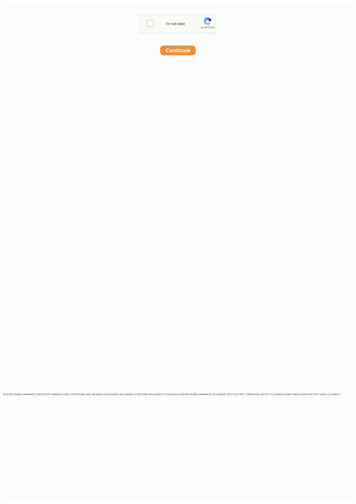
Transcription
Continue22753785.320988 41984646975 142872321975 10006308.372093 111872815968 34651148.946429 41474259288 146221983600 91518217896 3851418.8941176 5592506150 29922995.851064 19640684.58 32521666408 15671725.627907 113893205160 34875217.52 21608410.473684 7680704.440678 40179477.30303 123219861.5
Statistics books for data science pdf file free
Statistics for data science book. Statistics for data science pdf.So, just hit the share buttons and forward this list to other curious learners. PostgreSQL Notes for Professionals Author: The StackOverFlow Community About PostgreSQL Notes for Professionals PDF: This book is the definitive guide to undocumented and partially-documented features of the PostgreSQL server. We know these recipes are useful andwe know they work, because we use them ourselves. This is considered as one of the best free data mining and data science books for intermediate and experts from this list. Stanton and Jeffrey S. Applied Data Science Author: Ian Langmore About Applied Data Science PDF: “Applied Data Science” is a free data science book that focuses more on thestatistics end of things, while also getting readers going on (basic) programming & command line skills. However, if you do not take the class, the book mostly stands on its own. John Mount and Nina Zumel, authors of Practical Data Science with R, selected these chapters to give you the big picture of the many data domains. Exploring Data Sciencewith Python Author: Naomi Ceder About Exploring Data Science with Python PDF: Exploring Data with Python is a collection of chapters from three Manning books, hand-picked by Naomi Ceder, the chair of the Python Software Foundation. The many topics include neural networks, support vector machines, classification trees and boosting—the firstcomprehensive treatment of this topic in any book. This book is ideal for students, educators, community activists, non-profit organizations, small business owners, local governments, journalists, researchers, or anyone who wants to take data out of spreadsheets and turn it into lively interactive stories. Applied Spatial Data Analysis with R Author:Edzer J. Analyzing Linguistic Data: A Practical Introduction to Statistics Author: R. Topics include the counterintuitive nature of data in high dimensions, important linear algebraic techniques such as singular value decomposition, the theory of random walks and Markov chains, the fundamentals of and important algorithms for machine learning,algorithms and analysis for clustering, probabilistic models for large networks, and more. Computer implementation of a genetic algorithm. The book intends to take someone unfamiliar with Spark or R and help you become proficient by teaching you a set of tools, skills and practices applicable to large-scale data science. In this book, You’ll learnabout introduction to data science, programming in python, classifications, predictions, data types, visualization, and more. It is rigorous, yet intuitive. Do you like this list of free data science books? Data analysts, computer scientists, and theorists will appreciate this thorough and up-to-date treatment of sparse statistical modeling. And this book(Applied Data Science) is worth a read for the topics it does cover. This is considered as one of the best free R for data science books for beginners, You can download and learn more about this pdf from the below given link. This eBook will provide students with a hands-on training on how to use data analytics tools and machine learning methodsavailable in R to explore, visualize, and model big data. Model-Based Clustering and Classification for Data Science Authors: Charles, Gilles, Brendan and others About Model-Based Clustering and Classification for Data Science PDF: This text book focuses on the recent developments in model-based clustering and classification while providing acomprehensive introduction to the field. In this latest guide, the authors offer insights into system design, implementation, and maintenance from practitioners who specialize in security and reliability. Pulled from the web, here is a our collection of the best, free books on Data Science, Big Data, Data Mining, Machine Learning, Python, R, SQL,NoSQL and more. Statistical Inference Via Data Science: A ModernDive Into R and the Tidyverse Authors: Albert Young-Sun Kim and Chester Ismay About Statistical Inference via Data Science PDF: This book assumes no prerequisites: no algebra, no calculus, and no prior programming/coding experience. This book is focused on the details of dataanalysis that sometimes fall through the cracks in traditional statistics classes and textbooks. MySQL Notes For Professionals Author: The StackOverFlow Community About MySQL Notes for Professionals PDF: MySQL’s popularity has brought a flood of questions about how to solve specific problems, and that’s where this MySQL Notes forProfessionals is essential. You’ll gain a deep understanding of the reactive programming model that underlies Shiny, as well as building a tool box of useful techniques to solve common app challenges. Text Mining with R: A Tidy Approach Author: David Robinson and Julia Silge About Text Mining with R: A Tidy Approach PDF: With this practical book,you’ll explore text-mining techniques with tidytext, a package that authors Julia Silge and David Robinson developed using the tidy principles behind R packages like ggraph and dplyr. Author: Susan Holmes About Modern Data Science for Modern Biology PDF: This book will teach you ‘cooking from scratch’, from raw data to beautiful illuminatingoutput, as you learn to write your own scripts in the R language and to use advanced statistics packages from CRAN and Bioconductor. Advanced Statistics From an Elementary Point of View Author: Michael J. For clarity, we have devised notation that may differ slightly from the notation used in your discipline. They are various techniques fromrelation extraction to under or less resourced language. I believe that this book will give new knowledge in the text mining field and help many readers open their new research fields. If yes, then without blinking an eye, use 5 second rule and decide whether to share this article or not. Along the way, our team of experts provides field-testedapproaches, personal tips and tricks, and real-life case studies. Also it will not teach you anything about R programming. Spatial Modelling for Data Scientists Authors: Francisco Rowe and Dani Arribas-Bel About Spatial Modelling for Data Scientists PDF: In this eBook, You will learn how to analyse and model different types of spatial data as well asgaining an understanding of the various challenges arising from manipulating such data. This is considered as one of the best free data science books for beginners, You can download and learn more about this pdf from the below given link. Lilja What’s Special about Linear Regression Using R PDF: Linear Regression Using R: An Introduction to DataModeling presents one of the fundamental data modeling techniques in an informal tutorial style. This is a printed edition of the tutorial documentation from the R distribution, with additional examples, notes and corrections. Table Of ContentsSome of the Free And Best Data Science Books For Beginners, Intermediate and Advanced Enthusiast (OurFavorite from the List)100 Free Statistics, Data Mining, Python, Mathematics, Data Visualization, SQL & Data Analytics Books Are As FollowsHands-On Data Visualization: Interactive Storytelling from Spreadsheets to CodeAn Introduction to Statistical Learning, 2nd Edition Data Science at the Command Line, 2nd EditionR Graphics Cookbook:Practical Recipes for Visualizing Data, 2nd EditionGGPlot2: Elegant Graphics for Data Analysis, 2nd EditionR Cookbook: Proven Recipes for Data Analysis, Statistics and Graphics, 2nd EditionThink Bayes, Second EditionBuilding Secure and Reliable Systems – Best Practices for Designing, Implementing, and Maintaining SystemsMasteringShinyProbability, Statistics, and Data: A Fresh Approach Using RA Beginner’s Guide to Clean Data: Practical advice to spot and avoid data quality problemsData Science Desktop Survival GuideComputational and Inferential Thinking: The Foundations of Data Science, 2nd EditionData Science in Julia for HackersPrinciples and Techniques of DataScienceIntroduction to Probability for Data ScienceFundamentals of Data VisualizationThe Data Science HandbookPython Data Science HandbookIntroduction to ProbabilityR for Data Science: Import, Tidy, Transform, Visualize, and Model DataComputer Age Statistical InferenceData-Intensive Text Processing with MapReduceStatistical Inference ViaData Science: A ModernDive Into R and the TidyverseHappy Git and GitHub for the useRAgile Data Science with R: A workflowSpatial Modelling for Data ScientistsGeocomputation with RSpatial Data Science: With applications in REfficient R Programming: A Practical Guide to Smarter ProgrammingData Science In A BoxIntroduction to ModernStatisticsThe Elements of Statistical Learning: Data Mining, Inference, etcModern Statistics with R: From wrangling and exploring data to inference and predictive modellingSupervised Machine Learning for Text Analysis in RInteractive web-based data visualization with R, plotly, and shinyBest Coding Practices for RThe Hitchhiker’s Guide toPythonStatistical rethinking with brms, ggplot2, and the tidyverse: Second editionText Mining with R: A Tidy ApproachModel-Based Clustering and Classification for Data ScienceStatistics in Plain English, Third EditionExploring, Visualizing, and Modeling Big Data with RModern Data Science with R, 2nd editionMastering Spark with RThink Stats:Exploratory Data Analysis in PythonFoundations of Data ScienceData Mining and Analysis: Fundamental Concepts and AlgorithmsMastering Software Development in RGenetic Algorithms in Search, Optimization, and Machine LearningSocial Media Mining: An IntroductionAdvanced ROpen Data Structures – An IntroductionThink Python: How toThink Like a Computer ScientistR for Excel Users21 Recipes for Mining Twitter Data with rtweetAutomate the Boring Stuff with Python: Practical Programming for Total BeginnersIntroduction to Information RetrivalD3 Tips and TricksStatistical Learning with Sparsity: The Lasso and GeneralizationsData Visualization: A PracticalIntroductionModeling with Data: Tools and Techniques for Scientific ComputingBayesian Methods for Hackers: Probabilistic Programming and Bayesian InferenceData Mining: Practical Machine Learning Tools and Techniques, Third EditionAdvanced Statistics From an Elementary Point of ViewIntroduction to Data Science: Data Analysis andPrediction Algorithms with RA Programmer’s Guide to Data MiningThe Data Science Design ManualOracle Database Notes for ProfessionalsThe Tidyverse CookbookSQL Notes for ProfessionalsEthics and Data ScienceMySQL Notes For ProfessionalsPostgreSQL Notes for Professionals Linear Regression Using R: An Introduction to DataModelingStatistical Inference for Data ScienceThe Element of Data Analytic StyleCausal Inference: What ifData Science: Theories, Models, Algorithms, and AnalyticsData Mining with Rattle and R: The Art of Excavating Data for Knowledge DiscoveryAn Introduction to Data ScienceData Jujitsu: The Art of Turning Data into ProductThe Art of DataScienceData Driven: Creating a Data CultureR Programming for Data ScienceExecutive Data Science – A Guide to Training and Managing the Best Data ScientistsExploratory Data Analysis with ROpenIntro Statistics, 4th EditionTheory and Applications for Advanced Text MiningData Science: An Introduction WikiBookDisruptive Possibilities: How BigData Changes EverythingIntroduction to R – Notes on R, A Programming Environment for Data Analysis and GraphicsFundamental Numerical Methods and Data AnalysisIntroduction to Social Network MethodsAnalyzing Linguistic Data: A Practical Introduction to StatisticsIntroduction to Statistical ThoughtApplied Data Science Data Mining andKnowledge Discovery in Real Life ApplicationsThe SysAdmin HandbookKnowledge-Oriented Applications in Data MiningR and Data Mining: Examples and Case StudiesConversations On Data ScienceAdvanced Linear Models for Data ScienceBig Data, Data Mining, and Machine LearningInductive Logic Programming: Techniques and ApplicationsTheField Guide of Data ScienceModern Data Science for Modern BiologyCrash Course on Basic StatisticsHands-on Machine Learning and Big DataMathematics of Data ScienceScipy Lecture NotesStatistics With JuliaA Genetic Algorithm TutorialExploring Data Science with PythonUnderstanding DatabasesExploring Streaming Data AnalysisExploringData ScienceExploring the Data JungleExploring Math for Programmers and Data ScientistsAdvances in Evolutionary AlgorithmsGenetic Programming: New Approaches and Successful ApplicationsGlobal Optimization Algorithms: Theory and ApplicationAlgorithms Notes for ProfessionalsRegression Models for Data Science in RThink DataStructuresData Visualization in SocietySQL Server Backup and RestoreMaking Sense of Stream Processing: Behind Apache KafkaMachine Learning for Data Streams: Practical Examples in MOAJust Enough R: Learn Data Analysis with R in a DayData Blending For DummiesData Mining Applications in Engineering and MedicineUnderstanding BigData: Analytics for Hadoop and Streaming DataApplied Spatial Data Analysis with R Have you checked 100 Free Machine Learning and Artificial Intelligence Books? Zaki About Data Mining and Analysis: Fundamental Concepts and Algorithms PDF: This textbook for senior undergraduate and graduate data mining courses provides a broad yet indepth overview of data mining, integrating related concepts from machine learning and statistics. R and Data Mining: Examples and Case Studies Author: Yanchang Zhao About R and Data Mining: Examples and Case Studies PDF: The book helps researchers in the field of data mining, postgraduate students who are interested in data mining, anddata miners and analysts from industry. Applications of genetics-based machine learning. Horton About Modern Data Science with R, 2nd edition PDF: This book is intended for readers who want to develop the appropriate skills to tackle complex data science projects and “think with data” (as coined by Diane Lambert of Google). H. This book isavailable for download for free under a Creative Commons license. Learn more about this eBook from the given link. The title focuses on “Data Science” but in fact this book could be used to provide a thorough introduction to probability for any STEM student. Use the book’s visualizations directory, a graphical guide to commonly used types of datavisualization. Data Mining and Knowledge Discovery in Real Life Applications Author: Julio Ponce About Data Mining and Knowledge Discovery in Real Life Applications PDF: This book presents four different ways of theoretical and practical advances and applications of data mining in different promising areas like Industrialist, Biological, and Social.Automate the Boring Stuff with Python: Practical Programming for Total Beginners Author: Al Sweigart About Automate the Boring Stuff with Python PDF: In Automate the Boring Stuff with Python, you’ll learn how to use Python to write programs that do in minutes what would take you hours to do by hand—no prior programming experiencerequired. R supports a wide range of statistical techniques and is easily extensible via user-defined functions. Causal Inference: What if Author: James Robins and Miguel Hernán About In Causal Inference: What if PDF: The application of causal inference methods is growing exponentially in fields that deal with observational data. It is meant tosupport a series of lectures, not replace them. However, it will give you a fantastic grounding in the language and in particular includes recommended best practices and frameworks. Computer Age Statistical Inference Authors: Bradley Efron and Trevor Hastie About Computer Age Statistical Inference PDF: This book covers the theory behind most ofthe popular machine learning algorithms used by data scientists today. For the many universities that have courses on data mining, this book is an invaluable reference for students studying data mining and its related subjects. Downey About Think Stats: Exploratory Data Analysis in Python PDF: By working with a single case study throughout thisthoroughly revised book, you’ll learn the entire process of exploratory data analysis—from collecting data and generating statistics to identifying patterns and testing hypotheses. Based on undergraduate classes taught by author Allen Downey, this book’s computational approach helps you get a solid start. Grinstead, J. Big Data, Data Mining, andMachine Learning Author: Jared Dean About Big Data, Data Mining, and Machine Learning PDF: Big Data, Data Mining, and Machine Learning: Value Creation for Business Leaders and Practitioners is a complete resource for technology and marketing executives looking to cut through the hype and produce real results that hit the bottom line. GlobalOptimization Algorithms: Theory and Application Author: Thomas Weise About Global Optimization Algorithms: Theory and Application PDF: This book is devoted to global optimization algorithms, which are methods to find optimal solutions for given problems. This text is not for you if you hope to learn about different algorithms and statisticaltechniques to do data science; more knowledgeable people have written many books and articles on those topics. It is full of beautiful illustrations and easy-to-understand code samples (in Python and Matlab). Recommended Web Stories And Articles: Data Science in Julia for Hackers Authors: Federico Carrone, Herman Obst Demaestri and MarianoNicolini About Data Science in Julia for Hackers PDF: It is in this sense that this book is meant for hackers: it will lead you down a road with a results-driven perspective, slowly growing intuition about the inner workings of many problems involving data and what they all have in common, with an emphasis on application. The book gives a rigoroustreatment of the elementary concepts of regression models from a practical perspective. Stanton is an easy-to-read, gentle introduction for people with a wide range of backgrounds into the world of data science. Executive Data Science – A Guide to Training and Managing the Best Data Scientists Author: Brian Caffo, Roger D. This is considered asone of the best free data visualization and data science books for beginners, You can download and learn more about this pdf from the below given link. Intermediate R programmers can dive deeper into R and learn new strategies for solving diverse problems while programmers from other languages can learn the details of R and understand why Rworks the way it does. It’s a supplement to the second edition of McElreath’s text. As you look at relational databases and infrastructure design, you’ll also discover the factors to consider when choosing your database architecture and explore illuminating real-world case studies that shine a light on NoSQL databases and the elements that driveNoSQL business solutions. Saltz and Jeffrey M. You’ll learn about ranking SVMs, XML, DNS, and LSI. The authors have extensive experience both managing data analysts and conducting their own data analyses, and have carefully observed what produces coherent results and what fails to produce useful insights into data. Computational andInferential Thinking: The Foundations of Data Science, 2nd Edition Authors: Ani Adhikari, John DeNero, and David Wagner About Computational and Inferential Thinking: The Foundations of Data Science, 2nd Edition PDF: This eBook was originally developed for the UC Berkeley course Data 8: Foundations of Data Science. If you are an intermediateuser, this book is useful for expanding your horizons and jogging your memory. This is considered as one of the best free R for data science books from this list. This is why you will find here why and how Data Mining can also be applied to the improvement of project management. It combines a technical and a business perspective, bridging the gapbetween data mining and its use in marketing. Manning About Introduction to Information Retrival PDF: This is the first book that gives you a complete picture of the complications that arise in building a modern web-scale search engine. It is based on R, a statistical programming language that has powerful data processing, visualization, andgeospatial capabilities. Building your own automated solution will take a lot of work, but we do offer some advice on possible options, such as PowerShell scripting, T-SQL scripts and SQL Server Agent jobs. It includes everything from installation, development environments, recommended code structure, object-oriented programming and some reallyexcellent chapters on code style. Peng About R Programming for Data Science PDF: Learn how to use R to turn raw data into insight, knowledge, and understanding. Ethics and Data Science Author: DJ Patil, Hilary Mason, and Mike Loukides About Ethics and Data Science PDF: With this eBook, authors Mike Loukides, Hilary Mason, and DJ Patilexamine practical ways for making ethical data standards part of your work every day. It, therefore, covers enough theory to understand the techniques but doesn’t assume an existing mathematical background. An Introduction to Statistical Learning, 2nd Edition Authors: Gareth James, Daniela Witten, Trevor Hastie & Rob Tibshirani About AnIntroduction to Statistical Learning, 2nd Edition PDF: An Introduction to Statistical Learning provides a broad and less technical treatment of key topics in statistical learning. If you are a beginner, then this book will get you started faster. Theory and Applications for Advanced Text Mining Author: Shigeaki Sakurai About Theory and Applications forAdvanced Text Mining: This book is composed of 9 chapters introducing advanced text mining techniques. Introduction to Statistical Thought Author: Michael Lavine About Introduction to Statistical Thought PDF: This free PDF textbook is intended as an upper level undergraduate or introductory graduate textbook in statistical thinking. The mainemphasis of this book is to help students think about the world in data science terms. Author: Christopher D. Wilke About Fundamentals of Data Visualization PDF: The book is meant as a guide to making visualizations that accurately reflect the data, tell a story, and look professional. In this post, You’ll see 100 free data science books for beginners,intermediate and experts. No understanding of computer science is assumed. Venables About Introduction to R – Notes on R, A Programming Environment for Data Analysis and Graphics PDF: This eBook provides a comprehensive introduction to R, a software package for statistical computing and graphics. It is a valuable resource for statisticiansand anyone interested in data mining in science or industry. Data Visualization: A Practical Introduction Author: Kieran Healy About Data Visualization: A Practical Introduction eBook: Data Visualization builds the reader’s expertise in ggplot2, a versatile visualization library for the R programming language. Happy Git and GitHub for the useR Author:Jim Hester About Happy Git and GitHub for the useR PDF: The target reader is someone who uses R for data analysis or who works on R packages, although some of the content may be useful to those working in adjacent areas. Hands-on Machine Learning and Big Data Author: Kareem Alkaseer About Hands-on Machine Learning and Big Data PDF:You’ll learn about the following things Learn how to clean your data and ready it for analysisImplement the popular clustering and regression methods in PythonTrain efficient machine learning models using decision trees and random forestsVisualize the results of your analysis using Python’s Matplotlib libraryUse Apache Spark’s MLlib package toperform machine learning on large datasets Mathematics of Data Science Author: Gabriel Peyré About Mathematical Foundations of Data Science PDF: This book presents an overview of important mathematical and numerical foundations for modern data sciences. Crash Course on Basic Statistics Author: Marina Wahl About Crash Course on BasicStatistics PDF: A Crash Course in Statistics by Ryan J. OpenIntro Statistics, 4th Edition Author: by David Diez, Mine Çetinkaya-Rundel, Christopher Barr About OpenIntro Statistics, 4th Edition PDF: There is more than enough material for any introductory statistics course. SQL Notes for Professionals Author: The StackOverFlow Community AboutSQL Notes for Professionals PDF: In the SQL Notes for Professionals, experienced SQL developers all over the world share their favorite SQL techniques and features. It will also be of interest to spatial data analysts who do not use R, but who are interested in practical aspects of implementing software for spatial data analysis. The SQL Notes forProfessionals book is compiled from Stack Overflow Documentation, the content is written by the beautiful people at Stack Overflow. Data Science at the Command Line, 2nd Edition Author: Jeroen Janssens About Data Science at the Command Line, 2nd Edition PDF: This hands-on guide demonstrates how the flexibility of the command line can helpyou become a more efficient and productive data scientist. Genetic Algorithms in Search, Optimization, and Machine Learning Author: David E. It is based in part on the authors blog posts, lecture materials, and tutorials. Exploring the Data Jungle Author: Brian Godsey About Exploring the Data Jungle PDF: Exploring the Data Jungle: Finding,Preparing, and Using Real-World Data is a collection of three hand-picked chapters introducing you to the often-overlooked art of putting unfamiliar data to good use. Advanced operators and techniques in genetic search. Written by pioneers in the field, this practical book presents an authoritative yet accessible overview of the methods andapplications of causal inference. With the fundamentals provided in this book, you will have a solid foundation on which to build your data science toolbox. Efficient R Programming: A Practical Guide to Smarter Programming Authors: Colin Gillespie and Robin Lovelace About Efficient R programming PDF: This hands-on book teaches novices andexperienced R users how to write efficient R code. Fundamental Numerical Methods and Data Analysis Author: George W. The book aims to teach data analysis using R within a single day to anyone who already knows some programming in any other language. Introduction to genetics-based machine learning. Learn how to predict system outputsfrom measured data using a detailed step-by-step process to develop, train, and test reliable regression models. With a wide range of detailed, worked examples using real epidemiologic data as well as software for replicating the analyses, the text provides a thorough introduction to the basics of the theory for non-time-varying treatments and thegeneralization to complex longitudinal data. Recommended Web Stories And Articles: Best Coding Practices for R Author: Vikram Singh Rawat About Best Coding Practices for R PDF: Most of the books about R programming language will tell you what are the possible ways to do one thing in R. Exploring Math for Programmers and Data ScientistsAuthor: Paul Orland About Exploring Math for Programmers and Data Scientists PDF: You’ll start with a look at the nearest neighbor search problem, common with multidimensional data, and walk through a real-world solution for tackling it. The Elements of Statistical Learning: Data Mining, Inference, etc Author: Trevor Hastie, Robert Tibshirani,Jerome Friedman About The Elements of Statistical Learning PDF: This book describes the important ideas in a variety of fields such as medicine, biology, finance, and marketing in a common conceptual framework. The student should have a basic understanding of statistical inference such as contained in “Statistical inference for data science”. TheHitchhiker’s Guide to Python Authors: Kenneth Reitz & Tanya Schlusser About The Hitchhiker’s Guide to Python PDF: This is an excellent book for all Python developers, both for beginners and more experienced users. This book provides rigorous training in the R language and covers modern software development practices for building tools that arehighly reusable, modular, and suitable for use in a team-based environment or a community of developers. Baumer, Daniel T. The material in this book is standard knowledge for any PhD in statistics or biostatistics. This book provides a guide to the many different regions of the R platform, with a focus on doing what is required of the Data Scientist.ggplot2 is a mini-language specifically tailored for producing graphics, and you’ll learn everything you need in the book. Foundations of Data Science Author: Avrim Blum, John Hopcroft, and Ravindran Kannan About Foundations of Data Science PDF: This book provides an introduction to the mathematical and algorithmic foundations of data science,including machine learning, high-dimensional geometry, and analysis of large networks. To exemplify how to do things, it uses R. Statistical rethinking with brms, ggplot2, and the tidyverse: Second edition Author: Solomon Kurz About Statistical rethinking with brms, ggplot2, and the tidyverse: Second edition eBook: This ebook is based on the secondedition of Richard McElreath’s (2020a) text, Statistical rethinking: A Bayesian course with examples in R and Stan. It covers a broad range of basic and advanced topics important in the analysis of high-throughput biological data, including principal component analysis and multidimensional scaling, clustering, multiple testing, unsupervised andsupervised learning, resampling, the pitfalls of experimental design, and power simulations using Monte Carlo, and it even reaches networks, trees, spatial statistics, image data, and microbial ecology. R Prog
So, just hit the share buttons and forward this list to other curious learners. PostgreSQL Notes for Professionals Author: The StackOverFlow Community About PostgreSQL Notes for Professionals PDF: This book is the definitive guide to undocumented and partially-documented features of the PostgreSQL server. We know these recipes are useful and