationally2
AI is now enabling new possibilities in healthcareAll Rights Reserved, Health Sciences Authority3
Use Cases of AI in HealthcareAll Rights Reserved, Health Sciences Authority4
AI In HealthcareThere are three significant changes which AI brings:1. Increasing complexity of potential inputs and outputsa. Improving models and algorithms enables AI to perform increasingly complex tasks, whichoften involve multiple complicated inputs, and a significant variety of potential outputs2. Increasing potential for automation and the removal of direct human supervisiona. As AI gets better at performing more complex tasks, it increases the potential to completelyremove the need for humans to be involved in medical process3. Ability to constantly learn and evolvea. New developments in AI involve algorithms which are capable of continuously learning. Thiswould push the boundary of a medical device with clearly defined fixed inputs and outputs.All Rights Reserved, Health Sciences Authority5
Challenges AI/ML performance is dependent on the quality of the learning dataset that is used in training the engine Good quality data will improve the performance while poor quality data orinaccurate data may affect the device performance Data acquisition, data pre-processing. AI/ML unlike other software have the ability to continuously learn postdeployment during use With continuous learning, the performance of the AI/ML changes from what itwas originally validated May provide a different set of output/ resultAll Rights Reserved, Health Sciences Authority6
How do we regulate AI-MDs?All Rights Reserved, Health Sciences Authority7
“Medical Devices” DefinitionFirst Schedule of the Health Products Act 2007Any instrument, apparatus, implement, machine, appliance, implant, in vitro reagent or calibrator, software,material or other similar or related article that is intended by its manufacturer to be used, whether alone orin combination, for humans for one or more of the specific purposes of: —I.diagnosis, prevention, monitoring, treatment or alleviation of any disease;II. diagnosis, monitoring, treatment, alleviation of or compensation for an injury;III. investigation, replacement, modification, or support of the anatomy or of a physiological process;IV. supporting or sustaining life;V. control of conception;VI. disinfection of medical devices; orVII. providing information for medical or diagnostic purposes by means of in vitro examination ofspecimens derived from the human body,and which does not achieve its primary intended action in or on the human body by pharmacological,immunological or metabolic means, but which may be assisted in its intended function by such means;and(b) Includes the following articles:i.any implant for the modification or fixation of any body part;ii.any injectable dermal filler or mucous membrane filler;iii. any instrument, apparatus, implement, machine or appliance intended to be used for the removal ordegradation of fat by invasive means.All Rights Reserved, Health Sciences Authority8
What is a Medical Device?As a general rule, if the device is intended for medical purpose, such asinvestigation, detection, diagnosis, monitoring, treatment or management ofany medical condition, disease, anatomy or physiological process, the devicewill be classified as a medical deviceAll Rights Reserved, Health Sciences Authority9
MD Regulatory ControlsPre-marketPost-marketAll Rights Reserved, Health Sciences Authority10
Risk-based Regulatory Oversight MDs are categorized into 4 risk classes, Class A to Class D (Class A being thelowest risk) in line with the international rule-based classification systemControls stratified according to the device’s Risk ClassRisk ClassDCDealers Licence ISO 13485 /SS 620* Requireregistration usingCSDT*** Format Declaration ofconformity to aQMS**XDeclared underClass A MedicalDevice RegisterBAProductRegistrationPost-marketObligation * Singapore Standard SS 620 : 2016 – Good Distribution Practice for Medical Devices - Requirements** QMS - Quality Management System: ISO 13485 / SS 620 GDPMDS*** ASEAN Common Submission Dossier TemplateQuick Guide to Medical Device Product Registration and Dealer egulatory-overviewAll Rights Reserved, Health Sciences AuthorityQuick Guide11
Artificial Intelligence in Medical Devices AI that is intended for medical purposes (i.e. diagnosis, treatment,patient monitoring) are regulated by HSA as MDs (referred to as AIMDs)– AI that are used in hospitals solely for administrative functions (e.g. patientappointment scheduling) are not regulated by HSA as MDs AI-MDs are risk classified based on their intended purpose assignedby their manufacturer/developer (i.e. by design and by claims)– Functionalities and Features (e.g. analyse, monitor, adjust or controltherapy)– Output from the AI-MD (e.g. triage, recommend, diagnose, therapyrecommendations)– The risk classification approach and considerations are similar to othersoftware medical devices Algorithm-agnostic and Technology-agnosticRisk class tool: /risk-classification-ruleAll Rights Reserved, Health Sciences AuthorityRisk Classification Tool12
Risk Classification AI-MDsIncreasing riskAll Rights Reserved, Health Sciences Authority13
AI-MDs: Human InterventionThe degree of humanintervention with the AI /MLsoftware will not affect the riskclassification of the deviceExamplesDiagnostic tools thatshortlist/annotate relevantimages or test results forthe doctorSoftware analyseshistopathology images andidentifies and shortlists fewspecific images for thepathologist to review insteadof having to scan thenumerous images manuallyDiagnostic tools that analyseand provide diagnosticrecommendations to thedoctorSoftware that analyses thehistopathology images andhighlights abnormal sections inselected images and presentspossible outcomes/results forpathologist to considerAll Rights Reserved, Health Sciences AuthorityDiagnostic tools thatanalyse and providediagnostic outcomesindependentlySoftware which analyses thehistopathology images,identifies the abnormalsections in the images andgenerates a report withdiagnostic outcomesindependently14
Regulating AI-MDs –Product Lifecycle ApproachExperience gathered from Real World use is applied toimprove the next version or model of the MDPilot and Pivotalclinical studiesMedical Device Monitoring real worldRegistrationeffectiveness,(SMDR)Adverse Events andFSCA/Recalls AI-MDs are largely comparable to the “Software” that are alreadyregulated as medical devices Short lifecycle Iteratively modified and updated Ability to be used together with hardware devices or used by themselvesas standalone softwareAll Rights Reserved, Health Sciences Authority15
Regulating AI-MDsPre-market Evaluation of AI-MDs will be doneconsideration various factors such as:holistically,takinginto Intended purpose including the functionalities and output of the AI-MDs Built-in quality control mechanism as part of the design/workflow Level of human intervention in the workflow How the AI-MDs value-add to the clinical workflow (data analysis tool/diagnosis/ interpretation/ therapy recommendation) Risk of incorrect output and mitigation measures if any Continuous learning plan and version controls for next generation of AI-MDsAll Rights Reserved, Health Sciences Authority16
Regulating AI-MDsPre-market AI-MDs must define its boundary:AI-MDs Version 1.0Intended use andperformance specificationso Defining the intended use and functionalities What it can do and what it will not do Inputs and outputso Performance specifications and control measures(e.g. how the extremes/outliers are detected/managed) Based on the defined intended purpose and functionalities, softwareverification and validation tests are required to be performedo Demonstrate that the output meets the defined specifications under bothnormal and abnormal circumstances (e.g. when challenged with outliers)o Demonstrate that adequate control measures are in place to manage knownand foreseeable failure modesAll Rights Reserved, Health Sciences Authority17
Regulating AI-MDs Process of developing and deployment of the AI-MDs (LockedAlgorithm)Development/ Retraining/Deployment under Quality Management System ISO 13485All Rights Reserved, Health Sciences Authority18
Regulating AI-MDs Input data and features/attributes used togenerate the corresponding output Source and size of training, validation andtest dataset Data pre-processing Data labelling Any QC feature to ensure input data iswithin specification.Quality and Quantity of data What is good quality data? How much data is enough?All Rights Reserved, Health Sciences Authority19
Regulating AI-MDs Descriptiononthemachinelearning model used. Justificationfor selecting the model Model evaluation Versioning and configuration control– Traceability purposeTraceability An AI model is considered to be traceable if (a) its decisions,and (b) the datasets and processes that yield the AI model’sdecision, are documented in an easily understandable way Useful for investigation into why a particular prediction wasmadeAll Rights Reserved, Health Sciences Authority20
Regulating AI-MDs Performance specifications Test protocol and report for verificationand validation of the AI-MD, includingthe acceptance limits and informationon the anomalies identified Clinical Association between the AIMD’s output and clinical conditions(s)must be presented Clinical Evidence to support the intended clinical use of the AI-MDsAI-MDs with no human-intervention will have more stringent validationrequirement for pre-market registration, especially on its clinical evidenceAll Rights Reserved, Health Sciences Authority21
Regulating AI-MDs Mandatory Reporting– Serious Adverse Events, FieldSafety Actions & Recalls to HSA Real-world Performance– Monitoring post-deploymentperformance & reporting to HSA Deployment of updated /upgraded versions– Significant changes affectingperformance require HSA approvalAll Rights Reserved, Health Sciences Authority22
Additional Considerations for AI-MD withContinuous Learning Capabilities Process of developing and deployment of the AI-MDs (AdaptiveAlgorithm)Development/ Retraining/Deployment under Quality Management System ISO 13485All Rights Reserved, Health Sciences Authority23
Additional Considerations for AI-MD withContinuous Learning Capabilities Demonstrate process controls,verification, ongoing model monitoringmeasures Any safety mechanism to detectanomalies and any inconsistencies inthe output result and how these aremitigated Process to ensure no biasness in the learning process. Process to ensure data integrity, reliability and validity of the newdata setAll Rights Reserved, Health Sciences Authority24
Additional Considerations for AI-MD withContinuous Learning Capabilities Software version control Process to ensure traceability betweenreal world data for learning, learningprocess, software version number andthe AI-MD’s output during clinical use. Validation strategy and verification activities for continuous learningto ensure the performance is within the pre-defined boundaries /envelopeAI-MDs Version 1.0Intended use and expectedchanges in performancespecificationsAll Rights Reserved, Health Sciences Authority25
Benefit-Risk Considerations Should review and address allforeseeable risks and failuremodes of the software in itsproduct life cycle Where there are changes madeto a software, these should besystematicallyevaluatedtodetermine if any additional riskcould arise from these changes. Unknown Unknowns?A schematic representation of therisk management processAdapted from ISO 14971:2007 Medical devices -Application of risk management to medicaldevicesEnsure that residual risk is acceptableAll Rights Reserved, Health Sciences Authority26
Regulating AI-MDsPost-approval monitoring A post-approval condition is currently being imposed for AI-MDsrequiring the company to submit a summary report of allcomplaints / feedback received on the device performanceperiodically to HSAo Feedback/complaints relating to inaccurate or inconsistentoutput from the AI-MDo Closer monitoring of the AI-MD performance This is in additional to the AE/FSCA reportingAll Rights Reserved, Health Sciences Authority27
Regulating AI-MDsChanges to Registered AI-MDs Significant changes made to the AI-MDs (e.g. change in the type ofinput data), will require a Change Notification (CN) submission andapproval from HSA prior to supply. Requirements are aligned toour current approach for softwareAll Rights Reserved, Health Sciences Authority28
Change ManagementTechnical Change:For Class C/D medical devices whichenquires HSA’s approval prior toimplementation of the change(s) inSingapore.Review Change:For Class B medical devices whichenquires HSA’s approval prior toimplementation of the change(s) inSingapore.Notification Change:May be implemented immediately uponreceipt of the acknowledgement emailfrom HSA after submission.All Rights Reserved, Health Sciences Authority29
Change ManagementTechnical Change:For Class C/D medical devices whichenquires HSA’s approval prior toimplementation of the change(s) inSingapore.Review Change:For Class B medical devices whichenquires HSA’s approval prior toimplementation of the change(s) inSingapore.Notification Change:May be implemented immediately uponreceipt of the acknowledgement emailfrom HSA after submission.All Rights Reserved, Health Sciences Authority30
Regulating AI-MDs –Product Lifecycle ApproachExperience gathered from Real World use is applied toimprove the next version or model of the MDPilot and Pivotalclinical studiesAI-MDAI-MDDevelopment, Preclinical/ Analyticaland ClinicalvalidationPre-marketAll Rights Reserved, Health Sciences AuthorityMedical Device Monitoring real worldRegistrationeffectiveness,(SMDR)Adverse Events andFSCA/RecallsRegulatoryapproval from /Deployment ofupgraded versionPost-market31
AI-MDs RegulationRegulatory Guidelines for Software Medical Devices - A Life CycleApproachReference: cumentsAll Rights Reserved, Health Sciences AuthorityGuidance documents32
Medical Device Development ConsultationBefore regulatorysubmissionChannel for stakeholders to seek regulatoryadvice during medical device developmentphase to align with regulatory requirements.We have attended 13 Device DevelopmentConsultation for AI-MDsWe have approved 7 AI-MDs pre-marketapplication via FULL evaluation route*. All 7were submitted through our Priority ReviewScheme.SCOPE: Clarification on regulatoryrequirements applicable to thedevice in development, which mayinclude Regulatory strategy Regulatory requirements– Device claims– Safety / Performancestudies– Software verification andvalidation– Risk management– Clinical trials* Full Evaluation Route – MD with no prior approval from HSA’s referenceagencies (Australia TGA, Health Canada, EU, Japan MHLW, US FDA)Device Development Consultation: -schemesPriority Review Scheme: n/priority-reviewschemeAll Rights Reserved, Health Sciences AuthorityConsultationPriority Review33
THANK YOUFor Product Regulation related queries:All Rights Reserved, Health Sciences Authority34
patient monitoring) are regulated by HSA as MDs (referred to as AI-MDs) -AI that are used in hospitals solely for administrative functions (e.g. patient appointment scheduling) are not regulated by HSA as MDs AI-MDs are risk classified based on their intended purpose assigned by their manufacturer/developer (i.e. by design and by claims)