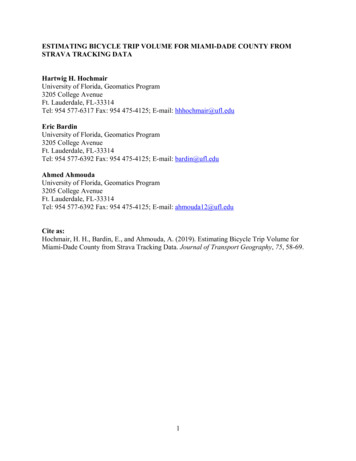
Transcription
ESTIMATING BICYCLE TRIP VOLUME FOR MIAMI-DADE COUNTY FROMSTRAVA TRACKING DATAHartwig H. HochmairUniversity of Florida, Geomatics Program3205 College AvenueFt. Lauderdale, FL-33314Tel: 954 577-6317 Fax: 954 475-4125; E-mail: hhhochmair@ufl.eduEric BardinUniversity of Florida, Geomatics Program3205 College AvenueFt. Lauderdale, FL-33314Tel: 954 577-6392 Fax: 954 475-4125; E-mail: bardin@ufl.eduAhmed AhmoudaUniversity of Florida, Geomatics Program3205 College AvenueFt. Lauderdale, FL-33314Tel: 954 577-6392 Fax: 954 475-4125; E-mail: ahmouda12@ufl.eduCite as:Hochmair, H. H., Bardin, E., and Ahmouda, A. (2019). Estimating Bicycle Trip Volume forMiami-Dade County from Strava Tracking Data. Journal of Transport Geography, 75, 58-69.1
ABSTRACTSports and fitness apps on GPS enabled cell phones and smart watches have become a rich sourceof GPS tracking data for nonmotorized traffic, including walking, running, and cycling. Thesecrowd-sourced data can be analyzed to better understand the cycling behavior of a large usercommunity. Using Strava tracking data from the Miami-Dade County area, this study identifieswhich transport network measures, characteristics of the built environment, and sociodemographicfactors are associated with increased or decreased bicycle ridership in census block groups. Forthis purpose, a set of linear regression models are estimated to predict non-commute and commutebicycle kilometers travelled per block group, as well as bicycle kilometers travelled on weekendsand weekdays. Eigenvector spatial filtering is applied to explicitly model spatial autocorrelationand to avoid parameter estimation bias. Results suggest that Strava data, due to its high spatialresolution and coverage, can identify in detail how the influence of explanatory variables onestimated bicycle trip volume varies between different trip purposes and days of the week. Basedon the regression results, the paper presents a set of guidelines for practical design detailing whichgroups of cyclists would benefit most from specific bicycle infrastructure improvements.Keywords: bicycle volume; tracking data; Strava; network analysis; eigenvector spatial filtering2
1. INTRODUCTIONLarge cycling volumes with sufficient spatial detail and temporal coverage are necessary tounderstand temporal fluctuations and spatial variation of bicycle ridership (Jestico et al. 2016).The growing prominence of fitness apps, such as Strava, Endomondo, or MapMyRide, which areoperated on smart phones, smart watches, or fitness bands, have increased the sampling size ofusers to the millions (Romanillos et al. 2016). These apps provide data coverage for a large areaon a continued basis, which makes app-based crowd-sourcing methods particularly suitable fortransportation planning tasks. Although crowd-sourced data have their drawbacks, including userselection bias or geographic bias, their sheer volume and coverage outweighs their disadvantagesfor many types of analyses. Crowd-sourced tracking data can, for example, supplement permanentcount stations that show long-term changes and trends in cycling frequency but lack spatial detail(Griffin et al. 2014).This study develops a set of linear regression models that explore how characteristics ofthe road network and the built environment and demographic variables are associated with noncommute and commute cycling volume and cycling volume on weekends and weekdays. Theanalysis is conducted at the census block group level in part of urban and rural Miami-DadeCounty. As tracking data source 2015 Strava Metro roll-ups from Miami-Dade County are used.This Strava product contains cycling activity counts per road segment summed up over a givenperiod of time. These counts can then be aggregated at the census block group level. This studyexpands the analysis of Strava data conducted in previous work (Griffin and Jiao 2015; Sanders etal. 2017; Conrow et al. 2018) in geographic coverage, the range of network related predictorvariables, and in analysis technique by integrating eigenvector spatial filtering (ESF) to handlespatial autoregression in residuals. Other studies conducted on the Miami-Dade road networkanalyzed, for example, the effect of road network architecture on observed automobile tripdistances (Parthasarathi et al. 2012) and on the shape and size of household activity spaces(Parthasarathi et al. 2015). Another study assessed the completeness of designated bicycle lanefeatures and off-road bicycle trails in the crowd-sourced OpenStreetMap (OSM) mapping platform(Hochmair et al. 2015). The presented study demonstrates that crowd-sourced GPS tracking dataare suitable to determine the association between the presence of local infrastructure features (e.g.bridges, bike parks) and cycling volume, and to identify distinct spatial patterns of commute, noncommute, weekday, and weekend cycling activities across the study area.2. LITERATURE REVIEWThe role of road network structure, bicycle facilities, and the built environment on cycling demandhas been studied for years. For this purpose, trip counts and cycling volume have been derivedfrom various data sources including household travel surveys, interviews, census data, fieldobservations, and more lately also GPS tracking data. One study that compared bicycle commuterdata between the 2000 and 2010 census at the block group level identified a significantly largerincrease in bicycle commuters in block groups that had on-road bicycle lanes installed during thestudy period compared to block groups with sharrows or no infrastructure installed (Ferenchak andMarshall 2016). Using data collected from household travel surveys and interviews, researchersalso used a combination of network-based roadway characteristics and contextual attributes of theneighborhood design to model cycling demand and travel choices. Results showed that block size,mixed land use, and bicycle friendly design are associated with the decision to ride a bike (Cerveroand Duncan 2003; Cervero et al. 2009). Using a combination of surveys, count data, and censusdata, previous studies also showed that the availability of bike lanes and higher levels of street3
connectivity are associated with more cycling (Dill and Voros 2007) and that adding new bicycleinfrastructure increases cycling ridership (Dill and Carr 2003; Parker et al. 2013).A detailed review of the history and growth in data-driven research on cycling is providedelsewhere (Romanillos et al. 2016). The review is divided into three groups according to the natureof data collection, which include GPS data (collected via smart-phone and other GPS enabledmobile devices), live point data (collected at a particular location such as from a traffic camera),and journey data (providing origin and destination locations and travel times for an individualjourney). The paper also provides user numbers for commonly used fitness apps that collecttracking data, including Endomondo, Strava, and MapMyRide. According to Strava’s annual Yearin Sport report, cyclists shared a total of 203 million rides on Strava totaling 7.3 billion km in20171. During that year Strava had over 144,000 commute cycling trips uploaded each week in theUnited States alone. Data from sports tracking apps have recently been used to identify the effectof various characteristics of the built and natural environment on bicycle ridership numbers,including bicycle lanes and slope (Griffin and Jiao 2015). A study from two cities in Europe(Amsterdam and Osnabrück) which analyzed cycling trips posted on the GPSies platform foundthat cyclists prefer to take longer routes with less tumultuous traffic and routes that serve as socialspaces (Sultan et al. 2017). The use of activity trackers can be motivated by a variety of tasks,including directive tracking (goal driven, e.g. to lose weight), documentary tracking (e.g. todocument special walks), or collective rewards (e.g. score points) (Rooksby et al. 2014).Crowdsourcing has a large potential to complement or even replace traditional methods ofcollecting trip data without cost (Misra et al. 2014).Crowd-sourced data generally lacks a formalized data quality assurance approachcompared to data from traditional collection sources, such as governmental data (Goodchild andLi 2012). In this context several papers address and acknowledge the issue of user selection biaswith data from sport tracking apps. That is, studies found the Strava user base to be skewed towardsmale cyclists, e.g. 84% (Watkins et al. 2016), 77 % (Jestico et al. 2016), and 80% (Heesch andLangdon 2016) compared to the general cyclist community, which was, based on census data,determined to be 71% male for bicycle commuters in Seattle (Sanders et al. 2017) and, based onhousehold surveys, determined to be 72% male for cyclists in Queensland, Australia (Heesch andLangdon 2016). That latter study also showed that Strava users are younger (69.4% under the ageof 45 years) than the general cyclist population (61.8% under the age of 45 years). Comparing thecharacteristics of several user samples from bicycle smartphone applications (CycleTracks, MonReso Velo, Cycle Lane, ORcycle) deployed in North America with survey cycling samples fromtravel survey data sets revealed that smartphone apps tended to undersample females, older adults,and lower-income populations and to oversample some minority ethnicity populations (Blanc etal. 2016).A comparison between the number of GPS-tracked commuters in Strava with AmericanCommunity Survey commuter counts revealed a Spearman’s Rho value of r 0.60, where thecorrelation was higher in high density population areas (Whitfield et al. 2016). Several studiesanalyzed how well fitness app data represent actual ridership through comparison with manualcycling counts, which typically produces r2 values ranging from 0.40 to 0.60 (Oksanen et al. 2015;Jestico et al. 2016). Previous research pointed out various systematic effects of user bias in bicycletracking data, such as location, land use, or socio-economic characteristics. For example, one studyfound higher matching rates (that is, lower rank differences) between manual and Strava counts indowntown areas (Conrow et al. 2018). The observed spatial patterns of varying matching try-news/strava-data-reveals-year-sport-2017/4
vary, however, considerably between the different studies, revealing a lack of consistency.Alternative indirect techniques of automated estimation of cyclist counts on road segments besidesGPS tracking data are currently being developed, and include the use of Google Street Viewimagery (Goel et al. 2018) among others. Automated extraction of count data from such methodscould be potentially used for comparison with Strava data and its calibration in the near future.3. STUDY SETUP3.1 Study area and data sourcesThe study area for this research comprises the Urbanized Area of Miami-Dade County, as well asadditional tracts of agricultural land and the built environment stretching into the southwesternpart of the county towards the Everglades National Park. Bicycle ridership is obtained from StravaMetro roll-ups which are available for different time periods. These data come in shapefile formatand aggregate cycling activities at the road segment level, where activity counts can be snapped todifferent road networks per request, such as OpenStreetMap (OSM). Strava data purchasedthrough the Florida Department of Transportation (FDOT), which were used in this study, weresampled to HERE NAVSTREETS road geometries. The roll-ups do not come with metadata aboutits contributors, such as percent male or age distribution. However, the Strava user selection biashas been estimated in studies that used other Strava data products, such as minute-to-minute coredata or origin-destination files, as described above. For the conducted study, three types of bicycleridership roll-ups are used:1. January-May 2015: All activities2. 1st Quarter 2015 (January-March): Weekend activities3. 1st Quarter 2015 (January-March): Weekday activitiesThese roll-up summaries are further broken down into total activities per segment, and commuteractivities per segment. FIGURE 1a maps the Strava total activity count per segment betweenJanuary and May 2015 for Miami-Dade County. According to the Strava Metro User Guide, Stravaderives commuter data through three methods: (1) a commute flag; (2) an automated process thatlocates point-to-point cycling trips that are within duration and distance constraints; (3) fuzzy namematching from the activity titles. Unfortunately, no more details about these three methods areprovided in the user guide. However, there are additional third-party tools, such as CommuteMarker, which helps Strava athletes to distinguish between commutes and other trip purposesduring their workouts, and which marks them on Strava automatically. Tools like this can help toincrease Strava tagging accuracy. Multiplication of Strava activity counts per segment by segmentlength and summing up these products in analyzed areal units results in bicycle km travelled (BKT)(Griffin and Jiao 2015). FIGURE 1b shows the result of this process based on total activity countsbetween January-May 2015 for the selection of 1527 census block groups in Miami-Dade Countythat were used for the different regression models in the presented study. Due to the observed userselection bias in Strava the resulting BKT measure does not represent overall community BKT.For this reason, we use from here on the term “Strava BKT” for cycling volume that is derivedfrom Strava activity counts.5
(a)(b)FIGURE 1 Strava January-May 2015 summary data: Activity counts on street segments (a)and derived bicycle kilometers travelled (Strava BKT) for analyzed census block groups (b).Sociodemographic data for the predictor variables were taken from the 5-year (2009-2014)summary of the American Community Survey (ACS) and job data were obtained from the CensusBureau LEHD Workplace Area Characteristics. The HERE NAVSTREETS 2015 1st Quarterdataset was used to compute network supply measures, such as the total length of local roads perblock group, and topological and morphological network measures, such as betweennesscentrality. Location data of on-road bicycle facilities, such as marked bicycle lanes, was obtainedfrom the Miami-Dade Transportation Planning Organization (TPO) and then updated by theauthors in spring 2016 using Google Maps Aerial View and Google Street View imagery. Thelocations of traffic signals were also obtained from the TPO.Participation inequality has been identified as a potential source of bias on trip counts(Oksanen et al. 2015). To assess whether in the analyzed block groups Strava BKT could havebeen heavily affected by a small group of very active users, the number of cycling activities perathlete were computed for each Strava road segment. Very high numbers would suggest thepresence of one or several exceptionally active Strava users. When considering roads with at leastone Strava activity, activities per athlete range between 1 and 108 (mean 2.48, median 1.67).More specifically, 26.6% of segments have exactly one activity per athlete, 1.8% have an averageof more than 10 activities per athlete, and only 0.07% have an average of more than 50 activitiesper athlete. Thus, roads with exceptionally active Strava users are rare in the analyzed study area.Visual inspection of the activity-to-athlete ratio on road segments (FIGURE 2a) does notreveal any distinct spatial pattern of high activity-to-athlete ratio segments. Instead, such segments6
appear on short, disconnected sections and do not extend over longer stretches, like it could beexpected with repeated rides of a cyclist along the same route. To assess whether those segmentswith a high activity-to-athlete ratio affect Strava BKT, the road segment with the highest activityto-athlete ratio was identified in each census block group and plotted against the Strava BKT valuefor that block group (FIGURE 2b). Visual inspection does not reveal any association betweenStrava BKT and maximum activity-to-athlete ratio values. Furthermore, statistical analysisconfirms a negligible positive correlation between those two variables (Pearson’s r 0.07, p 0.01).(a)(b)FIGURE 2 Activity-to-athlete ratio for road segments (a) and their maximum values withinanalyzed census block groups plotted against the census block Strava BKT (b).For an estimate as to how closely Strava resembles ridership patterns in the study area, Stravaactivity counts were compared to automated video imaging counts of cyclists for selectedstations in Miami-Dade County and nearby Broward and Palm Beach Counties. The count studywas administered through FDOT between October 27, 2016 and December 24, 2016 (FDOT2017). Considering different context land use zones (e.g. rural, suburban residential, state park)and facility types (e.g., sidewalk/no bicycle lane, paved shoulder, trail) FDOT selected 26 sitesfor data collection. Using count data in different directions (e.g. at split lanes) at selected sitesallowed for comparison with Strava activity counts at 32 sites (FIGURE 3). Video imagingcounts were performed on three days for each site, which included typically two weekdays and a7
weekend day. For comparison with Strava the average trip count on weekdays was used for eachobservation site. Whereas the FDOT data collection study also tested other count methods,including pneumatic tube, passive infrared, and Bluetooth, data quality assessment throughFDOT showed that automated video imaging was the most accurate method. As for Strava theroll-up between January and June 2016 was used for comparison, since this roll-up periodmatched the year of the FDOT data collection study. The correlation between the averagenumber of Strava activity counts per day (FIGURE 3a) and the average number of trips perweekday from the FDOT data collection study (FIGURE 3b) is moderate at r 0.55 (p 0.01).One plausible reason for inconsistencies in the relative order of count numbers between both datasources (leading to an imperfect correlation) is the limited observation time of about only twoweekdays for FDOT count data. Another plausible reason for the inconsistency is bias in Stravadata in the sense that the proportion of conducted bicycle trips tracked in the Strava app variesbetween locations, for example due to different demographics or different primary trip purposesin different regions.Comparison of FIGURE 3a and b shows that the average trip numbers per day aresignificantly higher for the video imaging method than for Strava, which can be expected sincenot each cyclist tracks his or her bicycle trip with the Strava app. This general difference inmagnitude aside, a visual comparison of average daily trip count pattern between the two sourcesdoes not reveal any systematic relative over- or underestimation (bias) in geographic sub-regions.However, the ratio of Strava to video count numbers is by a factor 6.6 larger for stations alongsegments with motorized traffic than for the three stations located on bicycle trails, which meansthat Strava (at least for this small set of available stations) underestimates off-road cycling countsrelative to other stations. A possible explanation is that the Strava user base is biased towardscyclists who aim to ride at a faster pace for exercise purposes. Since multi-use trails may becrowded with pedestrians, dog walkers, and slower bicyclists, they may have fewer Strava users.As opposed to our results, other studies showed that Strava matches reference count data better atsites located at shared-use paths (Sanders et al. 2017). Because the percentage of bicycle or multipurpose trails among the complete network mileage in the test region is small, no mathematicalcorrection factor for measured bicycle travel volume at the census block level was introduced forsubsequent Strava data analysis.8
(a)(b)FIGURE 3 Average number of cycling activities per day in Strava (based on data betweenJanuary and June 2016) (a) and average number of cyclist counts per weekday from videoimaging (based on two-day periods between October 27 and December 24, 2016) (b) for 32stations in South-East Florida3.2 Analysis methodThis research explores the relationship between bicycle ridership (R), characteristics of the roadnetwork (N) and the built environment (B), and sociodemographic variables (S). A stylizedrepresentation of this relationship in functional form can be given asR f (N, B, S)(1)where: R is bicycle kilometers travelled (Strava BKT) in a census block group (or the differencein z-scores of Strava BKT between two trip purposes or groups of days, respectively), N ismeasures of network characteristics within a block group (e.g. total length of arterial roads), B ischaracteristics of the built environment in the block group (e.g. presence of bridge over bay), andS is sociodemographic characteristics within a block group (e.g. total population). A set of four9
linear regression models is used to explore these relationships. Whereas the set of predictorvariables on the right side of Equation 1 is the same for the four models to make them comparable,the ridership variable on the left side changes as follows: Model 1: This model uses the five-month roll-up data (January-May 2015) to predict theStrava BKT for non-commute trips at the census block group level Model 2: This model uses data from the same roll-up to predict the Strava BKT forcommute trips Model 3: This model predicts the difference between the z-scores of non-commute StravaBKT and commute Strava BKT values at the block group level, using the same five-monthrollup data Model 4: This model predicts the difference between the z-scores of weekend Strava BKTand weekday Strava BKT values at the block group level, using January-March 2015weekend and weekday roll-up dataA fundamental assumption of regression analysis is residual independence. Incorporating spatialdata in nonspatial models typically results in residuals that exhibit spatial autocorrelation. Ignoringspatial dependence in spatial data can lead to coefficient estimation bias and biased standard errors,(Anselin 1988). Therefore, spatial autocorrelation needs to be explicitly modeled in statisticalanalysis (Tita and Radil 2009). In this case study, for each linear model an additional spatiallyfiltered linear model is estimated which uses eigenvector spatial filtering (ESF) to model spatialautocorrelation. ESF utilizes eigenvector decomposition to extract a set of eigenvectors from thespatial weight matrix that is incorporated in the numerator of the Moran’s I coefficient (Griffith2000). A spatial filter that comprises all relevant eigenvectors can then be used as an additionalpredictor variable in standard statistical techniques. The method involves the following steps(Chun and Griffith 2013; Thayn and Simanis 2013; Helbich and Arsanjani 2014): Extract the spatial weight matrix from neighborhood relations between all spatial unitswhich is used for the computation of Moran’s I. This requires a neighborhood definition,e.g. Queen’s case, and a standardization of the weight matrix, e.g. using C- or W-stylecoding Generate eigenvalues and eigenvectors from the spatial weight matrix Construct a candidate set of eigenvectors for which the ratio of eigenvalue/(maximumeigenvalue) 0.25 Regress candidate eigenvectors on the response (e.g. Strava BKT) through a stepwiseselection approach that minimizes the Akaike information criterion (AIC) Construct the spatial filter through a linear combination of significant eigenvectors as asingle explanatory regression variable.This spatial filter is used as a predictor in equation (1) and accounts for redundancy in thelocational information by providing a surrogate for potentially lacking explanatory variables. It istherefore expected to explain a considerable part of the variance in the Strava BKT distribution.4. EXPLANATORY VARIABLESThe list of explanatory variables that are considered for the regression models to predict bicyclevolume are drawn from related studies that model bicycle travel behavior (see TABLE 1).10
4.1Network measuresNetwork measures can be broadly categorized into four classes, which are hierarchy, topology,morphology and scale (Parthasarathi et al. 2013; Parthasarathi et al. 2015). Hierarchy measuresthe heterogeneity of a street network. Topology provides measures of network connectivity thatare based on elementary concepts of graph theory. Morphology describes the regularity of streetnetworks as well as their shape and fragmentation. Scale captures the network supply in a particulararea. This study applies measures from the last three categories.Network supply is measured as total road length per census block group, separated by local,collector, and arterial roads. Road categories were derived from the NAVSTREETS road data setafter removal of road segments that were not accessible to bicyclists (e.g. interstates, ramps, orclosed port areas). In addition to road length, all combinations of road classes (local, collector,arterial) and on-street facilities types (marked bike lane, paved shoulder, shared lane marking(“sharrows”)) were included as supply variables as well. FIGURE 4a maps for part of the studyarea the location of on-road bicycle facilities. Off-road network supply was measured as the lengthof off-road trails where motorized traffic was not permitted.Regarding network topology, the importance of an edge in a network can be characterizedby its centrality, for which many indicators exist, such as the betweenness centrality (Barthélemy2011). An edge with a high betweenness value represents a bridge-like connector between differentparts of the network (Lu and Zhang 2013). Different variations of network centrality have beenused to predict traffic counts in earlier studies (Lowry 2014). For this study the edge betweennesswas computed on the NAVSTREETS dataset under consideration of one-way restrictions usingthe R-package igraph. FIGURE 4b visualizes for Miami Downtown, Miami Beach and KeyBiscayne the obtained betweenness centrality values. A morphological measure used in the studyis the size of the street polygons in a block group, where a larger polygon means greater travelimpedance to circumvent the area.11
(a)(b)FIGURE 4 On-road bicycle facilities (a) and betweenness centrality (b) for part of theanalyzed road network.4.2 Characteristics of the built environment and sociodemographic variablesNon-motorized trips, especially recreational trips, tend to be gravitating around roads with pleasantcharacteristics, such as attractive scenery or green landscape. This is captured within the group ofbuilt environment variables which also includes other variables relating to location, such as themixed density index. Knowledge about the association of sociodemographic characteristics withbicycle ridership can help to better target bicycling campaigns to promote cycling in areas whichtend to show low ridership levels.Block groups that were missing any of the sociodemographic variables (e.g. medianhousehold income), were lacking roads necessary to derive network measures, or had no Stravaactivities, were excluded. After this step a total of 1527 block groups were retained for regressionanalysis out of the 1594 block groups from the original study area.12
TABLE 1 Explanatory variables considered for analysisVariableNetworkLocal/arterial/collector road [m]Local/arterial/collector road with bikelane/paved shoulder/sharrows [m]Walk/bike path [m]IntersectionsOperationalizationRelevanceTotal length of road segments in differentNAVSTREETS functional classesTotal length of road segments with differenttypes of bicycle facilitiesHigh street densities increase odds of cycling (Cervero et al. 2009);bicycle volume is higher on major roads (Hankey et al. 2012)Levels of bicycle infrastructure and rates of bicycle commuting arepositively correlated (Dill and Carr 2003; Buehler and Pucher 2012),more so for bicycle lanes than for sharrows (Ferenchak and Marshall2016)There is a positive association between the proportion of off-streetbicycle routes and bicycle ridership (Parkin et al. 2008)Cyclists avoid signal-controlled intersections (Menghini et al. 2010), butuse traffic signals for crossing major highways and turning (AultmanHall et al. 1997)Intersection density is positively associated with likelihood of cycling(Winters et al. 2010); cul-de-sacs increase cycling distances to transithubs (Hochmair 2015)Large and elongated street polygons increase network impedance,leading to lower vehicle kilometers traveled per individual commuter(Parthasarathi et al. 2012)A high value marks a path between separated regions (Barthélemy 2011)Total length of walk ways suitable for cyclingand off-road bicycle trailsNumber of controlled and uncontrolledintersections1/3/4 degree nodesNumber of cul-de-sacs, T-intersections, andintersections with more than three roadsEnclosed polygon [m2]Maximum polygon area enclosed by roadsBetweenness centralityMaximum betweenness centrality value on anyroad segmentBuilt environmentBicycle park (binary)Bay bridge (binary)Distance to CBD [m]Distance to ocean or bay [m]University (binary)Mixed density indexGreennessSociodemographicPopulationPresence of park with dedicated bicycle trailsBlock group polygon intersecting with bridgethat crosses the intercostal bayDirect distance between centroid and MiamiDade Central Business DistrictDirect distance between centroid and ocean orintercostal bayBlock group with centroid located within 1 milefrom university campus with 5000 studentsMDI (ED*RD)/(ED RD) where ED is workersper km2 and RD is housing units per km2Normalized Difference Vegetation Index(NDVI), derived from 30m resolution LandsatThematic Mapper 5 satellite imageryParks are associated with more cycling (Nola
ESTIMATING BICYCLE TRIP VOLUME FOR MIAMI-DADE COUNTY FROM STRAVA TRACKING DATA Hartwig H. Hochmair University of Florida, Geomatics Program 3205 College Avenue Ft. Lauderdale, FL-33314 Tel: 954 577-6317 Fax: 954 475-4125; E-mail: hhhochmair@ufl.edu Eric Bardin University of Florida, Geomatics Program 3205 College Avenue Ft. Lauderdale, FL-33314