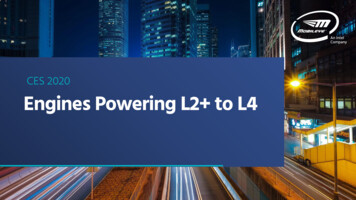
Transcription
CES 2020Engines Powering L2 to L4
Mobileye in NumbersEyeQ Shipped54MOverEyeQs shipped to date46% CAGRIn EyeQ shipping since 201433In 2019:DesignWins28M units over life4 high-end L2 wins with 4major EU and Chinese OEMs47RunningProgramsGlobally across 26 OEMs16ProductLaunchesIndustry first 100 camera with HondaVW high-volume launch (Golf, Passat)
Mobileye Solution PortfolioCovering the Entire Value Chain20252022TodayTodayL4/ L5Mobility-as-a-ServiceL2 / L2 L1-L2 ADASDriver assistanceFront camera SoC & SW:AEB, LKA, ACC, and moreConditional AutonomyScalable proposition forFront vision sensingFull AutonomyFull-Service provider-owningthe entire MaaS stackREM HD mapSDS to MaaS operatorsDriver monitoring, surroundvision, redundancySDS as a ProductMay also include:“Vision Zero”- RSS forADASData andREM MappingCrowdsourcing data from ADAS forHD mapping for AV and ADASProviding smart city eco system with Safety/Flow Insights and foresightsL3/4/5Passenger carsConsumers AutonomySDS to OEMsChauffeur modeScalable robotaxi SDS design fora better position in the privatelyowned cars segment
The ADAS SegmentVisual perceptionEvolution
L2 - The Next Leap in ADASThe opportunityL2 global volume expectation (M)Source: Wolfe research, 2019L2 common attributesMulti-camera sensingHD mapsMulti-camera frontsensing to full surroundtoRAGC63%3.6L2 functionalities range fromEverywhere,all-speedlane centring13L2 - significant added value in comfort, not only safetyEverywhere,all-speedconditional handsfree drivingHigher customer adoption and willingness to paySignificantly higher ASP- 3-15X more than legacy L1-L2System complexity leads to high technological barrier
Mobileye Scalable Solution for L2 Camera-based 360 sensing is the enabler for the next leap in ADAS360 cameras sensor suiteAffordability allows massadoption in ADASFull 3D environmental modelAlgorithmic redundancyLean computeplatformREM HD MapsFirst in the industry to offer:“HD Maps Everywhere”High refresh rateEntire system running on 2xEyeQ 5H3rdparty programmability46 TOPS, 54WDriving Policy layerRSS-basedFormal safety guaranteesPrevention driven system forADAS
L2 Business StatusMore than 70% of the L2 systems running today are powered by Mobileye’s technologyFor example:Nissan ProPilot 2.0VW Travel Assist Cadillac Supercruise Additional 12 active programs with L2 variants and 13 open RFQsBMW KaFAS 4
Next Generation ADASUnlocking “Vision Zero” with RSS for Humans DriversADAS TodayADAS Future PotentialAEB, LKA Emergency drivenESC/ ESP Prevention drivenAEB, LKA, ESC All in oneApplication of brakeslongitudinally & laterallyPrevention driven systemFormal GuaranteesVision ZeroScalable surroundCV systemRSS Jerk-boundedbraking profilelongitudinal & lateralStandard fitment/Rating
Under the Hood of Mobileye’sComputer Vision
The MotivationBehindSurround CVThegoalWhyFull stack camera only AV10 4MTBF for sensing mistake leading to RSS violation(per hour of driving) 10 4 Humans probability of injury per hour of driving 10 6 Humans probability of fatality per hour of drivingsensing system desired MTBF (with safety margins) 10 7 TheDriving 10M hours without a safety critical errorTo meet the 10 7MTBF, we break it down into two independent sub-systems:MTBF 107 MTBF1103.5 MTBF2103.5Critical MTBF of 104 10,000 (with safety margins) hours is plausible.Thechallenge10 4 MTBFstill requires an extremely powerful surround visionEquivalent to driving 2 hours a day for 10 years without a safety critical sensing mistake
Mobileye’s Sensing has Three Demanding CustomersSensing state for Driving Policyunder the strict role ofindependency and redundancy.Smart agent for harvesting,localization and dynamicinformation for REM based mapADAS products workingeverywhere and at all conditionson millions of vehicles
Comprehensive CVEnvironmental ModelFour General CategoriesRoad SemanticsRoad-side directives (TFL/TSR),on-road directives (text, arrows,stop-line, crosswalk) and theirDriving Path (DP) association.Road BoundariesAny delimiter/ 3D structure/semantics of the drivable area, bothlaterally (FS) and longitudinally(general objects/debris).Road Users360 degrees detection of anymovable road-user, and actionablesemantic-cues these users convey(light indicators, gestures).Road GeometryAll driving paths, explicitly /partially / implicitly indicated, theirsurface profile and surface type.
Redundancy inthe CV SubsystemIn order to satisfy an MTBFof 10 4 hours of driving ofthe CV-Sub-system:This creates internal redundancy layers for both detection and ebasedGeometrybasedMultiple independent CV enginesoverlap in their coverage of the fourcategoriesMeasurementsDetection2DRobust Sensing3D
Object DetectionGenerated and solidified using 6 different enginesScene segmentation (NSS)VIDARDetection3DVDFull image detectionWheelsTop View FS
2D to 3D ProcessGenerated and solidified using 4 different enginesVIDARVisual road modelMeasurements2DRange Net3DMap world model (REM)
Full Image DetectionTwo dedicated 360-stitching engines for completeness and coherency of the unified objects map:Vehicle signatureVery close (part-of) vehicle in field of view: face & limitsFront right camRear right camFront right camRear right cam
Inter-cameras trackingObject signature network
Range NetMetric Physical Range estimationdramatically improve measurementquality using novel methodsRangeRange net outputTraditional classifier outputFrame
Pixel-level SceneSegmentationRedundant to the object-dedicated networksCatches extremely-small visible fragments of road users;Used also for detecting “general objects”.
Surround SceneSegmentation withInstanceFront leftFront rightRear leftRear right
Road Users – open doorUniquely classified , as it is both extremely common, critical,and of no ground intersection
Road Users - VRUBaby strollers and wheel chairs are detected througha dedicated engine on top of the pedestriansdetection system
Parallax NetParallax Net engine provides accurate structure understanding by assessingresidual elevation (flow) from the locally governing road surface (homography).
VIDAR“Visual Lidar”: DNN-based Multi-view StereoRedundant to the appearance and measurement engineshandling “rear protruding” objects – which hover above theobject’s ground plane.
VIDAR InputFront leftRear leftFront rightMainParking leftParking rightRear right
VIDAR OutputDNN based multi-view stereo
VIDAR OutputDNN based multi-view stereo
Road Users from VIDARLeveraging Lidar Processing Module for StereoCamera Sensing – “VIDAR”Dense depth image from VIDARHigh-res Pseudo-LidarUpright obstacle ‘stick’extractionObject detection
ObstacleClassificationObstacle classificatione.g., how to differentiate a doubleparked car from a traffic jamUsing cues from theenvironment Behavior of other road usersWhat’s in front of the objectObject locationOpened doorEmergency lightsVisual perception
Road Users SemanticsHead/pose orientationPedestrians posture/gesture.Vehicle light indicatorsEmergency vehicle/Personnel classification.Emergency vehicle , light indicatorsPedestrian understanding
Road Users SemanticsPedestrian Gesture UnderstandingCome closerYou can passStop!On the phone
The full unedited 25min ride is availableat Mobileye’s YouTube Channelhttps://www.youtube.com/watch?v hCWL0XF f8Y&t 15s
REM Mapping and Data
REM Process1. Harvesting2Collecting road andAnonymizing andlandmarks throughEyeQ-equipped vehiclesencrypting REM data3. AggregationGenerating HDcrowdsourcedRoadBook forautonomous drivingAlso available via retrofit solutions45. LocalizingMap tile distributedLocalizing the carto the carwithin 10cm accuracyin the road book.
REM VolumesHarvesting agreements with 6 major car makersHarvesting:Over 1M Harvesting vehicles in EU by 2020Harvesting volumesOver 1M Harvesting vehicles in US by 2021Collecting 6 million km per day from14Mserial production vehicles12Msuch as:10MVolkswagen Golf, Passat, BMW 5 series, 3 series, Nissan Skyline, and more8MLocalization:3 additional major OEMsPrograms for using Roadbook for L2 :6M4M1M2 OEMs2 OEMs2 OEMs20182019202020212022
REM-data AggregationRSD Coverage Global Snapshot
REM MilestonesMapping all of Europe by Q1 2020Mapping most of the US by end2020
REM for Autonomous DrivingAlready operational and is provingto be a true segment game changer45For roads above 45 MphMaps created in a fully automatedprocess TODAYContains all static, dynamic, and semanticlayers to allows fully autonomous driveMphFor roads below 45 MphSemi-automated processFull automation in 2021Las Vegas Fwy, interstate 15 REM map
REM in ChinaData harvesting agreements in China complying with regulatory constraintsStrategic collaboration with SAIC Motor for REM data harvestingAccelerate the AV development for passenger vehicles in ChinaHarvesting data in China as part of a collaboration with NIO on L4synergy for Robotaxi and consumer AVJV agreement with Unigroup to enable the collection, processing,and monetization of data in China
The Smart CitiesOpportunity
Mobileye Data ServicesProduct PortfolioInfrastructureAsset InventoryAutomated, AI-poweredroad asset surveyingEfficient asset management,precise GIS data and changedetectionStrategic collaboration withOrdnance Survey (UK)Pavement ConditionAssessmentAutomated surveying &assessment of road conditionsEfficient road maintenancewith precise GIS data ofsurface distressDynamic MobilityMappingNear real-time & historicaldata on movement in thecity; dynamic mobility GISdatasetsEvidence-based urbanplanning improvements
Infrastructure Asset Inventory
Pavement Conditions Assessment5 levels score0 – Excellent conditions - requires no repairRoad Conditions Score – Poor (5)
Pavement Conditions AssessmentCracks and potholes harvester in actionRoad Conditions Score – Poor (5)
Pavement Conditions AssessmentCracks and potholes harvester in actionRoad Conditions Score – Poor (5)
Pavement Conditions AssessmentCracks and potholes harvester in action
Visual perceptionRSS Driving Policy and Driving Experience
The Driving Policy Challenge Do we allow an accident due to a “lapse of judgement” of Driving Policy? Should the occurrence of “lapse of judgement” be measured statistically?Safety is a technological layer living outside of MachineLearning. It is like “Ethics” in AI - a set of rules. It all boils down to a formal definition of “what it means to be careful”There is a need for “regulatory science andinnovation”. Technological innovation is not sufficient.
What is RSS?A formal model for safety, that providesmathematical guarantees for the AV to nevercause an accidenthttp://arxiv.org/abs/1708.06374The Method01Defining reasonable boundaries on thebehavior of other road users02Within the boundaries specified by RSS, onemust always assume the worst-case behaviorof other agents03The boundaries capture the common sense ofreasonable assumptions that human driversmake04Any action beyond the defined boundaries isnot reasonable to assume
For ExampleEgo car A is following car B on a single-lane straight roadAThe GoalThe ImplementationThe PolicyThe GuaranteesBEfficient policy for A that guarantees not to hit B in the worst-caseSafe distance for A to not hit B in the worst-case – under areasonable assumption on V b max brakeDefine Dangerous Situation- a time is dangerous if the distance is non-safeDefine Proper Response- as long as the time is dangerous, brake until stopProof by inductionMore complex situations (n agents) need to prove “no conflicts” (efficiently verifiable)
More Complex SituationsRSS sets the boundaries of reasonable assumptions for all driving scenariosWhat is reasonable to assume on B in the scenarios belowMultiple GeometryLateral ManeuversIf B can brake at Bmin brakewithout violating right-of-way, B willbrake, otherwise A must stopIf B can brake at B lat min brake ,B will brake laterally, otherwiseA must brake laterallyOcclusionsAssuming the max velocity of B dictates the max speed for ABBAAAB
In SummaryAssuming cooperative behavior on theroadway is the key for drivability and“human-like” drivingFormal definition of the “reasonableassumptions” provides mathematicalguarantees for safetyThe parameters dictates thecautiousness and utility tradeoff andallow transparent and conciseregulatory frameworkThe RSS adheres to 5 principles:0102030405Soundness- full compliance with common senseof human drivingCompleteness- covering all driving scenarios by alwaysassuming the worst case under the reasonable assumptionsUsefulness- Policy for efficient and not overlyconservative drivingTransparency- The model should be a white-boxEfficiently Verifiable- proof of guarantee byinduction, insuring no butterfly effect
Industry AcceptanceThe RSS is gaining global acceptance as an Automated Vehicle Safety StandardPreviously announced adoptionsof RSS:Safety First for Automated Driving(SaFAD)IEEE to define a formal model forAV safety with Intel-Mobileyeleading the workgroupCompanies involved are:BMW, Daimler, Audi, VW, FCA, Aptiv,Continental, here, Baidu, InfineonTogether with 11 industry leaders, weestablished an industry-widedefinition of safety with the SaFADwhite paper, based on RSS definitionsThe new standard will establish aformal mathematical model for safetyinspired by RSS principles
Industry AcceptanceThe RSS is gaining global acceptance as an Automated Vehicle Safety StandardChina ITS Industry Alliance (C-ITS) toformally approve an RSS-based standardThe standard, “Technical Requirement of Safety Assurance of AV DecisionMaking”, has been released to public and will take effect on March, 2020 The world’s first standard, based on RSS Proof point that RSS can handle one of the world’s most challenging drivingenvironments: China The world’s first proposed parameter set that defines the balance between safetyand usefulness
The Path to Becoming an End-to-EndMobility-as-a-Service Provider
MaaS Business StatusMobileye is forging driverless MaaS as a near term revenue-generating channel The JV to bring robotaxi MaaS to TelAviv is officially signed Deploying and testing in Tel-Avivduring this year Establishing the regulatory frameworkin Israel This year Mobileye will start using Nio ES8 for AVtesting and validation In 2022 launching a next-gen platform withMobileye’s L4 tech offered to consumers in China Robotaxi variant will be launched exclusively forour robotaxi fleets RATP and Mobileye partnered with the City ofParis to deploy a driverless mobility solution The first EU city where testing withMobileye’s AV will start this year Daegu City and Mobileye announce today apartnership to start testing robotaxi MaaS inSouth Korea this year Deployment during 2022
Our Self-Driving-SystemHW GenerationsEPM 6EPM 59EPM 52 In deployment Up to 2x EQ5H Up to 7x8MP 4x1.3MPUp to 48 TOPs Deployment in 2023 Single EQ6H to support E2Efunctionality Deployment in Q2 2020 Up to 6x EQ5H Additional 2-3 for FOP Additional EQ6H FOPUp to 220 TOPs E2E support in all aspects- fusion, policy, controlUp to 216 TOPs
Main Takeaways01L2 a growing new category for ADAS where Surround-CV unlocks considerable value atvolume production cost.02Realization of (safe) L4 and unlocking the full potential of L2 requires Surround-CV at astandalone (end-to-end) quality03L2 required HD-map-everywhere at growing use-case (types of roads)L4 requiresHD-mapsConsumer-AV requires HD-maps-everywhereAutomation at scale isenabled by crowd-sourced data (REM)04Crowd-sourced data from ADAS-enabled vehicles (REM) unlocks great value for Smart Cities05To unlock the value of automation there is a need for “regulatory science” (RSS)06The road to Consumer-AV goes through Robotaxi MaaS
Thank You!
Full Autonomy. Full-Service provider-owning the entire MaaS stack. SDS to MaaS operators. SDS as a Product. 2025 L3/4/5 Passenger cars. Consumers Autonomy. SDS to OEMs. Chauffeur mode. Scalable robotaxi SDS design for a better position in the privately owned cars segment Crowdsourcing data from ADAS for . HD mapping for AV and ADAS