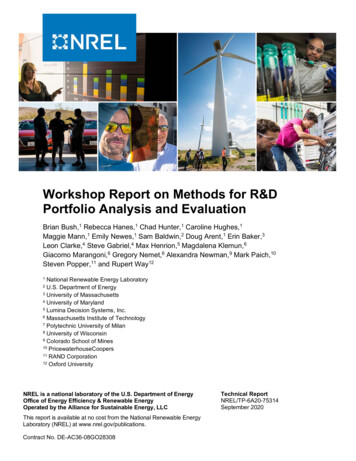
Transcription
Workshop Report on Methods for R&DPortfolio Analysis and EvaluationBrian Bush,1 Rebecca Hanes,1 Chad Hunter,1 Caroline Hughes,1Maggie Mann,1 Emily Newes,1 Sam Baldwin,2 Doug Arent,1 Erin Baker,3Leon Clarke,4 Steve Gabriel,4 Max Henrion,5 Magdalena Klemun,6Giacomo Marangoni,6 Gregory Nemet,6 Alexandra Newman,9 Mark Paich,10Steven Popper,11 and Rupert Way12National Renewable Energy LaboratoryU.S. Department of Energy3 University of Massachusetts4 University of Maryland5 Lumina Decision Systems, Inc.6 Massachusetts Institute of Technology7 Polytechnic University of Milan8 University of Wisconsin9 Colorado School of Mines10 PricewaterhouseCoopers11 RAND Corporation12 Oxford University12NREL is a national laboratory of the U.S. Department of EnergyOffice of Energy Efficiency & Renewable EnergyOperated by the Alliance for Sustainable Energy, LLCThis report is available at no cost from the National Renewable EnergyLaboratory (NREL) at www.nrel.gov/publications.Contract No. DE-AC36-08GO28308Technical ReportNREL/TP-6A20-75314September 2020
Workshop Report on Methods for R&DPortfolio Analysis and EvaluationBrian Bush,1 Rebecca Hanes,1 Chad Hunter,1 Caroline Hughes,1Maggie Mann,1 Emily Newes,1 Sam Baldwin,2 Doug Arent,1 Erin Baker,3Leon Clarke,4 Steve Gabriel,4 Max Henrion,5 Magdalena Klemun,6Giacomo Marangoni,6 Gregory Nemet,6 Alexandra Newman,9 Mark Paich,10Steven Popper,11 and Rupert Way12National Renewable Energy LaboratoryU.S. Department of Energy3 University of Massachusetts4 University of Maryland5 Lumina Decision Systems, Inc.6 Massachusetts Institute of Technology7 Polytechnic University of Milan8 University of Wisconsin9 Colorado School of Mines10 PricewaterhouseCoopers11 RAND Corporation12 Oxford University12Suggested CitationBush Brian, Rebecca Hanes, Chad Hunter, Caroline Hughes, Maggie Mann, EmilyNewes, et al. 2020. Workshop Report on Methods for R&D Portfolio Analysis andEvaluation. Golden, CO: National Renewable Energy Laboratory. sti/75314.pdf.NREL is a national laboratory of the U.S. Department of EnergyOffice of Energy Efficiency & Renewable EnergyOperated by the Alliance for Sustainable Energy, LLCTechnical ReportNREL/TP-6A20-75314September 2020This report is available at no cost from the National Renewable EnergyLaboratory (NREL) at www.nrel.gov/publications.National Renewable Energy Laboratory15013 Denver West ParkwayGolden, CO 80401303-275-3000 www.nrel.govContract No. DE-AC36-08GO28308
NOTICEThis work was authored in part by the National Renewable Energy Laboratory, operated by Alliance for SustainableEnergy, LLC, for the U.S. Department of Energy (DOE) under Contract No. DE-AC36-08GO28308. Fundingprovided by U.S. Department of Energy Office of Efficiency and Renewable Energy Strategic Priorities and ImpactAnalysis Office. The views expressed herein do not necessarily represent the views of the DOE or the U.S.Government.This report is available at no cost from the National RenewableEnergy Laboratory (NREL) at www.nrel.gov/publications.U.S. Department of Energy (DOE) reports produced after 1991and a growing number of pre-1991 documents are availablefree via www.OSTI.gov.Cover Photos by Dennis Schroeder: (clockwise, left to right) NREL 51934, NREL 45897, NREL 42160, NREL 45891, NREL 48097,NREL 46526.NREL prints on paper that contains recycled content.
List of RANDSTREAMSEDSTRANSIMSU.S.UMCPUSGCRPAmerican Association for the Advancement of ScienceComité permanent inter-Etats de lutte contre la sécheresse dans le SahelCritical Infrastructure Protection Decision Support SystemColorado School of MinesGerman Institute for Economic ResearchU.S. Department of EnergyOffice of Energy Efficiency & Renewable EnergyEnvironmental Protection AgencyInstitute for Data, Systems, and SocietyInterdependent Energy Infrastructure Simulation SystemIntegrative Graduate Education and Research TraineeshipInstitute for Operations Research and the Management SciencesIntergovernmental Panel on Climate ChangeLos Alamos National Laboratorylearning by doingMassachusetts Institute of TechnologyNational Atmospheric and Oceanic AdministrationNational Renewable Energy LaboratoryNorwegian University of Science and TechnologyOffice of Science and Technology PolicyOffice of Technology AssessmentPacific Gas & Electricresearch and developmentResearch and DevelopmentSystematic Technology Reconnaissance, Evaluation and Adoption MethodologyStochastic Energy Deployment SystemTransportation Analysis Simulation SystemUnited StatesUniversity of Maryland-College ParkU.S. Global Change Research ProgramiiiThis report is available at no cost from the National Renewable Energy Laboratory (NREL) at www.nrel.gov/publications.
Executive SummaryMotivation: Risk and uncertainty are core characteristics of research and development (R&D)programs. Attempting to do what has not been done before will sometimes end in failure, just asit will sometimes lead to extraordinary success. The challenge is to identify an optimal mix ofR&D investments in pathways that provide the highest returns while reducing the costs offailure. The goal of the R&D Pathway and Portfolio Analysis and Evaluation project is todevelop systematic, scalable pathway and portfolio analysis and evaluation methodologies andtools that provide high value to the U.S. Department of Energy (DOE) and its Office of EnergyEfficiency & Renewable Energy (EERE). This work aims to assist analysts and decision makersidentify and evaluate, quantify and monitor, manage, document, and communicate energytechnology R&D pathway and portfolio risks and benefits. The project-level risks typicallyconsidered are technology cost and performance (e.g., efficiency and environmental impact),while the portfolio level risks generally include market factors (e.g., competitiveness andconsumer preference).The Workshop: The Workshop on Methods for R&D Portfolio Analysis and Evaluationconvened July 17–18, 2019, at the National Renewable Energy Laboratory in Golden, Colorado,and it examined strengths and weaknesses of the various methodologies applicable to R&Dportfolio modeling, analysis, and decision support, given pragmatic constraints such as dataavailability, uncertainties in estimating the impact of R&D spending, and practical operationaloverheads. Participants employed their deep expertise in approaches such as stochasticoptimization, real options, Monte Carlo analysis, Bayesian networks, decision theory, complexsystems analysis, deep uncertainty, and technology-evolution modeling to critique the initialexample models developed by the project’s core team and to conduct thought experimentsgrounded in real-life technology models, progress data, expert elicitation, and portfolioinformation. This engagement of participants’ methodological expertise with the practicalrequirements of real-life portfolio decision support yielded ideas for improved approaches,alternative methodological hypotheses, and hybridization of methodologies that are wellgrounded theoretically, computationally sound, and realistically executable given dataavailability and other practical constraints. These ideas will be explored in the subsequentresearch following this workshop.Major Challenges: A variety of challenges were identified in work leading up to this workshop,including addressing proprietary and competitiveness concerns; establishing consistent protocolsacross risk analysts and external experts; assessing and addressing correlations and dependencieswithin and between technologies; avoiding biases such as overconfidence, confirmation, andmotivation; parsing projected costs due to R&D, learning, commodity price changes, etc.;optimizing multiple, sometimes conflicting, criteria such as economic cost, environmentalpollution, greenhouse gas emissions, materials use, reliability, robustness, and resiliency; andothers. Furthermore, these analyses were and must be done in the context of deep uncertaintyabout many of the resources, technologies, markets, competitors, and numerous other factors.How risks might be perceived were also of concern: for example, if one R&D investment hadonly a 10% chance of success and another had 70% but with a smaller potential payoff than thefirst, how would decision makers respond? If key benefits of a technology are not captured inhigh-level portfolio evaluations—for instance, if the evaluation considered only cost and notbroader metrics such as temporal and spatial availability, economic impact, or consumerivThis report is available at no cost from the National Renewable Energy Laboratory (NREL) at www.nrel.gov/publications.
preferences—this could substantially misrepresent the value of particular R&D investments. Thefollowing discussion of key findings from the workshop generally confirmed the significance ofthese challenges, amplified areas of concern, and suggested avenues of research and potentialsolutions.Key Issues and Discussion: Many of the major discussion issues raised by the invitedparticipants involved better aligning modeling and analysis activities with requirements for R&Dinvestment decision support. Models should have transparency in their assumptions and structureand treat the major determinants of R&D progress, including non-hardware or “soft” costs.Bottom-up technology-cost models were identified as a useful starting point for the developmentof more complex (e.g., combined) modeling approaches. Computations should estimate not onlythe basic economy, technology, and energy metrics, but also encompass market, societal, andqualitative impacts. There exists a pressing need for significantly improved data sources andestimation techniques to better understand the relationships between R&D investment levelsand specific technological improvements.Participants also emphasized the importance of expert elicitation as another primary foundationalinput to the technology cost and performance modeling. Elicitations require deliberate framing,employment of bias-reduction techniques, and careful synthesis. Advances in expert-elicitationresearch over the past decade and recent experiments with new elicitation modalities promisesubstantial improvements in the quality of these difficult elicitations for R&D investmentimpacts, but further investigation and evaluation of online techniques pre-elicitation interactionof experts, allowance for feedback (for example showing R&D solutions to decision makers,then iterating to adjust the selection of optimal portfolios), aggregation methods, and framing isrequisite. In particular, the hypothesis that technology experts may provide better informationusing learning rates (or individual components of experience curves) and odds ratios rather thancurrent costs and probabilities, especially conditional ones, requires testing. Initial experimentsby several of the participants indicate the potential for online expert elicitations to provide resultscomparable to in-person expert elicitations while reducing costs and logistical challenges, butmay require more extensive testing and quality control of the elicitation survey tool (Baker et al.2019). Further experimentation comparing on-line and in-person expert elicitations in the contextof the present study would be useful.Conscientiously accounting for and communicating uncertainty in R&D project and portfolioevaluation is critical. Expected outcomes, distributional information (e.g., error bars, quantiles,and tornado plots), and measures of regret (via the “minimax” principle) should be estimatedusing ensemble methods in a real-options and deep-uncertainty context to develop robuststrategies that support decision-making. Two-stage stochastic, multi-objective optimization cancomprise the primary computational technique used to develop such strategies. Multistageoptimization techniques beyond two-stage optimization were deemed by participants as notproviding sufficient additional information to justify their increased computational intensity.Scenario-based analysis and techniques for decision-making under deep uncertainty complementstochastic optimization approaches. When probability distributions for the uncertain factors areunavailable, robust optimization is another option.vThis report is available at no cost from the National Renewable Energy Laboratory (NREL) at www.nrel.gov/publications.
Any decision-support tool for R&D investment should assist decision makers in discovering andinterpreting information that they might otherwise overlook or misinterpret, provide a relativelysmall set of critical criteria on which decisions can be made, and adapt to the decision-makingstyle and concerns of the users. Presenting decision makers with a set of satisfactory portfolios inoptimal risk-informed visualizations comprising both influence diagrams and quantitative plots(including those showing Pareto optimality frontiers), rather than presenting one optimal answer,can assist them in robust decision-making that engenders trust through increased transparencyand builds intuition over complex dimensional spaces to inform decision-making. This isparticularly important to decision makers who might be disinclined towards probabilisticanalysis or when specific probability distributions are not readily available. Tools must allowdecision-makers to alter input parameters and assumptions interactively and immediately viewupdated results: this entails having fast-running analytic models.Supplemental Material: The appendix to this report include biographies of the workshopattendees, revised copies of the material presented at the workshop, fact sheets describingexploratory analyses that raise methodological issues, and an extensive bibliography of portfolioanalysis literature.viThis report is available at no cost from the National Renewable Energy Laboratory (NREL) at www.nrel.gov/publications.
Table of ContentsIntroduction . 1Challenges. 2Approaches to Decision Support . 3Technology Analysis . 3Model Design .3Experience Curves and Learning by Doing .4Handling Uncertainty .4Analysis Methodology .5Data Gathering .5Expert Elicitation . 6Portfolio Analysis . 7Metrics .7Markets and Policy .8Communication and Interaction with Stakeholders .8References. 10Appendix . 11Workshop Prospectus.11Workshop Agenda .12Biographies of Attendees .13Workshop Presentations .20Fact Sheets.20R&D Pathway and Portfolio Analysis and Evaluation: Overview .21Stochastic Energy Deployment System (SEDS) .28Toy Biorefinery Model Fact Sheet.32Polysilicon Cell Cost Model Fact Sheet .36Real Options Toy Model .40Monte Carlo Toy Model .47Modeling Technology Readiness and Performance Levels .54Simple Petri Net Model for Dual-Junction III-V PV.59Bayesian Combination of Expert Assessments .64Expert Elicitation Issues Fact Sheet.68Bibliography on Portfolio Analysis .70viiThis report is available at no cost from the National Renewable Energy Laboratory (NREL) at www.nrel.gov/publications.
IntroductionThis report summarizes the key discussions and ideas generated at the Workshop on Methods forR&D Portfolio Analysis and Evaluation, convened on 17–18 July 2019 at the NationalRenewable Energy Laboratory in Golden, Colorado. The goal of the R&D Pathway and PortfolioAnalysis and Evaluation project is to assist funding decision-making across technology pathwaysand portfolios by developing methodologies and tools for systematic, scalable pathway andportfolio analysis and evaluation. Such tools will provide high value to the U.S. Department ofEnergy (DOE) and the Office of Energy Efficiency & Renewable Energy (EERE) by assistinganalysts and decision makers in identifying, evaluating, quantifying, monitoring, managing,documenting, and communicating the risks and benefits of prospective energy technology R&Dpathways and portfolios. Key questions that these methodologies and tools must help analystsand decision makers address include the following: Where should the next dollar of R&D be invested to increase the likelihood of achievingdesired returns at the project and portfolio levels?o How impactful will specific investments be in advancing a particular technology?o What is the likelihood that particular R&D pathways will achieve their goals?o At what point should R&D investment be cut or alternative pathways explored?o What are the opportunity costs of not investing in a research pathway?o What are ideal balances between supporting fewer projects with more resources asopposed to a wider range of projects with fewer resources? How should the portfolio be balanced taking into consideration risk, return, time,technology mix, and markets?How can risk scoring be made more consistent across projects, portfolios, markets, expertelicitations, and time?How can the results of these analyses be quantified and validated? Are the resultsstatistically significant and reproducible, and are they robust when audited by decisionmakers and external experts?What are the most effective mechanisms for communicating these evaluations in differentcontexts of decision-making?Addressing these questions can provide significant value by helping decision-makers target R&Dopportunities, thereby accelerating the pace of technology development while meetingstakeholder-defined objectives, such as cost, efficiency, environmental impact, etc. They mayalso help external stakeholders to better understand and assist EERE and DOE R&D decisionsand activities.1This report is available at no cost from the National Renewable Energy Laboratory (NREL) at www.nrel.gov/publications.
ChallengesThe following issues were emphasized by workshop participants.Technology modeling: Numerous tradeoffs must be considered and many modeling decisionsmust be made in constructing appropriately detailed technology models in support of pathwayand portfolio analysis. Modeling challenges are exacerbated by the uncertain techno-economicinput data (of varied quality) for speculative, nascent, and even established technologies. In orderfor technology modeling to be tractable, it must focus on the points of leverage for R&Dinvestment—points of leverage which in many cases are poorly known and must be determinedin consultation with experts and from exploratory analysis—and on metrics relevant for decisionmaking stakeholders.Analysis approaches: Decision support analyses must account for the considerable uncertaintiesregarding techno-economic input parameters to models, model structure, and the response of thestate of technology to R&D investments. A pragmatic method for R&D portfolio decisionsupport must be constructed from the numerous approaches proposed in the academic literatureor applied in other practical application areas. It is not obvious whether a single approachadequately meets the requirements for the type of problems considered here or whether ahybridization of techniques can combine the strengths of several methods while avoiding theirweaknesses. For instance, some methods rely extensively on propagating probabilitydistributions that originate from expert elicitations whereas other eschew distributionalassumptions. The computational resources and runtime of methods vary by orders of magnitude.Expert elicitation: Past efforts have highlighted both the necessity and challenges of elicitingexpert opinions in support of technological forecasts, but there is much active research anddiffering schools of thought in this area. Primary challenges are the intensity of effort (overheadand resources) required by some elicitation methods, the need to correct experts’ cognitive biasessuch as overconfidence and confirmation, and the selection of precise elicitation questions thatyield ranges or distributions. Emerging variations or alternatives to classical expert elicitationsuch as on-line methods, patent analysis, and historical data may warrant consideration.Data collection: Techno-economic data on R&D pathways and historical data on thosepathways’ progress complements the results of expert elicitation but can be similarly difficult togather and harmonize. In particular, detailed correlations between past R&D investments andprogress in specific determinants of technology performance would be invaluable for futuretechnology forecasts. Both timeliness and detail in data pose challenges.Portfolio analysis: Perhaps inevitably, some technology system or subsystem models may be farmore detailed than others, a situation which poses challenges for meaningful consistentcomparisons of disparate technologies. There is a risk that lack of information will unfairly biasportfolio decisions towards or away from emerging or high-risk technologies. Portfolio-leveldecisions may require the simultaneous consideration of a disparate variety of hard and softmetrics, the evaluation of numerous technology models across multiple renewable-energy andenergy-efficiency domains, and the treatment of a diversity of levels of maturity.2This report is available at no cost from the National Renewable Energy Laboratory (NREL) at www.nrel.gov/publications.
Communication of results: The variety of decision-making questions, styles, and contextschallenges the creation of tools informing decisions. Complex risk analysis may require complexvisualizations and intensive computation, but streamlined, intuitive, and rapid presentation ofresults may be most effective for decision support. Tools may be designed to be run interactivelyversus in batch mode, individually versus collectively, for point estimates versus probabilisticones, on single versus multiple metrics, or prospectively versus retrospectively.Approaches to Decision SupportDecisions must be framed carefully, with agreement between model-builders, analysts, anddecision makers on what question is being asked and what decision is being made. Agreement onand transparency around which basic assumptions are to be used in making the decision is alsocritical. Any re-framing of decisions must be done carefully and deliberately, with transparencyaround any changes in assumptions. This clarity is necessary to determine the scope and level ofdetail required in the modeling effort.A decision support tool should assist decision makers in discovering and interpreting informationthat they might otherwise overlook or misinterpret. The tool should provide a relatively small setof critical criteria on which decisions can be made. These criteria can be expressed asexpectations over probability distributions of uncertain model inputs and parameters or as regretrepresenting lost opportunities or opportunity cost. Both types of criteria will aid decisionmakers in understanding the long-term consequences, positive and negative, of specific decisionsand short-term actions. In addition to the critical criteria, a decision support tool should be ableto account for institutional lock-in and be flexible enough to inform decisions made amongst asubset of available options.Technology AnalysisModel DesignLevel of detail: Attendees agreed that models should be computationally tractable and capturethe most significant points of leverage for R&D investment and the metrics required fordecision-making. There was no explicit agreement regarding the level of detail to include in themodels. Model tractability, data availability, and user preferences were discussed as importantcriteria.Bottom-up approach: There were some advocates for starting with simple, top-down modelingand perhaps including more detail as the importance of individual components or subcomponentsbecomes apparent. A predominance of attendees advised bottom-up, cost modeling, wherebymodels represent the impact of engineering properties and other technology characteristics on thecost of components and subsystems. Both engineering- and physics-based models can serve asstarting points for further analysis. The level of technical detail should be adjusted to theavailability of data and the metrics relevant to decision makers. Key considerations for this arethe synergies between expert elicitation and model building. Workshop discussion advocatedexploring how to effectively merge elements of these two approaches using existing work andhow to balance these efforts to minimize overhead resources.3This report is available at no cost from the National Renewable Energy Laboratory (NREL) at www.nrel.gov/publications.
Staged decisions: Workshop attendees encouraged focusing on influential components, asrepresented graphically through tornado diagrams, and removing fixed or non-impactfulcomponents from the model in order to more clearly compete potential investments in the moreinfluential components. Decision-making might proceed in stages, with the most impactfulportfolio-level decisions being made first.Experience Curves and Learning by DoingExperience curves and learning by doing are important to consider in evaluating R&D impactsby setting a baseline for expert elicitation and when evaluating how the cost of a technology willadapt post R&D. Discussion in the workshop examined the importance of these experiencecurves from many perspectives: the choice of dependent and independent variables for thecurves; the availability of data; the techniques and quality of statistical models for experiencecurves; and uncertainties associated with them.Soft costs: Soft costs, which can be encompassed in learning curves, include labor such asmarketing and sales for customer acquisition, permitting, and installation, and are important toconsider. These are more likely to vary regionally when compared with hard costs since learningis local, and information transfers as people move and companies expand (Nemet 2019).Experience curves and learning by doing (LBD): Some discussion supported directlymodeling the impact of investments on experience curves and including this in learning rates.Challenges of this approach include determining the appropriate learning rate baselines for noveltechnologies and at later stages of R&D and commercialization. Thus, it is practical to includeuncertainty bands when examining learning curves, to assign maximum and minimum potentiallearning based on past measurements along the curve (Lafond, et al., 2018).Handling UncertaintyUncertainty inherent to forecasting future events is a primary source of uncertainty in R&DPathway and Portfolio Analysis. Representing
IGERT Integrative Graduate Education and Research Traineeship . . UMCP University of Maryland-College Park . . (R&D) programs. Attempting to do what has not been done before will sometimes end in failure, just as it will sometimes lead to extraordinary success. The challenge is to identify an optimal mix of