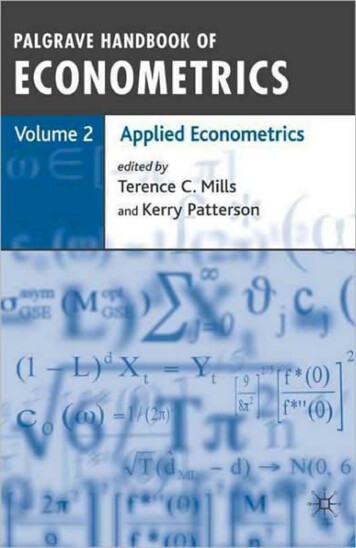
Transcription
Palgrave Handbook ofEconometricsVolume 2: Applied EconometricsEdited ByTerence C. MillsandKerry Patterson2009
ContentsNotes on ContributorsviiiEditors’ IntroductionxiPart I The Methodology and Philosophy of Applied Econometrics123The Methodology of Empirical Econometric Modeling: AppliedEconometrics Through the Looking GlassDavid F. Hendry, Nuffield College, Oxford UniversityHow Much Structure in Empirical Models?Fabio Canova, Universitat Pompeu FabraIntroductory Remarks on Metastatistics for the Practically MindedNon-Bayesian Regression RunnerJohn DiNardo, University of Michigan36898Part II Forecasting45Forecast Combination and EncompassingMichael P. Clements, Warwick University, and David I. Harvey,School of Economics, University of NottinghamRecent Developments in Density ForecastingStephen G. Hall, University of Leicester, and James Mitchell,National Institute of Economic and Social Research169199Part III Time Series Applications678910Investigating Economic Trends and CyclesD.S.G. Pollock, University of LeicesterEconomic Cycles: Asymmetries, Persistence, and SynchronizationJoe Cardinale, Air Products and Chemicals, Inc., and Larry W. Taylor,College of Business and Economics, Lehigh UniversityThe Long Swings Puzzle: What the Data Tell When Allowed toSpeak FreelyKatarina Juselius, University of CopenhagenStructural Time Series Models for Business Cycle AnalysisTommaso Proietti, University of Rome ‘Tor Vergata’Fractional Integration and Cointegration: An Overview and anEmpirical ApplicationLuis A. Gil-Alana and Javier Hualde, Universidad de Navarrav243308349385434
viContentsPart IV111213Discrete Choice ModelingWilliam Greene, Stern School of Business, New York UniversityPanel Data Methods and Applications to Health EconomicsAndrew M. Jones, University of YorkPanel Methods to Test for Unit Roots and CointegrationAnindya Banerjee, University of Birmingham, and Martin Wagner,Institute forAdvanced Studies, ViennaPart V1415Cross-section and Panel Data trics: Current Methods and Some Recent DevelopmentsA. Colin Cameron, University of California, DavisComputational Considerations in Empirical Microeconometrics:Selected ExamplesDavid T. Jacho-Chávez and Pravin K. Trivedi, Indiana University729775Part VI Applications of Econometrics to Economic Policy161718The Econometrics of Monetary Policy: An OverviewCarlo Favero, IGIER-Bocconi UniversityMacroeconometric Modeling for PolicyGunnar Bårdsen, Norwegian University of Science and Technology,and Ragnar Nymoen, University of OsloMonetary Policy, Beliefs, Unemployment and Inflation: Evidencefrom the UKS.G.B. Henry, National Institute of Economic and Social Research821851917Part VII Applications to Financial Econometrics19202122Estimation of Continuous-Time Stochastic Volatility ModelsGeorge Dotsis, Essex Business School, University of Essex,Raphael N. Markellos, Athens University of Economics and Business,and Terence C. Mills, Loughborough UniversityTesting the Martingale HypothesisJ. Carlos Escanciano, Indiana University, and Ignacio N. Lobato,Instituto Tecnológico Autónomo de MexicoAutoregressive Conditional Duration ModelsRuey S. Tsay, Booth Business School, University of ChicagoThe Econometrics of Exchange RatesEfthymios G. Pavlidis, Ivan Paya, and David A. Peel,Lancaster University Management School95197210041025
ContentsviiPart VIII Growth Development Econometrics232425The Econometrics of ConvergenceSteven N. Durlauf, University of Wisconsin-Madison,Paul A. Johnson, Vassar College, New York State, andJonathan R.W. Temple, Bristol UniversityThe Methods of Growth EconometricsSteven N. Durlauf, University of Wisconsin-Madison,Paul A. Johnson, Vassar College, New York State, andJonathan R.W. Temple, Bristol UniversityThe Econometrics of Finance and GrowthThorsten Beck, European Banking Center, Tilburg University, and CEPRPart IX2627282911191180Spatial EconometricsSpatial Hedonic ModelsLuc Anselin, School of Geographical Sciences and Urban Planning,and Nancy Lozano-Gracia, GeoDa Center for GeospatialAnalysis and Computation, Arizona State UniversitySpatial Analysis of Economic ConvergenceSergio J. Rey, Arizona State University, and Julie Le Gallo,Université de Franche-ComtéPart X108712131251Applied Econometrics and ComputingTesting Econometric SoftwareB.D. McCullough, Drexel UniversityTrends in Applied Econometrics Software Development 1985–2008:An Analysis of Journal of Applied Econometrics Research Articles,Software Reviews, Data and CodeMarius Ooms, VU University Amsterdam12931321Author Index1349Subject Index1374
Notes on ContributorsLuc Anselin is Foundation Professor of Geographical Sciences and Directorof the School of Geographical Sciences and Urban Planning at Arizona StateUniversity, USA.Aninyda BanerjeeBirmingham, r Bårdsen is Professor of Economics at the Norwegian University of Scienceand Technology, Norway.Thorsten Beck is Professor of Economics and Chair at the European BankingCenter, Tilburg University and Research Fellow, CEPR.Colin Cameron is Professor of Economics at the University of California,Davis, USA.Fabio Canova is ICREA Research Professor in Social Science at Universitat PompeuFabra, Barcelona, Spain.Joe Cardinale is a Manager, Economics at Air Products and Chemicals, Inc., USA.Michael P. Clements is Professor of Economics at Warwick University, UK.John DiNardo is Professor of Economics and Public Policy at the University ofMichigan, Ann Arbor, USA.George Dotsis is Lecturer in Finance at the Essex Business School, University ofEssex, UK.Steven N. Durlauf is Professor of Economics at the University of WisconsinMadison, USA.Juan Carlos Escanciano is Assistant Professor of Economics at Indiana University,Bloomington, USA.Carlo A. Favero is Professor of Economics at IGIER-Bocconi University, Italy.Julie Le Gallo is Professor of Economics and Econometrics at the Université deFranche-Comté, France.Luis A. Gil-Alana is Professor of Econometrics at the University of Navarra, Spain.William Greene is Professor of Economics at the Stern School of Business,New York, USA.Stephen G. Hall is Professor of Economics at University of Leicester, UK.David I. Harvey is Reader in Econometrics at the School of Economics, Universityof Nottingham, UK.David F. Hendry is Professor of Economics and Fellow, Nuffield College, OxfordUniversity, UK.viii
Notes on ContributorsixBrian Henry is Visiting Fellow at the National Institute of Economic and SocialResearch, NIESR, UK.Javier Hualde is Ramon y Cajal Research Fellow in Economics at the PublicUniversity of Navarra, Spain.David Jacho-Chávez is Assistant Professor of Economics at Indiana University,Bloomington, USA.Paul A. Johnson is Professor of Economics at Vassar College, New York State, USA.Andrew M. Jones is Professor of Economics at the University of York, UK.Katarina Juselius is Professor of Empirical Time Series Econometrics at theUniversity of Copenhagen, Denmark.Ignacio N. Lobato is Professor of Econometrics at the Instituto TecnológicoAutónomo de México, Mexico.Nancy Lozano-Gracia is Postdoctoral Research Associate in the GeoDa Center forGeospatial Analysis and Computation at Arizona State University, USA.Raphael N. Markellos is Assistant Professor of Quantitative Finance at the AthensUniversity of Economics and Business (AUEB), Greece.Bruce D. McCullough is Professor of Decision Sciences and Economics at DrexelUniversity, Philadelphia, USA.Terence C. Mills is Professor of Applied Statistics and Econometrics atLoughborough University, UK.James Mitchell is Research Fellow at the National Institute of Economic and SocialResearch, UK.Ragnar Nymoen is Professor of Economics at University of Oslo, Norway.Marius Ooms is Associate Professor of Econometrics at the VU University,Amsterdam, The Netherlands.Kerry Patterson is Professor of Econometrics at the University of Reading, UK.Efthymios G. Pavlidis is Lecturer in Economics at the Lancaster UniversityManagement School, Lancaster University, UK.Ivan Paya is Senior Lecturer in Economics at the Lancaster University ManagementSchool, Lancaster University, UK.David A. Peel is Professor in Economics at the Lancaster University ManagementSchool, Lancaster University, UK.D. Stephen G. Pollock is Professor of Economics at the University of Leicester, UK.Tommaso Proietti is Professor of Economic Statistics at the University of Rome‘Tor Vergata’, Italy.Sergio Rey is Professor of Geographical Sciences at Arizona State University, USA.Larry W. Taylor is Professor of Economics at the College of Business andEconomics, Lehigh University, Pennsylvania, USA.
x Notes on ContributorsJonathan R.W. Temple is Professor of Economics at Bristol University, UK.Pravin Trivedi is Professor of Economics at Indiana University, Bloomington, USA.Ruey S. Tsay is Professor of Econometrics and Statistics at the University of ChicagoBooth School of Business, USA.Martin Wagner is Senior Economist at the Institute for Advanced Studies inVienna, Austria.
Editors’ IntroductionTerence C. Mills and Kerry PattersonThe Palgrave Handbook of Econometrics was conceived to provide an understanding of major developments in econometrics, both in theory and in application.Over the last twenty-five years or so, econometrics has grown in a way that fewcould have contemplated, and it became clear to us, as to others, that no single person could have command either of the range of technical knowledge thatunderpins theoretical econometric developments or the extent of the applicationof econometrics. In short, econometrics is not, as it used to be considered, a set oftechniques that is applied to a previously well-defined problem in economics; it isnot a matter of finding the “best” estimator from a field of candidates, applyingthat estimator and reporting the results. The development of economics is nowinextricably entwined with the development of econometrics.The first Nobel Prize in Economics was awarded to Ragnar Frisch and Jan Tinbergen, both of whom made significant contributions to what we now recognize asapplied econometrics. More recently, Nobel Prizes in Economics have been awardedto Clive Granger, Robert Engle, James Heckman and Daniel McFadden, who haveall made major contributions to applied econometrics. It is thus clear that the discipline has recognized the influential role of econometrics, both theoretical andapplied, in advancing economic knowledge.The aim of this volume is to make major developments in applied econometrics accessible to those outside their particular field of specialization. The responseto Volume 1 was universally encouraging and it has become clear that we werefortunate to be able to provide a source of reference for others for many years tocome. We hope that this high standard is continued and achieved here. Typically,applied econometrics, unlike theoretical econometrics, has always been ratherpoorly served for textbooks, making it difficult for both undergraduate and postgraduate students to get a real “feel” for how econometrics is actually done. Tosome degree, the econometric textbook market has responded, so that now theleading textbooks include many examples; even so, these examples typically areof an illustrative nature, focusing on simple points, simply exposited, rather thanon the complexity that is revealed in practice. Thus our hope is that this volume will provide a genuine entry into the detailed considerations that have to bexi
xii Editors’ Introductionmade when combining economics and econometrics in order to carry out seriousempirical research.As in the case of Volume 1, the chapters here have been specially commissionedfrom acknowledged experts in their fields; further, each of the chapters has beenreviewed by the editors, one or more of the associate editors and a referee. Thus,the process is akin to submission to a journal; however, whilst ensuring the higheststandards in the evaluation process, the chapters have been conceived of as partof a whole rather than as a set of unrelated contributions. It has not, however,been our intention to provide just a series of surveys or overviews of some areas ofapplied econometrics, although the survey element is directly or indirectly servedin part here. By its very nature, this volume is about econometrics as it is appliedand, to succeed in its aim, the contributions, conceived as a whole, have to meetthis goal.We have organized the chapters of this volume of the Handbook into ten parts.The parts are certainly not watertight, but serve as a useful initial organization ofthe central themes. Part I contains three chapters under the general heading of“The Methodology and Philosophy of Applied Econometrics.” The lead chapter isby David Hendry, who has been making path-breaking contributions in theoreticaland applied econometrics for some forty years or so. It is difficult to conceive howeconometrics would have developed without David’s many contributions. Thischapter first places the role of applied econometrics in an historical context andthen develops a theory of applied econometrics. As might be expected, the keyissues are confronted head-on.In introducing the first volume we noted that the “growth in econometrics is tobe welcomed, for it indicates the vitality and importance of the subject. Indeed,this growth and, arguably, the dominance over the last ten or twenty years ofeconometric developments in taking economics forward, is a notable change fromthe situation faced by the subject some twenty-five years or so ago.” Yet in Chapter1, Hendry notes that, next to data measurement, collection and preparation, on theone hand, and teaching, on the other, “Applied Econometrics” does not have a highcredibility in the profession. Indeed, whilst courses in theoretical econometrics oreconometric techniques are de rigueur for a good undergraduate or Masters degree,courses in applied econometrics have no such general status.The intricacies, possibly even alchemy (Hendry, 1980), surrounding the mix oftechniques and data seem to defy systematization; perhaps they should be keptout of the gaze of querulous students, who may – indeed should – be satisfied withillustrative examples! Often to an undergraduate or Masters student undertaking aproject, applied econometrics is the application of econometrics to data, no more,no less, with some relief if the results are at all plausible. Yet, in contrast, leadingjournals, for example, the Journal of Econometrics, the Journal of Applied Econometrics and the Journal of Business and Economic Statistics, and leading topic journals,such as the Journal of Monetary Economics, all publish applied econometric articleshaving substance and longevity in their impact and which serve to change thedirection of the development of econometric theory (for a famous example, seeNelson and Plosser, 1982). To some, applying econometrics seems unsystematic
Terence C. Mills and Kerry Pattersonxiiiand so empirical results are open to question; however, as Hendry shows, it ispossible to formalize a theory of applied econometrics which provides a coherent basis for empirical work. Chapter 1 is a masterful and accessible synthesis andextension of Hendry’s previous ideas and is likely to become compulsory readingfor courses in econometrics, both theory and applied; moreover, it is completed bytwo applications using the Autometrics software (Doornik, 2007). The first application extends the work of Magnus and Morgan (1999) on US food expenditure,which was itself an update of a key study by Tobin (1950) estimating a demandfunction for food. This application shows the Autometrics algorithm at work in asimple context. The second application extends the context to a multiple equationsetting relating industrial output, the number of bankruptcies and patents, and realequity prices. These examples illustrate the previously outlined theory of appliedeconometrics combined with the power of the Autometrics software.In Chapter 2, Fabio Canova addresses the question of how much structure thereshould be in empirical models. This has long been a key issue in econometrics, andsome old questions, particularly those of identification and the meaning of structure, resurface here in a modern context. The last twenty years or so have seentwo key developments in macroeconometrics. One has been the development ofdynamic stochastic general equilibrium (DSGE) models. Initially, such models werecalibrated rather than estimated, with the emphasis on “strong” theory in theirspecification; however, as Canova documents, more recently likelihood-based estimation has become the dominant practice. The other key development has beenthat of extending the (simple) vector autoregression (VAR) to the structural VAR(SVAR) model. Although both approaches involve some structure, DSGE models,under the presumption that the model is correct, rely more on an underlying theory than do SVARs. So which should be used to analyze a particular set of problems?As Canova notes: “When addressing an empirical problem with a finite amount ofdata, one has . . . to take a stand on how much theory one wants to use to structurethe available data prior to estimation.” Canova takes the reader through the advantages and shortcomings of these methodologies; he provides guidance on what todo, and what not to do, and outlines a methodology that combines elements ofboth approaches.In Chapter 3, John DiNardo addresses some philosophical issues that are atthe heart of statistics and econometrics, but which rarely surface in econometric textbooks. As econometricians, we are, for example, used to working withina probabilistic framework, but we rarely consider issues related to what probability actually is. To some degree, we have been prepared to accept the axiomaticor measure theoretic approach to probability, due to Kolgomorov, and this hasprovided us with a consistent framework that most are happy to work within.Nevertheless, there is one well-known exception to this unanimity: when it comesto the assignment and interpretation of probability measures and, in particular, theinterpretation of some key conditional probabilities; this is whether one adopts aBayesian or non-Bayesian perspective. In part, the debate that DiNardo discussesrelates to the role of the Bayesian approach, but it is more than this; it concernsmetastatistics and philosophy, because, in a sense, it relates to a discussion of the
xiv Editors’ Introductiontheory of theories. This chapter is deliberately thought-provoking and certainlycontroversial – two characteristics that we wish to encourage in a Handbook thataims to be more than just an overview. For balance, the reader can consult Volume1 of the Handbook, which contains two chapters devoted to the Bayesian analysis ofeconometric models (see Poirier and Tobias, 2006, and Strachan et al., 2006). Thereader is likely to find familiar concepts here, such as probability and testing, butonly as part of a development that takes them into potentially unfamiliar areas.DiNardo’s discussion of these issues is wide-ranging, with illustrations taken fromgambling and practical examples taken as much from science, especially medicine,as economics. One example from the latter is the much-researched question of thecausal effect of union status on wages: put simply, do unions raise wages and, if so,by how much? This example serves as an effective setting in which to raise issuesand to show that differences in approach can lead to differences in results.For some, the proof of the pudding in econometrics is the ability to forecastaccurately, and to address some key issues concerning this aspect of econometrics Part II contains two chapters on forecasting. The first, Chapter 4, by MichaelClements and David Harvey, recognizes that quite often several forecasts are available and, rather than considering a selection strategy that removes all but the beston some criterion, it is often more fruitful to consider different ways of combiningforecasts, as suggested in the seminal paper by Bates and Granger (1969). In anintuitive sense, one forecast may be better than another, but there could still besome information in the less accurate forecast that is not contained in the moreaccurate forecast. This is a principle that is finding wider application; for example,in some circumstances, as in unit root testing, there is more than one test availableand, indeed, there may be one uniformly powerful test, yet there is still potentialmerit in combining tests.In the forecasting context, Clements and Harvey argue that the focus for multiple forecasts should not be on testing the null of equal accuracy, but on testingfor encompassing. Thus it is not a question of choosing forecast A over forecast B,but of whether the combination of forecasts A and B is better than either individual forecast. Of course, this may be of little comfort from a structuralist point ofview if, for example, the two forecasts come from different underlying models; butit is preferable when the loss function rewards good fit in some sense. Bates andGranger (1969) suggested a simple linear combination of two unbiased forecasts,with weights depending on the relative accuracy of the individual forecasts, andderived the classic result that, even if the forecasts are equally accurate in a meansquared error loss sense, then there will still be a gain in using the linear combination unless the forecasts are perfectly correlated, at least theoretically. Clements andHarvey develop from this base model, covering such issues as biased forecasts, nonlinear combinations, and density or distribution forecasts. The concept of forecastencompassing, which is not unique in practice, is then considered in detail, including complications arising from integrated variables, non-normal errors, seriallycorrelated forecast errors, ARCH errors, the uncertainty implied by model estimation, and the difficulty of achieving tests with the correct actual size. A number ofrecent developments are examined, including the concept of conditional forecast
Terence C. Mills and Kerry Pattersonxvevaluation (Giacomini and White, 2006), evaluating quantile forecasts, and relaxing the forecast loss function away from the traditional symmetric squared error.In short, this chapter provides a clear, critical and accessible evaluation of a rapidlydeveloping area of the econometrics literature.Chapter 5 is by Stephen Hall and James Mitchell, who focus on density forecasting. There has been a great deal of policy interest in forecasting key macroeconomicvariables such as output growth and inflation, some of which has been institutionally enshrined by granting central banks independence in inflation targeting. Inturn, there has been a movement away from simply reporting point forecasts ofinflation and GDP growth in favor of a fan chart representation of the distributionof forecasts. A density forecast gives much more information than a simple pointforecast, which is included as just one realization on the outcome axis. As a corollary, forecast evaluation should also include techniques that evaluate the accuracy,in some well-defined sense, of the density forecast. However, given that generallywe will only be able to observe one outcome (or event) per period, some thoughtneeds to be given to how the distributional aspect of the forecast is evaluated. Halland Mitchell discuss a number of possibilities and also consider methods of evaluating competing density forecasts. A further aspect of density forecasting is theability of the underlying model to generate time variation in the forecast densities. If the underlying model is a VAR, or can be approximated by a VAR, then,subject to some qualifications, the only aspect of the forecast density which is ableto exhibit time variation is the mean; consequently, models have been developedthat allow more general time variation in the density through, for example, ARCHand GARCH errors and time-varying parameters. This chapter also links in with theprevious chapter by considering combinations of density forecasts. There are twocentral possibilities: the linear opinion pool is a weighted linear combination ofthe component densities, whereas the logarithmic opinion pool is a multiplicativecombination. Hall and Mitchell consider the problem of determining the weightsin such combinations and suggest that predictive accuracy improves when theweights reflect shifts in volatility, a characteristic of note for the last decade or soin a number of economies.Part III contains four chapters under the general heading of “Time Series Applications.” A key area in which the concept of a time series is relevant is incharacterizing and determining trends and cycles. Chapter 6, by Stephen Pollock,is a tour de force on modeling trends and cycles, and on the possibilities andpitfalls inherent in the different approaches. In the simplest of models, cyclicalfluctuations are purely sinusoidal and the trend is exponential; although simple,this is a good base from which to understand the nature of developments thatrelax these specifications. Such developments include the view that economic timeseries evolve through the accumulation of stochastic shocks, as in an integratedWeiner process. The special and familiar cases of the Beveridge–Nelson decomposition, the Hodrick–Prescott filter, the Butterworth filter and the unifying place ofWeiner–Kolgomorov filtering are all covered with admirable clarity. Other considerations include the complications caused by the limited data that is often availablein economic applications, contrary to the convenient assumptions of theory. In an
xviEditors’ Introductionappealing turn of phrase, Pollock refers to obtaining a decomposition of components based on the periodogram “where components often reside within strictlylimited frequency bands which are separated by dead spaces where the spectralordinates are virtually zeros.” The existence of these “spectral dead spaces” is keyto a practical decomposition of an economic time series, however achieved. Inpractice, trend fitting requires judgment and a clear sense of what it is that thetrend is capturing. Other critical issues covered in this chapter include the importance of structural breaks, a topic that has been influential elsewhere (for example,in questioning the results of unit root testing: Perron, 1989); and to aid the reader,practical examples are included throughout the exposition.Chapter 7, by Joe Cardinale and Larry Taylor, continues the time series theme ofanalyzing economic cycles whilst focusing on asymmetries, persistence and synchronization. This is a particularly timely and somewhat prophetic chapter giventhat we are currently experiencing what is perhaps the deepest recession in recenteconomic history. How can we analyze the critical question “When will it end?”This chapter provides the analytical and econometric framework to answer such aquestion. The central point is that cycles are much more interesting than just marking their peaks and troughs would suggest. Whilst “marking time” is important, itis just the first part of the analysis, and should itself be subjected to methods for distinguishing phases (for example, expansions and contractions of the output cycle).Once phases have been distinguished, their duration and characteristics becomeof interest; for example, do long expansions have a greater chance of ending thanshort expansions? Critical to the analysis is the hazard function: “the conditionalprobability that a phase will terminate in period t, given that it has lasted t or moreperiods.” Cardinale and Taylor consider different models and methods of estimating the hazard function and testing hypotheses related to particular versions of it.They also consider tests of duration dependence, the amplitudes of cycles, and thesynchronization of cycles for different but related variables; for example, outputand unemployment. Their theoretical analysis is complemented with a detailedconsideration of US unemployment.No handbook of econometrics could be without a contribution indicating theimportance of cointegration analysis for non-stationary data. In Chapter 8, Katerina Juselius considers one of the most enduring puzzles in empirical economics,namely, if purchasing power parity (PPP) is the underlying equilibrium state thatdetermines the relationship between real exchange rates, why is there “pronouncedpersistence” away from this equilibrium state? This has been a common finding ofempirical studies using data from a wide range of countries and different sampleperiods. Juselius shows how a careful analysis can uncover important structures inthe data; however, these structures are only revealed by taking into account thedifferent empirical orders of integration of the component variables, the identification of stationary relationships between non-stationary variables, the dynamicadjustment of the system to disequilibrium states, the appropriate deterministiccomponents, and the statistical properties of the model. As Juselius notes, andin contrast to common approaches, the order of integration is regarded here asan empirical approximation rather than a structural parameter. This opens up a
Terence C. Mills and Kerry Pattersonxviidistinction between, for example, a variable being empirically I(d) rather thanstructurally I(d); a leading example here is the I(2) case which, unlike the I(1)case, has attracted some “suspicion” when applied in an absolute sense to empirical series. The challenging empirical case considered by Juselius is the relationshipbetween German and US prices and nominal exchange rates within a sample thatincludes the period of German reunification. The methodology lies firmly withinthe framework of general-to-specific modeling, in which a general unrestrictedmodel is tested down (see also Hendry, Chapter 1) to gain as much informationwithout empirical distor
Title: Palgrave Handbook of Econometrics: Applied Econometrics Author: Terence C