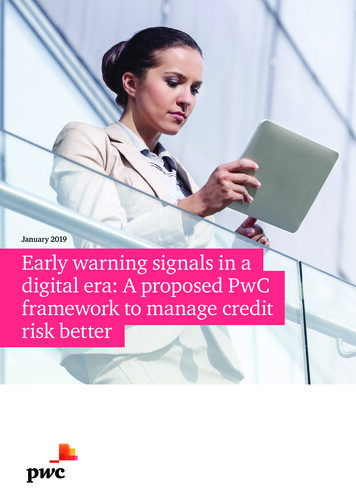
Transcription
January 2019Early warning signals in adigital era: A proposed PwCframework to manage creditrisk better
Moving towards global standards ofcredit monitoringAccording to RBI data, on 31 August 2018, 48.3% ofthe loan book of some of the large Indian scheduledcommercial banks (SCBs), taken together, consisted ofcorporate assets. The remaining 51.7% is spread betweenmicro, small and medium enterprises (MSMEs), retailcustomers and others (Figure 1). With this distributionof asset classes, the RBI estimates that the grossnon-performing assets (GNPA) of SCBs can rise from11.6% in March 2018 to 12.2% by March 2019. Themajor reasons for this is the inability of the banks toanticipate the incipient stress in accounts that are likely todefault. Simultaneously, the RBI’s regulations have beenevolving from rule-based monitoring to an early warningidentification approach over the last two decades (fromIncome Recognition and Asset Classification (IRAC) normsin the Nineties to monitoring specific signals in 2017). Thishas led investors to question the way loan accounts aremonitored by Indian banks in recent years.According to market participants, there is a dire needto revisit current practices and rework them accordingto globally accepted and advanced approaches used indeveloped economies. Banking organisations in India needto move from an essentially compliance-driven post factomechanism to a proactive control-based digital system tomonitor credit risk.To achieve this, lenders will have to constantly monitorpublicly available news on borrower- and industry-specificperformances. Analysing this information on advancedanalytical platforms will help lenders to take timelydecisions. Borrowers can then be grouped into three orfive watch list categories. Assuming a three-colour scale(green, amber and red), borrowers in the amber regionshould be monitored closely (here we are assumingcustomers lying in the red category have already turnedbad and giving them an early warning digitally may not2 PwCFigure 1 - Breakup of loan book of SCBs inIndia (Q1 FY19)Breakup of Loan BookRetail/MSME/OtherExposure 51.7%Corporate/Industry/Wholesale Exposure 48.3%create any significant value). Additionally, it may be notedthat as credit quality-related information takes some timeto impact the borrower, the system can be designed torun via batch processes, maybe daily or weekly, insteadof making it on an absolute real time basis. The initialinvestments to make it work on an absolute real time basiscan be very high.
Need to be proactive, rather than reactivein monitoring and managing credit riskPast experience has taught us that proactive controlscan help banks limit their exposure in bad segments andlead to reduction in overall losses (Figure 2). We havedivided Indian banks into two categories—Category A andCategory B, with Category A banks following traditionalpractices and Category B banks adopting an advancedmonitoring approach. The chart indicates that CategoryB banks have been successful in identifying bad assetsbetter than their counterparts, and hence, their gross NPAlevel and provisioning numbers are comparatively low.Therefore, we propose a digital credit risk monitoringframework to capture such early warning signals. Let usfirst try to understand the differences between the two.Figure 2 - Based on past experiences banks have been categorised (Category A – traditional andcategory B – advanced)16Category A Banks - Gross NPACategory A Banks - Loan loss provisionCategory B Banks - Gross NPACategory B Banks - Loan loss provision141210Δ GNPA levelof category Ais 1.85 timesof category -122010-112009-102008-092007-0802006-072Loan lossprovisioning forcategory A ismore than doublethan that ofcategory BComparison between the proposed framework and traditional practicesTraditional monitoring systemDigital monitoring system to capture early warning signalsApproachTraditional monitoring systems work on a postfacto basis, primarily based on past eventsand selective financial projections, i.e. they arereactive in nature.Digital monitoring early warning signals help financialinstitutions capture information in advance and takeprecautionary action before an account turns bad, i.e. it isproactive in nature.IntegrationFront-end business, underwriters and themonitoring authority would work in silos inthe past, which resulted in discrepancies ininformation.Seamless coordination of monitoring, underwriting and businessteams is crucial for the success of a system.EmphasisThe primary emphasis was on high-risk areasand the focus was limited to decisions basedon sample performances.The entire population-related data is analysed and thenaccounts are categorised according to a three-colour scale(green, amber and red). Customers in the amber region shouldbe under highest vigilance, assuming that those in the redarea have already become NPAs. This makes the system morecomprehensive in nature.PeriodicityAccounts were usually reviewed on a semiannual basis, or at the most, quarterly for highrisk accounts.Based on the capability of a system, assessments can beperformed in batch processes (on a daily or weekly basis).CoverageThe traditional system had a much narrowviewing angle. It failed to take a consolidatedview of individual loan accounts.Early warning signals (EWS) collates data from multiple sources,which helps the bank to have a consolidated view of individuals’loan accounts and decision-making becomes easier.UsageTraditionally, provisioning was based onregulatory guidelines (RBI’s IRAC Norms) andaccounting requirements (INDAS109).Systems can be upgraded if such signals are incorporated intothe INDAS109 staging decision process.OutputManual cleaning and analysis of data was theprimary option available in most cases.MIS dashboards can be prepared automatically and userinterfaces can be customised, based on simple drill-downs.How to manage credit risk better with early warning signals: A proposed PwC framework 3
Potential benefits of adopting a digital frameworkfor monitoring and managing credit risk Reduction of default rate at portfolio level: The rateof defaults at the portfolio level can be reduced byrestrictive exposure to particular customer segmentswhere warning signs have been observed. This will helpto control the probability of default (PD). Tightening of covenants: Lenders need to interveneearly by tightening the covenants and increasingcollateral levels to maximise recovery. Once a loanaccount comes into the watch list, regular supervisionwill help to maximise collateral-related requirements andreduce losses in the event of actual default. This willminimise loss given default (LGD). Securitisation of assets: Lenders must try to proactivelysecuritise or sell assets that have warning signalsassociated with them and where chances of revival arelow. There are certain non-banking financial companies(NBFCs) and asset reconstruction companies (ARCs) inIndia that may be interested in taking over such assets.This will help companies get bad loans off their booksand get a better price for them. Decision on IFRS9 staging-related aspects: The IFRS9framework can be integrated with the INDAS109 ECLmodelling framework. It will enable banks to quicklygauge the actual health of a borrower and accordinglydecide on the ‘stage’ of the account for provisioningpurposes. Identification of trends and opportunities in themarket: New trends can be identified in differentindustries and banks can readjust their portfolio mix.For example, it could have helped banks identify thetelecom sector as a whole turning adverse and slowlyreduce their exposure to it. Decisions taken on composition of portfolios:Monitoring at the portfolio level can help to reducebanks’ exposure to threatened sectors, and evenat individual levels reduce exposure by decreasingcommitted lines of credit, i.e., the credit conversionfactor. Modification of the credit policies of banks: High levelcredit policy-related decisions can be taken by banks,based on information provided by the system aboutsectoral regulations, performance and news.A segmental approach to reducefalse positives Large and medium companies: As these are high-valueaccounts, the ideal approach should be to assess therisk of bad loans at an account level. Based on theassessment, industry variables can be identified. Inanother approach, market data, movement in the spreadof commercial papers (CPs) can be used as a goodindicator for this segment, since many of these entitiesissue CPs, bonds and non-convertible debentures(NCDs), which are subscribed by banks. SME segment: Banks can create industry andgeographic clusters or a mix of both in this segment.They can also use already existing geographic clusters. Retail assets: Banks can create pools of homogeneousaccounts for designing purposes. Moreover, socioeconomic and geographic classification can be helpful. Agriculture portfolio: The biggest problems in thissegment include extremely small average agriculturalland holdings and variable repayment schedules. It isalso necessary to take into account factors that have asignificant impact on crop production and repaymentconditions, e.g., rainfall and political scenario.4 PwC
Keys areas for capabilitydevelopment Strengthening of data: The predictive power of thesystem lies on the accuracy of the databases, based onwhich it has been developed. At the core of the systemwill be the data warehouse. Apart from that, data willhave to be collated from various internal and externalsources, e.g. the core banking solutions of banks,external credit rating agencies and market-relatednews articles. Hence, sanctity of the data is of utmostimportance for this exercise to be successful. Advanced analytical tools: Meaningful insights orindications about assets can be harnessed from allthese data sources with the help of advanced analyticaltools. Artificial Intelligence (AI) and machine learning(ML) algorithms can be gainfully deployed to generatetools that predict early warning signs of default. Automated risk processes: These have the ability tomake a framework more secure and compliant withregulatory requirements and avoid risks associated withmanually driven processes. Pulling of data, analysingthe same and generation of meaningful insights shouldbe automated to the extent possible. Human capital: Employees with operational ownershipof these tools should enhance their capabilities tointerpret and gainfully embed such signals in credit riskmanagement strategies. To achieve this, investment onreskilling of employees is required for smooth transitionto such models.Data can be collected frommulti-dimensional sources Financial information of borrowers: Financialinformation can be accessed from unaudited interimfigures, creditor’s feedback and bank statements.For example, tie-ups can be made to monitor bankstatements and creditor’s feedback can be collected viae-mails and VBA-based automation. Market information: Movement in equity prices, yieldcurves, CP spreads, ratings and other credit bureaupublications are required data sources. Hence, AI orML algorithms can be helpful to pull them together.Automated checks can be set up with market datasources such as Bloomberg and Thomson Reuters. Fordata from credit bureau reports, rating publications canbe collected periodically and updated in the system. Market news: Vernacular news articles along withnegative press conferences or notices and news fromany other external sources are ideal. Banks need todevelop tools based on ‘Text Mining’ and ‘SentimentAnalysis’, which can be done with the help of NaturalLanguage Processing (NLP). Information from relationship managers: Relationshipmanagers sometimes have knowledge that is notcommonly known in the market, e.g. information onpromoters or key personnel, consumer complaints,deterioration in product quality and movement ofinventory. External macro scenario: Systems should be enabledto accommodate information on adverse regulatoryguidelines or notifications, since the Government’sdecisions may adversely impact a business, with itsimplementation of new regimes, e.g. Goods and ServiceTax (GST) and Insolvency and Bankruptcy Code (IBC),and the implications of monetary policies.How to manage credit risk better with early warning signals: A proposed PwC framework 5
Identify a suitable list of indicators foryour portfolio Financial indicators: Focus should be on indicators,which have not yet affected the bottom line of thebusiness, e.g. stock statement or creditor feedback. Geographical indicators: These are more relevant in thecase of loans to SME clusters, borrowers with a regionalpresence, and most importantly, for overseas borrowers. Perception indicator: The future trajectory of a businesscan be gauged from the market’s perception of it. Behavioural indicators: The capabilities and integrityof key stakeholders (promoters, management) of abusiness can indicate how a company behaves indifferent situations. Industry indicators: Short- and long-term industryperformance can be positive indicators.6 PwC
Proposed target operatingframeworkProposed application of digital monitoring of earlywarning signals has the potential to vastly improve thequality, timeliness and identification of bad accounts. Toseize this opportunity, banks need to move fast, but in aprudent manner, to operationalise the system. However,implementation demands significant rigour and time.Please refer to the target operating framework in Figure 3.Figure 3 Early warning signals along with the IFRS9 modelling framework can help decide the‘stage’ of an accountCourse of actionAutomated risk management processInputFinancialinformationyet to ack)MarketrelatedinformationCollating information frommultiple sources usinginterdepartmental linkagesManagement actionVernacularnews, pressconferencesor noticesaboutborrowerInformationsourced byrelationshipmanagers ofa bankExternalmacroeconomicscenarioCredit Risk Management DepartmentConveying limits and actionsSpecial Credit Monitoring CellProcessing information usingadvanced analytical tools togain insights (Google analyticsin l tomaximiserecoverySecuritisingor sellingassets thatemanatesignals ofincipientstress(NBFCs andARCs couldbe potentialbuyers.)Designing ofacceptablethreshold limits andplausible courses ofactionApprovingthreshold limitsand actioncoursesBoard-levelcommittees andStrategic BusinessPlanning GroupSending out early alerts ondeterioration in borrowers’ healthand providing actionable set ofrecommendationsFactoring inearly warningsignalsalong withthe IFRS9modellingframeworkcan helpdecide the‘stage’ of anaccountReducingdefaultrate at theportfolio levelby restrictiveexposureIdentifyingcurrent andupcomingtrends oropportunitiesin the marketDeciding onreengineeringcompositionof portfoliosModifyingcreditpolicies afterboard-leveldiscussionsThis target operating framework has the capability to harness insights that were previously unknown and help banks reducetheir rapidly growing non-performing assets.How to manage credit risk better with early warning signals: A proposed PwC framework 7
About PwCAt PwC, our purpose is to build trust in society and solve important problems. We’rea network of firms in 158 countries with over 250,000 people who are committed todelivering quality in assurance, advisory and tax services. Find out more and tell us whatmatters to you by visiting us at www.pwc.com.In India, PwC has offices in these cities: Ahmedabad, Bengaluru, Chennai, Delhi NCR,Hyderabad, Kolkata, Mumbai and Pune. For more information about PwC India’s serviceofferings, visit www.pwc.inPwC refers to the PwC network and/or one or more of its member firms, each of which is aseparate legal entity. Please seewww.pwc.com/structure for further details. 2019 PwC. All rights reservedContacts:Kuntal SurPartner, FRREmail: kuntal.sur@pwc.comAvinash SardesaiAssociate Director, FRREmail: avinash.sardesai@pwc.comKapil TodiAssociate Director, FRREmail: kapil.todi@pwc.comAsmita GangulyManager, FRREmail: asmita.ganguly@pwc.comShreyam SikdarConsultant, FRREmail: shreyam.sikdar@pwc.compwc.inData Classification: DC0This document does not constitute professional advice. The information in this document has been obtained or derived from sourcesbelieved by PricewaterhouseCoopers Private Limited (PwCPL) to be reliable but PwCPL does not represent that this information isaccurate or complete. Any opinions or estimates contained in this document represent the judgment of PwCPL at this time and aresubject to change without notice. Readers of this publication are advised to seek their own professional advice before taking anycourse of action or decision, for which they are entirely responsible, based on the contents of this publication. PwCPL neither acceptsor assumes any responsibility or liability to any reader of this publication in respect of the information contained within it or for anydecisions readers may take or decide not to or fail to take. 2019 PricewaterhouseCoopers Private Limited. All rights reserved. In this document, “PwC” refers to PricewaterhouseCoopersPrivate Limited (a limited liability company in India having Corporate Identity Number or CIN : U74140WB1983PTC036093), which isa member firm of PricewaterhouseCoopers International Limited (PwCIL), each member firm of which is a separate legal entity.KS/January2019-15830
Digital monitoring early warning signals help financial institutions capture information in advance and take precautionary action before an account turns bad, i.e. it is proactive in nature. Integration Front-end business, underwriters and the monitoring authority would work in silos in the past, which resulted in discrepancies in information.