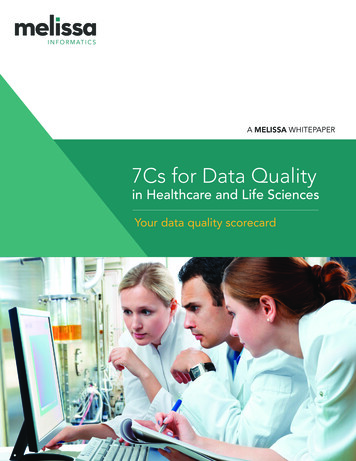
Transcription
A MELISSA WHITEPAPER7Cs for Data Qualityin Healthcare and Life SciencesYour data quality scorecard
TABLE OF CONTENTS2INTRODUCTION3CLEAN - CERTIFIED ACCURACY4CONNECTED5COORDINATED6-7COMPLIANT8COST EFFECTIVE9CONSUMER CENTERED10CONFIDENT7CS FOR DATA QUALITY IN HEALTHCARE AND LIFE SCIENCES1
INTRODUCTIONHOW DO YOU SCORE ONTHE 7 CS OF DATA QUALITY?One way or another, almost everyone in health careand life sciences industries must address the issueof data quality. Why? Because dirty, disconnecteddata represents lost intellectual property, whileclean, well-connected data is prepared to deliverknowledge, reduce costs and boost the bottom line.Data quality makes innovative analytics possible andreduces cost and time to market, while improvingefficacy for new drugs and precision medicine products. Organizations with healthy data and effectivelong-term data quality and integration strategiessave money on research, safety, trials and delivery.They formulate effective scientific, clinical and business strategies and marketing campaigns based onaccurate data. Their customers experience higherlevels of satisfaction. But how many business understand how to evaluate and correct issues related todata quality?In today’s data-driven marketplace, data quality issues can no longer be downplayed or farmed out tointernal development. The health of your company’sdata impacts initiatives and departments rangingfrom early stage discovery, development, safety andefficacy to regulatory submissions, trials, marketing,sales, billing, accounting, clinical care and compliance.Melissa Informatics’ aim is to help researchers,business managers and executives, marketingprofessionals and other personnel understand whatdata quality is, why it is important, and how theycan quickly clean up and connect their company’smission-critical data.That’s why the 7 Cs of data quality in healthcare lifesciences are essential. We’ll look at each one of the 7Cs in detail so you can absorb and apply the fundamental principles of data quality. The 7 Cs are:1. Clean – curated by deep data identification andcorrection methods for certified accuracy2. Connected – meaningfully linked and searchableacross previously disconnected data sources3. Coordinated – improved interoperability with otherexisting and new data sources4. Compliant – aligned with FAIR, HIPAA, EMEA, GDPRguidelines as required5. Cost Effective – prepared for revenue-bearing valueat lower near and long-term cost6. Consumer Centric – drug-centered, patient-centered,consumer-centered data as required, to more effectivelyprofile, communicate with and bring life saving value toyour market7. Confident – certified accuracy through automatedQA, with provenance and QC functionality, so you canbe confident your data is correctThe 7 Cs are building blocks for data quality. Theyprovide a quick reference ‘scorecard’ to help businesses - whether large or small - assess the health oftheir organization’s mission critical data. No matterwhether your business is located in Europe, Asia orNorth America, data quality is a must for the21st century.7CS FOR DATA QUALITY IN HEALTHCARE AND LIFE SCIENCES2
CLEAN - CERTIFIED ACCURACYHOW CLEAN IS YOUR DATA?Do you know what percentage of your historicalclinical trials data was correctly entered?*Companies are easily spending from 20 to 80 milliondollars or more for Phase 1-3 clinical trials. ElectronicData Capture (EDC) for phase 1 trial alone, with 200patients tracked over 10 visits, costing 500 / visitfor data acquisition, can cost over a million dollars –just for a small, preliminary trial.How can you be confident that the informationin your new database is accurate? Business cannow employ a solution that will validate, correct,and standardize clinical trials data – even withoutusing expensive specialized EDC applications andmethods.7CS FOR DATA QUALITY IN HEALTHCARE AND LIFE SCIENCESMelissa Informatics can take data directly fromEMR systems to create high quality research data,aligned as needed with FDA guidelines for clinicaltrial submissions. This is true of data from any source,including from discovery and safety studies, historicaltrials data, ongoing EMR data collection.Save money by using the data you are alreadyacquiring, rather than by creating expensive newEDC data acquisition efforts.*“Optimizing the Use of Electronic Data Sources in ClinicalTrials: The Landscape, Part 1”. Ed Kellar, Susan M. Bornstein,Aleny Caban. October 13, 2016 Sage Publications, TherapeuticInnovation & Regulatory Science 2016, Volume: 50 issue: 6,page(s): 682-696. Research Article 790166706893
CONNECTEDIT’S TIME TO GET YOUR DATACONNECTEDDirty, disconnected data costs the Pharma industrybillions of dollars a year to address. Even with thesecosts, more projects fail (and more money is spent)due to challenges with data harmonization andintegration.Extensive data harmonization may be required toconnect two datasets. Spelling errors and dataentry typos aside, one database may use the term“acetaminophen”, while the other calls the drug“Tylenol”. Additionally, duplicate data need to behandled and preferred terms need to be defined.Traditional methods for harmonizing and connectingdatasets are brittle. You may choose to harmonize toone standard (for example, FDA drug terminology).What if you need a different set of preferred termslater? Harmonizing and re-harmonizing data todifferent standards takes too much time and money.Now you can align your data to different standardsfor harmonization “out of the box”. Melissa providesrich resources for standards alignment and easy realignment to different preferred terms and standardsas needed, without refactoring the entire database.CREATING THE GOLDEN RECORDThe process of producing a golden record (alsoknown as survivorship) is a key goal for connecteddatasets. This is a central step in the recordmatching process involving partially duplicateddata. It requires identifying the record with the bestdata quality. There are three common techniques indetermining the surviving record:Most Recent – the most recent record can beconsidered elegible as the survivor.Most Frequent – matching records containingthe same information are also an indication forcorrectnessMost Complete – records with more valuespopulated for each available field are also viablecandidates for survivorship.Melissa Informatics can go beyond “genericsurvivorship” techniques to leverage context andreference data. This makes more sophisticatedunderstanding of data contents possible. WithMelissa, you can pull from multiple records, applyingmachine reasoning and reference information inorder to create golden records – even when originaldata quality is poor or inconsistent.Now you can employ Melissa Informatics’ solutionto connect data in innovative ways. Data modelsor “ontologies” link data in ways that makeconnections easier to create, easier to modify fordifferent purposes, more useful for creating “goldenrecords”, and ready for deep machine reasoning.Save time and money getting and keeping dataconnected – and open up new opportunities fordiscovery – by connecting your data under flexibleand meaningful NoSQL “ontologies”.7CS FOR DATA QUALITY IN HEALTHCARE AND LIFE SCIENCES4
COORDINATEDOH NO, WE DON’T WANT ANOTHER BIGDATA WAREHOUSE!Along with the problem inconsistent, disconnecteddata, another “dirty secret” plagues the healthcarelife sciences industry. This is the lack of coordinationacross existing and new data resources. Traditionaldatabases aren’t readily extensible, or prepared forintegration with new data.How many times has your company completed abig new “integrated data warehouse” project onlyto find that the expensive data warehouse isn’tprepared to connect to that other data resource youjust realized you need?With Melissa, any new integrated data resourceyou create is prepared for connection to otherresources without refactoring the original databaseschema and without rebuilding an entirely new datawarehouse. Adding new data is as easy as adding anew friend to your social network. Just connect thedata to any common classes and elements within theexisting data network, and add new links for the newdata. It’s that easy.If you have two or more datasources that have beencleaned and connected by Melissa, integrating themis as easy as “drag and drop”. Pull the two datasetstogether within Melissa’s semantic databaseenvironment and they will automagically connect onall common classes and terms.Melissa has customer examples where twocompletely different groups, without explicitcooperation, each applied the same open semanticstandards for their integration process. Later it wasdesired to connect these two databases. Followingthe semantic methods employed by Melissa, thesedatabases connected without additional integrationeffort. The customer calls this “coordination withoutcooperation”. This represents a huge step forwardfor data interoperability.Ensure long-term value for your integrateddata resources by making sure your methodsare coordinated to ensure extensibility andinteroperability at lower time, effort and cost.7CS FOR DATA QUALITY IN HEALTHCARE AND LIFE SCIENCES5
COMPLIANTENSURE COMPLIANCE WITHREGULATORY GUIDELINES ANDSTANDARDSIf you’ve never been a member of a standardsdefinition body, or if you’ve never been a part ofthe discussion about what standard(s) you need toalign your data with, consider yourself lucky. Therehas been much confusion and disagreement overthe past decades about standards. Billions of dollarshave been spent defining standards, determiningwhat standards should be used, why they should beused, and how they should be applied.Standards are useful. They provide critical commonconcepts and terms and useful disciplining structure.With Melissa you can align your data with thestandard you need, “out of the box”. Melissa bringsa curated set of published standards to you. We canhelp you meet FAIR, HIPAA and other guidelinesand standards at lower time and cost. We can alsocombine, create, apply and even modify standardsto meet your specific needsproviders must comply with specific governmentregulations regarding data handling, data sharing,data access, submission claims, even billingprocedures.One provision of HIPAA 5010 deals specificallywith, for example, address contact data. To be incompliance, providers must include a full 9-digit ZIPCode for billing provider and service locations onall claim submissions. Another HIPAA rules prohibitsthe use of a PO Box for the billing provider address.If a health care provider’s data is in chaos and theycannot comply, they will have to pay the price.While the specifics of compliance are differentdepending on the industry and regulation inquestion, the bottom line is that compliance issuesintersect with almost every facet of most health careand life science data, forcing businesses to look longand hard at improving and maintaining their dataquality and compliance.THE HIPAA EXAMPLEHIPAA provides an excellent real-world exampleof the relationship between data quality andcompliance. In response to HIPAA, health care7CS FOR DATA QUALITY IN HEALTHCARE AND LIFE SCIENCES6
COMPLIANT (CONT)MAINTAIN COMPLIANCE OVER TIME WITH AGILITYStandards can also be constraints! What if youaligned your data with one standard or set ofstandards and lexicons, then learn that you wouldlike to use the data for other purposes, requiringother standards? This often happens. For example,you may have defined your data according toa narrowly defined “SDTM” standard for studydata. Later your boss determines that this data isready for submission to FDA, perhaps for a NewDrug Application (NDA). This requires the CDISCstandard.*With traditional methods, realigning your data toa new standard can take more time and cost thanyou have to spend. By the time you’ve redefinedyour database to handle the changes classes andrelationships required by the new standard, thewindow of opportunity for that submission haspassed. You need technology that can make itpossible to align your dataset with new standardswithout refactoring the database.With Melissa, you can benefit right away bythe application of formal standards for dataharmonization, integration and compliance.However, you aren’t trapped by your initialdecisions. Now you can more efficiently align andre-align your data with different standards as youneed ctronicSubmissions/UCM511237.pdfWhat does FAIR stand for?To meet FAIR guidelines*, data must be findable,accessible, interoperable and reusable whilemaintaining regulatory compliance for protectedhealth information, contractual and businesscompliance, security, and confidentiality. To ensure Findable data, Melissa Informatics can addstructure and metadata to your data, includingsearchable globally unique persistent identifiers.We apply the World-wide-web Consortium’s(W3C) Resource Description Framework (RDF)standard for unique resource identifiers (URIs)to be provided for all data and metadata elements in a secure, standards-based data source.To be Accessible, (meta)data can be made beretrievable by their identifier using a standardized communications protocol that is universallyimplementable with authentication and authorization; and metadata will be managed to ensureaccessibility. To be Interoperable, all data canbe set to follow accessible, shared, and broadlyapplicable language and methods for knowledgerepresentation, using standards and lexiconsthat follow FAIR principles. To be Reusable, datacan be described with a plurality of relevant attributes, released with a clear and accessible datausage license, associated with provenance, meeting domain-relevant community standards. Ask usabout helping you meet FAIR guideline with 6187CS FOR DATA QUALITY IN HEALTHCARE AND LIFE SCIENCES7
COST EFFECTIVEHOW MUCH IS BAD DATA COSTINGYOUR ORGANIZATION?U.S. businesses lose over 600 billion a year becauseof bad data, with more than 25% of that due tocustomer data entry errors. This problem has grownexponentially for clinical data acquisition. MelissaInformatics has examples from electronic medicalrecords system where a single drug was recordedin over 190 different ways. Dealing with this sortof complexity in order to create unified, accurate,research or submission-ready datasets can be veryexpensiveHow much money would your company save everyyear if your data could be prepared for revenuebearing value at lower near and long-term cost? Areyou sure you’re finding all of the internal data (andsufficient external published data) relevant to yourresearch project – before you start? Can you be sureevery patient in this cohort are really not taking anycontraindicated drugs for the clinical trial treatment?To answer these sorts of questions, you need tounderstand how much bad data is costing yourorganization right now.7CS FOR DATA QUALITY IN HEALTHCARE AND LIFE SCIENCESKEEP BAD DATA OUTConsider the “1-10-100 Rule” which posits that ittakes 1 to verify the accuracy of a record at pointof-entry, 10 to clean it in batch mode, and 100per record if nothing is done (this includes costsassociated with repeated experiments, undetectedsafety issues, clinical trials rejections and overprescribed drugs, etc.). Therefore the best ROI canbe attained by employing a “data quality firewall”at point of entry, to immediately verify the accuracyof information. For historical data, best ROI can beattained by automating the data validation process.With Melissa, real-time data entry solutions areavailable via services, and enterprise integrationplatforms can be provided to automate historicaldata cleansing and integration. Save money bykeeping bad data out and by turning your old ‘dirty’data into “golden’ data, ready for accurate researchand business analysis.8
CONSUMER CENTEREDBRING YOUR DATA TO LIFE OR LEAVE ITIN A “DATA TOMB”Has your organization completed major dataacquisition or data cleaning and integrationprojects only to realize the data wasn’t effectivelytargeted to the actual requirement or consumer? Itcan be difficult if not impossible in many cases tounderstand every question your internal or externalcustomer will want to ask of the data beforethey’ve seen it.Billions of dollars are spent annually on new dataacquisitions and new integrated data warehouses, inprojects that end up languishing and under-utilizedbecause the ‘final’ dataset isn’t meeting the actual,ongoing needs of the customer.RIGHT DATASETS, RIGHT STANDARDS,RIGHT TIMEIn order to be sure you have the data your customerneeds, you need to be able to more efficientlyadd new data, take data out (for example, quicklyremove all required identifying information whenneeded for patient research data), align the datawith new terminologies and linkages as needed tomeet your research, partnering or business goals.Melissa’s semantically enabled “noSQL” data ispurpose-built to support rapid extension to addnew data sources, “no-re-factoring” modificationsto change or remove existing data structure andcontent as required to meet new goals, and toalign and re-align with different terminologies andstandards as needed, without breaking the existingdatabaseWith Melissa, you can be confident that you’ll havethe right data when you need it, aligned with theright standard for the customer, when you need it.7CS FOR DATA QUALITY IN HEALTHCARE AND LIFE SCIENCES9
CONFIDENTIT’S TIME TO BECOME E-CONFIDENTRepeated or failed research due to missing,disconnected data or cost and time required toclean and integrate data costs organizations billionsannually. Do you have confidence that you are usingthe data that you have already created, at great costand expense? Are you confident that the data youare using is accurate? Does it take too much time toclean and integrate the data you need to meet yourgoals?In today’s competitive global markets, you can’tafford to continue operating under low confidencedata environments. Verifying research and medicaldata at the point-of-entry eliminates inevitablecosts of bad data. Automating data integration andcuration makes it possible to avoid an exponentialincrease in downstream costs.With Melissa Informatics, you can be confident youhave the data quality you need to reduce costs andmaximize revenue for your business.Take the Next StepSo now that we’ve run through the 7 Cs ofhealthcare life science data quality, how would yourate your business? Are you a master data stewardof is there room for improvement?Regardless of whether data quality is already a toppriority at your company or if you are just beginningto discover its key concepts, we’re certain youcan utilize the 7 Cs of data quality to quickly makea significant contribution to your organization’sbottom line. Remember: the data quality investment7CS FOR DATA QUALITY IN HEALTHCARE AND LIFE SCIENCESyou make now will pay dividends down the roadin terms of improved outcomes, saved time andmoney.Now that you’ve had your introduction to the 7Cs, it’s time to take the next step. Visit MelissaInformatics at www.melissainformatics.io to learnmore about where your business stands today interms of data quality, and to explore how we canhelp you achieve the 7 Cs at the lowest time andcost with the highest ROI.10
www.melissainformatics.ioAbout Melissa InformaticsMelissa Informatics extends the capabilities of Melissa’s global intelligence software and services to support worldleaders in life sciences, biotechnology, pharmaceutical, and medical industries by harnessing the entire data lifecyclefor business, pharmaceutical and clinical data. Our software and services bring data quality and machine reasoningtogether for insight and discovery by intelligently cleaning, connecting and harmonizing multiple sources to offerinteroperable data. Melissa Informatics red
data quality? In today’s data-driven marketplace, data quality is-sues can no longer be downplayed or farmed out to internal development. The health of your company’s data impacts initiatives and departments ranging from early stage discovery, development, safety a