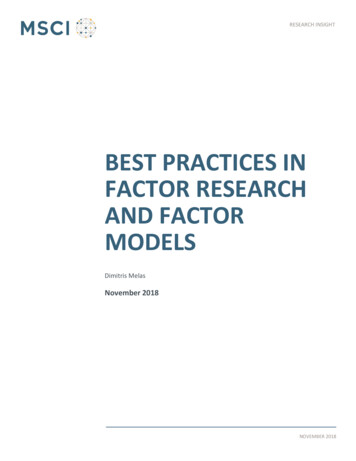
Transcription
RESEARCH INSIGHTBEST PRACTICES INFACTOR RESEARCHAND FACTORMODELSDimitris MelasNovember 2018NOVEMBER 2018
BEST PRACTICES IN FACTOR RESEARCH AND FACTOR MODELS NOVEMBER 2018CONTENTSExecutive Summary. 3Foundations of MSCI Factors . 3Foundations of MSCI Models . 6A New Frontier. 11References . 12 2018 MSCI Inc. All rights reserved. Please refer to the disclaimer at the end of this document.MSCI.COM PAGE 2 OF 17
BEST PRACTICES IN FACTOR RESEARCH AND FACTOR MODELS NOVEMBER 2018EXECUTIVE SUMMARYFactors define the sources of portfolio risk and return. In this paper, we reviewthe theoretical and empirical foundations of our factor research and factormodels. MSCI factor research is firmly grounded in academic theory andempirical evidence. MSCI factor models are based on robust econometrictechniques and reflect best investment practice. MSCI methodologies aretransparent and publicly available. This is why the world’s leading institutionalinvestors use MSCI factor models and analytics in their investment processes.FOUNDATIONS OF MSCI FACTORSThe theoretical foundations of the factors we use in our models and reflect inour factor indexes can be traced back to pioneering academic research publishedseveral decades ago. Markowitz (1952) provided an analytically tractabledefinition of risk and established mean-variance optimization as a formalmethod for building portfolios by trading off risk and return. Sharpe (1964)introduced the Capital Asset Pricing Model that elegantly captures the idea thatthe market is the most important common driver of portfolio performance. Ross(1976) extended the market model to include multiple factors that may exertcommon influences on asset prices and portfolio returns. Rosenberg andMarathe (1976) developed the theory that the effects of macroeconomic eventson individual securities could be captured through microeconomic characteristics– essentially common factors, such as industry membership, financial structureor growth orientation.A substantial body of empirical research followed over the next four decades,aiming to establish the precise nature of the common factors affecting risk andreturn in different asset classes and markets. Many studies also proposedhypotheses explaining why some of these factors may be priced and thereforewhy assets and portfolios that emphasize these characteristics may earn positiveexcess returns. Potential explanations include systematic risk, behavioral bias,asymmetric information, and institutional constraints. In equities, eight factorgroups have been documented through empirical research and have been usedextensively in portfolio risk models and in active investment strategies: value, 2018 MSCI Inc. All rights reserved. Please refer to the disclaimer at the end of this document.MSCI.COM PAGE 3 OF 17
BEST PRACTICES IN FACTOR RESEARCH AND FACTOR MODELS NOVEMBER 2018size, momentum, volatility, quality, yield, growth and liquidity. These are theeight factor groups we use in our Global Equity Model (GEMLT) and in our FactorClassification Standard (FaCS).Value and size were established early on as important common influences andpotential sources of excess return, for example, Basu (1977), Banz (1981), Brown(1983) and Fama and French (1993). Jegadeesh and Titman (1993) documentedthe existence of cross sectional momentum in U.S. equities while Carhart (1997)added momentum to the Fama and French three-factor model. Black (1972),Haugen and Baker (1991) and Frazzini and Pedersen (2014) documented the lowvolatility effect and established volatility as an important equity factor andpotential source of excess return.Sloan (1996) showed that accounting accruals are negatively correlated withfuture stock returns while Novy-Marx (2013) found that high profitabilitycompanies earn higher returns despite having higher valuations. Profitability andearnings quality are often viewed as different dimensions of the quality factor.Other metrics used to quantify quality include financial leverage, earningsvariability and asset growth (investment quality). Several studies found a linkbetween dividend yield and subsequent stock performance, including Blume(1980), Fama and French (1988) and Arnott and Asness (2003). Growth is afundamental input into all valuation models and has been investigated in anumber of empirical studies, for example, Ofer (1975), Bauman and Dowen(1988) and Fama and French (2006). Finally, several studies document the linkbetween liquidity and the cross section of returns, including Amihud andMendelson (1989), Amihud (2002) and Pastor and Stambaugh (2003).We use multiple descriptors (financial ratios and other stock level characteristics)to capture each factor. We do this for five reasons:1. Evaluating multiple descriptors reflects academic theory. For example, manyacademic studies go beyond price to book and examine other financial ratiosthat reflect value, for example, price to earnings (Basu, 1977), price to sales(Barbee, 1996), price to cash flow (Asness, 2000), etc.2. Evaluating multiple descriptors reflects investment practice. For example,value investors do not exclusively measure value through price to book; they 2018 MSCI Inc. All rights reserved. Please refer to the disclaimer at the end of this document.MSCI.COM PAGE 4 OF 17
BEST PRACTICES IN FACTOR RESEARCH AND FACTOR MODELS NOVEMBER 2018recognise that this is only one of several complementary measures to assessthe intrinsic value of a stock and use different financial ratios to capture themultiple dimensions of value.3. Multiple descriptors shed light on different aspects of a company’s financialposition and the relationship between market price and fundamentals. Forexample, book value may be a good measure of value for companies that relypredominantly on fixed tangible assets. But for other types of companies, forinstance those in the technology sector, that have intangible assets, othervaluation measures such as those based on sales, earnings, cash flow, andenterprise value may be more appropriate.4. By using multiple descriptors to construct a factor, we are able to deal withmissing data or data errors more effectively.5. Combining multiple similar attributes into one factor helps reduce crosssectional correlations between factors and therefore control for potentialcollinearity in the regressions.The weights used to combine descriptors into factors and factors into groups arenot the result of data mining or in sample optimization. Equal weights are usedunless there are compelling reasons to deviate from them. Any deviation fromequal weights is driven by economic rationale, followed and supported byempirical verification. The latter is conducted across different markets and timeperiods to avoid spurious relationships. Ultimately, expert analysis must beapplied to determine which descriptors to combine into factors and theirweights. This approach is consistent with the advice from Markowitz (1952), whowrote that the procedures for forecasting risk and return “ should combinestatistical techniques and the judgement of practical men” (p. 91). Grinold andKahn (1994) provide similar advice: “Research leading to the appropriate factors,then, depends both on statistical techniques and on investment intuition.Statistical techniques can identify the most incisive and interesting factors.Investment intuition can help identify intuitive factors. Factors can havestatistical significance or investment significance or both. Model research musttake both forms of significance into account.” (p. 72). 2018 MSCI Inc. All rights reserved. Please refer to the disclaimer at the end of this document.MSCI.COM PAGE 5 OF 17
BEST PRACTICES IN FACTOR RESEARCH AND FACTOR MODELS NOVEMBER 2018New common factors demonstrating long-term equity returns are not discoveredvery often. The first Barra Global Equity Model (GEM), launched in 1989,included four style factors: value, size, momentum and volatility. The currentBarra Global Equity Model (GEMLT), launched in 2015, includes eight factorgroups: value, size, momentum, volatility, quality, yield, growth and liquidity.The additional factors reflect the gradual evolution of global equity markets andthe global investment process over the last three decades. They also reflectadvances in academic research, improved data availability and betterunderstanding by investors of the sources of risk and return. We see similargradual evolution over time in familiar academic models. For example, theoriginal Fama French model (1993) only had three factors (market, value, size)while the latest version of this model (2015) has five factors (market, value, size,profitability, investment). The gradual evolution of markets and investmentprocesses is also captured in other analytical tools that are widely used byinvestors. For example, the GICS 1 industry classification standard has evolvedand today includes an information technology and a communication servicessector. Keeping these analytical tools constant and ignoring the evolution of theeconomy, markets and investment processes would mean that railroads and oil,once the dominant industries, should still dominate GICS today.FOUNDATIONS OF MSCI MODELSThe foundations of fundamental characteristics based cross-sectional factormodels were laid in the seminal paper of Rosenberg (1974). This paper showsthat “ there are highly significant extra-market components of covarianceamong security returns; moreover, these risk components are such that theloadings of individual security returns on the factors are determined byobservable characteristics of the firm: income statement and balance sheet data,industry membership, and historical behavior of returns on the security. Theresults also show that the conventional security beta is a function of these samecharacteristics.”1GICS, the global industry classification standard jointly developed by MSCI and Standard & Poor’s. 2018 MSCI Inc. All rights reserved. Please refer to the disclaimer at the end of this document.MSCI.COM PAGE 6 OF 17
BEST PRACTICES IN FACTOR RESEARCH AND FACTOR MODELS NOVEMBER 2018The procedure for estimating factor returns through cross-sectional regression ofasset returns on asset characteristics was also described in the seminal paper ofFama and MacBeth (1973). In this paper, a cross-sectional regression is run everymonth, where portfolio excess returns are regressed against factor exposures(betas), choosing factor returns that minimize the weighted sum of squaredspecific returns. 2 This is precisely the same core procedure used in estimating allcross sectional characteristics based fundamental factor models that MSCIprovides.The interpretation of factor exposures and factor returns from cross-sectionalcharacteristics based factor models is simple and intuitive. In the same way thatthe classical Capital Asset Pricing Model beta measures how much a stock pricemay move with every percentage change in the market, the exposure to a factorin a cross sectional model measures how much a stock price could move witheach percentage change in a factor. For example, if the factor return is 10% in agiven period, a stock or portfolio with an exposure of 0.8 to that factor will havereturn of 8% attributed to this particular factor exposure.Grinold and Kahn (1994) provide evidence that supports the validity of crosssectional factor models. They report that monthly R2 statistics for the modelsaverage about 30%-40%, meaning that the model explains, on average, about30%-40% of the observed cross-sectional variance of the universe of stockreturns. Another measure of model performance is the root mean square errorfrom the regression. According to this measure, the authors report that crosssectional factor models explain 64% of individual stock variance. Because thegoal of the model is to explain portfolio risk, a better way to evaluate the modelis by the fraction of portfolio risk it explains. For benchmark portfolios in theUnited States, the authors report that the multiple-factor risk model explained98% of portfolio variance. MSCI conducts these and many other tests whenreleasing a new model and makes all results publicly available. For example, seeMorozov (2015) for detailed statistics evaluating the explanatory power andforecasting accuracy of the GEMLT model.2See equation 10 in Fama and MacBeth. 2018 MSCI Inc. All rights reserved. Please refer to the disclaimer at the end of this document.MSCI.COM PAGE 7 OF 17
BEST PRACTICES IN FACTOR RESEARCH AND FACTOR MODELS NOVEMBER 2018Connor (1995) describes the three main types of factor models and provides abalanced assessment of their relative benefits and drawbacks: Fundamental models assign factor exposures and estimate factor returnsthrough cross sectional regression Macroeconomic models assign factor returns and estimate factor exposuresthrough time series regression Statistical models estimate factor exposures and factor returns throughprincipal components analysisBroadly speaking, these three modelling approaches trade off specification errorand measurement error, with fundamental models more likely to be exposed tothe former and statistical models to the latter, while macroeconomic models areexposed to both types of error. In fact, Connor observes that “ the three typesof factor models are not necessarily inconsistent. In the absence of estimationerror and with no limits on data availability, the three models are simplyrestatements or (to use a technical term from factor modelling) rotations of oneanother. In this eclectic view of the world, the three factor model
Factors define the sources of portfolio risk and return. In this paper, we review the theoretical and empirical foundations of our factor research and factor models. MSCI factor research is firmly .