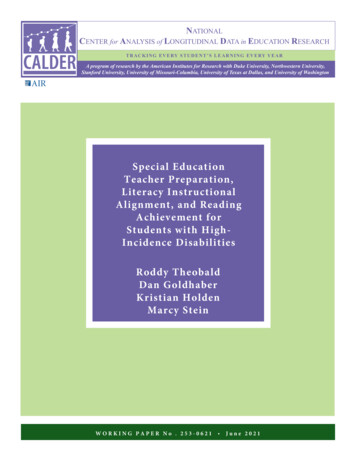
Transcription
NATIONALCENTER for ANALYSIS of LONGITUDINAL DATA in EDUCATION RESEARCHTRACKING EVERY STUDENT’S LEARNING EVERY YEARA program of research by the American Institutes for Research with Duke University, Northwestern University,Stanford University, University of Missouri-Columbia, University of Texas at Dallas, and University of WashingtonSpecial EducationTeacher Preparation,Literacy InstructionalAlignment, and ReadingAchievement forStudents with HighIncidence DisabilitiesRoddy TheobaldDan GoldhaberKristian HoldenMarcy SteinWORKING PAPER No . 253-0621 June 2021
Special Education Teacher Preparation, LiteracyInstructional Alignment, and ReadingAchievement for Students with High-IncidenceDisabilitesRoddy TheobaldCALDER, American Institutes for ResearchDan GoldhaberCALDER, American Institutes for ResearchUniversity of Washington SeattleKristian HoldenCALDER, American Institutes for ResearchMarcy SteinUniversity of Washington Tacoma
ContentsContents . iAcknowledgments. iiAbstract . iii1.Introduction . 12.Data and Methods . 52.1 Data . 52.2 Samples, Measures, and Summary Statistics . 102.3 Analytic Approach . 113.Results . 174.Discussion . 204.1 Limitations . 214.2 Policy Implications . 22References . 25Tables and Figures . 29Appendix A . 41i
AcknowledgmentsThe research presented here would not have been possible without the administrative data provided bythe Washington Office of the Superintendent of Public Instruction through data-sharing agreement2015DE-030 or without the student teaching data provided by teacher education programs from thefollowing institutions participating in the Teacher Education Learning Collaborative (TELC): CentralWashington University (CWU), City University, Evergreen State College, Gonzaga University,Northwest University, Pacific Lutheran University, St. Martin’s University, Seattle Pacific University,Seattle University, University of Washington Bothell, University of Washington Seattle, University ofWashington Tacoma, Washington State University, Western Governors University, and WesternWashington University. The research presented here utilizes confidential data from CWU. The viewsexpressed here are those of the authors and do not necessarily represent those of CWU or other datacontributors. Any errors are attributable to the authors.The research reported here was supported by the Institute of Education Sciences, U.S. Department ofEducation, through Grant R324A170016 to the American Institutes for Research. The opinionsexpressed are those of the authors and do not represent views of the Institute or the U.S. Department ofEducation. This research was also supported by the National Center for Analysis of Longitudinal Data inEducation Research (CALDER), which is funded by a consortium of foundations. For more informationabout CALDER funders, see www.caldercenter.org/about-calder. The collection and cleaning of theTELC data was funded by the Bill and Melinda Gates Foundation (grant #OPP1128040) and ananonymous foundation. The authors wish to thank participants at the Boston University WheelockEducational Policy Center Seminar Series, Kaylan Connally, Kirk Walters, and the expert planning teamand advisory board for this project, including Gail Coulter, Glenna Gallo, Kari Lewinsohn, Val Lynch,Laura Matson, Fran McCarthy, Darcy Miller, Bill Rasplica, Ilene Schwartz, and Janice Tornow, forcomments that improved this analysis. Finally, we wish to thank Natsumi Naito and Seraphina Shi foroutstanding research assistance, and Nate Brown, Jessica Cao, Elliot Gao, Andrew Katz, Tony Liang,Arielle Menn, Rain Ortega, Cameron Thompson, Stacy Wang, Malcolm Wolff, Hilary Wu, and YunqiZhang for their support with data collection and cleaning.CALDER working papers have not undergone final formal review and should be cited as workingpapers. They are intended to encourage discussion and suggestions for revision before final publication.Any opinions, findings, and conclusions expressed in these papers are those of the authors and do notnecessarily reflect the views of our funders.CALDER American Institutes for Research1400 Crystal Drive 10th Floor, Arlington, VA 22202202-403-5796 www.caldercenter.orgii
Special Education Teacher Preparation, Literacy Instructional Alignment, and ReadingAchievement for Students with High-Incidence DisabilitiesRoddy Theobald, Dan Goldhaber, Kristian Holden, Marcy SteinCALDER Working Paper No. 253-0621June 2021AbstractWe used survey and administrative data from Washington State to assess the degree to whichspecial education teacher preparation, district literacy instructional practices, and the alignmentbetween preparation and practice were associated with the reading test score gains of studentswith high-incidence disabilities taught by early-career special education teachers in grades 4-8.These students tended to have larger reading gains when their district emphasized evidencebased literacy decoding practices (e.g., phonological awareness, phonics, and reading fluency)and when their special education teacher graduated from a teacher education program that alsoemphasized these practices. Students with high-incidence disabilities in districts thatemphasized balanced literacy practices tended to have lower reading gains. Finally, studentswith high-incidence disabilities taught by early-career special education teachers tended to havelarger reading gains when their teacher’s student teaching placement was supervised by a moreexperienced cooperating teacher.iii
1. IntroductionA significant body of quantitative research demonstrates that teachers are the mostimportant schooling factor in predicting a student’s academic success (e.g., Rivkin et al., 2005).Notably, far fewer studies have investigated the influence of special education teachers on theoutcomes of students with disabilities (e.g., Feng & Sass, 2013; Gilmour, 2019; Theobald,Goldhaber, Gratz, & Holden, 2021). Considerable prior research has emphasized the importanceof special education teacher preparation (e.g., Brownell et al., 2005, 2010), particularly as itrelates to subject expertise (e.g., Brownell et al., 2009), pedagogical skills (e.g., Leko et al.,2012), and high-leverage practices (e.g., Billingsley et al., 2019). However, several recentreviews have noted that no large-scale empirical evidence connects specific features of specialeducation teachers’ preparation to the achievement of students with disabilities in theirclassrooms (Brownell et al., 2020; Sindelar et al., 2010).In response to this lack of empirical evidence, Brownell et al. (2020) recommended thatfuture research on special education teacher preparation should “leverage preparation programand existing state data to better understand the characteristics of effective teacher educationexperiences,” as measured by their “eventual performance once they transition to their firstteaching jobs” (p. 39). The current study follows this recommendation by connecting literacyinstructional practices taught in special education teacher education programs (TEPs) and/oremphasized by K–12 districts in special education instruction to the test achievement of studentswith high-incidence disabilities in English language arts (ELA).Our analysis was made possible by a unique data set from Washington State thatcombines information about preservice teacher candidate experiences provided by 13 specialeducation teacher education programs (TEPs) with data on K–12 teachers and their students1
provided by the Washington State Office of the Superintendent of Public Instruction (OSPI). Wecombined this data set with novel survey data on the literacy instructional practices taught inspecial education TEPs (as reported by teacher preparation faculty) and the instructionalpractices emphasized in special education instruction (as reported by district special educationdirectors in the state). This data collection allowed us to create a longitudinal data set that tracksspecific special education teacher candidates from their TEPs to their student teachingplacements and into specific special education placements in the state’s K–12 public schools.We used this data set to contribute to three different lines of research. First, prior researchhas linked some broad measures of special educators’ preservice experiences to outcomes forstudents with disabilities (Feng & Sass, 2013; Gilmour, 2019; Theobald, Goldhaber, Gratz, &Holden, 2021). For example, Feng and Sass (2013) found that teachers who were certified toteach special education, who majored in special education, and who took more special educationcoursework were more effective in terms of improving the achievement of students withdisabilities in reading. A growing body of literature that is not specific to special education hasalso investigated the relationship between more specific measures of teacher preparation—suchas the student teaching experiences of teacher candidates—and the achievement of their studentsonce they enter the workforce (e.g., Boyd et al., 2009; Goldhaber et al., 2017, 2020; Ronfeldt,2012, 2015; Ronfeldt et al., 2018). For example, Goldhaber et al. (2020) and Ronfeldt et al.(2018) found that candidates who were supervised by a more effective cooperating teacherduring student teaching tended to be more effective once they entered the teaching workforce.However, we are not aware of prior research that has considered similarly specific measures ofspecial educators’ student teaching experiences as predictors of their later effectiveness. Wetherefore investigated whether the specific measures of preservice preparation in our data set2
(e.g., cooperating teacher characteristics) predicted ELA achievement for students with highincidence disabilities taught by early-career special education teachers.Second, debates about the best way to teach reading—dubbed the “reading wars”(Pearson, 2004)—have raged for decades. These debates have historically pitted proponents ofphonics-based approaches (e.g., Chall, 1967) against those who advocate for language-basedapproaches (e.g., Goodman, 1967). To provide a more recent example, a special report on“Getting Reading Right” (Education Week, 2019) focused on the disconnect between the scienceand practice of reading instruction. The report concluded that the way in which reading istypically taught to early and struggling readers does not reflect “a settled body of research onhow best to teach early reading” (Schwartz, 2019, p. 1). Recognizing this perceived disconnectbetween science and practice, we decided to investigate the extent to which the literacyinstructional approaches emphasized by districts and TEPs predicted ELA achievement forstudents with high-incidence disabilities in Washington State.Finally, a considerable body of theoretical and qualitative work has argued that thealignment or “coherence” between a teacher candidate’s education experiences and inservicepractice is important (e.g., Darling-Hammond, 2000; Darling-Hammond et al., 2005; FeimanNemser & Buchmann, 1983; Grossman et al., 2008; Powell, 2015). These studies are bolsteredby a small body of quantitative literature that suggests that alignment between preparation andpractice may have important implications for teacher and student outcomes (e.g., Boyd et al.,2009; Goldhaber et al., 2017; Krieg et al., in press), although none of these papers contain aspecific focus on special education teachers. We are not aware of descriptive information aboutthe alignment between what is taught to special educators in their TEPs and what is asked ofthem once they become special education teachers, and the quantitative literature does not3
address why the alignment between a candidate’s student teaching experiences and early-careerteaching experiences might matter for teacher and student outcomes. We therefore used the TEPand district survey data to investigate the extent to which the literacy instructional practicesemphasized in special education TEPs and K–12 districts were aligned, and whether thisalignment predicted the ELA achievement of students with high-incidence disabilities taught byspecial education teachers.In summary, this study addressed four primary research questions (RQs), representedgraphically in the conceptual framework in Figure 1:1. To what extent do specific measures of preservice preparation (e.g., student teachingplacements, credentials, and licensure test scores) predict ELA achievement for studentswith high-incidence disabilities taught by early-career special education teachers?2. To what extent do the instructional approaches emphasized by districts and TEPspredict ELA achievement for students with high-incidence disabilities taught by earlycareer special education teachers?3. To what extent is there alignment between the literacy instructional practicesemphasized in special education teacher education programs and K–12 special educationsettings?4. To what extent does this alignment predict ELA achievement of students with highincidence disabilities taught by special education teachers?4
2. Data and Methods2.1 DataWe combined data from three sources for this study: data on teacher candidates collectedfrom the TEPs participating in the Teacher Education Learning Collaborative (TELC), 1 datacollected through surveys of special education TEP faculty and district special educationdirectors in Washington State, and data on K–12 students and teachers provided by OSPI.TELC DataThe broader TELC data set includes information from 15 of the state’s 21 college- anduniversity-based TEPs that were licensed to credential teachers during the years we studied, 13of which had special education endorsement programs. The data provided by these programsincluded information about teacher candidates themselves (e.g., race and gender) as well as dataabout when student teaching occurred, the schools and districts in which teacher candidatescompleted their student teaching, and the cooperating teachers who supervised their internships.Though many of the institutions in TELC provided student teaching data going back to the mid2000s (and in one case, to the late 1990s), we focused on student teaching data from 2009–10 to2017–18 in this analysis because—as described in the section on OSPI data—we were able to linkcooperating teacher information from these years to the students they taught, and use these as aproxy for the students candidates taught during their student teaching placement. Moreover,focusing on these more recent years of data made it more plausible that TEP faculty surveyTELC is a consortium of 15 TEPs providing data on student teaching experiences. The institutions that areparticipating in TELC and provided data for this study include Central Washington University, City University,Evergreen State College, Gonzaga University, Northwest University, Pacific Lutheran University, St. Martin’sUniversity, Seattle Pacific University, Seattle University, University of Washington Bothell, University ofWashington Seattle, University of Washington Tacoma, Washington State University, Western GovernorsUniversity, and Western Washington University. The six institutions that are not participating in TELC include onlyone relatively large (for Washington State) public institution in terms of teacher supply (Eastern WashingtonUniversity) and five smaller private institutions (Antioch University, Heritage University, University of PugetSound, Walla Walla University, and Whitworth University).15
responses (described in the next section) would represent the instruction provided to all candidatesin the sample. Following the work of Theobald, Goldhaber, Naito, and Stein (2021), this analysisfocused on graduates of special education endorsement programs at these 13 institutions (definedas graduating from one of these institutions with a special education endorsement).Survey DataWe designed and administered surveys to special education faculty from the 13 TELCinstitutions with special education endorsement programs, and we administered parallel surveysto special education directors of school districts in the state. The surveys were developed incollaboration with a team of special education TEP faculty and former special education districtadministrators. Questions were primarily derived from the Council for Exceptional Children’sInitial Level Special Educator Preparation Standards, but we also consulted an advisory board ofadditional TEP and district personnel to determine instructional practices that are currentlyemphasized for use with students with high-incidence disabilities in TEP coursework and schooldistricts.The surveys were initially piloted with out-of-state contacts during the 2017–18 schoolyear and were then administered during the 2018–19 school year. Faculty surveys wereadministered by e-mail to contacts within special education TEPs. Importantly, respondentscould opt out of any question and could provide contact information for another faculty memberbetter suited to answer a specific question. The final response rate across all questions in thissurvey and the 13 TEPs was 100%. District surveys were administered by project staff in personat meetings of special education district directors in the nine education service districts in thestate. Unlike the faculty survey, directors were required to respond to all questions in the survey(though in many cases, they completed the survey in consultation with other district6
administrators who were attending the meeting). We received complete district responses for82% of the candidates in the analytic sample.Though tangential to the primary analysis, we highlight one question from these surveysthat provides additional motivation for this study (Figure 2). Specifically, the TEP surveys askedfaculty about their perceptions of the extent to which their TEP graduates were prepared, onaverage, to perform a number of tasks required of special education teachers (e.g., superviseparaeducators and address challenging behaviors), while the district surveys asked directors thesame question about their incoming special education teachers. As shown in Figure 2, specialeducation TEP faculty members’ perceptions of the preparedness of their graduates weredramatically higher than directors’ perceptions of the preparedness of incoming specialeducation teachers. While perhaps not surprising, this finding provides some additionalmotivation for our focus on alignment in this analysis, given that one possible explanation forthese divergent perceptions is that early-career special education teachers are being asked to dosomething different from what they were taught in their TEPs.Although the survey contained additional questions addressing necessary knowledge andskills—such as classroom management and preparing individualized education programs—forthe purposes of this analysis, we primarily focused on one question that asked faculty anddirectors to select all literacy instructional practices for students with high-incidence disabilitiesthat were emphasized or used in their TEP or district. Because this question included a relativelylarge number of potential responses, we performed a factor analysis across TEP and districtsurvey responses to identify combinations of practices that tended to “go together,” and to reducethe dimensionality of the data. Table 1 summarizes the results of this analysis, which identifiedthree principal components with an eigenvalue of at least 1.0 (i.e., that explain more variation7
than the average principal component). 2 We labeled the three principal components “Phonics,Fluency, and Comprehension,” “Guided and Close Reading,” and “Balanced Literacy” to reflectthe instructional practices that load most positively onto these factors (all factor loadings with anabsolute value of at least 0.3 are bolded in Table 1). Interestingly, the five practices that loadonto the first factor—text comprehension, phonemic awareness, vocabulary, fluency, andphonics—are the five literacy instructional areas identified as evidence based by the NationalReading Panel (2000) and in follow-up research (e.g., Castles et al., 2018). We used the factorloadings in Table 1 to create measures of the extent to which each individual special educationteacher’s district and TEP emphasized the practices within each factor.OSPI DataWe merged the TELC and survey data with several sources of data on K–12 students andteachers maintained by OSPI. First, the state’s S-275 database provides annual employmentinformation for all public school employees in the state. We used this data set to identifyindividuals in public school teaching positions, teachers who had a master’s degree or higher,and teachers who were hired into the same district in which they student taught. Second, the S275 database can be linked to the state’s Credential and Endorsement database, which contains acomplete history of all teaching credentials (i.e., the credentials necessary for any public schoolteaching position), teaching endorsements (i.e., the subject areas teachers are endorsed to teach),and licensure test scores in the state. We used this database to identify whether candidates andtheir cooperating teachers held an endorsement in special education, another subject, or both; andto measure candidates’ performance on the state’s Washington Educator Skills Test – Basic(WEST-B) in mathematics, reading, and writing.The scree plot in Appendix Figure A1 also suggests that there is a “kink” in these eigenvalues after the thirdeigenvector, which suggests keeping three principal components in each subject.28
Finally, for 2009–10 through 2018–19 (the most recent year of available data), thesedatabases can be connected to the state’s Comprehensive Education Data and Research System(CEDARS), which allowed us to connect candidates both to the students they taught in theirstudent teaching (i.e., in their cooperating teacher’s classrooms) and to the students they taughtonce they entered the workforce. 3 The CEDARS database also allowed us to identify specialeducation teachers, which (following Theobald, Goldhaber, Naito, & Stein, 2021) we defined asteachers in classroom assignments in which at least 50% of students were receiving specialeducation services. The 50% cutoff is relatively arbitrary, but as the density distribution inFigure 3 shows, the classification of special education teachers was not terribly sensitive to thechosen cutoff as students with disabilities account for less than 40% or more than 90% ofstudents in the majority of classrooms in the state.The CEDARS database also allowed us to connect these special education teachers to thetest performance of students they taught. Students in Washington State take standardized testseach year in mathematics and ELA for Grades 3–8; we standardized these scores across allstudents in the state by grade and year and only considered ELA test scores for students whotook a test aligned with their current grade level. Because our analytic approach (described later)required both current and prior student test scores, our sample of teachers included specialeducation teachers who provided ELA instruction to students with high-incidence disabilities inGrades 4–8. The data also included student demographic information such as gender,race/ethnicity, and program participation. Most importantly for this analysis, the data allowed usCEDARS data include fields designed to link students to their individual teachers, based on reported schedules.However, limitations in reporting standards and practices across the state may result in ambiguities or inaccuraciesaround these links.39
to focus on students with high-incidence disabilities, which we defined as students with anemotional/behavioral disorder, health impairment, or specific learning disability. 42.2 Samples, Measures, and Summary StatisticsTable 2 provides summary statistics for all variables of interest across both of thesamples considered in this analysis. We observed 285 special education teachers (accounting for600 teacher-year observations) who appeared in the TELC data and provided ELA instruction tostudents with high-incidence disabilities. Of these, 243 teachers (and 506 teacher-yearobservations) were linked to survey responses from their TEP and district about the literacyinstructional practices emphasized in each. Column 1 of Table 2 summarizes the 10 variables ofinterest that were observed for all of these teachers. About two thirds of teachers in this samplehad a dual endorsement in special education and another subject, while slightly less than half hadat least a master’s degree. Teachers in this sample tended to score slightly higher on the WESTB mathematics test than the reading and writing tests.Turning to the student teaching variables, about 40% of the special education teacherswere teaching in the same district in which they student taught; this is comparable to estimatesfor all teachers in Washington State reported in Krieg et al. (in press). About 60% of specialeducation teachers in each sample did their culminating student teaching placement in specialeducation, while about 70% student taught with a teacher who had a special educationendorsement. Finally, when we considered the characteristics of candidates’ cooperatingteachers, we found that about 75% were supervised by a cooperating teacher with a master’sdegree, and that candidates’ cooperating teachers had 13 years of teaching experience onaverage. We then calculated summary statistics for these same measures and the principal4Summary statistics of these variables are provided in Appendix Table A1.10
component analyses (PCAs) derived from the TEP and district survey data for the subset ofcandidates who were linked to these survey data (column 2 of Table 2).2.3 Analytic ApproachOur research questions connect the variables of interest in Table 2, measured for specialeducation teachers in these samples, to the ELA achievement of students with high-incidencedisabilities taught by these teachers. We performed these analyses in two steps. First, weestimated first-stage “value-added” models (VAMs) across the full sample of special educationteachers who provided ELA instruction to students with high-incidence disabilities in the state.These models attributed student test score gains to specific teachers. We then used the resultingvalue-added estimates as the outcome variable in second-stage models that used the variables ofinterest described above to predict value added only within the samples in which we observedthese variables. One advantage of this approach is that we used the largest sample possible toremove variation due to student characteristics and prior test scores, classroom composition, andteacher experience that could confound our estimates.First-Stage Value-Added ModelsTo investigate the performance of students with high-incidence disabilities in ELA, weestimated VAMs that have been shown to produce unbiased estimates of the contributions ofindividual teachers to student test performance (e.g., Chetty et al., 2014) but are only rarelyapplied to special education teachers (e.g., Feng & Sass, 2013). One challenge unique to thespecial education context is that about 40% of students with high-incidence disabilities whoreceive ELA instruction from special education teachers also receive ELA instruction from ageneral education teacher. We therefore followed Chetty et al. (2014) and calculated the ELAvalue added of all general education teachers in the state (omitting the year each student in the11
sample was in these teachers’ classrooms) and included the general education teacher’s valueadded as an additional predictor of the performance of students with high-incidence disabilitiestaught by special education teachers. 5In addition to the general education teacher value added, the first-stage models controlledfor lagged student achievement, o
A program of research by the American Institutes for Research with Duke University, Northwestern University, Stanford University, University of Missouri-Columbia, University of Texas at Dallas, and University of Washington TRACKING EVERY STUDENT'S LEARNING EVERY YEAR Special Education Teacher Preparation, Literacy Instructional