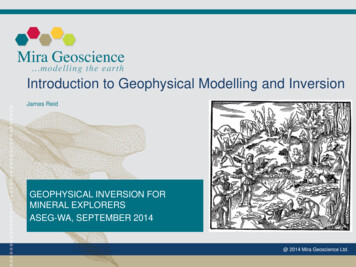
Transcription
Introduction to Geophysical Modelling and InversionJames ReidGEOPHYSICAL INVERSION FORMINERAL EXPLORERSASEG-WA, SEPTEMBER 2014@ 2014 Mira Geoscience Ltd.
Forward modelling vs. inversionForward Modelling: Given a model m and predicting data dd F(m)F is an operator representing the governing equations relating the model and dataFDataModel
InversionGeophysical inversion refers to the mathematical and statisticaltechniques for recovering information on subsurface physical properties(magnetic susceptibility, density, electrical conductivity etc) fromobserved geophysical data.
What is inversion?Inversion: Recording data d and predicting model m-1m F (d)DataModelF-1
What is inversion?Forward Modelling: Given a model and predicting datad F(m)Inversion: Recording data and predicting model-1m F (d)FNot Possible - Ill ConditionedDataModelF-1
Iterative inversionStarting modeland acquisitionparametersEach cyclethrough theinversion processis called aniterationCalculate modelresponse usingforward modellingalgorithmObjective functionlargeAlter modelparameters soas to reduce objective functionCompare observedand model responses,and calculateObjective functionObjective functionsmall, or maximumno. of iterationsexceededInversion processis complete:Output final model
How do inversions work?Given:- Field observations- Error estimates- Ability to forward model- Prior knowledgeThis chart summarizes therequirements for proceeding withinversion of geophysical data.Each box has important implicationsfor successful inversion.Ability to do forward modellingcalculations is assumed.Discretize the EarthChoose a suitabledata misfitDesign modelnormPerform inversionEvaluate resultsInterpret preferred model(s)Iterate
ModelsModel TypesSingle PhysicalProperty ValueParameterized object(susceptibility, length,depth, orientation)Physical propertyvaries as a functionof depthPlate in a free-space(vacuum)Plate in a half spacePlate in a layeredmodel(after: Inversion for Applied Geophysics)
ModelsModel Types2D modelsPhysical propertieschange in all 3directions.Generalized structureModel is unchangingperpendicular toprofile sectionModel objects havelimited strike length2.5D modelsConcatenated 1DmodelsGeometry ModelGeologic unitboundaries adjustlocation to create 3Dshapes and bodies.(after: Inversion for Applied Geophysics)
Why invert data?Helps explain complex data setse.g. DCIP, Gravity Gradiometry, AEM, ZTEM, DHEMRemoves topography effectsExplains the data with a model(s) of the earth:Provides a quantitative model that can be analysed What is the depth, geometry, volume, physical property of the model features?More easily relates to geology - easier for interpretation What geologic features can be determined in the model?Can QC the data, identify problematic dataacquisition problemsHelps separate the noise from the signal in the dataestimates the noise levelsestimates depth of penetration
Example: Target in presence of geological noiseData are sometimes difficult to interpretIP dataShallow anomalies representchargeable boulders in tillSubtle responses are importantInversion result is more easilyinterpretable in terms of geologyRecovered chargeability
Know The DataIn order for modelling to occur, all instrument system and surveyacquisition parameters have to be known.In general, try to do as little as possible to the data to preserve theinformationObviously erroneous data should be removed prior to inversion.This includes features/anomalies in the data which are not modelled by theforward modelling algorithm e.g., IP or SPM effects in EM data etcRUBBISH IN RUBBISH OUT
Inverse ModellingModelling Styles Parametric – few unknownse.g. TEM decayt1dB/dts115 Datat2t3time1D Conductivity models27 unknownmodel parameterss3(conductivity of each layer;thickness of upper three layers)s4
Inverse ModellingModelling Styles Parametric – few unknowns Generalized – many unknownse.g. TEM decaydB/dt1D Conductivity model15 Data40 unknownmodel parameters1D Mesh structure predefinedbut smaller than expectedstructure of geology.time structure inferred from theresulting model
Inverse ModellingModelling Styles Lithology based– VP suite (Fullagar Geophysics)– Geomodeller (Intrepid) Physical Property based– UBC-GIF codes– Geosoft Voxi– VP suite
Inverse ModellingPhysical Property Based Modelling Physical property values of many individual cells are adjusted.General structure is recoverede.g. Magnetic Data3D susceptibility model(low value cells removed)
Inverse ModellingPhysical Property Based Modelling Physical property values of many individual cells are adjusted.General structure is recovered.10,000 unknownmodel parameterse.g. Magnetic Data3D susceptibility model(low value cells removed)3D Mesh structure predefinedbut smaller than expectedstructure of geology. structure inferred from theresulting modelRESULT IS A PHYSICAL PROPERTY MODELCONTAINING STRUCTURE
Inverse ModellingLithology Based Modelling Provide physical properties (single value or distribution) for eachlithology and adjust the geometry to fit the data.RESULT IS AGEOLOGICALMODELSelected Spectrem EM Channels (Obs - blue, Calc - red)10 610 610 510 510 410 410001000100100Starting Model600550600550500450500450(courtesy Anglo American)Inverted Model600550600550500500450450
Inverse ModellingWhich Modelling Style to choose? Depends on the geophysical method, the survey design, and theexploration goal. Some examples might be: Is the goal to define the geometry/volume?Measure the physical properties well and choose a lithologic basedinversion (e.g. VPmg) Is the goal to define a thickness of cover from a few TEM soundings?Use a parametric inversion Is the goal to define both physical properties and geometry?Use a generalized inversion (e.g. UBC) What geologic information is available that can be integrated into themodelling?
Acceptable models and non-uniquenessThere are infinitely many models that can explain the observed dataWhy is this so? Because there are usually moreunknowns (model parameters)than observed data points(underdetermined problem) Some physically-based nonuniqueness Real data contain noise
Acceptable models and non-uniquenessThere are infinitely many models that can explain the observed data
How to chose one of infinitely many solutions?Narrow down the range of options using prior knowledgeGeophysical prior knowledge:Values are positive, and/or within boundsPhysical Properties: Estimates for host rock propertiesPoint-location values from drill hole informationLogical prior knowledge:Find a “simple” result - as featureless as possible.This sacrifices resolution but prevents over-interpreting the data.Geological prior knowledge:Character of the model (smooth, discontinuous)Some idea of scale length (or size) of the bodiesStructural Constraints
Model normThe model norm is a measure of the (mathematical) “size” of a modelThe inversion process is an automated decision making schemeThe model norm is a way of encoding prior information in a form suitablefor mathematical optimisation – we seek the “smallest” modelThe model norm is part of the solution to nonuniqueness Nonuniqueness is addressed by choosing the one model (frominfinitely many) that minimizes the defined model norm
Model normsSmoothMinimum horizontal structureMinimum vertical structureMinimise difference between the model and some “reference model”
Data misfitMeasure ofDIFFERENCE
What is a good measure of misfit?If we assume errors follow a particular distributionthen a measure of total misfit between predictionsand field data can be defined d(m): Predictions can be considered OK when d(m) tolerance We don’t want to fit the data too closely or we are fitting noise Not all measures of Data Misfit are equal
What contributes to data noise?Natural and cultural noise sourcesAccuracy and precision in data measurementsData positioning errorsApproximations made in forward modelling1D2D3DPlatesAnisotropyDiscretization of topography
Measures of misfitConsider the problem of fitting a straight line to the data shown below:2.5% Copper21.510.5001020304050Depth in drillhole (m)2.5e282% CuThe residuals are the differencesbetween the data points and thebest-fit line at each depth1.51y 0.0285x 0.33330.5They may be positive or negativee1100102030Depth in drillhole (m)4050
Measures of misfit – L1 and L2 norms0.650.60.550.50.450.40.350.30246810
Measures of misfit – L1 and L2 norms0.65y 0.0285x 0.3330.60.550.50.450.40.350.30246810Original dataMisfit sum of squares of residuals (L2 norm least-squares)
Measures of misfit – L1 and L2 norms0.650.60.550.50.450.40.350.302468Original dataMisfit sum of absolute values of residuals (L1 norm)10
Measures of misfit – L1 and L2 norms0.65y 0.0285x 0.3330.60.550.50.450.40.350.3024Add an outlying data point6810
Measures of misfit – L1 and L2 norms0.65y 0.0298x 0.3420.60.550.50.450.40.350.30246810One outlying data pointMisfit sum of squares of residuals (L2 norm least-squares)
Measures of misfit – L1 and L2 norms0.650.60.550.50.450.4L1 Less affected by outliers (noise)0.350.302468One outlying data pointMisfit sum of absolute values of residuals (L1 norm)10
Combining model norms and misfitA statement of the inverse problem is:Find the model whichMinimises the model norm ( M), andProduces an acceptably small data misfit ( D)Mathematically, this becomes a single optimisation“Minimise D b M ”(subject to d tolerance) is the combined objective functionb is the trade off parameter (regularisation parameter)
b is the regularization parameter d Solve: (m) d (m) b m(m) b too large underfitting the data.Structural information lost. b too small overfitting the data.Noise becomes imaged as structure. b just right ( d N ) optimal fit.Best estimate of a model whichadequately re-creates the observations.b *db 0 mTikhonov curve
Inverse ModellingNon-Uniqueness: Solution (partial ) Provide explicit geological information Constraints Combine information from independent geophysical methods Joint or Cooperative Inversionse.g. Gravity with Magnetics, Airborne EM with CSAMT, etc.
Integrated Modelling: ConstraintsSources of Data Geologic Mapping DH geological logs Interpreted cross-sections 3D geological models Physical property data per lithology Located physical property data measurementsSome information is subjective and some information is objective.As with the geophysical data we would desire to quantify the uncertaintyassociated with this data as an input to the inversion.
Rock properties are the link between geology and geophysics
Shameless plug – Mira Geoscience RockProperty Database Systemhttp://rpds.mirageoscience.com/Free!6 million measurements, including GSC database and published data
Rock Property Database SystemOrganise, understand, preserve and provide access to physicalproperty data
Common Earth ModellingExtending the model to include multiple properties,that honour multiple data sets, on a single modelobject.Goal:Obtain the most complete representation of the earth.Benefits:Improved resolution away from constraintsAllows more precise exploration using quantitative 3DGIS analysis.
Common Earth Modelling: Constrained Inversion Modelling2D Gravity Synthetic(Nick Williams)
Common Earth Modelling: Constrained Inversion Modelling2D Gravity Synthetic (Nick Williams)
Common Earth Modelling: Constrained Inversion Modelling2D Gravity Synthetic (Nick Williams)
Common Earth Modelling: Constrained Inversion Modelling2D Gravity Synthetic Surface constraintscan result in dramaticimprovements(Nick Williams)
Joint and cooperative inversionInversion using more than one geophysicalmethodMethods sensitive to same physicalproperty (e.g., TEM and CSAMT)Methods sensitive to related properties(e.g., seismic and gravity)Joint inversion – single objective functionCooperative inversion – iterative/sequentialapproachThese approaches require that we establishrelationships between the physical propertieseach method is sensitive to
Appraisal – How good is our model?Over-fitting vs under-fitting dataLimits to the dataLimits to the physicsDepth of investigationSuite of modelsPoint-spread functionsModel resolution analysisSensitivity analysisExtremal modelsModel Covariance MatrixCo-Kriging error
Summary and conclusionInversion has the potential to greatly improve the geologicalinterpretation of geophysical data High quality data is essential for the success of geophysical modelling More appropriate/efficient surveys can be designed Complex data sets can be understood (DH IP, 3D EM)Understanding physical property data is the key to successfulinversion interpretation. Rock type Alteration Mineralization
Summary and conclusionNon uniqueness in inversion is dealt with by imposing constraints Provide the constraints or they will be provided for you Minimum structure or geologicalInterpretation of inversion requires understanding of which partsof the model are driven by constraints and which parts are drivenby data. Requires inspection of multiple modelsInspect observed and predicted data before accepting a model. Did the inversion fit the data anomalies you are interested in? Beware of over-fitting and under-fitting your data
Summary and conclusionGeologically constrained inversion will greatly improve your results Constraints can be factual or conceptual (hypothesis testing) Sparse or detailed From different sourcesGeological mapsOutcrop samplesEstimates of overburden depthDetailed drill data
AcknowledgementsNigel PhillipsDianne MitchinsonScott NapierShannon FreyThomas Campagne- Mira Geoscience, VancouverRoss Brodie- Geoscience AustraliaKen Witherly- Condor ConsultingRegis Neroni- FMGLDoug Oldenburg- UBC-GIF
ReferenceInversion for Applied g/iag-outline.htm
Inversion Geophysical inversion refers to the mathematical and statistical techniques for recovering information on subsurface physical properties