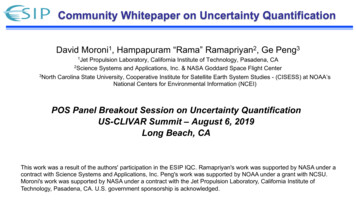
Transcription
Community Whitepaper on Uncertainty QuantificationDavid Moroni1, Hampapuram “Rama” Ramapriyan2, Ge Peng31Jet Propulsion Laboratory, California Institute of Technology, Pasadena, CAScience Systems and Applications, Inc. & NASA Goddard Space Flight Center3North Carolina State University, Cooperative Institute for Satellite Earth System Studies - (CISESS) at NOAA’sNational Centers for Environmental Information (NCEI)2POS Panel Breakout Session on Uncertainty QuantificationUS-CLIVAR Summit – August 6, 2019Long Beach, CAThis work was a result of the authors' participation in the ESIP IQC. Ramapriyan's work was supported by NASA under acontract with Science Systems and Applications, Inc. Peng's work was supported by NOAA under a grant with NCSU.Moroni's work was supported by NASA under a contract with the Jet Propulsion Laboratory, California Institute ofTechnology, Pasadena, CA. U.S. government sponsorship is acknowledged.
ESIP Information Quality ClusterVision: “Become internationally recognized as an authoritative and responsiveinformation resource for guiding the implementation of data quality standards and bestpractices of the science data systems, datasets, and data/metadata dissemination services.”Information Quality {Science Quality, Product Quality, Stewardship Quality, Service Quality}What do we do? Share experiences; collaborate internationally; invited speakers at monthly telecons; sessions and/orpresentations at AGU, AMS, ESIP, E2SIP, and OGC meetings Maintain wiki site with many useful references http://wiki.esipfed.org/index.php/Information QualityPublications Peng, G. et al., 2016: Scientific stewardship in the Open Data and Big Data era - Roles andresponsibilities of stewards and other major product stakeholders. D.-Lib Magazine, 22 (5/6), doi:https://doi.org/10.1045/may2016-peng. Ramapriyan, H K, Peng G, Moroni D, Shie C-L, Ensuring and Improving Information Quality for EarthScience Data and Products. D-Lib Magazine, 23 (7/8), July/August 2017, DOI:https://doi.org/10.1045/july2017-ramapriyan Moroni, et al.(22 authors), “Understanding and Communicating Uncertainty in Earth Science DataInformatics”, White Paper (in preparation)2
Information Quality - DefinitionScientific quality Accuracy, precision, uncertainty, validity and suitability for use (fitness for purpose)in various applicationsProduct quality How well the scientific quality is assessed and documented Completeness of metadata and documentation, provenance and context, etc.Stewardship quality How well data are being managed, preserved, and cared for by an archive orrepositoryService Quality How easy it is for users to find, get, understand, trust, and use data Whether archive has people who understand the data available to help users.3
White Paper ScopeBegan as a pilot plenary/breakout session at the ESIP 2017 meeting, featuringinvited researchers: Carol Anne Clayson (WHOI), Isla Simpson (NCAR), andAmy Braverman (JPL).Primary focus on “discovery” of the breadth of approaches with regard to Earthscience data UQ, UC, and the dissemination/utilization of UQ/UC informationby data providers and end users.Considers 4 perspectives: Mathematical, Programmatic, Observational, User.Will identify both commonalities and differences between perspectives.Authors and co-authors represent various aspects of Earth science datainformatics, metrology, data science/statistics, remote sensing, in situ, anddisciplinary fundamental research.Numerical modeling was considered for the sake of use case discussion, butwas decided to be left out for the sake of focusing on approaches usingobservational data.4
MathematicalChampioned by Jonathan Hobbs - JPLConsidered to be the foundational section of thepaper, establishing the key mathematicallybased definitions of uncertainty and relatedconstructs such as UQ, UC, mean square error,PDFs, quantiles, confidence intervals,confidence levels, etc Presents directly applicable use cases by whichthese mathematical definitions are applicable toobservational Earth science data, primarily froma remote sensing perspective, but much ofwhich utilizes consistent metrology for a varietyof measurement types, including in situ andsub-orbital.Schematic implementation of Bayes’ theorem for aunivariate QOI. The prior distribution is combinedwith information from an observation (via thelikelihood) to produce a posterior distribution.5
ProgrammaticChampioned by Rama – SSAI/NASAGSFC.Captures the governmental andintergovernmental approaches,starting with specific US-basedagencies and moving into theinternational arena.Considers US law that drives policy atkey agencies, including but not limitedto NASA and NOAA.Considers international agreements,such as by the U.N, IPCC, WMO, andCEOS.Considers multi-lateral agreements,statements and policies by EUsponsored agencies/organizations,such as by: ESA, FIDUCEO,UncertWeb, and MetEOC.6
ObservationalChampioned by Justin Goldstein –NOAA.Discusses the foundational approachesto UQ and UC from an Earth observationperspective, including perspectives fromboth point-based studies, invariant inspace but not in time (e.g., EulerianSpecifications), and those that conductobservations varying in both space andtime (e.g., Lagrangian Specifications).Cal/Val: looks at UQ and UC approachesfrom a calibration and validationperspective and the role played by“ground truth” data.Product Development: examines avariety of approaches andconsiderations toward makinguncertainty information available forcommon types of observational dataproducts, with a focus on making thisinformation available at the productionstage of data.Figure: Snow water equivalent with uncertainty shaded with 95% confidenceintervals. Blue line represents the point estimate. Hobbs et al. 2017, unpublished.7
UserChampioned by Bob Downs –Examples considered include:Columbia University.ISO-19157, UncertML, CO2SYS,and OGC’s Testbed-12 innovationFocuses on the ways in whichprogram (OGC, 2017).uncertainty information can beeffectively or ineffectivelyconsumed, interpreted andultimately leveraged by the typicaldata user.Provides insights in to methods ofcommunication, dissemination,visualization tools/services, andmulti-variate analysis.Figure: Applying UncertML to automated Bayesian interpolation algorithm. Left plot assumesGaussian random error while right plot incorporates obs-specific error characteristics viaUncertML encoding, improving the performance of interpolation. Williams et al. 2009.8
Next StepsComplete by August: Commonalities, differences, conclusions. Re-write the introduction to better align with main sections. Include more graphics/figures.Complete by September Prep for white paper publication; consult with ESIP studentfellow to apply improved styling and consistentreferences/citation styling adhering to AGU standard. Publish on ESIP Figshare site.9
Ideas beyond this publication Draft and publish a shortened “executive summary” paperin a more prominent journal, such as Data Science orEOS.Draft a part-2 paper, focusing on recommendations andactionable solutions.10
Authorship Acknowledgements (19)Jonathan Hobbs1, Robert Wolfe4, Chung-Lin Shie5,Christopher J. Merchant6, Janae Csavina7, Mark Bourassa8,Isla Simpson9, Jessica L. Matthews3, Matthew Plumlee10,Peter Cornillon11, Justin C. Goldstein12, Lucy Bastin13,Kenneth Kehoe14, Benjamin Smith15, Jeffrey L. Privette15,Robert R. Downs16, Aneesh C. Subramanian17, Otis Brown3,Ivana Ivánová1811
Co-Author .17.18.Jet Propulsion Laboratory, California Institute of Technology, Pasadena, CAScience Systems and Applications, Inc. & NASA Goddard Space Flight CenterNorth Carolina State University (NCSU), Cooperative Institute for Climate and Satellites-North Carolina (CICS-NC) at NOAA’sNational Centers for Environmental Information (NCEI), Asheville, NCNASA Goddard Space Flight CenterNASA Goddard Space Flight Center and University of Maryland, Baltimore CountyUniversity of Reading and National Centre for Earth Observation, Reading, UKNational Ecological Observatory NetworkCenter for Ocean-Atmospheric Prediction Studies, Florida State UniversityNational Center for Atmospheric ResearchNorthwestern UniversityUniversity of Rhode IslandRiverside Technology, Inc. supporting the NOAA NESDIS Technology, Planning, and Integration for Observation divisionJoint Research Centre, European Commission / Aston University, UKUniversity of Oklahoma- Cooperative Institute for Mesoscale Meteorological StudiesNOAA’s National Centers for Environmental InformationCenter for International Earth Science Information Network, Columbia UniversityUniversity of Colorado BoulderCurtin University, Australia12
ESIP Information Quality Cluster Vision: "Become internationally recognized as an authoritative and responsive information resource for guiding the implementation of data quality standards and best practices of the science data systems, datasets, and data/metadata dissemination services." Information Quality {Science Quality, Product Quality, Stewardship Quality, Service Quality}