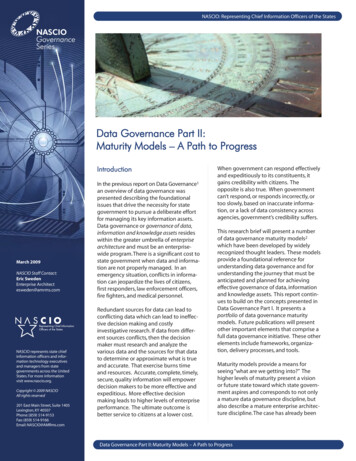
Transcription
NASCIO: Representing Chief Information Officers of the StatesData Governance Part II:Maturity Models – A Path to ProgressIntroductionMarch 2009NASCIO Staff Contact:Eric SwedenEnterprise Architectesweden@amrms.comNASCIO represents state chiefinformation officers and information technology executivesand managers from stategovernments across the UnitedStates. For more informationvisit www.nascio.org.Copyright 2009 NASCIOAll rights reserved201 East Main Street, Suite 1405Lexington, KY 40507Phone: (859) 514-9153Fax: (859) 514-9166Email: NASCIO@AMRms.comIn the previous report on Data Governance1an overview of data governance waspresented describing the foundationalissues that drive the necessity for stategovernment to pursue a deliberate effortfor managing its key information assets.Data governance or governance of data,information and knowledge assets resideswithin the greater umbrella of enterprisearchitecture and must be an enterprisewide program.There is a significant cost tostate government when data and information are not properly managed. In anemergency situation, conflicts in information can jeopardize the lives of citizens,first responders, law enforcement officers,fire fighters, and medical personnel.Redundant sources for data can lead toconflicting data which can lead to ineffective decision making and costlyinvestigative research. If data from different sources conflicts, then the decisionmaker must research and analyze thevarious data and the sources for that datato determine or approximate what is trueand accurate. That exercise burns timeand resources. Accurate, complete, timely,secure, quality information will empowerdecision makers to be more effective andexpeditious. More effective decisionmaking leads to higher levels of enterpriseperformance. The ultimate outcome isbetter service to citizens at a lower cost.When government can respond effectivelyand expeditiously to its constituents, itgains credibility with citizens. Theopposite is also true. When governmentcan’t respond, or responds incorrectly, ortoo slowly, based on inaccurate information, or a lack of data consistency acrossagencies, government’s credibility suffers.This research brief will present a numberof data governance maturity models2which have been developed by widelyrecognized thought leaders. These modelsprovide a foundational reference forunderstanding data governance and forunderstanding the journey that must beanticipated and planned for achievingeffective governance of data, informationand knowledge assets. This report continues to build on the concepts presented inData Governance Part I. It presents aportfolio of data governance maturitymodels. Future publications will presentother important elements that comprise afull data governance initiative. These otherelements include frameworks, organization, delivery processes, and tools.Maturity models provide a means forseeing “what are we getting into?” Thehigher levels of maturity present a visionor future state toward which state government aspires and corresponds to not onlya mature data governance discipline, butalso describe a mature enterprise architecture discipline.The case has already beenData Governance Part II: Maturity Models – A Path to Progress
NASCIO: Representing Chief Information Officers of the Statesmade in Data Governance Part I that stategovernment will never be able to effectively respond to citizens without properlygoverning its information and knowledgeassets.In early 2009, the states are under severeeconomic stress—major revenue shortfalls, growing deficits and reduced publicspending. State governments expectcontinued expenditure pressures from avariety of sources including Medicaid,employee pensions and infrastructure.Experts predict even more economictroubles for the states in fiscal year 2010and beyond. A key ingredient for establishing strategies for dealing withcontinuing fiscal crisis is the ability toeffectively harvest existing knowledgebases. Those knowledge bases mustprovide reliable, up to date information inorder to enable judgment, discernmentand intuition. These comprise what mightbe termed wisdom. Even with perfectinformation, wisdom is still required tomake the right decisions and to executeon those decisions. State leaders will beforced to make tough decisions in themonths ahead, certainly requiring wisdom.This research brief will focus on that firstkey ingredient—knowledge. So, stategovernment must make the commitmentto begin now to manage and govern itsinformation and knowledge assets.Maturity models assist in helping stategovernment prepare for the journey andthat is what this report is intended topresent. Governance will not happenovernight—it will take sustained effortand commitment from the entire enterprise.As state government moves up the maturity curve presented by these models, therewill be technological and business processramifications. However, nothing willcompare to the organizational fallout. Itwill take commitment and leadership fromexecutive management to bring the enterprise along in a way so that it will be apositive experience for governmentemployees and citizens. See NASCIO’spublication “Transforming Governmentthrough Change Management: The Role of2the State CIO” for further discussion oforganizational change management.3The growing importance of properlymanaging information and knowledgeassets is demonstrated by a number ofpredictions regarding data and datagovernance by the IBM Data GovernanceCouncil.4IBM Data Governance CouncilPredictions Data governance will become a regulatory requirement.Information assets will be treated as anasset and included on the balance sheet.Risk calculations will become morepervasive and automated.The role of the CIO will include responsibility for data quality.Individual employees will be required totake responsibility for governance.As described in the previous issue brief onthis subject the delivery process mustbegin with an understanding of what theend result will look like, and what value adata governance initiative will deliver tostate government. Value is defined byexecutive leadership and depends on thevision, mission, goals and objectivesexecutive leadership has established forthe state government enterprise. Thevalue delivery process must also providemethods and procedures for monitoringhow well state government is currentlyperforming and the incremental steps forreaching the desired level of performance.The process for establishing and sustaining an effective data governance programwill require employing the followingenablers: Strategic Intent: describes WHY datagovernance is of value, the end statethat government is trying to reach, andthe foundational policies that describethe motivation of executive leadership.This strategic intent should bedescribed in the enterprise businessData Governance Part II: Maturity Models – A Path to Progress
NASCIO: Representing Chief Information Officers of the Statesframework for data governance will coexist with other frameworks thatdescribe other major components ofthe state government enterprise architecture.architecture. If state government doesnot have quality data and information,it will not achieve its objectives.Flawed data and information will leadto flawed decisions and poor servicedelivery to citizens. Data Governance Maturity Model:describes the journey from the AS IS tothe SHOULD BE regarding the management of data, information andknowledge assets. In parallel to thisjourney regarding data governance isthe journey that describes a maturingenterprise architecture operating discipline. State government mustunderstand where it is today andwhere it needs to go. This is an important step in planning the journey inmanaging information as an enterpriseasset. Data governance maturitymodels provide the means for gaugingprogress. By presenting intermediatemilestones as well as the desired endstate, maturity models assist inplanning HOW state government willreach the next level of effectiveness, aswell as WHEN and WHERE within stategovernment.Organizational Models, Roles andResponsibility Matrices (RASICCharts)5: defines WHO should beinvolved in decision making, implementing, monitoring and sustaining.Organizational models are a component of the enterprise businessarchitecture. An enterprise wide initiative will require the authority ofexecutive leadership and buy in fromall participants. Proper representationfrom stakeholders is also necessary formanaging risk. Collective wisdom canavoid missteps and false starts.Stakeholders and decision rights willvary depending on the specific issue orthe nature of the decision.Framework: describes WHAT isgoverned including related concepts,components and the interrelationshipsamong them. Decomposition offrameworks will uncover the necessaryartifacts that comprise the components of the framework. TheData Governance Part II: Maturity Models – A Path to Progress Methodology for Navigating theFramework: describes the methodsand procedures for HOW to navigatethrough the framework, create theartifacts that describe the enterprise,and sustain the effort over time. Thismethodology will co-exist within theenterprise architecture methodologyand touch on business architecture,process architecture, data architecture,organizational governance, data /knowledge management processes,and records management processes. Performance Metrics: to measure andevaluate progress and efficacy of theinitiative. These are traceable back tostrategic intent and related maturitymodels. These metrics need to becontinually evaluated for relevancy. Valuation and Security of StateGovernment Information Assets. Aspresented in the previous issue briefon data governance, proper valuation ofdata and information will determine thelevel of investment to ensure quality andappropriate security throughout theinformation asset lifecycle. This is wherethe data architecture and security architecture domains touch within stategovernment enterprise architecture.This research brief will focus on presentingvarious data maturity models. Future briefsor webinars will treat other foundationalaspects of data and information governance. Some common themes presentedby the variety of maturity models and theirassociated migrations to the higher levelsof maturity can be described as followsand also reflect a maturing enterprisearchitecture. From reactive to proactive understanding of the management of dataand information3
NASCIO: Representing Chief Information Officers of the States Strategic PlanningAssumption: Through2010, more than75% of organizationswill not get beyondLevels 1 and 2 intheir data qualitymaturity (0.8 probability).Strategic PlanningAssumption: Through2012, less than 10%of organizations willachieve Level 5 dataquality maturity (0.8probability). - Gartner6From point solutions to managedenterprise solutionsFrom “siloed” data to synchronizeddata and information (i.e., consistent, quality data)From localized systems with inconsistent levels of data classificationand security to consistent dataclassification and standards basedmanaged securityFrom myopic approach to datamanagement to an enterprise wideview of informationMigration to the capability to buildefficient information and knowledgemanagementImplementing Data Governance –Maturity ModelsThere are a number of data governancematurity models that can assist in theplanning and implementation of datagovernance. Each has strengths and canbring valuable perspectives, presentcharacteristics, and form the foundationfor subsequently planning a data governance delivery process. Reviewing andevaluating maturity models should occurearly in the process in order to establish anunderstanding of the end state. Thisunderstanding is necessary to properlyplan a data governance program. It mustbe understood that the delivery process isan ongoing enterprise operating disciplinewhich fits under the greater umbrella ofenterprise architecture. As with many of theconcepts presented by NASCIO, successfulimplementation of data governancerequires an enterprise perspective. Thisperspective will be portrayed in the higherstages of the maturity models presentedin this report.It should be expected that data governance maturity models will also “mature”as industry, government and societycontinue to “learn” how to manage andleverage data, information and knowledge, and most importantly act on thatlearning. Geospatial resources and socialnetworks are but two examples of change.4GIS and geospatial resource managementinitiatives have demonstrated the value ofhigh level associations and correlations.Social networks have demonstrated thevalue of group knowledge, and masscollaboration.The current issues in information management began with the way systems weredeveloped. Application teams worked inisolation. Applications were built forimmediate return. And project teams wereincented and pressured to deliver immediate results without proper considerationfor long term enterprise value and cost.Point solutions contributed greatly to thecurrent circumstances described in DataGovernance Part I. Data governance initiatives must anticipate the necessity ofdealing with the data fragmentation thatexists as an aftermath of these circumstances.Current federal programmatic fundingguidelines and restrictions have notcontributed toward creating enterprisewide initiatives such as data governance.Therefore, funding for enterprise wideinitiatives must come from a state generalor technology fund. Data governanceincluding master data managementshould be factored into every projectincluding those that are federally funded.Reviews should be conducted to ensureprojects and programs are in compliancewith state government principles,standards and methods. Federal fundingreforms should take into account the levelof effort associated with such complianceand provide the latitude and flexibility toinvest responsibly at an enterprise level sostate government can do what it needs toin order to build long term value for thestate. This will require strong partneringand collaboration between state andfederal government.There is the need for proper governancestructures that provide appropriate representation, decision rights, and renewedmethods and procedures to ensure stategovernment is not simply responding tofederal mandates and restrictive reportingrequirements. And that state governmentData Governance Part II: Maturity Models – A Path to Progress
NASCIO: Representing Chief Information Officers of the Statesisn’t forced into “siloed” solutions becauseof funding restrictions. Rather, state andfederal government work together todevelop funding mechanisms that givestates the flexibility they need to buildlong term value, shareable resources andincreased efficiency. Such an approachshould also allow or even encouragecollaboration between state and localgovernment on joint initiatives.As state government begins to think ofdata, information and knowledge as one ofthe most critical enterprise assets, the useof maturity models provides a means forassessing where the organization is todayand what will be required to migrate tothe desired end state. Maturity modelsalso assist in setting expectations. Thejourney the enterprise must take in developing the capabilities to properly manage,and harvest value from its knowledgeassets will not be an easy trip. Maturitymodels also assist in planning what isfeasible in the near term—particularlyData Governance Part II: Maturity Models – A Path to Progresswhen state government is facing severefiscal stress. Nevertheless, even duringtimes of fiscal stress, state governmentmust make progress so it can bettermanage limited resources in the nearterm, and emerge from such times readyto move forward. It will require constancyof purpose8, consistency in executivesupport, and a sustained effort by theentire enterprise. One other aspect to thissubject is the need to view data, information and knowledge from the citizens’perspective versus agency specificperspective. The citizen would like to “see”one state government—not a collection ofagencies.This research brief will look at a samplingof data governance maturity models anddraw some conclusions regarding the roleof maturity models in developing datagovernance within state government.One of the key driversof EIM [EnterpriseInformationManagement] is toovercome decades of“silo-based,” application-centricdevelopment, inwhich each systemmaintained its ownversion of data andprocess rules to suitlocal performanceneeds. This resultedin duplication and alack of agility withinthe organization.Gartner75
NASCIO: Representing Chief Information Officers of the StatesTABLE 1: DataFlux DATA GOVERNANCE MATURITY MODEL“Process failure andinformation scrapand rework causedby defectiveinformation costs theUnited States alone 1.5 trillion ormore.” - LarryEnglish, InformationImpact International,Inc.10Level of MaturityCharacteristics1Undisciplined(Think Locally, ActLocally)There are few defined rules and policies about dataquality and integration. There is much redundant data,differing sources, formats and records. The existingthreat is that bad data and information will lead to baddecisions, and lost opportunities.2Reactive(Think Globally, ActLocally)This is the beginning of data governance. There is muchreconciliation of inconsistent, inaccurate, unreliabledata. Gains are experienced at the department level.3Proactive(Think Globally, ActCollectively)It is a very difficult step to move to this phase. Theenterprise understands the value of a unified view ofinformation and knowledge. The enterprise beginsthinking about Master Data Management (MDM). Theorganization is learning and preparing for the nextstage. The culture is preparing to change.4Governed(Think Globally, ActGlobally)Information is unified across the enterprise. The enterprise has a sophisticated data strategy and framework.A major culture shift has occurred. People haveembraced the idea that information is a key enterpriseasset.DataFluxThe DataFlux Data Governance MaturityModel is very comprehensive. As theenterprise moves through the sequencefrom stage one to stage four, the valueharvested increases and the risk associated with “bad data” decreases.Tony Fisher, President and GeneralManager of DataFlux, presents an excellentoverview of information governancematurity on the SAS website.9 Fisher isspeaking about “Data Maturity” in thatpresentation. The scope of his discussionis relevant to the subject of this researchbrief—the broader view of data governance maturity models. Again, the termscan get fuzzy in different conversations—data governance, data management,6knowledge management, data assets andinformation assets. DataFlux has recentlymodified their maturity model to emphasize a business perspective that drives theneed for managing data as an enterpriseasset, and the employment of means suchas organization, process, and technologyto achieve the necessary levels of dataquality. The phases in the DataFlux modelare presented here (Table 1).DataFlux developed each stage of datamaturity by describing the characteristicsof each phase of maturity and how tomove to the next phase. These characteristics are formulated into four majordimensions that must be addressed asstate government matures its data governance. The dimensions are: people, policies,technology and risk. DataFlux has presentedData Governance Part II: Maturity Models – A Path to Progress
NASCIO: Representing Chief Information Officers of the StatesTABLE 2: DIMENSIONS IN THE DataFlux DATA GOVERNANCE MATURITYMODEL: LEVEL FOUR – “GOVERNED”People Executive sponsorship.Data consumers actively participatein strategy and delivery.Roles are established such as datasteward.A data governance expertise centerexists.The organization truly embracesdata quality and adopts a “zerodefect” policy for data collectionand management.Policies Technology Data quality
IBM Data Governance Council Predictions Data governance will become a regula-tory requirement. Information assets will be treated as an asset and included on the balance sheet. Risk calculations will become more pervasive