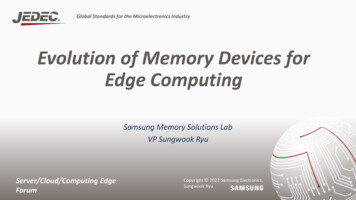
Transcription
Global Standards for the Microelectronics IndustryEvolution of Memory Devices forEdge ComputingSamsung Memory Solutions LabVP Sungwook RyuServer/Cloud/Computing EdgeForumCopyright 2022 Samsung Electronics,Sungwook Ryu
Contents Evolution of Computer Systems Domain-Specific Computing Computational Storage Processing In Memory (PIM) Summary
Evolution of Computer SystemsUbiquitousComputingEdge [1965-1985]CloudNetwork ClusterRack Mainframe[1960’s-1970’s]80’60’70’SCSI Disk[1981-present] nt]10’Cloud Storage (S3)[2006-present]HCI[2014-present]IT tech is swinging between Centralized and Decentralized Scalability and ManageabilityNetwork / Storage TechnologyNVMeOF, CXL FabricDisaggregation[2017-present]Domain SpecificComputing
Heavy Data Route in Hybrid Clouds4WAN Backbone Subsea cables Terrestrial Fiber DR replication13Last Mile Consumers Mobile devices Enterprise DCs6Data Center 75K machines per building 1 Pbit/s network bandwidth97Edge CDN Edge ExpressRoute2Internet Cable Cellular Network Internet ExchangeCluster Single cluster on asingle floor Consistent latency5Intra-region Regional network DC networks 3 availability zones per region8Rack Compute racks Storage racks Network racksServer Custom-made servers Compute server Storage server
IT Paradigm ShiftsClusterGoalRequirement1Cloud2Cost-Effective solution toLarge-scale applicationsOn-demand provisioningas a serviceEfficient networktechnologyH/W VirtualizationFrameworkEdge3Fast and reliable serviceswithout network bottleneckto Cloud Space and power constraints Low latency Flexible deploymentUbiquitousComputing4Data processing wherever isbest in terms of performance,power, and costDistributed device managementframework
Data Gravity is AcceleratingEdge ComputingMassive data is pulling Applications and ServicesHigh Egress ChargeAsymmetric Price PolicyFree IngressCloudSource: medium.com
Domain Specific ComputingGPU/TPU/NPUData in Any StatesData in UseCPU IntelAMDARMRISC-V NVIDIA, Intel GPUGoolge TPUXilinx DPUNPU for MobileData Processing Unitvs.Data in TransitData at Rest NVIDIA BlueField Broadcom Stingray Intel IPU Computational Memory/StorageSamsung PIMSamsung SmartSSDNGDScaleFlux
PerformanceToday’s Compute and Storage CompromiseCPUPerformance Ceiling# SSDs / ServerPerformance Limit:Storage to Host Server Ratio
Computational Storage ResolutionPerformanceScalable Accelerationwith SmartSSD2x – 10x# SmartSSD / ServerPerformance Enabled:SmartSSD local Compute
Computational Storage ExplainedComputational Storage ArchitectureClassic ArchitectureCPU OverloadedLarge datatransfersPerformanceMove computeto the dataOffloaded processes runnear the data high,scalable total internalbandwidth processing andbandwidth scales withdataPerformance Ceiling# SSDs / ServerPerformance Scales with Data
Computational Storage Architecture Feeding data directly from SSD toAccelerators using Peer-to-Peercommunication without CPUinterventionReducing CPU utilization Overall data movement TCOCPU (Host)SSDRead/WritePCIeSwitchPCIe Address SpaceCompute / cceleratorP2P communicationDRAMDRAMAccelerator
Value of In-storage Computingfrom Real ApplicationsCyber-forensicsFile CompressionData Warehouse18.8TBLewisRhodesLabs4.7x eticomSwarm64 270,6844TBXFSNoLoad SmartSSD Drive 100 TB Storage Appliance1 PB Storage RackSearch time 25 minutesSearch time 25 minutesSource: nal(TPC-C)Analytics(TPC-H)Source: greSQL 81,212Swarm64, PostgreSQL& SmartSSDAmazon RedshiftLegacy /Swarm64-SmartSSD-Overview.pdf
Using PIM to overcome memory bottleneckProcessing In Memory (PIM)Various bandwidth increase methods have beenproposed but it is Physically ImpossibleImproving performance and energy efficiency byReducing computing-memory data MovementDIMM basedsystemPhysically impossibleto increase # of CPU ballsand PCB wiresDevice basedsystemCPackage onPackageCCCBusMMCCCCCCCCCCCCMMMM
PIM System Level Evaluation PIM naturally complements xPUs for optimal system balance and performance per watt for memorybound workloads Speech Recognition, Natural Language Translation, Recommendation PIM system can deliver over 2X system performance while reducing energy consumption by more than70%.
DIMM-PIM ConceptCPU-memory data movement bottlenecks system performance rank-level parallelism is neededImprove the performance and energy efficiency of the system with in-DIMM processingUtilize higher in-DIMM bandwidth by multi-Rank parallel operation, 1.8x by 2-rankReduce data movement energy by utilizing in-DIMM data processing unit, -42.6% by 2-ranksRank0BufferDDDDDDRRRDDDDRank1DDRRRSystem Performance andEnergyDIMM-PIM Data erformanceNormal Data MovementEnergy Consumption 1.8xCPU RDIMMCPU DIMM-PIM(2-rank)42.6%CPU RDIMMCPU DIMM-PIM(2-rank)
SummaryEdge ComputingUbiquitous ComputingFrameworkDomain SpecificComputingBenefitSecuritySeamless software ecosystemForm Factor (Embedded/Rugged)Low PowerRequirementLow LatencyCost SavingFlexibledeployment
Normal Data Movement. Buff er. DD R. DD. DDR. R. DD R. DD. DDR. R. Rank0. Rank1. DIMM-PIM Data Movement. Nx BW. P H Y. DD R. DD R. DD R. DD R. R. DD R. Rank0. Rank1. PIM. PIM. DIMM-PIM Concept CPU-memory data movement bottlenecks system performance rank-level parallelism is needed Improve the performance and energy efficiency of the .