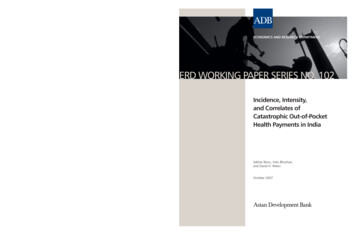
Transcription
About the PaperSekhar Bonu, Indu Bhushan, and David H. Peters write that despite posting morethan 8% annual GDP growth in the past few years, the catastrophic health paymentshave not changed significantly in India. Around 39.5 million people fell below thepoverty line in India due to out-of-pocket health payments in 2004–2005. Policiesto reduce poverty in India need to include measures to reduce catastrophic out-ofpocket health payments.Economics and Research DepartmentAbout the Asian Development BankADB aims to improve the welfare of the people in the Asia and Pacific region,particularly the nearly 1.9 billion who live on less than 2 a day. Despite manysuccess stories, the region remains home to two thirds of the world’s poor. ADB isa multilateral development finance institution owned by 67 members, 48 from theregion and 19 from other parts of the globe. ADB’s vision is a region free of poverty.Its mission is to help its developing member countries reduce poverty and improvetheir quality of life.ERD Working Paper Series No. 102ADB’s main instruments for helping its developing member countries are policydialogue, loans, equity investments, guarantees, grants, and technical assistance.ADB’s annual lending volume is typically about 6 billion, with technical assistanceusually totaling about 180 million a year.Incidence, Intensity,and Correlates ofCatastrophic Out-of-PocketHealth Payments in IndiaADB’s headquarters is in Manila. It has 26 offices around the world and morethan 2,000 employees from over 50 countries.Sekhar Bonu, Indu Bhushan,and David H. PetersOctober 2007Asian Development Bank6 ADB Avenue, Mandaluyong City1550 Metro Manila, Philippineswww.adb.org/economicsISSN: 1655-5252Publication Stock No. 100507 0100 5073 Printed in the Philippines
ERD Working Paper No. 102Incidence, Intensity, and Correlatesof Catastrophic Out-of-Pocket HealthPayments in IndiaSekhar Bonu, Indu Bhushan,andDavid H. PetersOctober 2007Sekhar Bonu is Senior Urban Development Specialist in the South Asia Regional Department, Asian Development Bank;Indu Bhushan is Chairman of the Health Community of Practice and Director of the Pacific Regional Department,Asian Development Bank; and David H. Peters is Associate Professor at the Johns Hopkins Bloomberg School ofPublic Health. The authors thank the National Sample Survey Organization of India for giving access to the data,and Rana Hasan for valuable feedback on an earlier draft.
Asian Development Bank6 ADB Avenue, Mandaluyong City1550 Metro Manila, Philippineswww.adb.org/economics 2007 by Asian Development BankOctober 2007ISSN 1655-5252The views expressed in this paperare those of the author(s) and do notnecessarily reflect the views or policiesof the Asian Development Bank.
ForewordThe ERD Working Paper Series is a forum for ongoing and recently completedresearch and policy studies undertaken in the Asian Development Bank or onits behalf. The Series is a quick-disseminating, informal publication meant tostimulate discussion and elicit feedback. Papers published under this Seriescould subsequently be revised for publication as articles in professional journalsor chapters in books.
ContentsAbstractI. INTRODUCTION1 II.DATA AND METHODS2 A.Study Setting and DataB.Dependent VariablesC.Independent VariablesD.Statistical Methods2233 III.RESULTS6 A.Bivariate AnalysisB.Multivariate Analysis611 IV.DISCUSSION17REFERENCES21
AbstractThis study investigates the incidence, intensity, and correlates of catastrophichealth payments in India. The paper confirms the continuing high incidence ofcatastrophic health payments and increase in poverty headcount and poverty gapdue to health payments. Despite India’s remarkable economic growth, catastrophichealth spending remains a major cause of poverty. Using bivariate analysis andHeckman sample selection and multinomial logistic regression for multivariateregression analysis, the paper finds that health payments were 4.6% of totalhousehold expenditure and 9.7% of household nonfood expenditure. Povertyheadcount increased from 27.5% to 31.0% due to health payments, whichtranslates to 39.5 million people falling below the poverty line due to healthpayments. It is important for India to develop effective risk pooling arrangementsfor health care.
I. INTRODUCTIONDespite buoyant economic growth in the past decade, India continues to have the world’slargest population—approximately 350 million or 35% of the population—living below 1-a-dayincome (World Bank 2006, Asian Development Bank 2007). Poor health, high health care expenses,high-interest private debt, and large social and customary expenses constitute 85% of the reasonsfor household’s declining into poverty (Narayan et al. 2000, Krishna 2004). In the last few years,a number of studies have explored the incidence and intensity of catastrophic out-of-pocket (OOP)health payments in Asia (Peters et al. 2002, Xu et al. 2003, Wagstaff and van Doorslaer 2003, O’Donnell et al. 2005, van Doorslaer et al. 2005, van Doorslaer et al. 2006 and 2007). These studieshave demonstrated widespread incidence of catastrophe and impoverishment from health paymentsall across South Asia.Studies have identified that too much reliance on OOP health payments at the time of care,in a health care financing context dominated by private expenditures combined with weak publichealth systems, and almost negligible health insurance are largely responsible for high prevalenceof catastrophic health payments in South Asia (Peters et al. 2002; Xu et al. 2003; Wagstaff andvan Doorslaer 2003; O’ Donnell et al. 2005; van Doorslaer et al. 2005, 2006, and 2007). The Equityin Asia-Pacific Health Systems (EQUITAP) project in particular has generated a very useful body ofevidence on catastrophic payments for health care, among others, for India (see O’ Donnell et al.2005; van Doorslaer et al. 2005, 2006, and 2007; Garg and Karan 2005). However, much of thisevidence on India has been generated from the National Sample Survey for the year 1999–2000.Meanwhile, as India has had buoyant economic growth rates, and the Indian economy hasbeen undergoing major structural changes (World Bank 2006), there is growing concern that India’seconomic growth is aggravating relative income inequalities (Asian Development Bank 2007). Thechallenges for poverty eradication in India remain formidable. Eradicating poverty in India needsinclusive economic growth and measures to prevent people, both below and above the poverty line,from getting impoverished due to catastrophic events.This study uses the 61st round of the National Sample Survey (NSS) data from 2004 to 2005 toinvestigate and update the evidence on incidence, intensity, and correlates of catastrophic healthcare payments in India. In addition, the study investigates the correlates of households that fallbelow the poverty line due to health payments. Given the rapidly changing economic context andconcerns over increasing relative inequalities in India, the study, along with evidence generatedfrom the EQUITAP project, should provide robust empirical basis for policy and program initiativesto mitigate the impoverishing effects of health payments in India.
Incidence, Intensity, and Correlates of Catastrophic Out-of-Pocket Health Payments in IndiaSekhar Bonu, Indu Bhushan, and David H. PetersII. DATA AND METHODSA. Study Setting and DataThe 61st round of the NSS conducted from July 2004 to June 2005 is the seventh quinquennialseries of consumer expenditure surveys. The NSS followed a stratified multi-stage design (NationalSample Survey Organization 2006). The sample covers most of India, consisting of 124,644 householdsspread over 7,999 villages and 4,602 urban blocks. The sample survey used both uniform recall period(30-day reference recall for all items) and mixed recall period (30-day reference recall for all exceptfive infrequently purchased nonfood items, namely, clothing, footwear, durable goods, educationand institutional medical expenses). This study used data from the uniform recall period.Health payments were derived from expenditures on institutional care, noninstitutional care,and therapeutic appliances. Both health expenditures on institutional (code 410 to 414) andtherapeutic appliances (code 620 and 621) were collected using the 1-month recall period. Dataon noninstitutional expenditures (codes 420 to 424) were collected using a 1-month recall period.The institutional (in-patient) health payments include expenditures on medicines, diagnostics,doctor’s fees, hospital and nursing home charges, and other medical expenses. The non-institutional(out-patient) health payments include expenditures on medicine, diagnostics, doctor’s fees, familyplanning, and other medical expenses. The expenditures on therapeutic appliances include hearingand orthopedic equipment and other medical equipment.B.Dependent Variables1.Poverty HeadcountWe measure the fraction of people living below the poverty line before health payments(Hpre) and the fraction of people living below the poverty line after health payments (Hpost). Thedifference between postpayment headcount ratio and prepayment headcount ratio gives the povertyimpact (PIH) in terms of poverty headcount of health payments (World Bank 2002a, World Bank2000b, Wagstaff and van Doorslaer 2003). The poverty line is not changed but the fraction of peopleliving below the poverty line is recalculated after removing per capita health payments from percapita expenditures as shown below:H1Poverty impact (PIH) Hpost - Hpre where pre n 1 pi ( pre ) and n is the sample size, and Pi 1 ifper capital household expenditure is less than the poverty line and “0” if it is otherwise.2.nPoverty Payment GapTo assess the intensity of poverty, we assess the poverty gap. Poverty gap (G) is the average1 n pi (PL x1 ) wheren 1shortfall of consumption below poverty line, and is estimated as follows:n is the sample size, PL is the poverty line, Pi 1 if xi PL and is zero otherwise (World Bank 2002aand 2000b, Wagstaff and van Doorslaer 2003).G October 2007
Section IIData and Methods3.Incidence of Catastrophic Health PaymentsIncidence of catastrophic health payments is the fraction of households whose healthpayments as a proportion of household consumption expenditure exceed a particular threshold ofoverall household expenditure or household nonfood expenditure. Consistent with the literature oncatastrophic health payments, (Berki 1986; Merlis 2002; Xu et al. 2003; Wagstaff and van Doorslaer2003; O’ Donnell et al. 2005; van Doorslaer et al. 2005, 2006, and 2007; Wyszewianski 1986a), thestudy uses two different cutoff points to define catastrophic health payments.C.(i)Catastrophe-1: Health payments over 10% of overall household consumptionexpenditure(ii)Catastrophe-2: Health payments over 40% of nonfood consumption expenditureIndependent VariablesThe independent variables are modeled based on literature on social, cultural, political, andadministrative aspects specific to India and health expenditure (Bonu et al. 2005, Kawabata et al.2002, Berki 1986, Xu et al. 2003, Su et al. 2006, O’ Donnell et al. 2005). States in India have animportant role in the provision of health services. Over the past decades, the states have evolveddifferent grades of governance and public service provision. Religion- and social group-baseddifferences in access to health services have been previously recorded (Bonu et al. 2005). Formultivariate analysis, we use log of monthly household consumption expenditure.D. Statistical MethodsWe first ran univariate analysis to assess the distribution of the sample (Table 1). Bivariateanalysis was done to find the association of various independent variables. Three different modelswere used for regression analysis of seven outcome variables as follows:(i) Heckman sample selection linear regression for (A) log of household health payments;(B) health payments proportion of total household expenditures; and (C) health paymentsproportion of household nonfood expenditures.(ii) Heckman sample selection probit mode for (D) correlates of Catastrophe-1; and (E) correlatesof Catastrophe-2.(iii)Multinomial logit model for (F) correlates of household above the poverty line that remainabove the poverty line despite health payments compared to households above the povertyline that had no health payments (reference group); and (G) correlates of householdsabove the poverty line that fell below the poverty line due to health payments comparedto households above the poverty line that had no health payments (reference group).Heckman sample selection model was used since the number of households that had no healthpayments was significant (38% of the households had no health care payments). Heckman selectionmodel is based on the notion that some of the independent variables that determine decisions toseek health care and health payments are different from the independent factors that are associatedwith scale of health payments (Diehr et al. 1999, World Bank 2002c, Baum 2006).ERD Working Paper Series No. 102
Incidence, Intensity, and Correlates of Catastrophic Out-of-Pocket Health Payments in IndiaSekhar Bonu, Indu Bhushan, and David H. PetersTable 1Distribution of the Sample and Bivariate AnalysisBivariate uralStateUnion TerritoriesJammu and KashmirHimachal PradeshPunjabUttarakhandHaryanaDelhiRajasthanUttar PradeshBiharNorth-East statesincluding AssamWest BengalJharkhandOrissaChattisgarhMadhya PradeshGujaratMaharashtraAndhra PradeshKarnatakaGoaKeralaTamil NaduHousehold Size1 to 45 or more than 5Consumption holdHealthPaymentMonthly Household Health Paymentas Percent of Household:Monthly TotalExpenditureMonthly NonfoodExpenditureIncidence ofCatastrophe-1Incidence 16.16.7continued. October 2007
Section IIData and MethodsTable 1. continued.Bivariate 0Richest decile10.0Household Type (Rural–Agricultural –agricultural labor19.4Rural–other ��self-employed10.3Urban–regular wage/salary-earning11.2Urban–casual ian2.4Others3.0Social GroupScheduled tribes8.8Schedules castes19.7Other backward castes40.2Others31.4Head - EducationIlliterate37.6Literate but primary10.1Primary14.4Middle15.5Secondary/Sn. Secondary14.5Diploma/Degree7.9Age Category of Head of Household15 to 29 yrs16.530 to 44 yrs41.145 to 5925.2 60 years17.2Institutional Health PaymentNo98.6Yes1.4Noninstitutional Health onthly Household Health Paymentas Percent of Household:Monthly TotalExpenditureMonthly NonfoodExpenditureIncidence ofCatastrophe-1Incidence 78.34.360.4342970.47.30.515.30.820.70.67.8ERD Working Paper Series No. 102
Incidence, Intensity, and Correlates of Catastrophic Out-of-Pocket Health Payments in IndiaSekhar Bonu, Indu Bhushan, and David H. PetersThe model is a two equation model. First, there is a selection model, y v β u1 . Second,there is a regression model, zγ u2 0 where the following hold:u1 N(0, 1)u2 N(0, σ )corr (u1 , u2 ) ρ(1)When ρ 0 ordinary least square regression provides unbiased estimates; when ρ 0 theordinary least square estimates are biased. The Heckman selection model allows use of informationfrom households that do not seek health care or do not have any health payments to improve theestimates of the parameters of the regression model.The study also uses multinomial logit regression to test the correlates of households (p 1)above the poverty line that fall below the poverty line due to health payments, and households thatdespite health payments remain above the poverty line (p2). Both are compared with householdsabove the poverty line that do not have any health payments (p3). For the three-category dependentvariable, the multinomial logit regression model in this study was expressed with two log-linearfunctions as follows:log (p1/p3) β10 β11*X1 β12*X2 β1j*Xr,(2)andlog (p2/p3) β20 β21*X1 β22*X2 β2j*Xr,where(3)pi probability of event i for i 1,2,3,β1js and β2js are parameters with 0 j mXrs are independent variables with 1 r mAll the estimates and the standard errors were adjusted for the multistage sampling designand clustering at the primary sampling unit, and were weighted at the national level to give resultsthat are unbiased and representative of the population (White 1982). Stata version 8 was used forthe analysis (Stata 2002).III. RESULTSA. Bivariate AnalysisThe weighted sample distribution is given in Table 1. The mean household size is 4.74. Duringthe 1-month recall period, 1.4% of the households reported institutional health payments, while61.8% reported noninstitutional health payments.1.Monthly Household Health PaymentsThe monthly household health payments (including both institutional and noninstitutional)was Rs196, which translates into US 14.5 billion annual private health payments in 2005. Themonthly household health payments varied from a low of Rs78 in Bihar to as high as Rs432 in October 2007
Section IIIResultsKerala. A household from the richest decile spent close to 20 times more than the poorest decilehousehold on health payments. The scheduled tribes (STs) spent one-fourth of the amount thatother castes spent on health payments.2.Proportion of Household Expenditure on Health PaymentsHousehold health payments were 4.6% of the total (including food and nonfood expenditure)household expenditure. The proportion of health payments in total household expenditure variedfrom 2.5% in the poorest decile to 7.3% in the richest decile, and from 1.9% in the North-Eaststates to 7.5% in Kerala state. Households with institutional health payments spent 35.1% of theirhousehold expenditures on health payments, while households with noninstitutional health paymentsspent 7.3%. The household health payments were 9.7% of the household nonfood expenditure.The proportion of health payment of total and nonfood household expenditure was higher in ruralareas, and varied widely at the state level. The health payments were two to three times higher inthe richest decile compared to the poorest decile. The proportion of health payments of householdexpenditure was lowest in households whose head had a diploma or graduate education comparedto households whose head was illiterate or had primary education. The health payment proportionwas higher in households with older heads of household.3.Incidence of Catastrophe-1 and Catastrophe-2The incidence of Catastrophe-1 (proportion of households with health payments morethan 10% of the household overall consumption expenditure) was 13.1%, and the incidence ofCatastrophe-2 (proportion of households with health payments more than 40% of the householdnonfood consumption expenditure) was 5.1%. Both Catastrophe-1 and Catastrophe-2 were higherin rural areas compared to urban areas, in households with less than five household memberscompared to households with five or more, in richer deciles of households compared to the poorerdecile households, and in households with older head of household compared to households withyounger head of household. Incidence of Catastrophe-1 and Catastrophe-2 was 79% and 60% inhouseholds with institutional health payments, respectively; and 21% and 8% in households withnoninstitutional health payments. Catastrophe-1 and Catastrophe-2 were lower in households whoseheads had higher education compared to households whose heads had lower education. Bothcatastrophes were higher in other backward caste groups, and lowest in the scheduled tribes.Catastrophe-1 and Catastrophe-2 also varied by household employment type and religion(Table 1). Around 8.5% of the people (nearly 26 million) below the poverty line had householdmonthly health payments more than 10% of the household monthly expenditure, which was higherthan 13% in Chattigarh, Kerala, and Uttar Pradesh. Around 14.5% above poverty line had healthpayments more than 10% of their household expenditure (total 117.5 million).4.Poverty Headcount, Poverty Gap, and Falling below the Poverty LineThe prepayment poverty headcount was 27.5%, which increased by 3.5 percentage pointspostpayment to 31%. The postpayment and prepayment headcount difference varied from 0.4percentage points in Delhi to 5.6 percentage points in Uttar Pradesh (Table 2). The prepaymentpoverty gap was Rs23.6, which increased to Rs27.5 post-payment. The postpayment and prepaymentpoverty gap was highest in Uttar Pradesh Uttar Pradesh(Rs6.6). The amount of money inducing Rs4ERD Working Paper Series No. 102
Incidence, Intensity, and Correlates of Catastrophic Out-of-Pocket Health Payments in IndiaSekhar Bonu, Indu Bhushan, and David H. Petersof additional poverty gap due to health payments is Rs53 billion ( 1.3 billion). Figure 1 providesa prepayment and post payment snapshot in rural and urban areas of India with the poverty cutoff line, depicting people above the poverty line regressing below the poverty line due to healthpayments. Around 39.5 million people above the poverty line regressed below the poverty line dueto health payments, which accounted for 4.9% of people above the poverty line (25% came froma single state, Uttar Pradesh) (Table 2).Table 2Change in Poverty Head Count Due to Health Payments, Poverty Gap Induced from HealthPayments, and Incidence of Catastrophe-1 Below and Above Poverty Line for Different Statesin IndiaPoverty HeadcountIndiaAndhra chalPradeshJammu a PradeshNorth EastOrissaPunjabRajasthanTamil NaduUnion TerritoriesUttar PradeshUttarakhandWest BengalPopulation(2006estimated;in 46.216.112.119.716.5Poverty Gap Induced by Health PaymentsPreDifference(% points)3.51.72.65.20.41.22.72.9paymentPoverty Poverty Gap(Rs)27.51432.14217.714.414.313.8Poverty GapInduced byMedicalPaymentsDifferenceRs.(in million 70continued. October 2007
Section IIIResultsTable 2. continued.Regressing below Poverty LineIndiaAndhra chalPradeshJammu a PradeshNorth EastOrissaPunjabRajasthanTamil NaduPeople abovePeople abovePoverty Line Who Poverty Line WhoFell below Poverty Fell below PovertyLine due to Health Line due to 2,3988.71,1720.5721.3183.31,5083.3675Catastrophe-1 below Poverty LineCatastrophe-1 above Poverty LinePercentNumber of Peopleage AffectedAffected bybelow PovertyCatastrophe-1Linebelow Poverty 69.1155.85468.2260Percentage Affectedabove PovertyLine(%)14.516.36.118.42.13.411.612.6Number of PeopleAffected byCatastrophe-1above 1,0543,0473,0586,3206,298Union Territories1.9493.8268.8223Uttar Pradesh8.310,30213.17,90420.825,872UttarakhandWest D Working Paper Series No. 102
Incidence, Intensity, and Correlates of Catastrophic Out-of-Pocket Health Payments in IndiaSekhar Bonu, Indu Bhushan, and David H. PetersFigure 1Rural and Urban Poverty Line, Pre-payment (Medical), and Postpayment Monthlyper Capita Consumption Expenditure, India5,000Per capita monthly consumption (Rs)Per capita monthly consumption (Rs)3,5003,0002,5002,0001,500Poverty line rural: 04,0003,5003,0002,5002,000Poverty line urban: Rs538.601,5001,0005000Individuals ranked in ascending order ofper capita consumption (Rural)050,000100,000150,000200,000Individuals ranked in ascending order ofper capita consumption (Urban)5. State-level Scatter PlotsThere was weak association between OOP health payments as a proportion of total householdconsumption expenditure and state per capita gross domestic product (GDP) (R-square 0.0314).Figure 2 shows that there was a high inverse correlation between state per capita GDP and percentof people above the poverty line who regressed below the poverty line due to health payments (Rsquare 0.46). Figure 3 is a scatter plot depicting the incidence of Catastrophe-1 and Catastrophe-2vis-a-vis people falling below the poverty line as a result of health payments. Figure 3 shows thatthe correlation of people falling below the poverty line with Catastrophe-2 is relatively strongerthan with Catastrophe-1.Figure 2Per Capita State GDP vis-à-vis Percent of People Regressing Below Poverty Line Post-payment, andOOP Health Payments as a Proportion of Total Consumption Expenditure70,00070,000GoaGoa60,000y -12428Ln(x) - 16678R 2 0.4591Delhi50,00040,000PunjabHimachal Pradesh30,00020,000HaryanaGujaratMaharashtraTamil NaduKeralaWest BengalUttaranchalJammu & KashmirRajasthanMadhya PradeshOrissaJharkhandAndhra Pradesh10,000Karnataka IndiaC hhatisgarhUttar Pradesh100123456789Percent of people regressing below poverty line post-payment (%)October 200750,000Delhiy -7071.6Ln(x) 2240.82R ashtraHimachalKeralaPradeshAndhra PradeshIndiaWest BengalJammu & UttaranchalRajasthan C hhatisgarhKashmirMadhya PradeshOrissaUttar PradeshJharkhandGujaratTamil NaduKarnatakaBiharBihar0Per capita state annual gross GDP (Rs)Per capita state annual gross GDP (Rs)60,0001002.03.04.05.06.07.08.0Out-of-pocket payments as a proportion of total consumption expenditure (%)
Section IIIResultsFigure 3State-wise Incidence of Health Expenditure-InducedCatastrophe-1 and Catastrophe-2 vis-à-vis Increase inPeople Living below Poverty Line after Adjusting for Health ExpenditureCatastrophe-1 Catastrophe-26UPPercentage points of increase in poverty due to healthexpenditurePercentage points of increase in poverty due to sthanMaharashtraJharkhand IndiaUttaranchalKarnataka HaryanaGujaratBiharHPPunjaby 0.1773x 0.9416TNR 2 aGujaratBiharHPPunjabTN432Goa1APUTNEJ&Ky 0.4338x 1.0742R 2 0.6869Delhi0005101520Incidence of Catastrophe-1 (%)B.UPChattisgarhMP525300246810Incidence of Catastrophe-2 (%)Multivariate AnalysisThe Wald’s test of independence (rho 0) confirms that estimation of outcome equation withouttaking selection into account would yield inconsistent results (except for Catastrophe-2). HenceHeckman sample selection model is consistent with more reliable estimates.1.Linear RegressionFor health payments and health payments as a proportion of total and nonfood expenditure(Table 3), rural households have less health payments compared to urban households but were morelikely to have a higher proportion of their total and nonfood expenditures dedicated to healthpayments, after controlling for all other factors in the model. Compared to the control state, UttarPradesh, most of the states had lower health payments and lower proportion of their householdincome dedicated to health payments, with few exceptions. Rural agricultural households had higherhealth payments and higher proportion of nonfood expenditure dedicated to health payments.Compared to upper castes, scheduled castes and other backward castes (OBCs) had higher healthpayments. Scheduled castes had lower health payments, and OBCs had a higher proportion of totalexpenditures dedicated to health payments. Health payments and proportion of total expenditureand nonfood expenditure increased with i
a number of studies have explored the incidence and intensity of catastrophic out-of-pocket (OOP) health payments in Asia (Peters et al. 2002, Xu et al. 2003, Wagstaff and van Doorslaer 2003, O' . (out-patient) health payments include expenditures on medicine, diagnostics, doctor's fees, family planning, and other medical expenses. The .