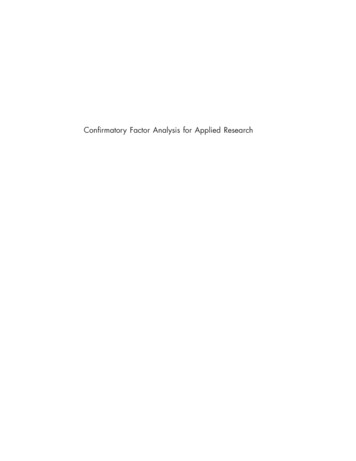
Transcription
Confirmatory Factor Analysis for Applied Research
Methodology in the Social SciencesDavid A. Kenny, Series EditorSPECTRAL ANALYSIS OF TIME-SERIES DATARebecca M. WarnerA PRIMER ON REGRESSION ARTIFACTSDonald T. Campbell and David A. KennyREGRESSION ANALYSIS FOR CATEGORICAL MODERATORSHerman AguinisHOW TO CONDUCT BEHAVIORAL RESEARCH OVER THE INTERNET:A BEGINNER’S GUIDE TO HTML AND CGI/PERLR. Chris FraleyPRINCIPLES AND PRACTICE OF STRUCTURAL EQUATION MODELINGSecond EditionRex B. KlineCONFIRMATORY FACTOR ANALYSIS FOR APPLIED RESEARCHTimothy A. Brown
Confirmatory FactorAnalysis forApplied ResearchTimothy A. BrownSERIES EDITOR’S NOTE byDavid A. KennyTHE GUILFORD PRESSNew York London
2006 The Guilford PressA Division of Guilford Publications, Inc.72 Spring Street, New York, NY 10012www.guilford.comAll rights reservedNo part of this book may be reproduced, translated, stored in aretrieval system, or transmitted, in any form or by any means,electronic, mechanical, photocopying, microfilming, recording,or otherwise, without written permission from the Publisher.Printed in the United States of AmericaThis book is printed on acid-free paper.Last digit is print number:987654321Library of Congress Cataloging-in-Publication DataBrown, Timothy A.Confirmatory factor analysis for applied research /Timothy A. Brown.p. cm. — (Methodology in the social sciences)Includes bibliographical references and index.ISBN-13: 978-1-59385-274-0 (pbk.)ISBN-10: 1-59385-274-6 (pbk.)ISBN-13: 978-1-59385-275-7 (hardcover)ISBN-10: 1-59385-275-4 (hardcover)1. Factor analysis. I. Title. II. Series.BF39.2.F32B76 2006150.1′5195354—dc222006001103
For my father, Kaye,and Nick and Greg
About the AuthorTimothy A. Brown, PsyD, is a professor in the Department of Psychologyat Boston University, and Director of Research at Boston University’sCenter for Anxiety and Related Disorders. He has published extensively inthe areas of the classification of anxiety and mood disorders, vulnerabilityto emotional disorders, psychometrics, and methodological advances insocial sciences research. In addition to conducting his own grant-supported research, Dr. Brown serves as a statistical investigator or consultanton numerous federally funded research projects. He has been on the editorial boards of several scientific journals, including recent appointments asAssociate Editor of the Journal of Abnormal Psychology and BehaviorTherapy.vii
Series Editor’s NoteSeries Editor’s NoteFor some reason, the topic of confirmatory factor analysis (CFA) has notreceived the attention that it deserves. Two closely related topics, exploratory factor analysis (EFA) and structural equation modeling (SEM), havedozens of textbooks written about them. Book-length treatments of CFAare rare and that is what makes this book distinctive.One might think that there are so few books on CFA because it is sorarely used. However, this is not the case. Very often, those who conductEFA follow up the analysis with CFA. Additionally, SEM always involves ameasurement model and very often the best way to test that model is withCFA. Poor-fitting structural equation models are almost always due to CFAproblems. Thus, to be proficient at SEM, the analyst must know CFA. Thisbook very nicely explains the links between CFA and these two differentmethods, in particular the natural process of beginning with EFA, proceeding to CFA, and then SEM.I think it is ironic that SEM has received so much more attention thanCFA, because the social and behavioral sciences have learned much morefrom CFA than from SEM. In particular, through CFA we are able tounderstand the construct validity of attitudes and personality, and CFAprovides important information about the relative stability of individualdifferences throughout the lifespan.Unlike most books on factor analysis, this one spares us all the matrices with their transposes, Kronecker products, and inverses. Certainlymatrix algebra is critical in the theory, proofs, and estimation of CFA, butfor day-to-day practitioners, it just gets in the way. This is not to say thatthe author, Timothy A. Brown, doesn’t discuss technical issues where necessary. The text is complicated where appropriate.ix
xSeries Editor’s NoteAn example of one such complicated topic is the multitrait–multimethod matrix, first proposed by Donald Campbell and DonaldFiske. I am pleased that Brown decided to devote a full chapter to thetopic. Interestingly, a generation of researchers tried to find EFA modelsfor the matrix and never developed a completely satisfactory model.Another generation of researchers worked on several CFA models for thematrix, and Brown very nicely summarizes the models they produced.Another useful feature of this book is that it contains an entire chapterdevoted to issues of statistical power and sample sizes. Investigators needto make decisions, costly both in terms of time and money, about samplesize. Very often they make those decisions using rather arbitrary procedures. The book outlines a formal and practical approach to that question.For breadth of applications, the book provides examples from severaldifferent areas of the social and behavioral sciences. It also illustrates theanalyses using several different software programs. Preferences for computer programs change as fast as preferences do for hair styles; thus, it isan advantage that the book is not tied to one computer program. Mostreaders would benefit from analyzing data of their own as they read thebook.Construct validity, instrument development and validation, reductionof the number of variables, and sources of bias in measurement, to namejust a few, are subjects supported by high-quality CFA. Almost all researchdata include many variables; therefore, Brown’s detailed and careful treatment of this important topic will be of benefit in almost all research situations. A gap in the field of multivariate data analysis that has existed for fartoo long has finally been filled. Researchers now have a readable, detailed,and practical discussion of CFA.DAVID A. KENNY
PrefacePrefaceThis book was written for the simple reason that no other book of its kindhad been published before. Although many books on structural equationmodeling (SEM) exist, this is the first book devoted solely to the topic ofconfirmatory factor analysis (CFA). Accordingly, for the first time, manyimportant topics are brought under one cover—for example, the similarities/differences between exploratory factor analysis (EFA) and CFA, theuse of maximum likelihood EFA procedures as a precursor to CFA, diagnosing and rectifying the various sources for the ill-fit of a measurementmodel, analysis of mean structures, modeling with multiple groups (e.g.,MIMIC), CFA scale reliability evaluation, formative indicator models, andhigher-order factor analysis. After covering the fundamentals and varioustypes of CFA in the earlier chapters, in later chapters I address issues likeCFA with non-normal or categorical indicators, handling missing data,and power analysis/sample size determination, which are germane to SEMmodels of any type. Although it is equally important to CFA practice,another reason I included this material was because of the lack of adequatecoverage in preexisting SEM sourcebooks. Thus, I hope the book will serveas a useful guide to researchers working with a latent variable model of anytype. The book is not tied to specific latent variable software packages, andin fact the five most popular programs are featured throughout (Amos,EQS, LISREL, Mplus, SAS/CALIS). However, readers will note that thisbook is the first to provide an extensive treatment of Mplus, a programthat is becoming increasingly popular with applied researchers for its easeof use with complex models and data (e.g., categorical outcomes, categorical latent variables, multilevel data).The target readership of this book is applied researchers and graduatestudents working within any domain of social and behavioral sciencesxi
xiiPreface(e.g., psychology, education, political science, management/marketing,sociology, public health). In the classroom, this book can serve as a primary or supplemental text in courses on advanced statistics, factor analysis, SEM, or psychometrics/scale development. For applied researchers,this book can be used either as a resource for learning the procedures ofCFA or, for more experienced readers, as a reference guide for dealing withcomplex CFA models or data issues. What each chapter specifically coversis described in Chapter 1. The first five chapters deal with the fundamentals of CFA: what the researcher needs to know to conduct a CFA of anytype. Thus, especially for readers new to CFA, it is recommended that thefirst five chapters be read in order, as this material is the foundation for theremainder of the book. Chapters 6 through 10 address specific types ofCFA and other issues such as dealing with missing or categorical data andpower analysis. The reading order of the second group of chapters is lessimportant than for the first.Advances in quantitative methodology are often slow to be picked upby applied researchers because such methods are usually disseminated in amanner inaccessible to many end users (e.g., formula-driven articles inmathematical/statistical journals). This is unfortunate, because multivariate statistics can be readily and properly employed by any researcherprovided that the test’s assumptions, steps, common pitfalls, and so on, arelaid out clearly. Keeping with that philosophy, this book was written be auser-friendly guide to conducting CFA with real data sets, with an emphasis more on conceptual and practical aspects than on quantitative formulas. Several strategies were used to help meet this goal: (1) every key concept is accompanied by an applied data set and the syntax and output fromthe leading latent variable software packages; (2) tables are included thatrecap the procedures or steps of the methods being presented (e.g., how toconduct an EFA, how to write up the results of a CFA study); (3) numerous figures are provided that graphically illustrate some of the more complicated concepts or procedures (e.g., EFA factor rotation, forms of measurement invariance, types of nonpositive definite matrices, identificationof formative indicator models), and (4) many chapters contain appendiceswith user-friendly illustrations of seemingly complex quantitative operations (e.g., data generation in Monte Carlo simulation research, calculationof matrix determinants and their role in model fit and improper solutions). I have also provided a website (http://people.bu.edu/tabrown/)with data and computer files for the book’s examples and other materials(e.g., updates, links to other CFA resources). I hope that through the useof the aforementioned materials, even the most complicated CFA model or
Prefacexiiidata issue has been demystified and can now be readily tackled by thereader.In closing, I would like to thank the people who were instrumental inthe realization of this volume. First, thanks to Series Editor David A.Kenny, who, in addition to providing very helpful comments on specificsections, played an enormous role in helping me to shape the scope andcoverage of this book. In addition, I would like to extend my appreciationto C. Deborah Laughton, Publisher, Methodology and Statistics, who provided many excellent suggestions and positive feedback throughout theprocess and who secured several terrific outside reviews. Indeed, I amgrateful to the following reviewers, whose uniformly constructive andthoughtful feedback helped me strengthen the book considerably: LarryPrice, Texas State University–San Marcos; Christopher Federico, University of Minnesota; and Ke-Hai Yuan, University of Notre Dame. I wouldalso like to thank William Meyer, Production Editor at The Guilford Press,for his work in bringing a very technically complex manuscript to press.And finally, special thanks to my wife, Bonnie, for her continuous encouragement and support.
ContentsContents1 Introduction1Uses of Confirmatory Factor Analysis / 1Psychometric Evaluation of Test Instruments / 1Construct Validation / 2Method Effects / 3Measurement Invariance Evaluation / 4Why a Book on CFA? / 5Coverage of the Book / 6Other Considerations / 8Summary / 112 The Common Factor Model12and Exploratory Factor AnalysisOverview of the Common Factor Model / 12Procedures of EFA / 20FactorFactorFactorFactorExtraction / 21Selection / 23Rotation / 30Scores / 36Summary / 373 Introduction to CFA40Similarities and Differences of EFA and CFA / 40Common Factor Model / 40Standardized and Unstandardized Solutions / 41Indicator Cross-Loadings/Model Parsimony / 42Unique Variances / 46Model Comparison / 47xv
xviContentsPurposes and Advantages of CFA / 49Parameters of a CFA Model / 53Fundamental Equations of a CFA Model / 59CFA Model Identification / 62Scaling the Latent Variable / 62Statistical Identification / 63Guidelines for Model Identification / 71Estimation of CFA Model Parameters / 72Illustration / 76Descriptive Goodness-of-Fit Indices / 81Absolute Fit / 82Parsimony Correction / 83Comparative Fit / 84Guidelines for Interpreting Goodness-of-Fit Indices / 86Summary / 88Appendix 3.1. Communalities, Model-Implied Correlations, and FactorCorrelations in EFA and CFA / 90Appendix 3.2. Obtaining a Solution for a Just-Identified Factor Model / 93Appendix 3.3. Hand Calculation of FML for the Figure 3.8 Path Model / 964 Specification and Interpretation of CFA ModelsAn Applied Example of a CFA Measurement Model / 103Model Specification / 106Substantive Justification / 106Defining the Metric of Latent Variables / 106Data Screening and Selection of the Fitting Function / 107Running the CFA Analysis / 108Model Evaluation / 113Overall Goodness of Fit / 113Localized Areas of Strain / 114Residuals / 115Modification Indices / 119Unnecessary Parameters / 124Interpretability, Size, and Statistical Significance of the ParameterEstimates / 126Interpretation and Calculation of CFA Model ParameterEstimates / 132CFA Models with Single Indicators / 138Reporting a CFA Study / 144Summary / 148Appendix 4.1. Model Identification Affects the Standard Errors of theParameter Estimates / 150Appendix 4.2. Goodness of Model Fit Does Not Ensure Meaningful ParameterEstimates / 153Appendix 4.3. Example Report of the Two-Factor CFA Model of Neuroticismand Extraversion / 155103
Contents5 CFA Model Revision and Comparisonxvii157Goals of Model Respecification / 157Sources of Poor-Fitting CFA Solutions / 159Number of Factors / 159Indicators and Factor Loadings / 167Correlated Errors / 181Improper Solutions and Nonpositive Definite Matrices / 187EFA in the CFA Framework / 193Model Identification Revisited / 202Equivalent CFA Solutions / 203Summary / 2096 CFA of Multitrait–Multimethod Matrices212Correlated versus Random Measurement Error Revisited / 212The Multitrait–Multimethod Matrix / 213CFA Approaches to Analyzing the MTMM Matrix / 217Correlated Methods Models / 218Correlated Uniqueness Models / 220Advantages and Disadvantages of Correlated Methods andCorrelated Uniqueness Models / 227Other CFA Parameterizations of MTMM Data / 229Consequences of Not Modeling Method Variance andMeasurement Error / 231Summary / 2337 CFA with Equality Constraints,Multiple Groups, and Mean StructuresOverview of Equality Constraints / 237Equality Constraints within a Single Group / 238Congeneric, Tau-Equivalent, and Parallel Indicators / 238Longitudinal Measurement Invariance / 252CFA in Multiple Groups / 266Overview of Multiple-Groups Solutions / 266Multiple-Groups CFA / 268Selected Issues in Single- and Multiple-Groups CFA InvarianceEvaluation / 299MIMIC Models (CFA with Covariates) / 304Summary / 316Appendix 7.1. Reproduction of the Observed Variance–Covariance Matrix withTau-Equivalent Indicators of Auditory Memory / 318236
xviiiContents8 Other Types of CFA Models:320Higher-Order Factor Analysis, Scale ReliabilityEvaluation, and Formative IndicatorsHigher-Order Factor Analysis / 320Second-Order Factor Analysis / 322Schmid–Leiman Transformation / 334Scale Reliability Estimation / 337Point Estimation of Scale Reliability / 337Standard Error and Interval Estimation of Scale Reliability / 345Models with Formative Indicators / 351Summary / 3629 Data Issues in CFA:363Missing, Non-Normal, and Categorical DataCFA with Missing Data / 363Mechanisms of Missing Data / 364Conventional Approaches to Missing Data / 365Recommended Missing Data Strategies / 367CFA with Non-Normal or Categorical Data / 378Non-Normal, Continuous Data / 379Categorical Data / 387Other Potential Remedies for Indicator Non-Normality / 404Summary / 41010 Statistical Power and Sample Size412Overview / 412Satorra–Saris Method / 413Monte Carlo Approach / 420Summary and Future Directions in CFA / 429Appendix 10.1. Monte Carlo Simulation in Greater Depth:Data Generation / 434References439Author Index455Subject Index459Web address for the author’s data and computer filesand other resources: http://people.bu.edu/tabrown/
CONFIRMATORY FACTOR ANALYSIS FOR APPLIED RESEARCHIntroduction1IntroductionUSES OF CONFIRMATORY FACTOR ANALYSISConfirmatory factor analysis (CFA) is a type of structural equation modeling (SEM) that deals specifically with measurement models, that is, therelationships between observed measures or indicators (e.g., test items, testscores, behavioral observation ratings) and latent variables or factors. Afundamental feature of CFA is its hypothesis-driven nature. It is unlike itscounterpart, exploratory factor analysis (EFA), in that the researcher mustprespecify all aspects of the CFA model. Thus, the researcher must have afirm a priori sense, based on past evidence and theory, of the number offactors that exist in the data, of which indicators are related to which factors, and so forth. In addition to its greater emphasis on theory andhypothesis testing, the CFA framework provides many other analytic possibilities that are not available in EFA. These possibilities include the evaluation of method effects and the examination of the stability or invarianceof the factor model over time or informants. Moreover, for the reasons discussed below, CFA should be conducted prior to the specification of anSEM model.CFA has become one of the most commonly used statistical procedures in applied research. This is because CFA is well equipped to addressthe types of questions that researchers often ask. Some of the most common uses of CFA are as follows.Psychometric Evaluation of Test InstrumentsCFA is almost always used during the process of scale development toexamine the latent structure of a test instrument (e.g., a questionnaire). In1
2C ONFIRMATORY F ACTOR A NALYSISFORA PPLIED R ESEARCHthis context, CFA is used to verify the number of underlying dimensions ofthe instrument (factors) and the pattern of item–factor relationships (factor loadings). CFA also assists in the determination of how a test should bescored. When the latent structure is multifactorial (i.e., two or more factors), the pattern of factor loadings supported by CFA will designate how atest might be scored using subscales; that is, the number of factors is indicative of the number of subscales, and the pattern of item–factor relationships (which items load on which factors) indicates how the subscalesshould be scored. Depending on other results and extensions of the analysis, CFA may support the use of total scores (composite of all items) inaddition to subscale scores (composites of subsets of items). For example,the viability of a single total score might be indicated when the relationships among the latent dimensions (subscales) of a test can be accountedfor by one higher-order factor, and when the test items are meaningfullyrelated to the higher-order factor (see higher-order CFA; Chapter 8). CFAis an important analytic tool for other aspects of psychometric evaluation.It can be used to estimate the scale reliability of test instruments in a manner that avoids the problems of traditional methods (e.g., Cronbach’salpha; see Chapter 8). Given recent advances in the analysis of categoricaldata (e.g., binary true/false test items), CFA now offers a comparable analytic framework to item response theory (IRT). In fact, in some ways, CFAprovides more analytic flexibility than the traditional IRT model (seeChapter 9).Construct ValidationAkin to a factor in CFA, a construct is a theoretical concept. In clinical psychology and psychiatry, for example, the mental disorders (e.g., majordepression, schizophrenia) are constructs manifested by various clusters ofsymptoms that are reported by the patient or observed by others. In sociology, juvenile delinquency might be construed as a multidimensionalconstruct defined by various forms of misconduct (e.g., property crimes,interpersonal violence, drug use, academic misconduct). CFA is an indispensable analytic tool for construct validation in the social and behavioralsciences. The results of CFA can provide compelling evidence of the convergent and discriminant validity of theoretical constructs. Convergentvalidity is indicated by evidence that different indicators of theoreticallysimilar or overlapping constructs are strongly interrelated; for example,symptoms purported to be manifestations of a single mental disorder load
Introduction3on the same factor. Discriminant validity is indicated by results showingthat indicators of theoretically distinct constructs are not highly intercorrelated; for example, behaviors purported to be manifestations of different types of delinquency load on separate factors, and the factors are notso highly correlated as to indicate that a broader construct has been erroneously separated into two or more factors. One of the most elegant usesof CFA in construct validation is the analysis of multitrait–multimethodmatrices (see Chapter 6). A fundamental strength of CFA approaches toconstruct validation is that the resulting estimates of convergent and discriminant validity are adjusted for measurement error and an error theory(see the “Method Effects” section, below). Thus, CFA provides a strongeranalytic framework than traditional methods that do not account for measurement error (e.g., ordinary least squares approaches such as correlation/multiple regression assume variables in the analysis are free of measurement error).Method EffectsOften, some of the covariation of observed measures is due to sourcesother than the substantive latent factors. For instance, consider the situation where four measures of employee morale have been collected; twoindicators are employees’ self-reports (e.g., questionnaires), the othertwo are obtained from supervisors (e.g., behavioral observations). Itwould be presumed that the four measures are intercorrelated becauseeach is a manifest indicator of the underlying construct of morale. However, it is also likely that the employee self-report measures are morehighly correlated with each other than with the supervisor measures,and vice versa. This additional covariation is not due to the underlyingconstruct of morale, but reflects shared method variance. A method effectexists when additional covariation among indicators is introduced by themeasurement approach. Method effects can also occur within a singleassessment modality. For example, method effects are usually present inquestionnaires that contain some combination of positively and negatively worded items (e.g., see Chapters 3 and 6). Unfortunately, EFA isincapable of estimating method effects. In fact, the use of EFA whenmethod effects exist in the data can produce misleading results—that is,yield additional factors that are not substantively meaningful but insteadstem from artifacts of measurement. In CFA, however, method effectscan be specified as part of the error theory of the measurement model.
4C ONFIRMATORY F ACTOR A NALYSISFORA PPLIED R ESEARCHThe advantages of estimating method effects within CFA include theability to (1) specify measurement models that are more conceptuallyviable; (2) determine the amount of method variance in each indicator;and (3) obtain better estimates of the relationships of the indicators tothe latent factors, and the relationships among latent variables (seeChapters 5 and 6).Measurement Invariance EvaluationAnother key strength of CFA is its ability to determine how well measurement models generalize across groups of individuals or across time. Measurement invariance evaluation is an important aspect of test development.If a test is intended to be administered in a heterogeneous population, itshould be established that its measurement properties are equivalent insubgroups of the population (e.g., gender, race). A test is said to be biasedwhen some of its items do not measure the underlying construct comparably across groups. Test bias can be serious, such as in situations where agiven score on a cognitive ability or job aptitude test does not represent thesame true level of ability/aptitude in male and female respondents. Statedanother way, the test would be biased against women if, for a given level oftrue intelligence, men tended to score several IQ units higher on the testthan women. These questions can be addressed in CFA by multiplegroups solutions and MIMIC (multiple indicators, multiple causes) models (Chapter 7). For instance, in a multiple-groups CFA solution, the measurement model is estimated simultaneously in various subgroups (e.g.,men and women). Other restrictions are placed on the multiple-groupssolution to determine the equivalence of the measurement model acrossgroups; for instance, if the factor loadings are equivalent, the magnitude ofthe relationships between the test items and the underlying construct (e.g.,cognitive ability) are the same in men and women. Multiple-groups CFAsolutions are also used to examine longitudinal measurement invariance.This is a very important aspect of latent variable analyses of repeated measures designs. In the absence of such evaluation, it cannot be determinedwhether temporal change in a construct is due to true change or tochanges in the structure or measurement of the construct over time.Multiple-groups analysis can be applied to any type of CFA or SEM model.For example, these procedures can be incorporated into the analysis ofmultitrait–multimethod data to examine the generalizability of constructvalidity across groups.
Introduction5WHY A BOOK ON CFA?It also seems appropriate to begin this volume by addressing the question,“Is there really a need for a book devoted solely to the topic of CFA?” Onthe author’s bookshelf sit 15 books on the subject of SEM. Why not go toone of these SEM books to learn about CFA? Given that CFA is a form ofSEM, virtually all of these books provide some introduction to CFA. However, this coverage typically consists of a chapter at best. As this book willattest, CFA is a very broad and complex topic and extant SEM books onlyscratch the surface. This is unfortunate because, in applied SEM research,most of the work deals with measurement models (CFA). Indeed, manyapplied research questions are addressed using CFA as the primary analytic procedure (e.g., psychometric evaluation of test instruments, construct validation). Another large proportion of SEM studies focus on structural regression models, that is, the manner in which latent factors areinterrelated. Although CFA is not the ultimate analysis in such studies, aviable measurement model (CFA) must be established prior to evaluatingthe structural (e.g., regressive) relationships among the latent variables ofinterest. When poor model fit is encountered in such studies, it is morelikely that it stems from misspecifications in the measurement portion ofthe model (i.e., the manner in which observed variables are related tolatent factors) than from the structural component that specifies the interrelationships of latent factors. This is because there are usually morethings that can go wrong in the measurement model than in the structuralmodel (e.g., problems in the selection of observed measures, misspecifiedfactor loadings, additional sources of covariation among observed measures that cannot be accounted for by the latent factors). Existing SEMresources do not provide sufficient details on the sources of ill fit in CFAmeasurement models or how such models can be diagnosed and respecified. Moreover, advanced applications of CFA are rarely discussed ingeneral SEM books (e.g., CFA with categorical indicators, scale reliabilityevaluation, MIMIC models, formative indicators).Given the importance and widespread use of CFA, this book was written to provide an in-depth treatment of the concepts, procedures, pitfalls,and extensions of this methodology. Although the overriding objective ofthe book is to provide critical information on applied CFA that has notreceived adequate coverage in the past, it is important to note that the topics pertain to SEM in general (e.g., sample size/power analysis, missingdata, non-normal or categorical data, formative indicators). Thus, it is
6C ONFIRMATORY F ACTOR A NALYSISFORA PPLIED R ESEARCHhoped that this book will also be a useful resource to researchers using anyform of SEM.COVERAGE OF THE BOOKThe first five chapters of this book present the fundamental concepts andprocedures of CFA. Chapter 2 introduces the reader to the concepts andterminology of the common factor model. The common factor model isintroduced in context of EFA. This book is not intended to be a comprehensive treatment of the principles and practice of EFA. However, an overview of the concepts and operations of EFA is provided in Chapter 2 forseveral reasons: (1) most of the concepts and terminology of EFA generalize to CFA; (2) it will foster the discussion of the similarities and differences of EFA and CFA in later chapters (e.g., Chapt
CFA or, for more experienced readers, as a reference guide for dealing with complex CFA models or data issues. What each chapter specifically covers is described in Chapter 1. The first five chapters deal with the fundamen-tals of CFA: what the researcher needs to know to conduct a CFA of any type.