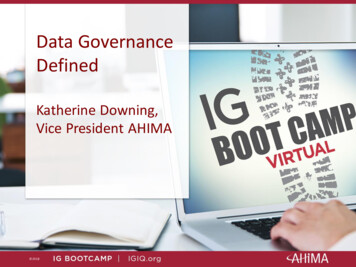
Transcription
Data GovernanceDefinedKatherine Downing,Vice President AHIMA 2018 2018
Objectives for this Session Define Data Governance Examine the urgent drivers for Data Governance Describe DG key functions, including master dataand metadata management Initiate planning for enterprise data governance 2018
Data Everywhere 2018More: Applications Medical Devices Consumer Devices Systems
What is Data? The dates, numbers, images,symbols, letters, and words thatrepresent basic facts andobservations about peopleprocesses, measurements, andconditions Raw facts without meaning orcontextAmerican Health Information Management Association. Pocket Glossary of Health InformationManagement and Technology. 5th Edition. AHIMA Press. 2017. 2018
Data Continues Expand 2018Dark DataNon-relational data storesBlockchainMachine Learning and Artificial IntelligenceData LakesAnd many others
Value of DataData in its raw form is of limited value. Itneeds to be processed in a meaningful wayto create information that would berelevant to a situation. 2018
Defining Information Governance 2018
AHIMA’sInformationGovernanceAdoption Model(IGAM ) 201810 competencies75 maturity markers1 IG programAll of healthcareIGAM is available through www.IGHealthRate.com
WHAT IS DATA GOVERNANCE (DG)?Data Governance (DG) provides for the design and execution ofdata needs planning and data quality assurance in concert withthe strategic information needs of the organization. DataGovernance includes data modeling, data mapping, data audit,data quality controls, data quality management, dataarchitecture, and data dictionaries. 2018
Why Data Governance?“If we lose, can’t find, can’t understand, or can’t integratevaluable enterprise data or if it is of questionable qualitythe maximum value of the data is not realized, leading to aloss of business insight or sub-optimal operations. At theend of the day, we govern data not for the sake of the data,but for the sake of the business!!”– Pete g/2012/06/12/data-governance-vs-data-management/ 2018
2018
DATA GOVERNANCEMaster Data QualityDataStandardsandDefinitionsDATA INPUTSINFORMATIONOUTPUTSINFORMATIONSTRATEGY 2018OPERATIONSCLINICAL CAREINDUSTRY DEMANDS
The Flood of BIGDATAIn the world of “Big Data,” the five Vs summarize the changing habits ofdata as it is exponentially increasing. The five Vs also emphasize thereasons why information governance is more important now than ever.The five Vs include: Volume, Velocity, Variety, Veracity and Value. 2018
Project Prioritization if Funding isAvailable 2018
What Data Are We ing-healthcare-data-governance 2018
What Are Some DG InitiativesKey Functional Components of DG Master Data ManagementMetadata ManagementData Quality ManagementData ArchitectureOther Key DG Initiatives 2018Data Cleansing (Scrubbing)Data TransformationData MappingData ClassificationData Definitions
Types of Master DataParty Data Individuals or organizations Includes employees, patients, providers, companies, vendors MPI Example – Manages master data related to patientsFinancialMaster DataProduct MasterDataLocationMaster Data Data about business units, cost centers, and accounts Used in accounts payable, payroll and materials management Cost Center Example – Includes cost center name, cost center code, description Describe an organization’s products Includes data about components making up a product Product Example – product and service identification codes, description, price,manufacturer information Describes data about the location of an organization’s customers, suppliers and others Includes postal codes, latitude, longitude, geopolitical boundaries, and sales territory Location Master Example – Critical in terms of public health and surveillance of diseaseand health hazardshttp://library.ahima.org/doc?oid 106378#.WX9A1IffN-Z 2018
Metadata Management 2018
Types of MetadataApplicationMetadataDocumentMetadataFile SystemMetadataEmbeddedMetadata Crea ted by the a pplication specific to the ESI being addressed, embedded i n the file, and movedwi th the file when copied. Copyi ng may a lter a pplication metadata. Exa mples i nclude patient a ccount number, patient last name, patient first name, date ofa dmission. Properti es about the file stored i n the file, as opposed to stored i n the document content. Often this data is not i mmediately vi ewable i n the software application used to create/edit thedocumentation, but ca n generally be seen i n “Properties” vi ew. Exa mples i nclude a uthor, company, creation/revision dates. Generated by the system to track the demographics (name, s ize, l ocation, usage, etc) of the ESItha t a re stored externally from, ra ther than embedded within, the ESI. Generally hidden, but an i ntegral part of ESI. Some metadata may be extra cted during processing and conversion for eDiscovery, embeddedda ta may not be. Ma y onl y be available in the original, native file. Exa mples i nclude “tra ck changes” or “comments” in Word document, or “notes” i n PowerPoint.http://library.ahima.org/doc?oid 106378#.WX9A1IffN-Z 2018
Data Quality Management 2018
Data Quality Terms Data Quality Management: The business processes thatensure the integrity of an organization's data duringcollection, application (including aggregation),warehousing, and analysis. Data Quality Measurement: A quality measure is amechanism to assign a quantity to quality of care bycomparison to a criterion. Quality measurementstypically focus on structures or processes of care thathave a demonstrated relationship to positive healthoutcomes and are under the control of the healthcaresystem.http://library.ahima.org/doc?oid 105607#.WYCESoffN-Y 2018
AHIMA’s Data Quality Management ModelApplication: The purpose for the datacollectionCollection: The processes by which dataelements are accumulatedWarehousing: Processes and systemsused to archive dataAnalysis: The process of translating datainto meaningful informationhttp://library.ahima.org/doc?oid 105607#.WYCESoffN-Y 2018
Data Architecture 2018
Other Key DG Initiatives 2018Data Cleansing (Scrubbing)Data TransformationData MappingData ModelingData ClassificationsData StandardsData Definitions
Data Cleansing (Scrubbing) The process of amending or removing data ina database; it’s incorrect, incomplete,improperly formatted, or duplicated. 2018
Data Cleansing (Scrubbing)Sources and Causes of Poor DataQuality5 Simple Data Scrubbing Stepsfor Your Team1. Older systems withobsolete data.2. Inaccurate data entry(good, old-fashionedhuman error).3. Too many databases andchannels to maintainproperly.4. Lack of coding standards.5. Simple errors like missingdata in database fields.1.2.3.4.5.Determine at least one area in yourbusiness with a data quality problem.Enlist the participation of topmanagement by demonstratingbusiness benefits due to data qualityimprovements.Review current data quality in the“target” area.Use data scrubbing software and otherdata scrubbing tools to produce asample report about the current dataquality in the target area.Within your various teams, find “DataQuality Champions” to lead the way byspreading awareness andcommunicating both big and smallachievements in improving data bbing/ 2018
Data Transformation The process of converting data or informationfrom one format to another, usually from theformat of a source system into therequirement of a new destination system. 2018
Data Mapping Data mapping involves "matching" betweena source and a target. Can be unidirectionalor bidirectional.A 2018B
Data Modeling The process of determining the users’information needs and identifyingrelationships among the data. 2018
IG Executive Training Video 2018
2018
Questions? 2018
AHIMA’s Information Governance Adoption Model (IGAM ) 10 competencies 75 maturity markers 1 IG program All of healthcare IGAM is available through www.IGHealthRate.com 2018 WHAT IS DATA GOVERNANCE (DG)? Data Governan