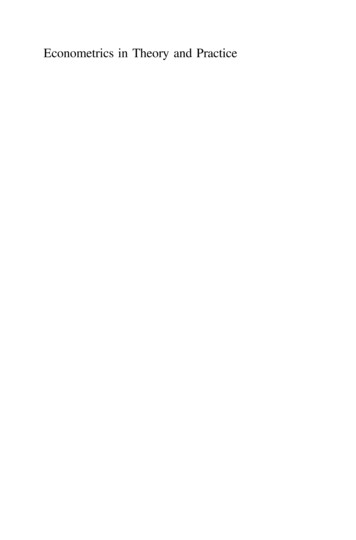
Transcription
Econometrics in Theory and Practice
Panchanan DasEconometrics in Theoryand PracticeAnalysis of Cross Section, Time Seriesand Panel Data with Stata 15.1123
Panchanan DasDepartment of EconomicsUniversity of CalcuttaKolkata, IndiaISBN 978-981-32-9018-1ISBN 2-9019-8(eBook) Springer Nature Singapore Pte Ltd. 2019This work is subject to copyright. All rights are reserved by the Publisher, whether the whole or partof the material is concerned, specifically the rights of translation, reprinting, reuse of illustrations,recitation, broadcasting, reproduction on microfilms or in any other physical way, and transmissionor information storage and retrieval, electronic adaptation, computer software, or by similar or dissimilarmethodology now known or hereafter developed.The use of general descriptive names, registered names, trademarks, service marks, etc. in thispublication does not imply, even in the absence of a specific statement, that such names are exempt fromthe relevant protective laws and regulations and therefore free for general use.The publisher, the authors and the editors are safe to assume that the advice and information in thisbook are believed to be true and accurate at the date of publication. Neither the publisher nor theauthors or the editors give a warranty, expressed or implied, with respect to the material containedherein or for any errors or omissions that may have been made. The publisher remains neutral with regardto jurisdictional claims in published maps and institutional affiliations.This Springer imprint is published by the registered company Springer Nature Singapore Pte Ltd.The registered company address is: 152 Beach Road, #21-01/04 Gateway East, Singapore 189721,Singapore
Dedicated to my father late Bibhuti BhusanDas
PrefaceThis book is an outcome of my experience in learning and teaching econometricssince more than three decades. Good quality books of econometrics are available,but there is a dearth of user-friendly books with a proper combination of theory andapplication with statistical software. The books particularly by Maddala,Wooldridge, Greene, Enders, Maddala and Kim, Hsiao and Baltagi are very muchinvaluable. The book by Gujarati is also a good one in its ability to elaborateeconometric theories for graduate students. However, many scholars and studentsand researchers, today, use statistical software to do empirical analysis. I also haveused both EViews and Stata in my teaching and research works and personallyfound that Stata is as powerful or flexible compared to EViews. Furthermore, Statais used extensively to process large data sets. This book is a proper combination ofeconometric theory and application with Stata 15.1.The basic purpose of this text is to introduce econometric analysis of crosssection, time series and panel data with the application of statistical software. Thisbook may serve as a basic text for those who wish to learn and apply econometricanalysis in empirical research. The level of presentation is kept as simple as possible to make it useful for undergraduate as well as graduate students. It containsseveral examples with real data and Stata programmes and interpretation of theresults.This book is intended primarily for graduate and post-graduate students inuniversities in India and abroad and researchers in the social sciences, business,management, operations research, engineering or applied mathematics. In this book,we view econometrics as a subject dealing with a set of data analytic techniques thatare used in empirical research extensively. The aim is to provide students with theskills required to undertake independent applied research using modern econometric methods. It covers the statistical tools needed to understand empirical economic research and to plan and execute independent research projects. It attempts toprovide a balance between theory and applied research. Various concepts andtechniques of econometric analysis are supported by carefully developed examplesvii
viiiPrefacewith the use of statistical software package, Stata 15.1. Hopefully, this book willsuccessfully bridge the gap between learning econometrics and learning how to useStata.It is an attempt to incorporate econometric theories in a student-friendly mannerto understand properly the techniques needed for empirical research. It demandsboth students and professional analysts because of its balanced discussion of thetheories with software applications. However, this book should not be claimed as asubstitute for the well-established texts that are being used in academia; rather it canserve as a supplementary text in both undergraduate- and post-graduate-leveleconometric courses. The discussion in this book is based on the assumption thatthe reader is somewhat familiar with the Stata software and other statistical programming. The Stata help manuals from the Stata Corporation offer detailedexplanation and syntax for all the commands used in this book. The data used forillustration are taken mainly from official sources like CSO, NSSO and ILO.The topics covered in this book are divided into four parts. Part I is the discussionon introductory econometric methods covering the syllabus of graduate courses inthe University of Calcutta, Delhi University and other leading universities in Indiaand abroad. This part of the book provides an introduction to basic econometricmethods for data analysis that economists and other social scientists use to estimatethe economic and social relationships, and to test hypotheses about them, usingreal-world data. There are 5 chapters in this part covering the data managementissues, details of linear regression models and the related problems due to the violation of the classical assumptions. Chapter 1 provides some basic steps used ineconometrics and statistical software, Stata 15.1, for useful application of econometric theories. Chapter 2 discusses linear regression model and its application withcross section data. Chapter 3 deals with this problem of statistical inference of a linearregression model. Chapter 4 relaxes the homoscedasticity and non-autocorrelationassumptions of the random error of a linear regression model and shows how theparameters of the linear model are correctly estimated. Chapter 5 discusses thedetection of multicollinearity and alternatives for handling the problem.Part II discusses some advanced topics used frequently in empirical researchwith cross section data. This part contains 3 chapters to include some specificproblems of regression analysis. Chapter 6 explains how qualitative explanatoryvariables can be incorporated into a linear model. Chapter 7 provides econometricmodels with limited dependent variables and problems of truncated distribution,sample selection bias and multinomial logit. Special emphasis is given to multivariate analysis, particularly principal component analysis and factor analysis,because of their popularity in empirical research with cross section data. Chapter 8captures these issues.Part III deals with time series econometric analysis. Time series data have somespecial features, and they should be taken care of very much cautiously. Time serieseconometrics was developed in modern approach since the early 1980s with thepublications of Engle and Granger, and it becomes very much popular in empiricalresearch with the development of user-friendly software. This book covers intensively both the univariate and multivariate time series econometric models and their
Prefaceixapplications with software programming in 6 chapters. This part starts with thediscussion on data generating process of time series data in Chap. 9. Chapter 10deals with different features of the data generating process (DGP) of a time series ina univariate framework. The presence of unit roots in macroeconomic time serieshas received a major area of theoretical and applied research since the early 1980s.Chapter 11 presents some issues regarding unit root tests and explores some of theimplications for macroeconomic theory and policy. Chapter 12 explores the basicconceptual issues involved in estimating the relationship between two or morenonstationary time series with unit roots. Chapter 13 examines the behaviour ofvolatility in terms of conditional heteroscedasticity model. Forecasting is importantin economics, commerce and various disciplines of social science and pure science.Chapter 14 aims to provide an overview of forecasting based on time seriesanalysis.Part IV takes care of panel data analysis in 4 chapters. Panel data have severaladvantages over the cross section and time series data. Panel data econometricsgains popularity because of the availability of panel data in the public domaintoday. Different aspects of fixed effects and random effects are discussed here.I have extended panel data analysis by taking dynamic panel data models which arethe most suitable for macroeconomic research. Chapter 15 discusses different typesof panel data model in a static framework. Chapter 16 deals with testing ofhypotheses to examine panel data in a static framework. Panel data with long timeperiod have been used predominately in applied macroeconomic research likepurchasing power parity, growth convergence, business cycle synchronisation andso on. Chapter 17 provides some theoretical issues and their application in testingfor unit roots in panel data. Dynamic model in panel data framework is very muchpopular in empirical research. Chapter 18 focuses on some issues of dynamic paneldata model.All chapters in this book provide applications of econometric models by usingStata. Simple presentation of some difficult topics in a rigorous manner is the majorstrength of this book. While the Bayesian econometrics, nonparametric and semiparametric, are popular methods today to capture the behaviour of the data in amore complex real situation, I do not attempt to cover these topics because of mycomparative disadvantage in these areas and to keep the technical difficulty at alower possible level. Despite these limitations, the topics covered in this book arebasics and necessary for econometrics training of every student in economics andother disciplines. I hope the students of econometrics will share my enthusiasm andoptimism in the importance of different econometric methods they will learnthrough reading this book. Hopefully, it will enhance their interest in empiricalresearch in economics and other fields of social science.Kolkata, IndiaMay 2019Panchanan Das
AcknowledgementsMy interest in econometrics was initiated by my teachers at different level sincemore than three decades back. I acknowledge the contribution of Amiya KumarBagchi, my teacher and Ph.D. supervisor, in the field of empirical research thatencourages me to learn econometrics at least indirectly. Among others I shouldmention Dipankor Coondoo of Indian Statistical Institute, Kolkata, who helped meto understand clearly different issues of the subject. Sankar Kumar Bhoumik, mysenior colleague and friend, helped a lot to learn the subject by providing access toteaching at post-graduate level at the Department of Economics, University ofCalcutta, even much before my joining the Department as a permanent faculty.I also gratefully acknowledge my teacher, Manoj Kumar Sanyal, who in fact is acontinuous source of encouragement in learning and thinking. I think, in some way,they have prepared the background for this book being written.A number of friends and colleagues have commented on earlier drafts of thebook, or helped in other ways. I am grateful to Maniklal Adhikary, AninditaSengupta, Pradip Kumar Biswas and others for their assistance and encouragement.Discussions with Oleg Golichenko and Kirdina Svetlana of Higher SchoolEconomics, Moscow, were helpful in clarifying some of my ideas.Any remaining errors and omissions are, of course, my responsibility, and I shallbe glad to have them brought to my attention.I am grateful to the Department of Economics, University of Calcutta, forproviding an adequate infrastructure where I spent time during my learning andteaching of economics. Special thanks are due to the Head of the Department ofEconomics and the authority of the University of Calcutta.I am extremely grateful to my wife, Krishna, who took over many of my roles inthe household during the preparation of the manuscripts.xi
xiiAcknowledgementsFinally, thanks to the editorial team of Springer for help with indexing andproof-reading. I am grateful to Sagarika Ghosh of Springer for encouragement for thisproject.Kolkata, IndiaMay 2019Panchanan Das
ContentsPart I1Introductory EconometricsIntroduction to Econometrics and Statistical Software . . . . . .1.1Introduction . . . . . . . . . . . . . . . . . . . . . . . . . . . . . . . . .1.2Economic Model and Econometric Model . . . . . . . . . . .1.3Population Regression Function and Sample RegressionFunction . . . . . . . . . . . . . . . . . . . . . . . . . . . . . . . . . . . .1.4Parametric and Nonparametric or Semiparametric Model .1.5Steps in Formulating an Econometric Model . . . . . . . . . .1.5.1Specification . . . . . . . . . . . . . . . . . . . . . . . . . .1.5.2Estimation . . . . . . . . . . . . . . . . . . . . . . . . . . .1.5.3Testing of Hypothesis . . . . . . . . . . . . . . . . . . .1.5.4Forecasting . . . . . . . . . . . . . . . . . . . . . . . . . . .1.6Data . . . . . . . . . . . . . . . . . . . . . . . . . . . . . . . . . . . . . . .1.6.1Cross Section Data . . . . . . . . . . . . . . . . . . . . .1.6.2Time Series Data . . . . . . . . . . . . . . . . . . . . . .1.6.3Pooled Cross Section . . . . . . . . . . . . . . . . . . .1.6.4Panel Data . . . . . . . . . . . . . . . . . . . . . . . . . . .1.7Use of Econometric Software: Stata 15.1 . . . . . . . . . . . .1.7.1Data Management . . . . . . . . . . . . . . . . . . . . . .1.7.2Generating Variables . . . . . . . . . . . . . . . . . . . .1.7.3Describing Data . . . . . . . . . . . . . . . . . . . . . . .1.7.4Graphs . . . . . . . . . . . . . . . . . . . . . . . . . . . . . .1.7.5Logical Operators in Stata . . . . . . . . . . . . . . . .1.7.6Functions Used in Stata . . . . . . . . . . . . . . . . .1.8Matrix Algebra . . . . . . . . . . . . . . . . . . . . . . . . . . . . . . .1.8.1Matrix and Vector: Basic Operations . . . . . . . .1.8.2Partitioned Matrices . . . . . . . . . . . . . . . . . . . .1.8.3Rank of a Matrix . . . . . . . . . . . . . . . . . . . . . .1.8.4Inverse Matrix . . . . . . . . . . . . . . . . . . . . . . . 0xiii
xivContents1.8.51.8.61.8.71.8.8References . . . .Positive Definite Matrix . . . . . . .Trace of a Matrix . . . . . . . . . . . .Orthogonal Vectors and Matrices .Eigenvalues and Eigenvectors . . .3131323235Regression Model: Properties and Estimation . . . . . .Introduction . . . . . . . . . . . . . . . . . . . . . . . . . . . . . . . .The Simple Linear Regression Model . . . . . . . . . . . . . .Multiple Linear Regression Model . . . . . . . . . . . . . . . .Assumptions of Linear Regression Model . . . . . . . . . . .2.4.1Non-stochastic Regressors . . . . . . . . . . . . . . .2.4.2Linearity . . . . . . . . . . . . . . . . . . . . . . . . . . .2.4.3Zero Unconditional Mean . . . . . . . . . . . . . . .2.4.4Exogeneity . . . . . . . . . . . . . . . . . . . . . . . . . .2.4.5Homoscedasticity . . . . . . . . . . . . . . . . . . . . .2.4.6Non-autocorrelation . . . . . . . . . . . . . . . . . . .2.4.7Full Rank . . . . . . . . . . . . . . . . . . . . . . . . . . .2.4.8Normal Distribution . . . . . . . . . . . . . . . . . . .2.5Methods of Estimation . . . . . . . . . . . . . . . . . . . . . . . .2.5.1The Method of Moments (MM) . . . . . . . . . . .2.5.2The Method of Ordinary Least Squares (OLS)2.5.3Maximum Likelihood Method . . . . . . . . . . . .2.6Properties of the OLS Estimation . . . . . . . . . . . . . . . . .2.6.1Algebraic Properties . . . . . . . . . . . . . . . . . . .2.6.2Statistical Properties . . . . . . . . . . . . . . . . . . .References . . . . . . . . . . . . . . . . . . . . . . . . . . . . . . . . . . . . . . 76.798082838989.909193.2Linear2.12.22.32.43Linear Regression Model: Goodness of Fit and Testingof Hypothesis . . . . . . . . . . . . . . . . . . . . . . . . . . . . . . . . . . . .3.1Introduction . . . . . . . . . . . . . . . . . . . . . . . . . . . . . . . .3.2Goodness of Fit . . . . . . . . . . . . . . . . . . . . . . . . . . . . .3.2.1The R2 as a Measure of Goodness of Fit . . . .3.2.2The Adjusted R2 as a Measure of Goodnessof Fit . . . . . . . . . . . . . . . . . . . . . . . . . . . . . .3.3Testing of Hypothesis . . . . . . . . . . . . . . . . . . . . . . . . .3.3.1Sampling Distributions of the OLS Estimators3.3.2Testing of Hypothesis for a Single Parameter .3.3.3Use of P-Value . . . . . . . . . . . . . . . . . . . . . . .3.3.4Interval Estimates . . . . . . . . . . . . . . . . . . . . .3.3.5Testing of Hypotheses for More Than OneParameter: t Test . . . . . . . . . . . . . . . . . . . . . .3.3.6Testing Significance of the Regression: F Test3.3.7Testing for Linearity . . . . . . . . . . . . . . . . . . .
Contentsxv3.3.83.3.93.3.10Tests for Stability . . . . . . . . . . . . . . . . . . . . . . .Analysis of Variance . . . . . . . . . . . . . . . . . . . . .The Likelihood-Ratio, Wald and LagrangeMultiplier Test . . . . . . . . . . . . . . . . . . . . . . . . .3.4Linear Regression Model by Using Stata 15.1 . . . . . . . . .3.4.1OLS Estimation in Stata . . . . . . . . . . . . . . . . . .3.4.2Maximum Likelihood Estimation (MLE) in StataReferences . . . . . . . . . . . . . . . . . . . . . . . . . . . . . . . . . . . . . . . . .45.9596.97101101104108Linear Regression Model: Relaxing the Classical Assumptions . .4.1Introduction . . . . . . . . . . . . . . . . . . . . . . . . . . . . . . . . . . .4.2Heteroscedasticity . . . . . . . . . . . . . . . . . . . . . . . . . . . . . . .4.2.1Problems with Heteroscedastic Data . . . . . . . . . . .4.2.2Heteroscedasticity Robust Variance . . . . . . . . . . .4.2.3Testing for Heteroscedasticity . . . . . . . . . . . . . . .4.2.4Problem of Estimation . . . . . . . . . . . . . . . . . . . .4.2.5Illustration of Heteroscedastic Linear Regressionby Using Stata . . . . . . . . . . . . . . . . . . . . . . . . . .4.3Autocorrelation . . . . . . . . . . . . . . . . . . . . . . . . . . . . . . . . .4.3.1Linear Regression Model with AutocorrelatedError . . . . . . . . . . . . . . . . . . . . . . . . . . . . . . . . .4.3.2Testing for Autocorrelation: Durbin–Watson Test .4.3.3Consequences of Autocorrelation . . . . . . . . . . . . .4.3.4Correcting for Autocorrelation . . . . . . . . . . . . . . .4.3.5Illustration by Using Stata . . . . . . . . . . . . . . . . . .References . . . . . . . . . . . . . . . . . . . . . . . . . . . . . . . . . . . . . . . . . .109109110110112115116.127128130131132135Analysis of Collinear Data: Multicollinearity . . . . . .5.1Introduction . . . . . . . . . . . . . . . . . . . . . . . . . .5.2Multiple Correlation and Partial Correlation . . .5.3Problems in the Presence of Multicollinearity . .5.4Detecting Multicollinearity . . . . . . . . . . . . . . . .5.4.1Determinant of (X′X) . . . . . . . . . . . . .5.4.2Determinant of Correlation Matrix . . .5.4.3Inspection of Correlation Matrix . . . .5.4.4Measure Based on Partial Regression .5.4.5Theil’s Measure . . . . . . . . . . . . . . . .5.4.6Variance Inflation Factor (VIF) . . . . .5.4.7Eigenvalues and Condition Numbers .5.5Dealing with Multicollinearity . . . . . . . . . . . . .5.6Illustration by Using Stata . . . . . . . . . . . . . . . .References . . . . . . . . . . . . . . . . . . . . . . . . . . . . . . . . .137137138140142143143143143144144146147149151. . 118. . 126
xviContentsPart IIAdvanced Analysis of Cross Section Data6Linear Regression Model: Qualitative Variables as Predictors6.1Introduction . . . . . . . . . . . . . . . . . . . . . . . . . . . . . . . . .6.2Regression Model with Intercept Dummy . . . . . . . . . . . .6.2.1Dichotomous Factor . . . . . . . . . . . . . . . . . . . .6.2.2Polytomous Factors . . . . . . . . . . . . . . . . . . . . .6.3Regression Model with Interaction Dummy . . . . . . . . . .6.4Illustration by Using Stata . . . . . . . . . . . . . . . . . . . . . . .1551551571571581601627Limited Dependent Variable Model . . . . . . . . . . . . . . . . . . . . .7.1Introduction . . . . . . . . . . . . . . . . . . . . . . . . . . . . . . . . . .7.2Linear Probability Model . . . . . . . . . . . . . . . . . . . . . . . . .7.3Binary Response Models: Logit and Probit . . . . . . . . . . . .7.3.1The Logit Model . . . . . . . . . . . . . . . . . . . . . . .7.3.2The Probit Model . . . . . . . . . . . . . . . . . . . . . . .7.3.3Difference Between Logit and Probit Models . . .7.4Maximum Likelihood Estimation of Logit and ProbitModels . . . . . . . . . . . . . . . . . . . . . . . . . . . . . . . . . . . . . .7.4.1Interpretation of the Estimated Coefficients . . . . .7.4.2Goodness of Fit . . . . . . . . . . . . . . . . . . . . . . . .7.4.3Testing of Hypotheses . . . . . . . . . . . . . . . . . . . .7.4.4Illustration of Binary Response Model by UsingStata . . . . . . . . . . . . . . . . . . . . . . . . . . . . . . . .7.5Regression Model with Truncated Distribution . . . . . . . . .7.5.1Illustration of Truncated Regression byUsing Stata . . . . . . . . . . . . . . . . . . . . . . . . . . . .7.6Problem of Censoring: Tobit Model . . . . . . . . . . . . . . . . .7.6.1Illustration of Tobit Model by Using Stata . . . . .7.7Models with Sample Selection Bias . . . . . . . . . . . . . . . . .7.7.1Illustration of Sample Selection Model by UsingStata . . . . . . . . . . . . . . . . . . . . . . . . . . . . . . . .7.8Multinomial Logit Regression . . . . . . . . . . . . . . . . . . . . .7.8.1Illustration by Using Stata . . . . . . . . . . . . . . . . .References . . . . . . . . . . . . . . . . . . . . . . . . . . . . . . . . . . . . . . . . 99201203206Multivariate Analysis . . . . . . . . . . . . . . .8.1Introduction . . . . . . . . . . . . . . . . .8.2Displaying Multivariate Data . . . . .8.2.1Multivariate Observations8.2.2Sample Mean Vector . . .8.2.3Population Mean Vector .8.2.4Covariance Matrix . . . . .2072072082082112112128. . . 180. . . 185
Contentsxvii8.2.5Correlation Matrix . . . . . . . . . . . . . . . . . .8.2.6Linear Combination of Variables . . . . . . . .8.3Multivariate Normal Distribution . . . . . . . . . . . . . . .8.4Principal Component Analysis . . . . . . . . . . . . . . . . .8.4.1Calculation of Principal Components . . . . .8.4.2Properties of Principal Components . . . . . .8.4.3Illustration by Using Stata . . . . . . . . . . . . .8.5Factor Analysis . . . . . . . . . . . . . . . . . . . . . . . . . . . .8.5.1Orthogonal Factor Model . . . . . . . . . . . . .8.5.2Estimation of Loadings and Communalities8.5.3Factor Loadings Are not Unique . . . . . . . .8.5.4Factor Rotation . . . . . . . . . . . . . . . . . . . . .8.5.5Illustration by Using Stata . . . . . . . . . . . . .8.6Multivariate Regression . . . . . . . . . . . . . . . . . . . . . .8.6.1Structure of the Regression Model . . . . . . .8.6.2Properties of Least Squares Estimators of B8.6.3Model Corrected for Means . . . . . . . . . . . .8.6.4Canonical Correlations . . . . . . . . . . . . . . .References . . . . . . . . . . . . . . . . . . . . . . . . . . . . . . . . . . . . 39239242.24724724825025225325425525810 Stationary Time Series . . . . . . . . . . . . . . . . . . . . . . . . . . . .10.1 Introduction . . . . . . . . . . . . . . . . . . . . . . . . . . . . . . .10.2 Univariate Time Series Model . . . . . . . . . . . . . . . . . .10.3 Autoregressive Process (AR) . . . . . . . . . . . . . . . . . . .10.3.1 The First-Order Autoregressive Process . . . .10.3.2 The Second-Order Autoregressive Process . .10.3.3 The Autoregressive Process of Order p . . . .10.3.4 General Linear Processes . . . . . . . . . . . . . . .10.4 The Moving Average (MA) Process . . . . . . . . . . . . . .10.4.1 The First-Order Moving Average Process . . .10.4.2 The Second-Order Moving Average Process .261262262264265269275276278278279Part III9Analysis of Time Series DataTime Series: Data Generating Process . . . . . .9.1Introduction . . . . . . . . . . . . . . . . . . . . .9.2Data Generating Process (DGP) . . . . . . .9.2.1Stationary Process . . . . . . . . . .9.2.2Nonstationary Process . . . . . . .9.3Methods of Time Series Analysis . . . . . .9.4Seasonality and Seasonal Adjustment . . .9.5Creating a Time Variable by Using StataReferences . . . . . . . . . . . . . . . . . . . . . . . . . . . .
xviiiContents10.4.3 The Moving Average Process of Order q . . .10.4.4 Invertibility in Moving Average Process . . .10.5 Autoregressive Moving Average (ARMA) Process . . .10.6 Autocorrelation Function . . . . . . . . . . . . . . . . . . . . . .10.6.1 Autocorrelation Function for AR(1) . . . . . . .10.6.2 Autocorrelation Function for AR(2) . . . . . . .10.6.3 Autocorrelation Function for AR(p) . . . . . . .10.6.4 Autocorrelation Function for MA(1) . . . . . .10.6.5 Autocorrelation Function for MA(2) . . . . . .10.6.6 Autocorrelation Function for MA(q) . . . . . .10.6.7 Autocorrelation Function for ARMA Process10.7 Partial Autocorrelation Function (PACF) . . . . . . . . . .10.7.1 Partial Autocorrelation for AR Series . . . . . .10.7.2 Partial Autocorrelation for MA Series . . . . .10.8 Sample Autocorrelation Function . . . . . . . . . . . . . . . .10.8.1 Illustration by Using Stata . . . . . . . . . . . . . .References . . . . . . . . . . . . . . . . . . . . . . . . . . . . . . . . . . . . . 0311 Nonstationarity, Unit Root and Structural Break . . . . . . . . . . . .11.1 Introduction . . . . . . . . . . . . . . . . . . . . . . . . . . . . . . . . . . .11.2 Analysis of Trend . . . . . . . . . . . . . . . . . . . . . . . . . . . . . . .11.2.1 Deterministic Function of Time . . . . . . . . . . . . . .11.2.2 Stochastic Function of Time . . . . . . . . . . . . . . . .11.2.3 Stochastic and Deterministic Function of Time . . .11.3 Concept of Unit Root . . . . . . . . . . . . . . . . . . . . . . . . . . . .11.4 Unit Root Test . . . . . . . . . . . . . . . . . . . . . . . . . . . . . . . . .11.4.1 Dickey–Fuller Unit Root Test . . . . . . . . . . . . . . .11.4.2 Augmented Dickey–Fuller (ADF) Unit Root Test .11.4.3 Phillips–Perron Unit Root Test . . . . . . . . . . . . . .11.4.4 Dickey–Fuller GLS Test . . . . . . . . . . . . . . . . . . .11.4.5 Stationarity Tests . . . . . . . . . . . . . . . . . . . . . . . .11.4.6 Multiple Unit Roots . . . . . . . . . . . . . . . . . . . . . .11.4.7 Some Problems with Unit Root Tests . . . . . . . . . .11.4.8 Macroeconomic Implications of Unit Root . . . . . .11.5 Testing for Structural Break . . . . . . . . . . . . . . . . . . . . . . . .11.5.1 Tests with Known Break Points . . . . . . . . . . . . . .11.5.2 Tests with Unknown Break Points . . . . . . . . . . . .11.6 Unit Root Test with Break . . . . . . . . . . . . . . . . . . . . . . . . .11.6.1 When Break Point is Exogenous . . . . . . . . . . . . .11.6.2 When Break Point is Endogenous . . . . . . . . . . . .11.7 Seasonal Adjustment . . . . . . . . . . . . . . . . . . . . . . . . . . . . 37337341349349354355.
Contentsxix11.7.1Unit Roots at Various Frequencies: Seasonal UnitRoot . . . . . . . . . . . . . . . . . . . . . . . . . . . . . . . . .11.7.2 Generating Time Variable and Seasonal Dummiesin Stata . . . . . . . . . . . . . . . . . . . . . . . . . . . . . . .11.8 Decomposition of a Time Series into Trend and Cycle . . . .References . . . . . . . . . . . . . . . . . . . . . . . . . . . . . . . . . . . . . . . . . .12 Cointegration, Error Correction and Vector Autoregression12.1 Introduction . . . . . . . . . . . . . . . . . . . . . . . . . . . . . . . .12.2 Regression with Trending Variables . . . . . . . . . . . . . . .12.3 Concept of Cointegration . . . . . . . . . . . . . . . . . . . . . . .12.4 Granger’s Representation Theorem . . . . . . . . . . . . . . . .12.5 Testing for Cointegration: Engle–Granger’s Two-StepMethod . . . . . . . . . . . . . . . . . . . . . . . . . . . . . . . . . . . .12.5.1 Illustrations by Using Stata . . . . . . . . . . . . . .12.6 Vector Autoregression (VAR) . . . . . . . . . . . . . . . . . . .12.6.1 Stationarity Restriction of a VAR Process . . .12.6.2 Autocovariance Matrix of a VAR Pro
issues, details of linear regression models and the related problems due to the vio-lation of the classical assumptions. Chapter 1 provides some basic steps used in econometrics and statistical software, Stata 15.1, for useful application of econo-metric theories. Chapter 2 d