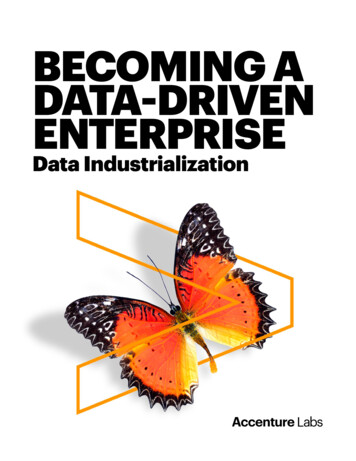
Transcription
BECOMING ADATA-DRIVENENTERPRISEData Industrialization
DATA AS APRODUCTToday, data is everywhere—in industry, in business,in our day-to-day lives. And it’s growing all the time.As digitalization takes ever greater hold, and as millions of new data-rich devices connectin new ways, the volume of data available to the enterprise is growing exponentially. It’senabling unprecedented levels of insight. In fact, becoming data-driven is the new tablestakes for enterprise success.So, what does it mean to be a data-driven enterprise? It means maximizing the value of yourdata, and treating it as an asset differentiated by its completeness, lineage, and quality. It meansusing data as the basis for critical business decision-making through transparent and highquality analytics as opposed to tribal knowledge. In short, it means thinking of your data, andthe analytics that rely on it, as a “product”—one that’s essential for driving business outcomes,whether that’s a medical device manufacturer creating new medical-grade data services tosupport patient healthcare decisions, an oil and gas producer achieving greatly enhancedefficiency in its plants, or a media and entertainment company serving personalized contentto its customers.Adopting a product mindset around enterprise data requires its viability, and the analytics modelsthat derive actionable insights from it, are examined, measured, and refreshed continuously. Aproduct lifecycle approach is necessary—one that considers everything from conceptualizationand design to manufacture and roll-out, as well as ongoing support. Technology-siloed,application-focused data architectures must become outcome-centric architectures, in whichdata is reused multiple times to support partner ecosystems, data-enhanced products, andmultichannel interactions with the user community.Above all, this mindset calls for a deliberate strategy to industrialize enterprise data and models.2BECOMING A DATA-DRIVEN ENTERPRISE
DATAMATURITYMODELAccenture has developed a data maturity model thatcharts the enterprise’s journey to data industrialization.It helps a business shift away from the idea of “single-use data”, where data is tightlycoupled to particular schemas and formats, requiring major rework to make it suitablefor use beyond the original business case. And it helps them instead tap into the bestavailable data at any time for highly relevant actionable insights to support optimizedbusiness decision-making.3BECOMING A DATA-DRIVEN ENTERPRISE
The data maturity model has five stages, covering initial ad-hoc data use through to a fullyindustrialized approach:00Ad-Hoc.Siloed activity. The enterprise lacks a product mindsetand treats its data as a second-class citizen. Data istightly coupled with applications, with limited capacityfor internal sharing thanks to a lack of data strategy,architecture, delivery, or risk management.01Organize.Sparking excitement. The enterprise recognizes thevalue of its data as an asset. It begins to decouple datafrom applications and develop a data-product mindsetthrough a business vision and data strategy.02Tactical.Building momentum. The enterprise proves the valueof its metrics and data-driven methods, standardizingits tools, templates, and methods to create an essentialfoothold as a data business.0304Critical.Moving to production. The enterprise builds welldefined and automated methods for developing its dataproducts, establishes a well-structured data productcatalogue, and provides self-service capabilities acrossthe organization. It transforms data into an independentdigital asset for the business and expands its use outinto the connected ecosystem.Industrial.Data as differentiator. Data is treated as a first-classcitizen, and drives optimal business outcomes.The competitive positioning of the enterprise isdifferentiated by the quality of its data products inthe digital ecosystem.The model charts these five stages across the dimensions of strategy and governance,architecture, development, regulation and ethics, and user support.4BECOMING A DATA-DRIVEN ENTERPRISE
Accenture’s DataMaturity ModelMISSION CRITICALAutomatedoutcome-centricdata AutomatedtestingData pipelineAutomatedrisk, reg &complianceData profiling& qualityRationalizedplatforms nagementSecurity &entitlementKey usecasesRegularauditPrescriptiveQA & reviewOverall archdesignStakeholderORGANIZEMetering &monetizationCross-geomanagementDefinition,policy &compliancecatalogModel-drivendevelopmentSLA modeling& t roadmap& releasesRationalizedata assetsCode ofEthicsDisclosuresand termsLegal review& inventoryStatic portalStrategy &GovernanceBECOMING A DATA-DRIVEN ENTERPRISEArchitectureBusiness &operationalpattern miningTransparencyin supply chainStandardizeddev rtnerecosystemEvangelize essReporting mated usagemonitoring & proactivesystem updatesSelf-serviceportalRegulation& EthicsUser Support
Strategy and GovernanceHANDLING DATA ASSETS LIKE A PRODUCT.A mature data strategy recognizes that data and analytics models are productsthat differentiate the business in the digital ecosystem. The strategy should thusdetermine the business objectives (whether that’s growing the customer base, creatingpersonalized recommendations, or anything else) and outline how to obtain the dataand models to best fulfill those objectives. This use of strategy to optimize deliverydrives the other dimensions of the data industrialization journey.6BECOMING A DATA-DRIVEN ENTERPRISE
In the context of strategy and governance, actions for the stages of the previously introduced datamaturity model can be prescribed as follows:01Organize.Define the product-centric vision of how data and modelssupport business outcomes. For each data initiative, identifythe business and technical stakeholders who will buy in tothat vision’s objectives, strategy, and tactics.02Tactical.Apply the data-product mindset to key use cases, consideringhow to get the best data and models for the desired businessoutcomes. Identify success metrics and build a data committeeto oversee development and release against the strategy.Define data policy and compliance requirements.03Critical.Scale the data-as-a-product approach by defining a roadmap,including subsequent launch cycles, and building a largerpartner ecosystem.04Industrial.At full maturity, the enterprise runs its data and models like aproduct, shifting to selling data outcomes, and extending outinto the digital ecosystem of related data products.TRANSFORMING A BUSINESS FROM FARMINGEQUIPMENT TO FARMING INSIGHTS.Consider a farming equipment manufacturer’sjourney from selling equipment and associatedmaintenance services to running enterprisedata and models as differentiating products.By adding IoT sensors to their farm equipment,they can collect data for new remotediagnostics, optimization, and predictivemaintenance services. By taking a productmindset, a new digital opportunity then opensup. With the smart farming equipment’s7BECOMING A DATA-DRIVEN ENTERPRISEunique visibility of the land being farmed –and the farmer’s approach – they can nowprovide information services on critical factorslike soil, planting, and equipment. The companycan thus become a player in an ecosystemfocused on maximizing farming productivity,working with futures markets, chemicalscompanies, insurers, and others, to transformitself into a provider of unique farming insightsas digital products.
ArchitectureFROM APPLICATION-CENTRIC TO DATA-CENTRIC.An industrialized data architecture is data-centric rather than application-centric.Its technology stacks, mechanisms, and orchestrations are focused on maximizingthe security and value of data for the enterprise. It uncouples valuable data fromapplications, enabling its easy reuse – both by the business and by the widerecosystem of partners.8BECOMING A DATA-DRIVEN ENTERPRISE
01Organize.Take an inventory of existing data and model assets andback-end systems. Rationalize the services that will formthe components of future enterprise-level data products.02Tactical.Standardize the overall architecture. Evaluate best practices andtechnology stacks to enhance cost-effectiveness, performance,scalability, and security. Create common configurable andcustomizable methods for data onboarding and use.03Critical.Build a common methodology to right-size the level of datamanagement and protection for data whether it resides inthe cloud or on-premise data centers. Apply metering andchargeback on service use and quality. Automate provisioningand scaling mechanisms.04Industrial.The enterprise architecture is data-centric and secured. It notonly supports publishing data out, but also enables the easyand flexible factoring of data and models from third parties topower a cross-ecosystem data business.COMPETING ON THE MANAGEMENT OFMEDIA AND ENTERTAINMENT CONTENT.A media and entertainment companycompetes on its ability to provide subscriberswith content customized by genre, cast,director, language, or numerous other factors.It must store and process vast volumes ofdata – most of which is provided by others—adding subtitles or other local distributorlogos as necessary. With an industrializedarchitecture, the company can store data inthe most cost-effective system for its needs,factoring in geographic distribution to managethe transmission limits of available Internetbandwidth. Real-time updates can be handled,so unscheduled events like overrunningsports games can be reflected immediately9BECOMING A DATA-DRIVEN ENTERPRISEin programming guides. Data is normalized andtransformed into common schema objects,such as linking an original English-languagemovie with its Spanish version or its sequel.Enterprise data assets are thus standardizedand suitable for reuse and discovery, enablinghighly personalized recommendations thatfactor in both proprietary content and thirdparty content (ratings, user-generated reviews,interviews and articles from other platforms,etc.). A data-centric architecture makes it easyfor the company to use and customize datafrom others and thus create new and enhancedproducts for their subscribers.
DevelopmentBECOMING AGILE AND DYNAMIC.An industrialized development process is agile and dynamic, streamlininginnovation to create new data-powered business outcomes in a fast-evolving,ever-changing market. A commitment to the best data and models requires aniterative approach. It includes data and model lifecycle and DevOps processesthat allow for rapid iteration to deploy, optimize, and redeploy new datasetsand models to best support the business.10BECOMING A DATA-DRIVEN ENTERPRISE
01Organize.Define standard methodologies, code templates, and use casesfor the development, testing, deployment, and production ofdata and models.02Tactical.As more data and model products are created, enforceformalized standards across architectural components,development tools, documentation, and quality assurance.Ensure data publication and consumption processes alignwith the business vision.03Critical.Implement DevOps processes for data products, adopting dataand model lifecycle management frameworks, configurable datapipelines, and automated testing and release cycles. Includecontinuous monitoring that guarantees data quality and modelhealth. Use governance and audit features to provide validationand closed-loop feedback.04Industrial.Custom data products are created, tested, and deployedby leveraging configuration and model-driven automatedprocesses that enable the “citizen user” within the business.Automation implements champion-challenger analytical modelcomparisons scaling the number of training and retrainingexperiments to improve outcomes.MANAGING MODEL DEVELOPMENT AT SCALETO IMPROVE PLANT OPERATIONS.To optimize plant operational efficiency,an oil and gas company developed aseries of composite real-time models,involving data from numerous sensors fedinto forecasting models, then into assethealth and performance models, and thenrolled up into an operations status used fordecision-making – all created, governed,and maintained by cross-organizationalbusiness owners, data scientists, and domainexperts. With each of these complex modelpipelines deployed thousands of timesacross the plant, some inevitably requiredrework, retraining, and feature engineering11BECOMING A DATA-DRIVEN ENTERPRISEover time. A model-specific DevOps processwas implemented to industrialize developdeploy-monitor cycles. Model failure wasconsidered from the outset, requiring datascientists to specify the data quality conditionsand accuracy and precision evaluations usedto automatically monitor running modelsand trigger actions like retraining models ortaking them out of deployment if needed.The result: an automated system to ensuremodel accuracy and a way to accelerate andscale model development ensures trust in theprescribed updates to plant operations.
Regulation and EthicsACQUIRING TRUST THROUGH TRANSPARENCY.An industrialized approach to regulation and ethics supports the transparency ofdata use to help the enterprise build a trusted digital reputation in the market. Itdefines a code of ethics as a common language for eliminating risk, standardizingaudit processes, and leveraging new technologies like blockchain to ensurecompliance and transparency for all data stakeholders12BECOMING A DATA-DRIVEN ENTERPRISE
01Organize.Create an inventory of the legal and regulatory rules applicableto data services.02Tactical.Provide mechanisms to ensure informed consent is acquired fromdata owners and data subjects. Define a code of conduct, as wellas policies and procedures, to ensure digital trust is establishedconsistently for data services.03Critical.Maintain a single logical view of data through a universal metadatamodel across the data supply chain. Log everything that happens tothe data and make the footprint visible to data stewards. Implementtransparency and explainability in AI models. Evaluate whetheractivities conducted on the data agreed upon usage standards.Audit regularly to assess security and risk control and monitoring.04Industrial.Automated monitoring programmatically supports regulatorycompliance, while proactively identifying ethical risks to theenterprise – and taking automated action to flag for human review.Transparency is baked into data use right across the value chain.SPOTTING FINANCIAL FRAUD THROUGHTRUSTED DATA SHARING.Consider a collaborative anti-moneylaundering application used by multiple banksin a way that allows them to share costs andimprove the detection of suspicious activity. Indeveloping the application, guaranteeing trustand data transparency was key, enabling eachbank to monitor and control how their data wasbeing used. Beyond their legal agreements,each bank could programmatically track13BECOMING A DATA-DRIVEN ENTERPRISEthe lineage of their data and its use acrossthe whole data supply chain. They could alsocontrol the models that were applied, whetherthey were shared models recommended by theapplication and other banks, or private modelsof their own. The result is a living system of trustand transparency, in which data access can beprogrammatically tracked and enforced basedupon the agreed upon rules set by each bank.
User SupportOPTIMIZING WITH SELF-SERVICE.An industrialized approach to user support rests on a product mindsetthat makes data and models easy and appealing to use. It extends selfservice capabilities beyond the data experts to the whole enterprise ina user-friendly way, while also supporting closed-loop optimization. Theambition: to unlock the greatest benefits for the enterprise by usingdata to power insights for “citizen” users like the business analyst, thedomain expert, or the operations engineer.14BECOMING A DATA-DRIVEN ENTERPRISE
01020304Organize.Set up a static portal for users to discover and accessenterprise data, and communicate it with their teams.Tactical.Foster innovation by proactively supporting the development ofnew use cases for the data. Calculate standardized cost-benefitmeasures, and incorporate business-level tools for definedreporting needs.Critical.Scale the data-as-a-product approach by defining a roadmap,including subsequent launch cycles, and building a largerpartner ecosystem.Industrial.Prescriptive insight-driven actions are enabled across theenterprise. A mature, optimized and contextualized data/insight service is available for all users as they need it,supported by knowledge graphs, AI-enabled interactions,and other technological innovations.PRESCRIBING OIL AND GAS WELL PLANNING VIAINTERNET-SEARCH-LIKE EXPERIENCES.Today, the process of creating an oil or gaswell design is largely expert-led. A drillingengineer plans where and how to drill basedon his or her experience. Even the dataused to create a well offset report, whichcompares the well with previous designs, isoften based on a combination of basic filteron the geographical region and the individualengineer’s experience. There is much value tobe had in augmenting this expert-led processwith the wealth of data that lies beyond theengineer’s immediate experience – providedthat finding and processing that data issimple. Internet search provides a valuableexample to follow. In automatically indexing15BECOMING A DATA-DRIVEN ENTERPRISEcontent and then extracting and presenting itin a contextual way (in other words, presentingresults differently when a search is about acompany, a person, or a work of art, etc.), itpresents content in a highly accessible andrelevant way. By applying the same techniques,an oil and gas company can create a knowledgemodel that covers a well and everything thatthe drilling engineer needs to consider. It canthen use automated techniques for extractingthese insights from enterprise data, creating anInternet-search-like capability that equips thedrilling engineer with the ability to make betterdata-driven decisions in their drilling plans.
INDUSTRIALIZEDDATAAs the volume of enterprise data explodes, and asthe number of potential data use cases acceleratesexponentially, an industrialized approach to data andmodels is quickly becoming a must-have capability.In today’s leading digital-native and data-driven enterprises, data and models arealready treated as first-class citizens—capable of taking a truly differentiated offeringto the market.Accenture’s data maturity model charts a path for traditional enterprises to develop thisindustrialized capability and take a lead in the digital ecosystem. This journey enables anever-greater degree of automation and rapid delivery to create the best data and the bestmodels to enable business outcomes.Industrialization helps the enterprise fully leverage and monetize its data and model assets,generating the insights that can both drive the core business forward and create the entirelynew outcome-driven digital business models that pivot into the new. In this way, companiesthat were once tied to particular products or services are transformed into robust data-drivencompanies. The journey to data industrialization is the key to unlocking the data-drivenenterprise of the future.16BECOMING A DATA-DRIVEN ENTERPRISE
CONTACTSTeresa Tung, Ph.D.Managing Director,Applied Intelligence Innovation Lead,Accenture LabsJean-Luc ChatelainManaging Director,CTO Applied IntelligenceCONTRIBUTORFang HouABOUT ACCENTURE LABSAccenture Labs incubates and prototypes new conceptsthrough applied R&D projects that are expected to havea significant strategic impact on clients’ businesses. Ourdedicated team of technologists and researchers workwith leaders across the company to invest in, incubate anddeliver
innovation to create new data-powered business outcomes in a fast-evolving, ever-changing market. A commitment to the best data and models requires an iterative approach. It includes data and model lifecycle and DevOps processes that allow for rapid iteration to deploy, optimize, and redep