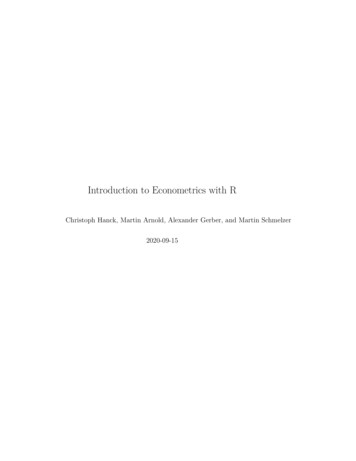
Transcription
Introduction to Econometrics with RChristoph Hanck, Martin Arnold, Alexander Gerber, and Martin Schmelzer2020-09-15
2
ContentsPreface91 Introduction111.1Colophon . . . . . . . . . . . . . . . . . . . . . . . . . . . . . . .131.2A Very Short Introduction to R and RStudio . . . . . . . . . . . .172 Probability Theory212.1Random Variables and Probability Distributions . . . . . . . . .212.2Random Sampling and the Distribution of Sample Averages . . .452.3Exercises59. . . . . . . . . . . . . . . . . . . . . . . . . . . . . . .3 A Review of Statistics using R613.1Estimation of the Population Mean . . . . . . . . . . . . . . . . .623.2Properties of the Sample Mean . . . . . . . . . . . . . . . . . . .653.3Hypothesis Tests Concerning the Population Mean . . . . . . . .723.4Confidence Intervals for the Population Mean . . . . . . . . . . .853.5Comparing Means from Different Populations . . . . . . . . . . .883.6An Application to the Gender Gap of Earnings . . . . . . . . . .893.7Scatterplots, Sample Covariance and Sample Correlation . . . . .923.8Exercises95. . . . . . . . . . . . . . . . . . . . . . . . . . . . . . .4 Linear Regression with One Regressor974.1Simple Linear Regression . . . . . . . . . . . . . . . . . . . . . .4.2Estimating the Coefficients of the Linear Regression Model . . . 100398
4CONTENTS4.3Measures of Fit . . . . . . . . . . . . . . . . . . . . . . . . . . . . 1074.4The Least Squares Assumptions . . . . . . . . . . . . . . . . . . . 1104.5The Sampling Distribution of the OLS Estimator . . . . . . . . . 1154.6Exercises. . . . . . . . . . . . . . . . . . . . . . . . . . . . . . . 1245 Hypothesis Tests and Confidence Intervals in the Simple LinearRegression Model1255.1Testing Two-Sided Hypotheses Concerning the Slope Coefficient . 1265.2Confidence Intervals for Regression Coefficients . . . . . . . . . . 1325.3Regression when X is a Binary Variable . . . . . . . . . . . . . . 1375.4Heteroskedasticity and Homoskedasticity . . . . . . . . . . . . . . 1405.5The Gauss-Markov Theorem5.6Using the t-Statistic in Regression When the Sample Size Is Small 1555.7Exercises. . . . . . . . . . . . . . . . . . . . 151. . . . . . . . . . . . . . . . . . . . . . . . . . . . . . . 1576 Regression Models with Multiple Regressors1596.1Omitted Variable Bias . . . . . . . . . . . . . . . . . . . . . . . . 1596.2The Multiple Regression Model . . . . . . . . . . . . . . . . . . . 1626.3Measures of Fit in Multiple Regression . . . . . . . . . . . . . . . 1656.4OLS Assumptions in Multiple Regression . . . . . . . . . . . . . 1676.5The Distribution of the OLS Estimators in Multiple Regression . 1786.6Exercises. . . . . . . . . . . . . . . . . . . . . . . . . . . . . . . 1807 Hypothesis Tests and Confidence Intervals in Multiple Regression1817.1Hypothesis Tests and Confidence Intervals for a Single Coefficient 1817.2An Application to Test Scores and the Student-Teacher Ratio . . 1837.3Joint Hypothesis Testing Using the F-Statistic . . . . . . . . . . 1867.4Confidence Sets for Multiple Coefficients . . . . . . . . . . . . . . 1897.5Model Specification for Multiple Regression . . . . . . . . . . . . 1907.6Analysis of the Test Score Data Set . . . . . . . . . . . . . . . . . 1957.7Exercises. . . . . . . . . . . . . . . . . . . . . . . . . . . . . . . 198
CONTENTS58 Nonlinear Regression Functions2018.1A General Strategy for Modelling Nonlinear Regression Functions 2018.2Nonlinear Functions of a Single Independent Variable8.3Interactions Between Independent Variables . . . . . . . . . . . . 2198.4Nonlinear Effects on Test Scores of the Student-Teacher Ratio . . 2378.5Exercises. . . . . . 205. . . . . . . . . . . . . . . . . . . . . . . . . . . . . . . 2469 Assessing Studies Based on Multiple Regression2479.1Internal and External Validity . . . . . . . . . . . . . . . . . . . . 2489.2Threats to Internal Validity of Multiple Regression Analysis . . . 2499.3Internal and External Validity when the Regression is Used forForecasting . . . . . . . . . . . . . . . . . . . . . . . . . . . . . . 2649.4Example: Test Scores and Class Size . . . . . . . . . . . . . . . . 2659.5Exercises. . . . . . . . . . . . . . . . . . . . . . . . . . . . . . . 27710 Regression with Panel Data27910.1 Panel Data . . . . . . . . . . . . . . . . . . . . . . . . . . . . . . 28010.2 Panel Data with Two Time Periods: “Before and After” Comparisons . . . . . . . . . . . . . . . . . . . . . . . . . . . . . . . . 28510.3 Fixed Effects Regression . . . . . . . . . . . . . . . . . . . . . . . 28710.4 Regression with Time Fixed Effects . . . . . . . . . . . . . . . . . 29110.5 The Fixed Effects Regression Assumptions and Standard Errorsfor Fixed Effects Regression . . . . . . . . . . . . . . . . . . . . . 29310.6 Drunk Driving Laws and Traffic Deaths . . . . . . . . . . . . . . 29610.7 Exercises. . . . . . . . . . . . . . . . . . . . . . . . . . . . . . . 30111 Regression with a Binary Dependent Variable30311.1 Binary Dependent Variables and the Linear Probability Model . 30411.2 Probit and Logit Regression . . . . . . . . . . . . . . . . . . . . . 30911.3 Estimation and Inference in the Logit and Probit Models . . . . 31711.4 Application to the Boston HMDA Data . . . . . . . . . . . . . . 32011.5 Exercises. . . . . . . . . . . . . . . . . . . . . . . . . . . . . . . 328
6CONTENTS12 Instrumental Variables Regression32912.1 The IV Estimator with a Single Regressor and a Single Instrument33012.2 The General IV Regression Model . . . . . . . . . . . . . . . . . 33512.3 Checking Instrument Validity . . . . . . . . . . . . . . . . . . . . 34112.4 Application to the Demand for Cigarettes . . . . . . . . . . . . . 34312.5 Where Do Valid Instruments Come From? . . . . . . . . . . . . . 35012.6 Exercises. . . . . . . . . . . . . . . . . . . . . . . . . . . . . . . 35013 Experiments and Quasi-Experiments35113.1 Potential Outcomes, Causal Effects and Idealized Experiments . 35213.2 Threats to Validity of Experiments . . . . . . . . . . . . . . . . . 35413.3 Experimental Estimates of the Effect of Class Size Reductions . . 35513.4 Quasi Experiments . . . . . . . . . . . . . . . . . . . . . . . . . . 36713.5 Exercises. . . . . . . . . . . . . . . . . . . . . . . . . . . . . . . 38014 Introduction to Time Series Regression and Forecasting38114.1 Using Regression Models for Forecasting . . . . . . . . . . . . . . 38214.2 Time Series Data and Serial Correlation . . . . . . . . . . . . . . 38314.3 Autoregressions . . . . . . . . . . . . . . . . . . . . . . . . . . . . 39114.4 Can You Beat the Market? (Part I) . . . . . . . . . . . . . . . . 39714.5 Additional Predictors and The ADL Model . . . . . . . . . . . . 39814.6 Lag Length Selection Using Information Criteria . . . . . . . . . 41014.7 Nonstationarity I: Trends . . . . . . . . . . . . . . . . . . . . . . 41314.8 Nonstationarity II: Breaks . . . . . . . . . . . . . . . . . . . . . . 42714.9 Can You Beat the Market? (Part II) . . . . . . . . . . . . . . . . 43515 Estimation of Dynamic Causal Effects44315.1 The Orange Juice Data . . . . . . . . . . . . . . . . . . . . . . . 44415.2 Dynamic Causal Effects . . . . . . . . . . . . . . . . . . . . . . . 44815.3 Dynamic Multipliers and Cumulative Dynamic Multipliers . . . . 45015.4 HAC Standard Errors . . . . . . . . . . . . . . . . . . . . . . . . 45115.5 Estimation of Dynamic Causal Effects with Strictly ExogeneousRegressors . . . . . . . . . . . . . . . . . . . . . . . . . . . . . . . 45415.6 Orange Juice Prices and Cold Weather . . . . . . . . . . . . . . . 461
CONTENTS16 Additional Topics in Time Series Regression747116.1 Vector Autoregressions . . . . . . . . . . . . . . . . . . . . . . . . 47216.2 Orders of Integration and the DF-GLS Unit Root Test . . . . . . 48216.3 Cointegration . . . . . . . . . . . . . . . . . . . . . . . . . . . . . 48616.4 Volatility Clustering and Autoregressive Conditional Heteroskedasticity . . . . . . . . . . . . . . . . . . . . . . . . . . . . 493
8CONTENTS
PrefaceChair of Econometrics Department of Business Administration and EconomicsUniversity of Duisburg-Essen Essen, Germany info@econometrics-with-r.orgLast updated on Tuesday, September 15, 2020Over the recent years, the statistical programming language R has become anintegral part of the curricula of econometrics classes we teach at the University ofDuisburg-Essen. We regularly found that a large share of the students, especiallyin our introductory undergraduate econometrics courses, have not been exposedto any programming language before and thus have difficulties to engage withlearning R on their own. With little background in statistics and econometrics, itis natural for beginners to have a hard time understanding the benefits of havingR skills for learning and applying econometrics. These particularly include theability to conduct, document and communicate empirical studies and having thefacilities to program simulation studies which is helpful for, e.g., comprehendingand validating theorems which usually are not easily grasped by mere broodingover formulas. Being applied economists and econometricians, all of the latterare capabilities we value and wish to share with our students.Instead of confronting students with pure coding exercises and complementaryclassic literature like the book by Venables and Smith (2010), we figured it wouldbe better to provide interactive learning material that blends R code with thecontents of the well-received textbook Introduction to Econometrics by Stockand Watson (2015) which serves as a basis for the lecture. This material isgathered in the present book Introduction to Econometrics with R, an empiricalcompanion to Stock and Watson (2015). It is an interactive script in the styleof a reproducible research report and enables students not only to learn howresults of case studies can be replicated with R but also strengthens their abilityin using the newly acquired skills in other empirical applications.Conventions Used in this Book Italic text indicates new terms, names, buttons and alike. Constant width text is generally used in paragraphs to refer to R code.9
10CONTENTSThis includes commands, variables, functions, data types, databases andfile names. Constant width text on gray background indicates R code that can betyped literally by you. It may appear in paragraphs for better distinguishability among executable and non-executable code statements butit will mostly be encountered in shape of large blocks of R code. Theseblocks are referred to as code chunks.AcknowledgementWe thank the Stifterverband für die Deutsche Wissenschaft e.V. and the Ministry of Culture and Science of North Rhine-Westphalia for their financial support. Also, we are grateful to Alexander Blasberg for proofreading and his effortin helping with programming the exercises. A special thanks goes to AchimZeileis (University of Innsbruck) and Christian Kleiber (University of Basel)for their advice and constructive criticism. Another thanks goes to RebeccaArnold from the Münster University of Applied Sciences for several suggestionsregarding the website design and for providing us with her nice designs for thebook cover, logos and icons. We are also indebted to all past students of ourintroductory econometrics courses at the University of Duisburg-Essen for theirfeedback.
Chapter 1IntroductionThe interest in the freely available statistical programming language and software environment R (R Core Team, 2020) is soaring. By the time we wrotefirst drafts for this project, more than 11000 add-ons (many of them providingcutting-edge methods) were made available on the Comprehensive R ArchiveNetwork (CRAN), an extensive network of FTP servers around the world thatstore identical and up-to-date versions of R code and its documentation. R dominates other (commercial) software for statistical computing in most fields ofresearch in applied statistics. The benefits of it being freely available, opensource and having a large and constantly growing community of users that contribute to CRAN render R more and more appealing for empirical economistsand econometricians alike.A striking advantage of using R in econometrics is that it enables students toexplicitly document their analysis step-by-step such that it is easy to update andto expand. This allows to re-use code for similar applications with different data.Furthermore, R programs are fully reproducible, which makes it straightforwardfor others to comprehend and validate results.Over the recent years, R has thus become an integral part of the curricula ofeconometrics classes we teach at the University of Duisburg-Essen. In somesense, learning to code is comparable to learning a foreign language and continuous practice is essential for the learning success. Needless to say, presentingbare R code on slides does not encourage the students to engage with hands-onexperience on their own. This is why R is crucial. As for accompanying literature, there are some excellent books that deal with R and its applications toeconometrics, e.g., Kleiber and Zeileis (2008). However, such sources may besomewhat beyond the scope of undergraduate students in economics having littleunderstanding of econometric methods and barely any experience in programming at all. Consequently, we started to compile a collection of reproduciblereports for use in class. These reports provide guidance on how to implementselected applications from the textbook Introduction to Econometrics (Stock11
12CHAPTER 1. INTRODUCTIONand Watson, 2015) which serves as a basis for the lecture and the accompanying tutorials. This process was facilitated considerably by knitr (Xie, 2020b)and R markdown (Allaire et al., 2020). In conjunction, both R packages providepowerful functionalities for dynamic report generation which allow to seamlesslycombine pure text, LaTeX, R code and its output in a variety of formats, including PDF and HTML. Moreover, writing and distributing reproducible reportsfor use in academia has been enriched tremendously by the bookdown package(Xie, 2020a) which has become our main tool for this project. bookdown buildson top of R markdown and allows to create appealing HTML pages like this one,among other things. Being inspired by Using R for Introductory Econometrics(Heiss, 2016)1 and with this powerful toolkit at hand we wrote up our own empirical companion to Stock and Watson (2015). The result, which you startedto look at, is Introduction to Econometrics with R.Similarly to the book by Heiss (2016), this project is neither a comprehensiveeconometrics textbook nor is it intended to be a general introduction to R. Wefeel that Stock and Watson do a great job at explaining the intuition and theoryof econometrics, and at any rate better than we could in yet another introductory textbook! Introduction to Econometrics with R is best described as aninteractive script in the style of a reproducible research report which aims toprovide students with a platform-independent e-learning arrangement by seamlessly intertwining theoretical core knowledge and empirical skills in undergraduate econometrics. Of course, the focus is on empirical applications with R. Weleave out derivations and proofs wherever we can. Our goal is to enable studentsnot only to learn how results of case studies can be replicated with R but wealso intend to strengthen their ability in using the newly acquired skills in otherempirical applications — immediately within Introduction to Econometrics withR.To realize this, each chapter contains interactive R programming exercises.These exercises are used as supplements to code chunks that display how previously discussed techniques can be implemented within R. They are generatedusing the DataCamp light widget and are backed by an R session which is maintained on DataCamp’s servers. You may play around with the example exercisepresented below.As you can see above, the widget consists of two tabs. script.R mimics an .Rfile, a file format that is commonly used for storing R code. Lines starting witha # are commented out, that is, they are not recognized as code. Furthermore,script.R works like an exercise sheet where you may write down the solutionyou come up with. If you hit the button Run, the code will be executed, submission correctness tests are run and you will be notified whether your approach iscorrect. If it is not correct, you will receive feedback suggesting improvementsor hints. The other tab, R Console, is a fully functional R console that can beused for trying out solutions to exercises before submitting them. Of course1 Heiss (2016) builds on the popular Introductory Econometrics (Wooldridge, 2016) anddemonstrates how to replicate the applications discussed therein using R.
1.1. COLOPHON13you may submit (almost any) R code and use the console to play around andexplore. Simply type a command and hit the Enter key on your keyboard.Looking at the widget above, you will notice that there is a in the right panel(in the console). This symbol is called “prompt” and indicates that the usercan enter code that will be executed. To avoid confusion, we will not show thissymbol in this book. Output produced by R code is commented out with # .Most commonly we display R code together with the generated output in codechunks. As an example, consider the following line of code presented in chunkbelow. It tells R to compute the number of packages available on CRAN. Thecode chunk is followed by the output produced.# check the number of R packages available on CRANnrow(available.packages(repos "http://cran.us.r-project.org"))# [1] 16272Each code chunk is equipped with a button on the outer right hand side whichcopies the code to your clipboard. This makes it convenient to work with largercode segments in your version of R/RStudio or in the widgets presented throughout the book. In the widget above, you may click on R Console and typenrow(available.packages(repos "http://cran.us.r-project.org"))(the command from the code chunk above) and execute it by hitting Enter onyour keyboard.2Note that some lines in the widget are out-commented which ask you to assigna numeric value to a variable and then to print the variable’s content to theconsole. You may enter your solution approach to script.R and hit the buttonRun in order to get the feedback described further above. In case you do notknow how to solve this sample exercise (don’t panic, that is probably why youare reading this), a click on Hint will provide you with some advice. If youstill can’t find a solution, a click on Solution will provide you with another tab,Solution.R which contains sample solution code. It will often be the case thatexercises can be solved in many different ways and Solution.R presents whatwe consider as comprehensible and idiomatic.1.1ColophonThis book was build with:# - Session info ------------# setting value# version R version 4.0.2 (2020-06-22)2 The R session is initialized by clicking into the widget. This might take a few seconds.Just wait for the indicator next to the button Run to turn green.
14CHAPTER 1. INTRODUCTION# osmacOS Catalina 10.15.4# systemx86 64, darwin19.5.0# uiunknown# language (EN)# collate en US.UTF-8# ctypeen US.UTF-8# tzEurope/Berlin# date2020-09-15# # - Packages ----------------# package* versiondatelib source# abind1.4-52016-07-21 [1] CRAN (R 4.0.2)# AER1.2-92020-02-06 [1] CRAN (R 4.0.0)# askpass1.12019-01-13 [1] CRAN (R 4.0.0)# assertthat0.2.12019-03-21 [1] CRAN (R 4.0.0)# backports1.1.82020-06-17 [1] CRAN (R 4.0.0)# base64enc0.1-32015-07-28 [1] CRAN (R 4.0.0)# bdsmatrix1.3-42020-01-13 [1] CRAN (R 4.0.0)# BH1.72.0-32020-01-08 [1] CRAN (R 4.0.2)# bibtex0.4.2.22020-01-02 [1] CRAN (R 4.0.0)# bitops1.0-62013-08-17 [1] CRAN (R 4.0.0)# blob1.2.12020-01-20 [1] CRAN (R 4.0.0)# bookdown0.202020-06-23 [1] CRAN (R 4.0.0)# boot1.3-252020-04-26 [2] CRAN (R 4.0.2)# broom0.7.02020-07-09 [1] CRAN (R 4.0.2)# callr3.4.32020-03-28 [1] CRAN (R 4.0.0)# car3.0-82020-05-21 [1] CRAN (R 4.0.0)# carData3.0-42020-05-22 [1] CRAN (R 4.0.0)# cellranger1.1.02016-07-27 [1] CRAN (R 4.0.0)# cli2.0.22020-02-28 [1] CRAN (R 4.0.0)# clipr0.7.02019-07-23 [1] CRAN (R 4.0.0)# colorspace1.4-12019-03-18 [1] CRAN (R 4.0.0)# conquer1.0.12020-05-06 [1] CRAN (R 4.0.2)# crayon1.3.42017-09-16 [1] CRAN (R 4.0.0)# cubature2.0.4.12020-07-06 [1] CRAN (R 4.0.2)# curl4.32019-12-02 [1] CRAN (R 4.0.0)# data.table1.12.82019-12-09 [1] CRAN (R 4.0.0)# DBI1.1.02019-12-15 [1] CRAN (R 4.0.0)# dbplyr1.4.42020-05-27 [1] CRAN (R 4.0.0)# desc1.2.02018-05-01 [1] CRAN (R 4.0.0)# digest0.6.252020-02-23 [1] CRAN (R 4.0.0)# dplyr1.0.02020-05-29 [1] CRAN (R 4.0.0)# dynlm0.3-62019-01-06 [1] CRAN (R 4.0.2)# ellipsis0.3.12020-05-15 [1] CRAN (R 4.0.0)# evaluate0.142019-05-28 [1] CRAN (R 4.0.0)# fansi0.4.12020-01-08 [1] CRAN (R 4.0.0)
1.1. COLOPHON# # # # # # # # # # # # # # # # # # # # # # # # # # # # # # # # # # # # # # # # # # # # # # ][1][1][1][2][2][1][1][1][2][1][1][1][1][1][1]CRAN (R 4.0.0)CRAN (R 4.0.2)CRAN (R 4.0.2)CRAN (R 4.0.2)CRAN (R 4.0.0)CRAN (R 4.0.2)CRAN (R 4.0.0)CRAN (R 4.0.2)CRAN (R 4.0.0)CRAN (R 4.0.0)CRAN (R 4.0.0)CRAN (R 4.0.0)CRAN (R 4.0.2)CRAN (R 4.0.0)CRAN (R 4.0.0)CRAN (R 4.0.0)CRAN (R 4.0.0)CRAN (R 4.0.0)CRAN (R 4.0.2)CRAN (R 4.0.0)Github (mca91/itewrpkg@bf5448c)CRAN (R 4.0.0)CRAN (R 4.0.2)CRAN (R 4.0.0)CRAN (R 4.0.0)CRAN (R 4.0.2)CRAN (R 4.0.0)CRAN (R 4.0.0)CRAN (R 4.0.2)CRAN (R 4.0.0)CRAN (R 4.0.0)CRAN (R 4.0.0)CRAN (R 4.0.0)CRAN (R 4.0.0)CRAN (R 4.0.2)CRAN (R 4.0.2)CRAN (R 4.0.0)CRAN (R 4.0.2)CRAN (R 4.0.0)CRAN (R 4.0.2)CRAN (R 4.0.0)CRAN (R 4.0.0)CRAN (R 4.0.0)CRAN (R 4.0.0)CRAN (R 4.0.0)CRAN (R 4.0.2)
16# # # # # # # # # # # # # # # # # # # # # # # # # # # # # # # # # # # # # # # # # # # # # # CHAPTER 1. 0.0)4.0.0)
1.2. A VERY SHORT INTRODUCTION TO R AND RSTUDIO# SparseM1.782019-12-13 [1] CRAN# spatial7.3-122020-04-26 [2] CRAN# stabledist0.7-12016-09-12 [1] CRAN# stargazer5.2.22018-05-30 [1] CRAN# statmod1.4.342020-02-17 [1] CRAN# stringi1.4.62020-02-17 [1] CRAN# stringr1.4.02019-02-10 [1] CRAN# strucchange1.5-22019-10-12 [1] CRAN# survival3.2-32020-06-13 [2] CRAN# sys3.42020-07-23 [1] CRAN# testthat2.3.22020-03-02 [1] CRAN# tibble3.0.32020-07-10 [1] CRAN# tidyr1.1.02020-05-20 [1] CRAN# tidyselect1.1.02020-05-11 [1] CRAN# tidyverse1.3.02019-11-21 [1] CRAN# timeDate3043.1022018-02-21 [1] CRAN# timeSeries3062.1002020-01-24 [1] CRAN# tinytex0.252020-07-24 [1] CRAN# TTR0.23-62019-12-15 [1] CRAN# urca1.3-02016-09-06 [1] CRAN# utf81.1.42018-05-24 [1] CRAN# vars1.5-32018-08-06 [1] CRAN# vctrs0.3.22020-07-15 [1] CRAN# viridisLite0.3.02018-02-01 [1] CRAN# whisker0.42019-08-28 [1] CRAN# withr2.2.02020-04-20 [1] CRAN# xfun0.162020-07-24 [1] CRAN# xml21.3.22020-04-23 [1] CRAN# xts0.12-02020-01-19 [1] CRAN# yaml2.2.12020-02-01 [1] CRAN# zip2.0.42019-09-01 [1] CRAN# zoo1.8-82020-05-02 [1] CRAN# # [1] /usr/local/lib/R/4.0/site-library# [2] /usr/local/Cellar/r/4.0.2 0)4.0.0)4.0.0)4.0.0)4.0.0)A Very Short Introduction to R and RStudioR BasicsAs mentioned before, this book is not intended to be an introduction to R buta guide on how to use its capabilities for applications commonly encountered inundergraduate econometrics. Those having basic knowledge in R programmingwill feel comfortable starting with Chapter 2. This section, however, is meant
18CHAPTER 1. INTRODUCTIONFigure 1.1: RStudio: the four panesfor those who have not worked with R or RStudio before. If you at least knowhow to create objects and call functions, you can skip it. If you would like torefresh your skills or get a feeling for how to work with RStudio, keep reading.First of all, start RStudio and open a new R script by selecting File, New File,R Script. In the editor pane, type1 1and click on the button labeled Run in the top right corner of the editor. Bydoing so, your line of code is sent to the console and the result of this operationshould be displayed right underneath it. As you can see, R works just like acalculator. You can do all arithmetic calculations by using the correspondingoperator ( , -, *, / or ). If you are not sure what the last operator does, tryit out and check the results.VectorsR is of course more sophisticated than that. We can work with variables or,more generally, objects. Objects are defined by using the assignment operator -. To create a variable named x which contains the value 10 type x - 10and click the button Run yet again. The new variable should have appeared inthe environment pane on the top right. The console however did not show anyresults, because our line of code did not contain any call that creates output.When you now type x in the console and hit return, you ask R to show you thevalue of x and the corresponding value should be printed in the console.x is a scalar, a vector of length 1. You can easily create longer vectors by usingthe function c() (c is for “concatenate” or “combine”). To create a vector ycontaining the numbers 1 to 5 and print it, do the following.
1.2. A VERY SHORT INTRODUCTION TO R AND RSTUDIO19y - c(1, 2, 3, 4, 5)y# [1] 1 2 3 4 5You can also create a vector of letters or words. For now just remember thatcharacters have to be surrounded by quotes, else they will be parsed as objectnames.hello - c("Hello", "World")Here we have created a vector of length 2 containing the words Hello and World.Do not forget to save your script! To do so, select File, Save.FunctionsYou have seen the function c() that can be used to combine objects. In general,all function calls look the same: a function name is always followed by roundparentheses. Sometimes, the parentheses include arguments.Here are two simple examples.# generate the vector z z - seq(from 1, to 5, by 1)# compute the mean of the enries in z mean(z)# [1] 3In the first line we use a function called seq() to create the exact same vectoras we did in the previous section, calling it z. The function takes on the arguments from, to and by which should be self-explanatory. The function mean()computes the arithmetic mean of its argument x. Since we pass the vector z asthe argument x, the result is 3!If you are not sure which arguments a function expects, you may consult thefunction’s documentation. Let’s say we are not sure how the arguments requiredfor seq() work. We then type ?seq in the console. By hitting return thedocumentation page for that function pops up in the lower right pane of RStudio.In there, the section Arguments holds the information we seek. On the bottomof almost every help page you find examples on how to use the correspondingfunctions. This is very helpful for beginners and we recommend to look out forthose.Of course, all of the commands presented above also work in interactive widgetsthrou
ature, there are some excellent books that deal with Rand its applications to econometrics, e.g., Kleiber and Zeileis (2008). However, such sources may be . ing PDF and HTML. Moreover, writing and distributing reproducible reports .