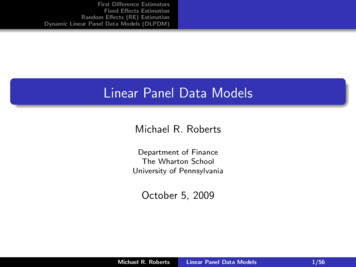
Transcription
First Difference EstimatorsFixed Effects EstimationRandom Effects (RE) EstimationDynamic Linear Panel Data Models (DLPDM)Linear Panel Data ModelsMichael R. RobertsDepartment of FinanceThe Wharton SchoolUniversity of PennsylvaniaOctober 5, 2009Michael R. RobertsLinear Panel Data Models1/56
First Difference EstimatorsFixed Effects EstimationRandom Effects (RE) EstimationDynamic Linear Panel Data Models (DLPDM)Two Period ModelPolicy AnalysisThree Period PanelGeneral Period PanelExampleLink between crime and unemployment. Data for 46 cities in 1982and 1987.Consider CS regression using 1987 data\crimeRate 128.38 4.16unem, R 2 0.033(20.76)(3.42)Higher unemployment decreases the crime rate (insignificantly)?!?!?!Problem omitted variablesSolution add more variables (age distribution, gender distribution,education levels, law enforcement, etc.)Use like lagged crime rate to control for unobservablesMichael R. RobertsLinear Panel Data Models2/56
First Difference EstimatorsFixed Effects EstimationRandom Effects (RE) EstimationDynamic Linear Panel Data Models (DLPDM)Two Period ModelPolicy AnalysisThree Period PanelGeneral Period PanelPanel Data ApproachPanel data approach to unobserved factors. 2 types:12constant across timevary across timeyit β0 δ0 d2t β1 xit ai uit , t 1, 2where d2 1 when t 2 and 0 when t 1Intercept for period 1 is β0 , for period 2 β0 δ0Allowing intercept to change over time is important to capturesecular trends.ai captures all variables that are constant over time but differentacross cross-sectional units. (a.k.a. unobserved effect,unobserved heterogeneity)uit is idiosyncratic error or time-varying error and representsunobserved factors that change over time and effect yitMichael R. RobertsLinear Panel Data Models3/56
First Difference EstimatorsFixed Effects EstimationRandom Effects (RE) EstimationDynamic Linear Panel Data Models (DLPDM)Two Period ModelPolicy AnalysisThree Period PanelGeneral Period PanelExample (Cont)Panel approach to link between crime and unemployment.crimeRateit β0 δ0 d78t β1 unemit ai uitwhere d87 1 if year is 1987, 0 otherwise, and ai is an unobservedcity effect that doesn’t change over time or are roughly constantover the 5-year window.Examples:123Geographical features of cityDemographics (race, age, education)Crime reporting methodsMichael R. RobertsLinear Panel Data Models4/56
First Difference EstimatorsFixed Effects EstimationRandom Effects (RE) EstimationDynamic Linear Panel Data Models (DLPDM)Two Period ModelPolicy AnalysisThree Period PanelGeneral Period PanelPooled OLS EstimationHow do we estimate β1 on the variable of interest?Pooled OLS. Ignore ai . But we have to assume that ai is to unemsince it would fall in the error term.crimeRateit β0 δ0 d78t β1 unemit vitwhere vit ai uit . SRF:\crimeRate 93.42 7.94d87 0.427unem, R 2 0.012(12.74)(7.98)(1.188)Positive coef on unem but insignificantMichael R. RobertsLinear Panel Data Models5/56
First Difference EstimatorsFixed Effects EstimationRandom Effects (RE) EstimationDynamic Linear Panel Data Models (DLPDM)Two Period ModelPolicy AnalysisThree Period PanelGeneral Period PanelFirst Difference EstimationDifference the regression equation across time to get rid of fixedeffect and estimate differenced equation via OLS.yi2 (β0 δ0 ) β1 xi2 ai ui2 , (t 2)yi1 β0 β1 xi1 ai ui1 , (t 1)Differencing yields yi δ0 β1 xi uiwhere denotes period 2 minus period 1.Key assumption: xi ui , which holds if at each time t,uit xit t. (i.e., strict exogeneity).This rules out lagged dependent variables.Key assumption: xi must vary across some iMichael R. RobertsLinear Panel Data Models6/56
First Difference EstimatorsFixed Effects EstimationRandom Effects (RE) EstimationDynamic Linear Panel Data Models (DLPDM)Two Period ModelPolicy AnalysisThree Period PanelGeneral Period PanelFirst Difference ExampleReconsider crime example:\crimeRate 15.40 2.22 unem, R 2 0.012(4.70)(0.88)Now positive and significant effect of unemployment on crimeIntercept crime expected to increase even if unemploymentdoesn’t change!This reflects secular increase in crime rate from 1982 to 1987Michael R. RobertsLinear Panel Data Models7/56
First Difference EstimatorsFixed Effects EstimationRandom Effects (RE) EstimationDynamic Linear Panel Data Models (DLPDM)Two Period ModelPolicy AnalysisThree Period PanelGeneral Period PanelPractical IssuesDifferencing can really reduce variation in xx may vary greatly in cross-section but x may notLess variation in explanatory variable means larger standard errorson corresponding coefficientCan combat by either12Increasing size of cross-section (if possible)Taking longer differences (over several periods as opposed to adjacentperiods)Michael R. RobertsLinear Panel Data Models8/56
First Difference EstimatorsFixed Effects EstimationRandom Effects (RE) EstimationDynamic Linear Panel Data Models (DLPDM)Two Period ModelPolicy AnalysisThree Period PanelGeneral Period PanelExampleMichigan job training program on worker productivity ofmanufacturing firms in 1987 and 1988scrapi t β0 δ0 y 88t β1 granti t ai ui twhere i, t index firm-year, scrap scrap rate # of items per 100that must be tossed due to defects, grant 1 if firm i in year treceived job training grant.ai is firm fixed effect and captures average employee ability, capital,and managerial skill.things constant over 2-year period.Difference to zap ai and run 1st difference (FD) regression scrap[ 0.564 0.739 grant, N 54, R 2 0.022(0.405)(0.683)Job training grant lowered scrap rate but insignificantlyMichael R. RobertsLinear Panel Data Models9/56
First Difference EstimatorsFixed Effects EstimationRandom Effects (RE) EstimationDynamic Linear Panel Data Models (DLPDM)Two Period ModelPolicy AnalysisThree Period PanelGeneral Period PanelExample (Cont)Is level-level model correct? log\(scrap) 0.57 0.317 grant, N 54, R 2 0.067(0.097)(0.164)Job training grant lowered scrap rate by 31.7% (or 27.2% exp(-0.317) - 1).Pooled OLS estimate implies insignificant 5.7% reductionLarge difference between pooled OLS and first difference suggeststhat firms with lower-ability workers (low ai ) are more likely toreceive a grant.I.e., Cov (ai , grantit ) 0. Pooled OLS ignores ai and we get adownward omitted variables biasMichael R. RobertsLinear Panel Data Models10/56
First Difference EstimatorsFixed Effects EstimationRandom Effects (RE) EstimationDynamic Linear Panel Data Models (DLPDM)Two Period ModelPolicy AnalysisThree Period PanelGeneral Period PanelProgram Evaluation ProblemLet y outcome variable, prog program participation dummy.yit β0 δ0 d2t β1 progit ai uitDifference regression yit δ0 β1 progit uitIf program participation only occurs in the 2nd period then OLSestimator of β1 in the differenced equation is just:βˆ1 ytreat ycontrol(1)Intuition:123 progit progi2 since participation in 2nd period only. (i.e., progitis just an indicator identify the treatment group)Omitted group is non-participants.So β1 measures the average outcome for the participants relative tothe average outcome of the nonparticipantsMichael R. RobertsLinear Panel Data Models11/56
First Difference EstimatorsFixed Effects EstimationRandom Effects (RE) EstimationDynamic Linear Panel Data Models (DLPDM)Two Period ModelPolicy AnalysisThree Period PanelGeneral Period PanelProgram Evaluation Problem (Cont)Note: This is just a difference-in-differences (dif-in-dif) estimator“Equivalent” model:yit β0 δ0 d2t β1 progit β2 d2t progit ai uitwhere β2 has same interpretation as β1 from above.If program participation can take place in both periods, we can’twrite the estimator as in (1) but it has the same interpretation:change in average value of y due to program participationAdding additional time-varying controls poses no problem. Justdifference them as well. This allows us to control for variables thatmight be correlated with program designation.yit β0 δ0 d2t β1 progit γ 0 Xit ai uitMichael R. RobertsLinear Panel Data Models12/56
First Difference EstimatorsFixed Effects EstimationRandom Effects (RE) EstimationDynamic Linear Panel Data Models (DLPDM)Two Period ModelPolicy AnalysisThree Period PanelGeneral Period PanelSetupN individuals, T 3 time periods per individualyit δ1 δ2 d2t δ3 d3t β1 xit1 . βk xitk ai uitGood idea to allow different intercept for each time period(assuming we have small T )Base period, t 1, t 2 intercept δ1 δ2 , etc.If ai correlated with any explanatory variables, OLS yields biasedand inconsistent estimates. We needCov (xitj , uis ) 0 t, s, j . βk xitk ai uit(2)(i.e., strict exogeneity after taking out ai )Assumption (2) rules out cases where future explanatory variablesreact to current changes in idiosyncratic errors (i.e., laggeddependent variables)Michael R. RobertsLinear Panel Data Models13/56
First Difference EstimatorsFixed Effects EstimationRandom Effects (RE) EstimationDynamic Linear Panel Data Models (DLPDM)Two Period ModelPolicy AnalysisThree Period PanelGeneral Period PanelEstimationIf ai is correlated with xitj then xitj will be correlated with compositeerror ai uitEliminate ai via differencing yit δ2 d2t δ3 d3t β1 xit1 . βk xitk uitfor t 2, 3Key assumptions is that Cov ( xitj , uit ) 0 j and t 2, 3.Note no intercept and time dummies have different meaning:t 2 d2t 1, d3t 0t 3 d2t 1, d3t 1Unless time dummies have a specific meaning, better to estimate yit α0 α3 d3t β1 xit1 . βk xitk uitfor t 2, 3 to help with R 2 interpretationMichael R. RobertsLinear Panel Data Models14/56
First Difference EstimatorsFixed Effects EstimationRandom Effects (RE) EstimationDynamic Linear Panel Data Models (DLPDM)Two Period ModelPolicy AnalysisThree Period PanelGeneral Period PanelSetupN individuals, T time periods per individualyit δ1 δ2 d2t δ3 d3t . δT dTt . β1 xit1 . β2 xitk ai uitDifferencing yields estimation equation yit α0 α3 d3t . αT dTt β1 xit1 . βk xitk uitfor t 1, ., T 1Michael R. RobertsLinear Panel Data Models15/56
First Difference EstimatorsFixed Effects EstimationRandom Effects (RE) EstimationDynamic Linear Panel Data Models (DLPDM)Two Period ModelPolicy AnalysisThree Period PanelGeneral Period PanelStandard ErrorsWith more than 2-periods, we must assume uit is uncorrelatedover time for the usual SEs and test statistics to be validIf uit is uncorrelated over time & constant Var, then uit iscorrelated over timeCov ( ui2 , ui3 ) Cov (ui2 ui1 , ui3 ui2 ) σu2i2Corr ( ui2 , ui3 ) 0.5If uit is stable AR(1), then uit is serially correlatedIf uit is random walk, then uit is serially uncorrelatedMichael R. RobertsLinear Panel Data Models16/56
First Difference EstimatorsFixed Effects EstimationRandom Effects (RE) EstimationDynamic Linear Panel Data Models (DLPDM)Two Period ModelPolicy AnalysisThree Period PanelGeneral Period PanelTesting for Serial CorrelationTest for serial correlation in the FD equation.Let rit uitIf rit follows AR(1) modelrit ρri,t 1 eitwe can test H0 : ρ 0 by1234Estimate FD model via pooled OLS and get residualsRun pooled OLS regression of r̂it on r̂i,t 1ρ̂ is consistent estimator of ρ so just test null on this estimate(Note we lose an additional time period because of lagged difference.)Depending on outcome, we can easily correct for serial correlation inerror terms.Michael R. RobertsLinear Panel Data Models17/56
First Difference EstimatorsFixed Effects EstimationRandom Effects (RE) EstimationDynamic Linear Panel Data Models (DLPDM)Two Period ModelPolicy AnalysisThree Period PanelGeneral Period PanelChow TestNull: Do the slopes vary over time?Can answer this question by interacting slopes with period dummies.The run a Chow test as before.Michael R. RobertsLinear Panel Data Models18/56
First Difference EstimatorsFixed Effects EstimationRandom Effects (RE) EstimationDynamic Linear Panel Data Models (DLPDM)Two Period ModelPolicy AnalysisThree Period PanelGeneral Period PanelChow TestCan’t estimate slopes on variables that don’t change over time —they’re differenced away.Can test whether partial effects of time-constant variables changeover time.E.g., observe 3 years of wage and wage-related datalog (wageit ) β0 δ1 d2t δ2 d3t β1 femalei γ1 d2t femalei γ2 d3t femalei λXit ai uitFirst differenced equation log (wageit ) δ1 d2t δ2 d3t γ1 ( d2t ) femalei γ2 ( d3t ) femalei λ Xit uitThis means we can estimate how the wage gap has changed overtimeMichael R. RobertsLinear Panel Data Models19/56
First Difference EstimatorsFixed Effects EstimationRandom Effects (RE) EstimationDynamic Linear Panel Data Models (DLPDM)Two Period ModelPolicy AnalysisThree Period PanelGeneral Period PanelDrawbacksFirst differencing isn’t a panacea. Potential issues12If level doesn’t vary much over time, hard to identify coef indifferenced equation.FD estimators subject to severe bias when strict exogeneityassumption fails.13Having more time periods does not reduce inconsistency of FDestimator when regressors are not strictly exogenous (e.g., includinglagged dep var)FD estimator can be worse than pooled OLS if 1 or more ofexplanatory variables is subject to measurement error12Differencing a poorly measured regressor reduces its variation relativeto its correlation with the differenced error caused by CEV.This results in potentially sizable biasMichael R. RobertsLinear Panel Data Models20/56
First Difference EstimatorsFixed Effects EstimationRandom Effects (RE) EstimationDynamic Linear Panel Data Models (DLPDM)IntroductionFE IdentificationFE MiscellaneousFixed Effects TransformationConsider a univariate modelyit β1 xit ai uit , t 1, 2, ., TFor each unit i, compute time-series mean.ȳi β1 x̄i ai ūi , whereȳi (1/T )ΣyitSubtract the averaged equation from the original model(yit ȳi ) β1 (xit x̄i ) (uit ūi ), t 1, 2, ., Tÿit β1 ẍit üit , t 1, 2, ., Tz̈ represents time-demeaned dataFixed Effect Transformation Within TransformationMichael R. RobertsLinear Panel Data Models21/56
First Difference EstimatorsFixed Effects EstimationRandom Effects (RE) EstimationDynamic Linear Panel Data Models (DLPDM)IntroductionFE IdentificationFE MiscellaneousFixed Effects EstimatorWe can estimate the transformed model using pooled OLS since ithas eliminated the unobserved fixed effect ai just like 1st differencingThis is called fixed effect estimator or within estimator“within” comes from OLS using the time variation in y and x withineach cross-sectional unitConsider general modelyit β1 xit1 . βk xitk ai uit , t 1, 2, ., TSame idea. Estimate time-demeaned model using pooled OLSÿit β1 ẍit . βk ẍitk üit , t 1, 2, ., TMichael R. RobertsLinear Panel Data Models22/56
First Difference EstimatorsFixed Effects EstimationRandom Effects (RE) EstimationDynamic Linear Panel Data Models (DLPDM)IntroductionFE IdentificationFE MiscellaneousFixed Effects Estimator AssumptionsWe need strict exogeneity on the explanatory vars to get unbiasedI.e., uit is uncorrelated with each x across all periods.Fixed effect (FE) estimation, like FD, allows for arbitrary correlationbetween ai and x in any time periodFE estimation, like FD, precludes estimation of time-invarianteffects that get killed by FE transformation. (e.g., gender)We need uit to be homoskedastic and serially uncorrelated for validOLS analysis.Degrees of Freedom is not NT k, where k # of xs.123Degrees of Freedom NT N k, since we lose one df for eachcross-sectional obs from the time-demeaning.For each i, demeaned errors add up to 0 when summed across t 1 less df.This is like imposing a constraint for each cross-sectional unit.(There’s no constraint on the original idiosyncratic errors.)Michael R. RobertsLinear Panel Data Models23/56
First Difference EstimatorsFixed Effects EstimationRandom Effects (RE) EstimationDynamic Linear Panel Data Models (DLPDM)IntroductionFE IdentificationFE MiscellaneousFE Implicit ConstraintsWe can’t include time-constant variables.1Can interact them with time-varying variables to see how their effectvaries over time.Including full set of time dummies (except one) can’t estimateeffect of variables whose change across time is constant.123E.g., years of experience will change by one for each person in eachyear. ai accounts for average differences across people or differencesacross people in their experience in the initial time period.Conditional on ai , the effect of a one-year increase in experiencecannot be distinguished from the aggregate time effects becauseexperience increases by the same amount for everyone!A linear time trend instead of year dummies would create a similarproblem for experienceMichael R. RobertsLinear Panel Data Models24/56
First Difference EstimatorsFixed Effects EstimationRandom Effects (RE) EstimationDynamic Linear Panel Data Models (DLPDM)IntroductionFE IdentificationFE MiscellaneousE.g., FE Implicit ConstraintsConsider an annual panel of 500 firms from 1990 to 2000Include full set of year indicators can’t include12firm agemacroeconomic variablesThese are all collinear with the year indicators and intercept.Michael R. RobertsLinear Panel Data Models25/56
First Difference EstimatorsFixed Effects EstimationRandom Effects (RE) EstimationDynamic Linear Panel Data Models (DLPDM)IntroductionFE IdentificationFE MiscellaneousDummy Variable RegressionWe could treat ai as parameters to be estimated, like intercept.Just create a dummy for each unit i.This is called Dummy Variable RegressionThis approach gives us estimates and standard errors that areidentical to the within firm estimates.R 2 will be very high.lots of parameters.âi may be of interest. Can compute from within estimates as:aˆi ȳi β̂1 x̄i1 . β̂k x̄ik , i 1, ., Nwhere x̄ is time-averageâi are unbiased but inconsistent (Incidental Parameter Problem).Note: reported intercept estimate in FE estimation is just average ofindividual specific intercepts.Michael R. RobertsLinear Panel Data Models26/56
First Difference EstimatorsFixed Effects EstimationRandom Effects (RE) EstimationDynamic Linear Panel Data Models (DLPDM)IntroductionFE IdentificationFE MiscellaneousFE or FD?With T 2, doesn’t matter. They’re identicalWith T 32, FE6 FDBoth are unbiased under similar assumptionsBoth are consistent under similar assumptionsChoice hinges on relative efficiency of the estimators (for large Nand small T ), which is determined by serial correlation in theidiosyncratic errors, uit123Serially uncorrelated uit FE more efficient than FD andstandard errors from FE are valid.Random walk uit FD is better because transformed errors areserially uncorrelated.In between.efficiency differences not clear.When T is large and N is not too large, FE could be badBottom line: Try both and understand differences, if any.Michael R. RobertsLinear Panel Data Models27/56
First Difference EstimatorsFixed Effects EstimationRandom Effects (RE) EstimationDynamic Linear Panel Data Models (DLPDM)IntroductionFE IdentificationFE MiscellaneousFE with Unbalanced PanelsUnbalance Panel refers to panel data where units have differentnumber of time series obs (e.g., missing data)Key question: Why is panel unbalanced?If reason for missing data is uncorrelated with uit , no problem.If reason for missing data is correlated with uit , problem. Thisimplies nonrandom sample. E.g.,123456Sample firms and follow over time to study investmentSome firms leave sample because of bankruptcy, acquisition, LBO,etc. (attrition)Are these exit mechanisms likely correlated with unmeasuredinvestment determinants (uit )? Probably.If so, then resulting sample selection causes biased estimators.Note, fixed effects allow attrition to be correlated with ai . So if someunits are more likely to drop out of the sample, this is captured by ai .But, if this prob varies over time with unmeasured things affectinginvestment, problem.Michael R. RobertsLinear Panel Data Models28/56
First Difference EstimatorsFixed Effects EstimationRandom Effects (RE) EstimationDynamic Linear Panel Data Models (DLPDM)IntroductionFE IdentificationFE MiscellaneousBetween EstimatorBetween Estimator (BE) is the OLS estimator on thecross-sectional equation:ȳi β1 x̄i1 . βk x̄ik ai ūi , whereI.e., run a cross-sectional OLS regression on the time-series averagesThis produces biased estimates when ai is correlated with x̄iIf ai is uncorrelated with x̄i , we should use random effectsestimator (see below)Michael R. RobertsLinear Panel Data Models29/56
First Difference EstimatorsFixed Effects EstimationRandom Effects (RE) EstimationDynamic Linear Panel Data Models (DLPDM)IntroductionFE IdentificationFE MiscellaneousR2When estimating fixed effects model via FE, how do we interpretR 2?It is the amount of time variation in yit explained by the timevariation in XDemeaning removes all cross-sectional (between) variation prior toestimationMichael R. RobertsLinear Panel Data Models30/56
First Difference EstimatorsFixed Effects EstimationRandom Effects (RE) EstimationDynamic Linear Panel Data Models (DLPDM)RE AssumptionSame model as beforeyit β1 xit1 . βk xitk ai uit , t 1, 2, ., TOnly difference is that Random Effects assumes ai is uncorrelatedwith each explanatory variable, xitj , j 1, ., k; t 1, ., TCov (xitj , ai ) 0, t 1, ., T ; j 1, ., kThis is a very strong assumption in empirical corporate finance.Michael R. RobertsLinear Panel Data Models31/56
First Difference EstimatorsFixed Effects EstimationRandom Effects (RE) EstimationDynamic Linear Panel Data Models (DLPDM)RE Cont.Under RE assumption:12Using a transformation to eliminate ai is inefficientSlopes βj can be consistently estimated using a singlecross-section.no need for panel data.13This would be inefficient because we’re throwing away info.Can use pooled OLS to get consistent estimators.1This ignores serially correlation in composite error (vit ai uit )term sinceCorr (vit , vis ) σa2 /(σa2 σu2 ), t 6 s23Means OLS estimates give wrong SEs and test statistics.Use GLS to solveMichael R. RobertsLinear Panel Data Models32/56
First Difference EstimatorsFixed Effects EstimationRandom Effects (RE) EstimationDynamic Linear Panel Data Models (DLPDM)RE and GLS EstimationRecall GLS under heteroskedasticity? Just transform data (e.g.,divide by σui ) and use OLS.same idea hereTransformation to eliminate serial correlation is:λ 1 [σu2 /(σu2 T σa2 )]1/2which is [0, 1]Transformed equation is:yit λȳi β0 (1 λ) β1 (xit1 λx̄i1 ) . βk (xitk λx̄ik ) (vit λv̄i )where x̄ is time average.These are quasi-demeaned data for each variable.like withintransformation but for λMichael R. RobertsLinear Panel Data Models33/56
First Difference EstimatorsFixed Effects EstimationRandom Effects (RE) EstimationDynamic Linear Panel Data Models (DLPDM)RE and GLS CommentsJust run OLS on transformed data to get GLS estimator.FGLS estimator just uses a consistent estimate of λ. (Use pooledOLS or fixed effects residuals to estimate.)FGLS estimator is called Random Effects EstimatorRE Estimator is biased, consistent, and anorm when N gets big andT is fixed.We can estimate coef’s on time-invariant variables with RE.When λ 0, we have pooled OLSWhen λ 1, we have FE estimator.Michael R. RobertsLinear Panel Data Models34/56
First Difference EstimatorsFixed Effects EstimationRandom Effects (RE) EstimationDynamic Linear Panel Data Models (DLPDM)RE or FE?Often hard to justify RE assumption (ai xitj )If key explanatory variable is time-invariant, can’t use FE!Hausman (1978) test:1234Use RE unless test rejects orthogonality condition between ai and xitj .Rejection means key RE assumption fails and FE should be used.Failure to reject means RE and FE are sufficiently close that itdoesn’t matter which is chosen.Intuition: Compare the estimates under efficient RE and consistentFE. If close, use RE, if not close, use FE.Bottom line: Use FE in empirical corporate applications.Michael R. RobertsLinear Panel Data Models35/56
First Difference EstimatorsFixed Effects EstimationRandom Effects (RE) EstimationDynamic Linear Panel Data Models (DLPDM)Autoregressive ModelMultivariate Dynamic ModelsSetupThe model and approach in this section follows Bond 2002:yit ρyit 1 ai uit , ρ 1; N 1, ., N; t 2, ., TAssume the first ob comes in t 1Assume uit is independent across i, serially uncorrelated, anduncorrelated with ai .1Within unit dependence captured by aiAssume N is big, and T is small (typical in micro apps)1Asymptotics are derived letting N get big and holding T fixedexogenous variables, xitk and period fixed effects, vt have nosubstantive impact on discussionMichael R. RobertsLinear Panel Data Models36/56
First Difference EstimatorsFixed Effects EstimationRandom Effects (RE) EstimationDynamic Linear Panel Data Models (DLPDM)Autoregressive ModelMultivariate Dynamic ModelsThe ProblemFixed effects create endogeneity problem.Explanatory variable yit 1 is correlated with error ai uitCov (yit 1 , ai uit ) Cov (ai uit 1 , ai uit ) Var (ai ) 0Correlation is 0 OLS produces upward biased andinconsistent estimate of ρ (Recall omitted variables bias formula.)Corr (yit 1 , ai ) 0 and Corr (yit , yit 1 ) 0Bias does not go away as the number of time periods increases!Michael R. RobertsLinear Panel Data Models37/56
First Difference EstimatorsFixed Effects EstimationRandom Effects (RE) EstimationDynamic Linear Panel Data Models (DLPDM)Autoregressive ModelMultivariate Dynamic ModelsWithin Estimator - Solve 1 ProblemWithin estimator eliminates this form of inconsistency by getting ridof fixed effect aiÿit β1 ÿit 1 üit , t 2, ., Twhereÿit 1/TTXyit ; ÿit 1 1/(T 1)i 2T 1Xyit ; üit 1/Ti 1Michael R. RobertsLinear Panel Data ModelsTXuiti 238/56
First Difference EstimatorsFixed Effects EstimationRandom Effects (RE) EstimationDynamic Linear Panel Data Models (DLPDM)Autoregressive ModelMultivariate Dynamic ModelsWithin Estimator - Create Another ProblemIntroduces another form of inconsistency sinceCorr (ÿit 1 , üit ) Corr (yit 1 T 1T1 X1 Xyit , uit uit )T 1Ti 1i 2is not equal to zero. Specifically,1Corr (yit 1 , uit 1 ) 0T 11Corr ( yit , uit ) 0T 111Corr ( yit 1 , uit 1 ) 0, t 2, ., T 1T 1T 1Negative corr dominate positive within estimator impartsnegative bias on estimate of ρ. (Nickell (1981))Bias disappears with big T , but not big NMichael R. RobertsLinear Panel Data Models39/56
First Difference EstimatorsFixed Effects EstimationRandom Effects (RE) EstimationDynamic Linear Panel Data Models (DLPDM)Autoregressive ModelMultivariate Dynamic ModelsBracketing TruthOLS estimate of ρ is biased upWithin estimate of ρ is biased down true ρ will likely lie between these estimates. I.e., consistentestimator should be in these bounds.When model is well specified and this bracketing is not observed,then12maybe inconsistency, orsevere finite sample biasfor consistent estimatorMichael R. RobertsLinear Panel Data Models40/56
First Difference EstimatorsFixed Effects EstimationRandom Effects (RE) EstimationDynamic Linear Panel Data Models (DLPDM)Autoregressive ModelMultivariate Dynamic ModelsML EstimatorsSee Blundell and Smith (1991), Binder, Hsiao, and Pesaran (2000),and Hsiao (2003).Problem with ML in small T panels is that distribution of yit fort 2, ., T depends crucially on distribution of yi1 , initial condition.yi1 could be123456stochastic,non-stochastic,correlated with ai ,uncorrelated with ai ,specified so that the mean of the yit series for each i ismean-stationary (ai /(1 ρ)), orspecified so that higher order stationarity properties are satisfied.Each assumption generates different likelihood functions, differentestimates.Misspecification generates inconsistent estimates.Michael R. RobertsLinear Panel Data Models41/56
First Difference EstimatorsFixed Effects EstimationRandom Effects (RE) EstimationDynamic Linear Panel Data Models (DLPDM)Autoregressive ModelMultivariate Dynamic ModelsFirst Difference EstimatorFirst-differencing eliminates fixed effects yit ρ yit 1 uit , ρ 1; i 1, ., N; t 3, ., Twhere yit yit yit 1Key: first differencing doesn’t introduce all of the realizations of thedisturbance into the error term like within estimator. But,Corr ( yit 1 , uit ) Corr (yit 1 yit 2 , uit uit 1 ) 0 downward bias & typically greater than within estimator.When T 3, within and first-difference estimators identical.Recall when T 2 and no lagged dependent var, within andfirst-difference estimators identical.Michael R. RobertsLinear Panel Data Models42/56
First Difference EstimatorsFixed Effects EstimationRandom Effects (RE) EstimationDynamic Linear Panel Data Models (DLPDM)Autoregressive ModelMultivariate Dynamic ModelsIV Estimators 1Require weaker assumptions about initial conditions than MLNeed predetermined initial conditions (i.e., yi1 uncorrelated withall future errors uit , t 2, .T .First-differenced 2SLS estimator (Anderson and Hsiao (1981, 1982)Need an instrument for yit that is uncorrelated with uitPredetermined initial condition serially uncorrelated uit lagged level yit 2 is uncorrelated with uit and available as aninstrument for yit 12SLS estimator is consistent in large N, fixed T and identifies ρ aslong as T 32SLS is also consistent in large T , but so is within estimatorMichael R. RobertsLinear Panel Data Models43/56
First Difference EstimatorsFixed Effects EstimationRandom Effects (RE) EstimationDynamic Linear
Dynamic Linear Panel Data Models (DLPDM) Two Period Model Policy Analysis Three Period Panel General Period Panel Example (Cont) Panel approach to link between crime and unemployment. crimeRate it 0 0d78 t 1unem it a i u it where d87 1 if year is 1987, 0 otherwise, and a i is an unobserved city e ect that doesn't change over time .