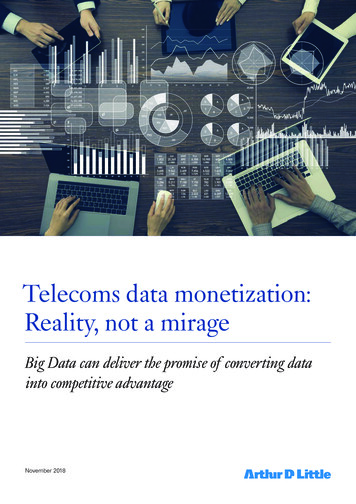
Transcription
Telecoms data monetization:Reality, not a mirageBig Data can deliver the promise of converting datainto competitive advantageNovember 2018
ContentExecutive summary31. Introduction42. Analytics value chain – Where are the value pools?53. Invest in big data technology as a capability to survive84. Choose the right operating model in your organization95. What is the way forward?10Authors:Karim TagaLokesh incipalTIMEDubaidadhich.lokesh@adlittle.comMichael OpitzJorge incipalTIMEMadridabril.jorge@adlittle.comArvind RajeswaranPranav omConsultantTIMEDubaiprince.pranav@adlittle.com* Telecommunications, Information, Media and Electronics Practice
Executive summaryGlobally, telcos consider data analytics a key strategic pillar, and continue to investin this direction. However, not many of them have reaped the benefits of theseinvestments, because they face multiple dilemmas around finding the right usecases, making the right technology choices from ever-growing options, andadopting the right operating and governance models to foster data-driven decisionmaking. Our experience of working with multiple telco operators, complementedby interviews with 10 Chief Data Analytics Officers from leading telcos acrossEMEA, reveals an addressable approach to these dilemmas. Telcos need to realizethat data analytics is a “must-have capability” in this digital age, and relatedinvestments will help them survive in the emerging competitive landscape. Theyneed to exploit analytics use cases across their core business functions, incustomer facing as well as other areas, such as supply chain, HR and operations.Telcos are uniquely positioned to capture external data monetization opportunities,yet these should be explored on a case-by-case basis, while maintaining a primaryfocus on internal monetization. Lastly, telco executives must lead efforts to driveorganization-wide change to foster data-driven decision-making. 3
1. IntroductionGlobally, telcos are facing increasing top-line pressure in theircore business due to intense competition from market players,as well as the growing threats from digital-native/OTT players.Telcos, thanks to their unique positioning in the ICT value chain,have access to arguably the deepest and richest insights intocustomers’ behaviors and usage. Leading operators have set upanalytics engines and capabilities to manage and process insightabout their customers, products, quality of service and internaloperations. Today, they are well positioned to enable digitalecosystems around emerging technologies such as artificialintelligence, Internet of Things and block chain. Telcos’ businessmodels in relation to all of these technologies must be enabledby real-time analytics capabilities so data can be processed inan efficient, secure and reliable manner, and with low latency.These ecosystems add new dimensions to the increasingvolume, speed and nature of data capture and advancedanalytics capabilities. Telcos that are well advanced in their datamonetization strategies have developed innovative externalpartnership models to leverage data for creating innovativeproducts and services in digital ecosystems.Telcos’ big data journey is not a recent trend; it began in early2000 with their attempts to develop business intelligence anddata warehousing capabilities. The journey before 2005 wasbased on technologies such as SQL, ETL and OLAP, and mostlylimited to the IT landscape. Since 2005, with the advent of “bigdata” technologies (such as Hadoop), the analytics ecosystemhas started to proliferate significantly with respect to technologymaturity and supply, data policies and regulations. It hasincreasingly attracted executive focus and attention.Several leading telcos have included “building big data andanalytics capabilities” as a key strategic pillar, and continueto invest in this direction. However, the benefits of theseinvestments have not been the same for all of them. We believeit is time for telcos to address the following complications whenharnessing value from their analytics investments.?Identifyingvaluevast numberof areuseconstantlycases areconstantlythedoeshorizon,is chain,the opportunityIdentifyingvaluepools:pools:A vast Anumberof use casesappearingon theappearinghorizon, butonwherevalue butlie inwherethe valueandcasesrepresentbestvaluerepresentcreation potential?the whichvalueusechain,andwhichtheusecasesthe best value creation potential?Makingtechnologydecisions:A ofplethorasolutionsandtechnologyoptionsin space.the dataandMakingtechnologydecisions:A plethorasolutionsofandtechnologyoptionsare emergingin thearedataemergingand analyticsWhatareanalyticsthekeythingsconsiderwhile making technologychoices, andwhat hasmaking?been the ROI?Whataretothekey considerationsfor oscapabilitiesto build dataanalyticsChoosingthetheoptimaloperatingmodel: Differentmodelarchitypesexist fortelcos to builddataforanalyticsin theirorganizations. What are the key considerations to underpin the analytics operating model design in specific contexts?Bringingmonetizationstrategyto action:toTelcosneedTelcosto figureneedout waysto executeanalyticsto gain datastrategicadvantage.How strategiccanBringingmonetizationstrategyaction:to figureoutdatawaysto executeanalyticsto gaintelcos ensure sustainable execution of their big data initiatives?inspace.capabilities in their organizations. What are the key considerations for operating model design in specific contexts?advantage. How can telcos ensure sustainable execution of their big data initiatives?41
2. Analytics value chain – Where are thevalue pools?Today, customers are expanding their digital footprints to moreand more applications and connected devices. The number ofsmartphone users has grown around 11 percent per annum inthe last few years, and is expected to reach 3 billion by the endof 2020. According to Ericsson, the data traffic per smartphonewill grow to 10 times its current volume by 2022. In Europealone, the data traffic per smartphone will be around 15 GB permonth by 2022.Rejuvenate the core business firstData has become more structured and data quality hasimproved significantly, leading to enriched customer databases.These can be used for plug-and-play solutions developed bythird parties. Additionally, non-customer related data frominternal business functions are presenting equally interestingopportunities to optimize telcos’ internal operations. In thisviewpoint, we examine the value and benefits available to telcosfrom both from internal and external monetization.Telcos with the most advanced data analytics programs havemaintained their focus on use cases in their core businessesand operations. For example, a challenger in Eastern Europehas employed a retention strategy using an advanced analyticsbased anti-churn model and next-best-recommendation engine.It has achieved 58 percent churn reduction in pay TV servicesand 17 percent in mobile services respectively. Anotherleading global telco is using customer data to enhance revenueassurance and fraud management. An incumbent in the MiddleEast combines mobile network probe data and customerinformation to analyze network quality of service and developnew insight into customer usage patterns. This insight is thenused for selective promotional targeting.Figure 1:Majority of telcos concur on internal/core business being themost tangible and valuable avenue to monetize their dataanalytics investments. There are numerous use cases ofenabling revenue uplift through customer life cycle managementand cost optimization through greater efficiency in networkplanning, sales, customer care, etc.Internal use casesFocus big data/advanced analytics building blockMarketing g &supportPersonalizedmarketingCustomeractivation andprovisioningAutomated tariffoptimizationAutomated ITsecurity threatdetectionPredictivemaintenance ofnetwork servicesHR processautomationReal-time salesprocess monitoringReal-time fieldforce schedulingReal-time productcreation andmanagementReal-time IT faultdetection andpredictionReal-time networkfault detection andpredictionField forceanalyticsReal-time mediaperformancetrackingBoosted failureanalysis forcomplaintsProduct renewalenhancementIT developmentquality assuranceEnd-to-end servicequality monitoringSmart procurementCustomerOnboarding andARPU growthCustomer journeyand satisfactionCredit scoring asserviceApplicationperformancemonitoringNetwork roll-outoptimizationInventoryoptimizationHeavy roamingabuse detectionAutomated naturalconversationProductconvergenceService esoptimizationOutdoor advertisingoptimizationContact centerproductivityRecommendationengine for externalclientsIPTC enueassurance andfraud managementSource: Arthur D. Little 5
The most prevalent use cases among telcos are usually incommercial, network and customer experience processes.A vast number of opportunities in support processes such asHR, procurement and logistics remain largely unexplored. Aleading European telco uses analytics techniques to manageprocurement activities in massive purchasing operations inorder to identify process gaps and deviations from standardprocedures. A leading operator in the United States uses a textanalytics and machine learning approach applied to customercomplaints data to achieve operational efficiency by optimizingthe trips of field engineers. An Asian operator responds to risingcustomer complaints by moving from a reactive to a proactiveapproach, in which it predicts complaints before customersregister them and takes actions to resolve root causes.Exploit new revenue streams on a case-by-case basisExternal data monetization is an advanced stage of a telco dataanalytics program and should be pursued on a case-by-casebasis only. External monetization business models range frommerely providing customized data as a service to developingcustom products and solutions for third parties. Footfall analyticsto track locations of customers and use them for marketingis one of the most popular monetization example for telcos.In many cases, this has enabled operators to diversify in theadvertising value chain, either inorganically through acquiringsuch platforms, or by developing in-house, location-basedanalytics capabilities.Figure 2:Selected examples of telcos playing in the location-basedtargeting value chainLocation-based targeting value ocation-basedadvertisingapp developersTelecomoperatorsAll telecomoperatorsConsumersEarlier, telecomoperators onlyacted as channelsto distribute theadvertising messageNow, a few telcoshave done backwardintegration by eitherM&A or developing inhouse LBA applicationsOperators such asSingtel and Verizon haveexpanded nearly to theend of the value chain togain the capabilities ofad agenciesSource: Arthur D. LittleSome interesting examples of external data monetization are asfollows:Credit scoring as a service: An Eastern European operatorleverages internal data to provide “credit-scoring-as-a-service” toexternal clients. This credit scoring is significantly more effectivethan a traditional banking model, reducing credit risk by 40percent as claimed by the operator.Market Locator: DingoDot has developed an application –“Market Locator” – that enables diverse companies to gainaccess to powerful analysis based on real hard data. SeveralEuropean telcos have been using Market Locator to createnew revenue streams by providing anonymized and aggregatedbig data to their B2B customers in the form of a self-servicepopulation analytics and mobile marketing solution.Digital advertising business: A leading US-based operatoruses web-browsing history, app usage and location data topersonalize rewards and deliver targeted advertising from thirdparties.Telecom players, that have taken proactive steps towardsexternal monetization of data, have primarily done so throughpartnership models across a variety of ecosystems in B2B andB2C segments. In some cases operators have also acquiredstart-ups for vertical integration across the value chain. Telefonicahas engaged in multiple partnerships with leading brands suchas Linio, Heineken, and United Colors of Benetton. In 2017, itacquired leading UK geolocation data start-up Statiq. Statiqprocesses billions of location data signals to identify the placespeople visit and build consumer profiles. This allows advertisersto better target ads based on a user’s physical location, and trackwhether the user visited a retail store after seeing a mobile ad.External data monetization efforts also accentuate the risk oflosing customers’ trust because of involuntary privacy breaches.Data privacy is a growing concern for regulators and nationalsecurity organizations, and efforts to develop robust regulatoryframeworks for data privacy are under way in several countriesand regions. The European Union has introduced General DataProtection Regulation (GDPR), which gives users the right toprotect their own personal data. This forces companies to bemore attentive to how they handle customer data and givesusers greater control over their data, including the ability toexport it, withdraw consent or request access to it. For thisreason, telcos should expedite advocacy efforts to shape theregulatory landscape and enable viable business models for themutual benefit of customers and operators.We recommend that external data monetization models arethoroughly assessed before resources are dedicated to externalmonetization efforts. In specific cases of attractive opportunities,telcos should develop partnership models by bringing players inthe ecosystem together – customers, users of the data (e.g.,B2B clients, start-ups) and intermediate parties (e.g., externalanalytics firms).36
However, despite these developments, there remains generalscepticism about external data monetization. Although externalmonetization opportunities exist, telcos struggle with whereand how to start, and as a result find it hard to realize concretebenefits. Most external monetization use cases are local andrequire well-developed partner ecosystems. Furthermore,external monetization opportunities appear in a range of industryverticals (e.g., mobility, financial services), and telco players facethe challenge of finding the appropriate entry points in specificvalue chains with data analytics offerings.Figure 3:Data external monetization use casesExternal data monetization – selective use casesRetail site planningRetailPersonalized marketingCommon billingReal-time, location-based targetingATM/branch location sportationProduct cross-sell/upsellCredit scoringFraudulent transaction detectionSociodemographicsanalysisBillboard placementPersonalized content designReal-time, location-based targetingTraffic planningNational security surveillanceGovernment services provisioningRoute planning and analysisSmart-city planningProfile-based dynamic pricingReal-time, traffic-based routingSource: Arthur D. LittleIncreasing complexity level 7
3. Invest in big data technology as acapability to surviveThe analytics space has become extremely cluttered, witha plethora of solutions available across layers (infrastructure,platforms, analytics, visualization, etc.). As a result, technologydecision-making is affected by multiple dilemmas, as discussedbelow. A common factor underlying most of these dilemmas isthat the ROI for telcos’ big data investments remains unprovenon an incremental basis.Cloud based versus own-facility based: In a cloud environment,full-stack implementation provides important operational andeconomic advantages. Based on our estimates of progressivecloud adoption for the six big data infrastructure categories forrelevant players, telcos can reduce the total cost of ownershipby 28 percent in moving from on premise set up to Platformas a Service (PaaS) models. However, strict data privacy lawsfor telecoms operators inhibit the adoption of full public cloud.Therefore, local on-premises servers, private cloud and publiccloud (with data centre inside the regional permits) are the mostlikely options. These arrangements often reduce requirementsfor complex analytics models that need a lot of computingpower. In such scenarios, these complex models can be trainedin public cloud with data anonymization, saving on the costsof procuring expensive servers. Model scoring can be done onlocal servers later, during the production stage.Figure 4:Cloud based vs on premise TCO comparisonTotal cost of ownership(Average for 5 years of transition, indexed to on-premise cost)-15%-27%1.000.230.110.860.180.72SW O&M0.050.14On-premise SW/PaaS licenses0.370.210.150.110.220.010.14On-premise IaaS model PaaS model/Full stackSource: Arthur D. Little8Hardware inc. O&MCompute0.66StorageHW O&M OS licensesFull stack versus incremental modules: The choice betweenimplementing a full stack versus incremental modules dependson the maturity of the big data program in the organization.Most telcos implement technologies as needed, and use amix of in-house and external technologies. The needs-drivenimplementation allows telcos to optimize the investmentsrequired, as most have yet to fully realize the benefits of bigdata. With technology evolution, this approach allows telcosto adopt best-of-breed solutions when they are available.However, it creates the challenge of integrating new tools withlegacy technical architecture. In a cloud environment, full stackimplementation provides important operational and economicadvantages. Such a model enables managed services andminimizes installation and maintenance efforts.Open source versus license: Open-source technology is amajor trend and preference for telcos, especially for advancedanalytical algorithms. A range of open-source libraries exist for R,Python, TensorFlow, etc., supported by passionate communitiesof developers, and which telcos can leverage without majorinvestment. For robust and standard analytics applications,traditional licensed tools such as SAS/SPSS are widely used.Business case – Investment or return: The positive convictionabout expected benefits (in core business areas) is themain driver of big data investments. The return on big datainvestments is not easy to ascertain, as the technology isused for a variety of purposes, such as reporting, dashboardvisualization, and data-quality improvements. Contrary totelecom operators, leading digital players de-prioritize big datainvestment monetization concerns, and instead start with aphilosophy of considering these capabilities as must-have tosurvive in the digital era, without over-emphasizing the traditionalcost/benefit equations.
4. Choose the right operating model inyour organizationWhile monetization opportunities are vast and technologicaldilemmas are easy to resolve, adopting a suitable operatingmodel in the specific context of the telco remains a criticalsuccess factor in the big data analytics journey. A range ofoptions exist for operating models in telcos, and we finddifferent models implemented, depending on their roles in thevalue chain, organization capabilities and progress towards bigdata analytics.Figure 5:Organization model for analytics unitAnalytics organization models in telcosDistributed modelCentralized model Pros: Greaterchange momentumwith teams closer tothe business Pros: Clearaccountability,resource andinvestment efficiency,standardized practices Risks: Difficult torealize a commonvision and pace,poor cross-functionalview Risks: Maximum Risks: Perception thatorganizational resistancethe unit lacks practicalwith less transparency inexpertise in functionalresource allocationareas and staff are onlyacross functionsalgorithm designers When: Limited needfor central steering,mainly autonomousBUs When: Gettingstarted, need forcentral steering andoperationalresponsibilityCenter ofexcellenceHybrid model?Source: Arthur D. Little Pros: Avoids duplicationof skilled and expensiveresources acrossorganization forspecialized tasks When: End stage,analytics ismainstream andeverywhereAnalytics unitsCentralized model: In the centralized model, a central dataand business analytics function within the organization takesownership of big data analytics and acts as a service provider tothe entire organization for data, insight and advanced analyticalofferings. The model facilitates centralized enterprise datamanagement and inhibits silo thinking. This model is conducivefor external monetization, as a single unit has a 360-degreeview of the services that can be offered. A disadvantage of thismodel is that the central function may not have the subjectmatter expertise required to exploit use cases across functions.An operator in the Middle East has implemented a centralizedmodel for analytics that plays an active role in serving internalrequirements, as well as products and services for customers.Distributed model: In the distributed model, big dataanalytical activities are distributed across different units in theorganization. For example, each customer-facing business unit,technology unit, etc., performs its own analytics. This model isrelevant when analytics-driven reporting requires subject-matterexpertise.Hybrid model: Many telcos start off with the centralized modeland then move to distributed model. In the hybrid model, oncebig data analytics capabilities are established after the initial twoto three years, big data lakes, data engineers and data stewardscontinue to be in the central function for enterprise-wideinitiatives. Additionally, each function has a team so the usecases remain close to the business.Large telecom groups with multi-geography operations aimto have centralized big data functions to serve all operatingcompanies (opcos). The model aims to leverage the learningsand capabilities (use cases, architecture, etc.) across all opcosin the group. However, a key concern with this model is thatthe regulations in individual opco markets restrict them fromexchanging customer data across geographic borders. Therefore,participation of opcos in this model may be limited. Telcos arealso imposing stronger governance policies on how data iscaptured and data-driven decisions are taken. According to anestimate by CrowdFlower (now Figure Eight), data scientistsspend around 80 percent of their time in collecting, cleaning,and organizing data, which calls for better data managementpolicies.Increasingly, data governance frameworks are the key themesfor C-level executives, who need to push for them from thetop. As big data analytics is becoming mainstream, telcosare upgrading their data policies and underlying data-relatedprocesses to ascertain data capture/updates according to theinitial data models, in order to ensure there is a single sourceof the truth, and to safeguard data privacy issues, etc. Anotherequally important dimension is building a fact-based decisionmaking culture in the organization, as was emphasized byseveral telcos during our interviews.Figure 6:Data Science Report 2016 by CrowdFlowerTime spent by data scientists4%65%3%Cleaning and organizing data9%Collecting datasetsMining data for patternRefining algorithms19%60%Building training setsOthersSource: Arthur D. Little 9
5. What is the way forward?Telcos have successfully begun leveraging their data as anasset already, as is evident from the hundreds of different usecases that we have seen. However, we expect a second waveof the data analytics revolution for telcos to commence due tonew trends around digitalization, as well as new technologies inrelation to 5G and the IoT that will drive a fundamental shift intheir service offerings and delivery models.Get started if you haven’t already done!1For some telcos that are still trying to separate mirage from reality, it is not too late to start leveraging their data as an asset. Theyshould aim to build upon small successes and then roll out big data initiatives organization-wide. In our previous viewpoint, "Bigdata: A gold mine in telcos’ backyard", in this same series, we recommend seven key steps to bring big data plans into reality.Drive organization-wide change to foster data-driven decision-making2In our view, change management for adoption of analytics-related business processes is more important than development ofanalytics techniques and related use cases. Telco executives must increasingly take data-driven decisions, as analytics withoutbusiness actions lead to failed investments. Internal analytics teams need support from the entire organization on management ofdata, adherence to data policies, and implementation of analytics solutions.Focus on developing innovative use cases in the core business3Internal data monetization presents maximum business impact. The latest developments in machine learning (deep learning)technologies and cloud-based analytical solutions have opened up several new use cases for telcos. Machine learning is the nextstage of big data investment monetization for telcos, and getting big data implementations right is key to build machine learningapplications. (Refer to our viewpoint, "Why machine learning is crucial to effective utilization of big data"). In addition, with limitedresources, analytics managers should strive to automate standard solutions in order to embed analytics into high-speed businessprocesses and utilize those resources to work on new projects/use cases. Lastly, telcos should also start exploring application ofanalytics to opportunities in functional domains such as HR, procurement, finance etc.Stimulate external data monetization on a case-by-case basis4Telcos should go after external monetization opportunities based on individual use cases. For selected high-potential use cases,telcos need to take the leadership role in developing partnerships with B2B businesses, start-ups, and technology vendors acrossthe value chain.Secure an executive push510Company-wide embracing of analytics drives changes in culture, processes, behavior, and skills for many employees. Telcosneed strong sponsors of analytics at very senior levels with strong understanding of the ecosystem and a passion for fact-baseddecisions. This is important for not only securing buy-in during investment decisions, but also enforcement of the data-drivenculture within the organization.
ContactsIf you would like more information or to arrange an informal discussion on the issues raised here andhow they affect your business, please contact:AustriaKarim Tagataga.karim@adlittle.comJapanShinichi Akayamaakayama.shinichi@adlittle.comSpainJesus Portalportal.jesus@adlittle.comBelgiumGregory Pankertpankert.gregory@adlittle.comKoreaHoonjin Hwanghwang.hoojin@adlittle.comSwedenAgron Laskulasku.agron@adlittle.comChinaRussell Pellpell.russell@adlittle.comLatin AmericaGuillem emens Schwaigerschwaiger.clemens@adlittle.comCzech RepublicDean Brabecbrabec.dean@adlittle.comMiddle EastSander Kochkoch.sander@adlittle.comTurkeyCoskun Babanbaban.coskun@adlittle.comFrancePaul Desjonqueresdesjonqueres.paul@adlittle.comThe NetherlandsMartijn han Rowanrowan.jonathan@adlittle.comGermanyMichael Opitzopitz.michael@adlittle.comNorwayLars Thurmann-Moethurmann-moe.lars@adlittle.comUSASean McDevittmcdevitt.sean@adlittle.comIndiaSrini nder rlo Agrestiagresti.giancarlo@adlittle.comSingaporeYuma Itoito.yuma@adlittle.com
Telecoms data monetization:Reality, not a mirageBig Data can deliver the reality of converting datainto competitive advantageArthur D. LittleArthur D. Little has been at the forefront of innovation since1886. We are an acknowledged thought leader in linkingstrategy, innovation and transformation in technology-intensiveand converging industries. We navigate our clients throughchanging business ecosystems to uncover new growthopportunities. We enable our clients to build innovationcapabilities and transform their organizations.Our consultants have strong practical industry experiencecombined with excellent knowledge of key trends anddynamics. ADL is present in the most important businesscenters around the world. We are proud to serve most of theFortune 1000 companies, in addition to other leading firms andpublic sector organizations.For further information please visit www.adlittle.com orwww.adl.com.Copyright Arthur D. Little Luxembourg S.A. 2018.All rights reserved.www.adl.com/BigData
customer complaints by moving from a reactive to a proactive approach, in which it predicts complaints before customers register them and takes actions to resolve root causes. Exploit new revenue streams on a case-by-case basis External data monetization is an advanced stage of a telco data analytics program and should be pursued on a case-by-case