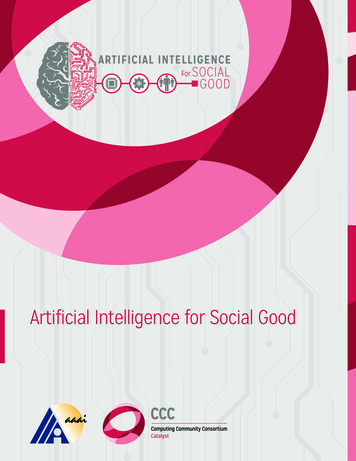
Transcription
Artificial Intelligence for Social Good
This material is based upon work supported by theNational Science Foundation under Grant No. 1136993.Any opinions, findings, and conclusions orrecommendations expressed in this material are thoseof the authors and do not necessarily reflect the viewsof the National Science Foundation.
Artificial Intelligence for Social GoodGregory D. Hager, Ann Drobnis, Fei Fang, Rayid Ghani, Amy Greenwald, Terah Lyons,David C. Parkes, Jason Schultz, Suchi Saria, Stephen F. Smith, and Milind TambeMarch 2017Sponsored by the Computing Community Consortium (CCC)
ARTIFICIAL INTELLIGENCE FOR SOCIAL GOODOverview.1Urban Computing.1Sustainability. 3Health. 8Public Welfare.11Cross Cutting Issues.15
OverviewArtificial Intelligence (AI) is currently seeing major media interest, significant interest from federal agencies, and interest fromsociety in general. From its origins in the 1950s, to early optimistic predictions of its founders, to some recent negative views putforth by the media, AI has seen its share of ups and downs in public interest. Yet the steady progress made in the past 50-60years in basic AI research, the availability of massive amounts of data, and vast advances in computing power have now broughtus to a unique and exciting phase in AI history. It is now up to us to shape the evolution of AI research.AI can be a major force for social good; it depends in part on how we shape this new technology and the questions we use toinspire young researchers. Currently there is a significant spotlight on the future ethical, safety, and legal concerns of futureapplications of AI. While understanding and grappling with these concerns, and shaping the long-term future, is a legitimateaspect of future AI research and policy making decisions, we must not ignore the societal benefits that AI is delivering and candeliver in the near future, and how our actions today can shape the future of AI.The Computing Community Consortium (CCC), along with the White House Office of Science and Technology Policy (OSTP), andthe Association for the Advancement of Artificial Intelligence (AAAI), co-sponsored a public workshop on Artificial Intelligence forSocial Good on June 7th, 2016 in Washington, DC. This was one of five workshops that OSTP co-sponsored and held around thecountry to spur public dialogue on artificial intelligence, machine learning, and to identify challenges and opportunities related toAI. In the AI for Social Good workshop, the successful deployments and the potential use of AI in various topics that are essentialfor social good were discussed, including but not limited to urban computing, health, environmental sustainability, and publicwelfare. This report highlights each of these as well as a number of crosscutting issues.Urban ComputingUrban computing pertains to the study and application of computing technology in urban areas. As such, it is intimately tiedto urban planning, specifically infrastructure, including transportation, communication, and distribution networks. The urbancomputing workshop session focused primarily on transportation networks, the goal being to use AI technology to improvemobility and safety. We envision a future in which it is significantly easier to get people to the things they need and the thingsthey want, including, but not limited to, education, jobs, healthcare, and personal services of all kinds (supermarkets, banks, etc.).Time spent commuting to school or to work is time not spent working, studying, or with one’s family. When people do nothave easy access to preventative healthcare, later costs to reverse adverse developments can far exceed those that wouldhave been incurred had appropriate preventative measures been applied (Preventive Healthcare, 2016). Lack of easy access tosupermarkets with healthful food is highly correlated with obesity (and hence heart disease, diabetes, etc.) (Studies Question thePairing of Food Deserts and Obesity, 2012). Likewise, lack of easy access for many people to standard bank accounts is costly(Celerier, 2014). AI technology has the potential to significantly improve mobility, and hence substantially reduce these and otherinefficiencies in the market to make daily living easier.AI is now in a position to drive transformations in transportation infrastructure in urban areas. Technology exists that can mobilizepeople who have been immobile, due to a lack of availability of inexpensive transport; to increase flow/decrease congestion,thereby decreasing mean travel time requirements as well as variance (a great source of stress for many) (Commuting: TheStress that Doesn’t Pay, 2015); and autonomous vehicles have the potential to decrease emissions (less speeding up and slowingdown). The easier it becomes for people to move about, the more vibrant our urban areas will be; likewise, the more fruitful thesocial and economic interactions that take place inside them will be.1
ARTIFICIAL INTELLIGENCE FOR SOCIAL GOODTechnology Enablersmodels will impact big cities. Fundamental to this effort,it is crucial that we understand the human behavioralchanges that new forms of mobility will induce, andthe impact those behaviors will have on the efficacy ofour systems.The coming transformation in transportation infrastructure is being powered by technological progress.Ubiquitous connectivity and instrumentation areenabling us to measure things that were previouslyimmeasurable; additionally, advances in data analyticsare enabling us to build sophisticated models fromthose data. Specifically, we can now collect informationabout individuals’ travel patterns, so that we can betterunderstand how people move through cities, therebyimproving our understanding of city life. AI technologycan then be leveraged to move from descriptive models(data analytics) to predictive ones (machine learning) toprescriptive decisions (optimization, game theory, andmechanism design). Like in other domains, AI enables usto go from “data to decision” in urban computing. Withthe data collections now happening at this scale to aidin decision-making, it is important to also consider theprivacy implications around the data.Evidence-based Policy MakingAI, as it pertains to urban computing, is in a uniqueposition to inform policy making in ways that could notbe envisioned even a few years ago. It is now possibleto carry out interventions that will help us understandmobility at scale, and to analyze how different segmentsof the population vary their transportation modesin response to various interventions. Consequently,we are in a position to conduct research that caninform regulators, prior to the full implementation oftransportation and urban planning policies. What isneeded, however, is to lower the standards for testingnovel AI technologies and transportation models, whichmay well require that we first find a way to addressthe psychological concerns raised by the radicaltransformations they promulgate.The potential of this transformation is beingdemonstrated in pilot systems that optimize the flowof traffic through cities, and in new on-demand, multimodal transportation systems. It is now within the realmof AI technology to optimize traffic lights in real time,continuously adapting their behavior based on currenttraffic patterns (Smith, 2016); and to dispatch fleets ofsmall vehicles to provide on-demand transportation,address the “first and last mile” problem that plaguesmany urban transit systems (Van Hentenryck, 2016).More pilot deployments are needed to fully understandthe scope of the transformation that is under way inour cities.Case Study: Real-Time, Adaptive Traffic SignalControl for Urban Environments.In US cities alone it is estimated that trafficcongestion costs over 160 Billion annually inlost time and fuel consumption (Schrank, 2015).Traffic congestion is also responsible for puttingan additional 50 Billion tons of CO2 annually intothe atmosphere. A major cause of this congestionis poorly timed traffic signals. The vast majorityof traffic signals run “fixed timing” plans, whichare pre-programmed to optimize for averageconditions observed at a particular snapshot intime and never change. These plans regularlyperform sub-optimally since actual traffic flowsare frequently quite different than averageconditions, and they quickly become outdatedover time as traffic flow patterns evolve.Technical ChallengesIn spite of the significant promise, many challengeslie ahead before these new opportunities can be fullyrealized. Transportation systems are complex, sociotechnical systems that operate over multiple spatialand temporal scales. It is critical that we scale upexisting pilots to multi-modal transportation models– incorporating pedestrians, bicycles, cars, vans, andbuses – so that we can begin to understand how theseRecent work by Stephen Smith and his researchgroup at Carnegie Mellon University has been2
Figure 1: Scalable URban TRAffic ControlCurrent research with Surtrac focuses onintegration of smart signal control with emergingDedicated Short Range Communication (DSRC)radio technology (Smith, 2016). This “connectedvehicle” technology, which will begin to appear insome makes of new passenger vehicles in the USstarting in the 2017 model year, will allow direct“vehicle-to-infrastructure” (V2I) communication.In addition to simple use of V2I communicationto promote safer travel (e.g., through advancewarning of pending signal changes), projectsaimed at utilizing V2I communication to enhanceurban mobility (particularly under the shorter-termassumption that the penetration level of equippedvehicles is low) are also underway.applying AI techniques for online planning andscheduling to the problem of real-time trafficsignal control, leading to development of theSurtrac (Scalable URban TRAffic Control) adaptivesignal control system (see Fig. 1) (Smith, 2013).Surtrac senses approaching traffic and allocatesgreen time to different approaches in real-time.It is designed specifically for optimizing trafficflows in complex urban road networks wherethere are multiple, competing dominant flowsthat shift dynamically through the day. An initialdeployment of the Surtrac technology in the Eastend area of Pittsburgh PA has produced significantperformance improvements, reducing travel timesthrough the network by 25%, wait times by over40%, and emissions by 21% (Smith, 2013). Over thepast 3 years, this Pittsburgh deployment has grownto an interconnected network of 50 intersections,and the City of Pittsburgh currently has plans andfunds in place to further expand and equip anadditional 150 intersections with this technology.SustainabilitySustainability can be interpreted narrowly as theconservation of endangered species and the sustainablemanagement of ecosystems. It can also be interpreted3
ARTIFICIAL INTELLIGENCE FOR SOCIAL GOODbroadly to include all aspects of sustainable biological,economic, and social systems that support humanwell being. Here we focus primarily on the ecologicalcomponent, but the larger issues of social and economicsustainability must be considered as well.DataSeveral activities concern the measurement andcollection of data relevant to ecosystems.One approach is to develop and deploy sensornetworks. For example, the TAHMO (Trans-Africa HyrdoMeteorological Observatory; www.tahmo.org) project isdesigning and deploying a network of 20,000 weatherstations throughout sub-Saharan Africa (van de Giesen,2014). Several efforts are deploying camera traps tocollect image data or microphone systems to collectbioacoustic data. Still other projects employ unmannedaerial vehicles to obtain video imagery for trackingelephants and other large animals. AI algorithms can beapplied to optimize the locations of these sensors andtraps in order to gather the most valuable informationShort-term Applications and ChallengesCurrent research and applications in AI for sustainabilitycan be organized in terms of data, modeling, decisionmaking, and monitoring. The goal is to manageecosystems with policies that are based on the highquality data and science.Figure 2: Image-Based Ecological Information System4
goal is to understand which climate variables affectspecies habitat carrying capacity.at the lowest cost. Once the data are collected, other AIalgorithms can be applied to identify species and tracktheir locations.A second important type of model seeks to characterizethe spatio-temporal dynamics of species. Such modelscan predict migration, dispersal, reproduction, andmortality of species. Developing such dynamicalmodels is critical to developing policies that can helpendangered species thrive and control the spread ofinvasive species. One recent example is the BirdCastbird migration model under that combines eBird andweather radar to test hypotheses about the behaviorof migrating birds (birdcast.info).A second approach to data collection is to engage citizenvolunteers. One of the oldest citizen science projects iseBird (www.ebird.org), in which bird watchers uploadchecklists of the birds they have seen at a particulartime and place. The Image-Based Ecological InformationSystem (IBEIS; www.ibeis.org) project analyzes animalphotos scraped from internet sources such as Flickrand Facebook and applies computer vision and activelearning methods to detect the animals, identify thespecies, and even identify individual animals (“Bob thegiraffe”) (see Fig. 2). Their AI techniques can identifyunique animals as long as they have stripes, wrinkles,or other unique textures.Policy OptimizationOnce we have models of species distribution, behavior,and habitat requirements, we can begin to designand optimize policies for successful management ofspecies and ecosystems. This requires articulating ourpolicy goals and objectives. Virtually every ecosystemmanagement problem combines an ecological model withan economic model of the economic costs and benefitsof various policy outcomes.A third approach employs technically trained people(e.g., government and corporate scientists) to collectdata. One example of this is the freshwater streamsurveys conducted by the EMAP project of the html/), and similar efforts by forest resource companiessuch as Weyerhauser about-our-forests/uswest/). These groups collect samples of freshwatermacroinvertebrates that live in streams. These insectsmust then be examined by humans to identify the genusand species of each. AI computer vision methods arenow being applied to accelerate this process.One example is the design of a schedule for purchasinghabitat parcels to support the spatial expansion of theRed Cockaded Woodpecker (Sheldon, 2010; Sheldon, 2015).An optimal policy takes into account uncertainty in thespread of the species and the availability of land parcelswhile seeking to link up existing patches of reservedhabitat. Algorithms for computing this policy combineideas from network cascade analysis (maximizing spreadin social networks) with techniques from AI planning andMonte Carlo optimization.ModelsAfter data are collected, the data can be analyzed byapplying techniques from data mining, statistics, andmachine learning to discover trends and fit models. Forspecies data, most efforts begin by counting individualsin order to produce estimates of population size andmaps of the spatial distribution of species. For thispurpose, it is particularly valuable to identify individualsand take into account multiple detections of thesame individual across time and space. These modelscan support some inferences about species habitatrequirements. In light of climate change, an importantA second example considers the temporary needsof migrating birds. Instead of making a permanentpurchase of land, the Nature Conservancy is applyingdetailed bird migration models developed by the CornellLab of Ornithology to rent rice fields in California(Axelson, 2014). The farmers who own those fields agreeto flood them at the right time to support migratingwaterfowl in the pacific flyway. The exact timing variesfrom year to year based on the predictive migration5
ARTIFICIAL INTELLIGENCE FOR SOCIAL GOODmodels developed using multi-scale machine learningtechniques (cite STEM).game theoretic models, this work assumes thatthe adversary behavior is governed by modelsderived from past adversary behavior. The use ofsecurity resource allocation using game theoryhas been explored in urban contexts, particularlyin counter-terrorism settings in the past (Tambe,2011). The current work builds on this past workwhile also significantly enhancing it with machinelearning based predictions of adversary behaviors.A third example of policy development confronts theissue of long-term planning in the face of climatechange and sea level rise. One approach, known asadaptive management, explicitly considers the need toupdate and revise models based on new data that willbecome available in the future. There is considerableuncertainty about the timing and degree of sea levelrise. Nicol, et al., (2015) formalize the problem ofplanning in the presence of this uncertainty. Theirgoal is to conserve appropriate coastal habitat formigrating birds under the risk of sea level rise. Lowlying land will become inundated, and migrating birdswill be threatened unless additional habitat furtherinland is available for them. As always, there are tightbudgetary constraints on the amount of land thatcan be purchased. AI algorithms for solving PartiallyObservable Markov Decision Problems are able to solvethese difficult constrained optimization problems(Pineau, 2003).Opportunities and Challenges in theMedium TermWith few exceptions, most work in ecosystemmanagement and conservation focuses on a smallnumber of species in particular regions. A majorchallenge for the medium term is to develop methodsthat can collect and model data encompassing a broadrange of species at continental scales. This will requireintegrating many different data sources (e.g., for birds,fish, plants, and insects) collected by many differentmethods (e.g., stationary sensors, earth orbit satellites,citizen scientists, sensors worn by animals, and so on).There are many research issues in data management anddata integration that must be addressed. For example,the most common approach to data integration is toassimilate all data to a fixed spatial and temporal scaleby smoothing fine-scale data and interpolating coarsescale data. This process introduces distortions into thedata. We need methods for integrating and modelingdata at multiple scales that can retain the resolutionand uncertainty associated with each data source.Case Study: Monitoring and EnforcementWhen policies are put into effect, there is oftena need for law enforcement to ensure theirsuccessful execution and for monitoring to detecterrors in the data and models that require reoptimizing the policy. For example, elephantpoachers in Africa routinely enter national parksand other bioreserves to hunt elephants illegallyfor the ivory tusks. The PAWS project (Fang, 2016;Nguyen, 2016; Yang, 2014) applies AI algorithms topredict poaching attacks and optimize the patrolroutes of game wardens in order to maximizetheir deterrent effect while minimizing costs.PAWS relies on two key underlying systems: (i)Predicting poacher behavior from past poachingdata: This system builds poacher behavior modelsusing machine learning algorithms (Nguyen, 2016);(ii) Game theoretic security resource allocation:Using these learned poacher behavior models, ituses game theoretic algorithms to prescribe newpatrolling strategies; thus, rather than assuming thestandard rational adversary response in standardA related shortcoming of current modeling efforts isthat they generally assume stationary (steady-state)climate, land use, and species behavior whereas thereal systems are experiencing climate change, rapideconomic development, and continuing evolution,dispersal, and natural selection of species. Modelingtechniques and supporting data are needed that cantake into account these drivers of change and the manyuncertainties associated with them.As the scale of questions grow, it is no longer possibleto focus only on the biological components of a6
system. Instead, one must take a “systems of systemsapproach” and incorporate models of social, cultural,and economic activity. For example, when choosinga site for a new dam, we must consider not only theimpact on native and invasive species in the riverineecosystem, but also the benefits for farming, thepotential inundation of important cultural and religioussites, and changes in sediment transport that mayaffect the distribution of pollutants and contaminatedsoils. Current AI technologies cannot currently operateat this scale and level of complexity.A third challenging aspect of sustainability work arisesdue to lack of technical infrastructure: poor networking,little access to high-performance computing resources,and lack of local personnel with sufficient educationand training. We must develop algorithms that can runlocally on small computers (or telephones) that onlyhave intermittent access to large cloud computingresources. We must take into account the possibilitythat human actors may fail to adhere to designatedpolicies. Finally, we must develop creative methods ofestablishing metrics for assessing the effectiveness ofdata collection and policy execution to compensate forthe lack of historical data.One trend that will enable broader and morecomprehensive modeling of ecosystems is thecontinuing improvement of sensors: reduction in size,power requirements, and cost. These improvementswill support and drive the demand for better modelsthat can support the development of higher-qualitypolicies. However, cheaper sensors can be less reliable,so research is needed on methods for automaticallydetecting and removing bad data and broken sensors.This is a theme that is also common to other areas suchas healthcare and public policy, as we discuss below,and poses a major challenge for many AI-powereddecision-support systems.A fourth challenge is finding business models that supportlong-term data collection and policy enforcement efforts.Many current projects rely on the enthusiasm of citizenscientists, the generosity of private donors, or grantsfrom funding agencies. None of these is likely to providesteady, long-term support. One possibility is to developbusiness models that generate continuing revenuestreams. For example, the TAHMO project seeks to sellits weather data to insurance companies, commoditiestraders, and other businesses that rely on high qualityweather data and forecasts.A second set of data challenges concerns the biasesand quality of data, particularly crowd-sourced data.Birders choose where they go bird watching; touristsand tour operators choose where people take picturesof wildlife. Even the data collected by game wardensis biased by the need to maintain unpredictability.New incentive mechanisms are needed to encouragevolunteers to collect less biased data. Examples ofmechanisms that are showing some success includethe Great Zebra Challenge and the eBird Global BigDay. New algorithms are needed to incorporate datacollection goals into the PAWS enforcement games.And methods for explicitly modeling the data collectionprocess (“measurement models”) must be improved. Amajor analytical challenge is that when measurementmodels are incorporated into machine learning, thevariables of fundamental interest are no longer directlyobserved. This raises questions about the identifiabilityand semantics of the inferred values of those variables.Long Term ProspectsSustainability is concerned with the long-term health ofecosystems and human societies. As we contemplate thecreation and deployment of policies over the long term,we must confront the fact that the long-term behaviorof ecological, economic, and social systems is radicallyuncertain. We can be very confident that our currentmodels are missing critical variables and importantinteractions. How can artificial intelligence methods dealwith the uncertainty of these “unknown unknowns”?One important strategy is to plan for the “learningprocess”. When a new policy is put into place, we mustalso develop and deploy an instrumentation plan tocollect data on a broad range of variables. We mustincorporate “precautionary monitoring”, in which wemonitor not only the variables that we expect to changeas a result of the policy, but also a wide range of variables7
ARTIFICIAL INTELLIGENCE FOR SOCIAL GOODthat could allow us to detect unexpected side effectsand unmodeled phenomena. We must plan to iterativelyextend our models to incorporate these phenomena andre-optimize the policies.The Surgical Critical Care Initiative (SC2i), aDepartment of Defense funded research program, hasdeployed two clinical decision support tools (CDSTs) torealize the promise of precision medicine for criticalcare (Belard, 2016). The invasive fungal infectionCDST was deployed in 2014 to assist military providerswith treatment decisions both near point of injuryand at definitive treatment centers. Trauma-relatedinvasive fungal infections are well recognized for theirdevastating impacts on patients in both military andcivilian populations. In addition to substantial morbidityresulting from recurrent wound necrosis (e.g., greaternumber of surgical procedures, amputations, anddelayed wound closure), the disease is also associatedwith high mortality rates (Tribble and Rodriguez, 2014;Warkentien, 2012; Lewandowski, 2016; Rodriguez, 2014).Finally, when formulating and optimizing managementpolicies, we should adopt risk-sensitive methods.Standard practice in solving economic models is tominimize the expected costs and maximize the expectedbenefits of the policy. But if a policy has substantialdownside risk (e.g., species extinction, economiccatastrophe), then we should apply AI methods thatfind robust policies that control these downside risks.This is an active area of research (see, e.g., Chow, 2015),and much more work is needed to understand howwe can ensure that our models are robust to both theknown unknowns (as in traditional risk managementmethods) and the unknown unknowns.The massive-transfusion protocol (MTP) CDST iscurrently being assessed under a two-year clinical trialat Emory-Grady, one of the two SC2i civilian hospitals.This CDST uses evidence-based predictive analyticsto help physicians identify which patients genuinelyrequire a massive transfusion, thereby reducingcomplications associated with over-transfusion orthe needless expenditure of blood products (Maciel,2015; McDaniel, 2014; McDaniel, 2014; O’Keeffe, 2008;Dente, 2009). The SC2i is also planning a clinical trialaround its WounDX, a CDST that predicts the timing oftraumatic wound closure. Once validated, this tool hasthe potential to substantially improve outcomes (by asmuch as 68%) and reduce resource utilization ( 3.4Bannual cost-savings) both nationally and within theMilitary Health System (Forsberg, 2015).HealthSuccess StoriesCurrent methods for gathering population-scaledata about public health through surveys of medicalproviders or the public are expensive, time consuming,and biased towards patients who are already engagedin the medical system. Social media analytics isemerging as an alternative or complementary approachfor instantly measuring the nation’s health at largescale and with little or no cost. Natural languageprocessing can accurately identify social media poststhat are self-reports of disease systems, even for rareconditions. The nEmesis system, for example, helpshealth departments identify restaurants that are thesource of food-borne illness (Sadilek, 2016). nEmesisfinds all the Twitter posts for a city that are sent byrestaurant patrons, and then checking if any of thepatrons tweet about the symptoms of foodborne illnessover the next 72 hours. When this happens, healthdepartment officials are alerted of the fact, so that theycan schedule inspection of the restaurant. nEmesissignificantly improved the effectiveness of inspectionsin Las Vegas and the Center for Disease Control isfunding the expansion of nEmesis nationwide.Case Study: Making ‘Meaningful Use’meaningfulSepsis is the 11th leading cause of death in theUS – seven hundred fifty thousand patientsdevelop severe sepsis and septic shock in theUnited States each year. More than half of themare admitted to an intensive care unit (ICU),accounting for 10% of all ICU admissions, and 20%to 30% of hospital deaths. Yet others experiencesepsis due to hospital acquired infections8
(HAIs) in the medical units. Several studies havedemonstrated that morbidity, mortality, andlength of stay are decreased when severe sepsisand septic shock are identified and treated early;Kumar et al. 2006 show that every hour delay intreatment is associated with a 7-8% increase inmortality. Screening t
Artificial Intelligence (AI) is currently seeing major media interest, significant interest from federal agencies, and interest from society in general. From its origins in the 1950s, to early optimistic predictions of its founders, to some recent negative views put forth by the media, AI