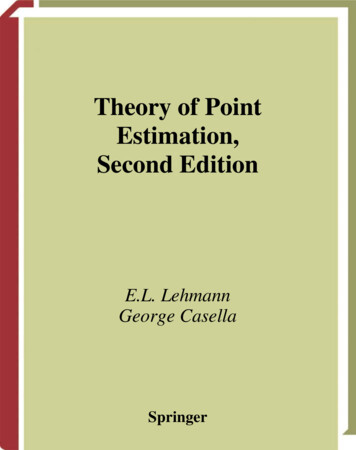
Transcription
Theory of PointEstimation,Second EditionE.L. LehmannGeorge CasellaSpringer
Springer Texts in StatisticsAdvisors:George Casella Stephen Fienberg Ingram Olkin
Springer Texts in StatisticsAlfred: Elements of Statistics for the Life and Social SciencesBerger: An Introduction to Probability and Stochastic ProcessesBilodeau and Brenner: Theory of Multivariate StatisticsBlom: Probability and Statistics: Theory and ApplicationsBrockwell and Davis: An Introduction to Times Series and ForecastingChow and Teicher: Probability Theory: Independence, Interchangeability,Martingales, Third EditionChristensen: Plane Answers to Complex Questions: The Theory of LinearModels, Second EditionChristensen: Linear Models for Multivariate, Time Series, and Spatial DataChristensen: Log-Linear Models and Logistic Regression, Second EditionCreighton: A First Course in Probability Models and Statistical InferenceDean and Voss: Design and Analysis of Experimentsdu Toit, Steyn, and Stumpf: Graphical Exploratory Data AnalysisDurrett: Essentials of Stochastic ProcessesEdwards: Introduction to Graphical Modelling, Second EditionFinkelstein and Levin: Statistics for LawyersFlury: A First Course in Multivariate StatisticsJobson: Applied Multivariate Data Analysis, Volume I: Regression andExperimental DesignJobson: Applied Multivariate Data Analysis, Volume II: Categorical andMultivariate MethodsKalbfleisch: Probability and Statistical Inference, Volume I: Probability,Second EditionKalbfleisch: Probability and Statistical Inference, Volume II: StatisticalInference, Second EditionKarr: ProbabilityKeyfitz: Applied Mathematical Demography, Second EditionKiefer: Introduction to Statistical InferenceKokoska and Nevison: Statistical Tables and FormulaeKulkarni: Modeling, Analysis, Design, and Control of Stochastic SystemsLehmann: Elements of Large-Sample TheoryLehmann: Testing Statistical Hypotheses, Second EditionLehmann and Casella: Theory of Point Estimation, Second EditionLindman: Analysis of Variance in Experimental DesignLindsey: Applying Generalized Linear ModelsMadansky: Prescriptions for Working StatisticiansMcPherson: Applying and Interpreting Statistics: A Comprehensive Guide,Second EditionMueller: Basic Principles of Structural Equation Modeling: An Introduction toLISREL and EQS(continued after index)
E.L. LehmannGeorge CasellaTheory of Point EstimationSecond Edition
E.L. LehmannDepartment of StatisticsUniversity of California, BerkeleyBerkeley, CA 94720USAGeorge CasellaDepartment of StatisticsUniversity of FloridaGainesville, FL 32611-8545USAEditorial BoardGeorge CasellaStephen FienbergIngram OlkinDepartment of StatisticsUniversity of FloridaGainesville, FL 32611-8545USADepartment of StatisticsCarnegie Mellon UniversityPittsburgh, PA 15213-3890USADepartment of StatisticsStanford UniversityStanford, CA 94305USALibrary of Congress Cataloging-in-Publication DataLehmann, E.L. (Erich Leo), 1917–Theory of point estimation. — 2nd ed. / E.L. Lehmann, GeorgeCasella.p.cm. — (Springer texts in statistics)Includes bibliographical references and index.ISBN 0-387-98502-6 (hardcover : alk. paper)1. Fix-point estimation. I. Casella, George. II. Title.III. Series.QA276.8.L43 1998519.5′44—dc2198-16687Printed on acid-free paper. 1998 Springer-Verlag New York, Inc.All rights reserved. This work may not be translated or copied in whole or in part without thewritten permission of the publisher (Springer-Verlag New York, Inc., 175 Fifth Avenue, New York,NY 10010, USA), except for brief excerpts in connection with reviews or scholarly analysis. Usein connection with any form of information storage and retrieval, electronic adaptation, computersoftware, or by similar or dissimilar methodology now known or hereafter developed is forbidden.The use of general descriptive names, trade names, trademarks, etc., in this publication, even if theformer are not especially identified, is not to be taken as a sign that such names, as understood bythe Trade Marks and Merchandise Marks Act, may accordingly be used freely by anyone.Production managed by Timothy Taylor; manufacturing supervised by Joe Quatela.Photocomposed copy prepared from the author’sfiles.Printed and bound by Maple-Vail Book Manufacturing Group, York, PA.Printed in the United States of America.9 8 7 6 5 4 3 2 1ISBN 0-387-98502-6SPIN 10660103Springer-Verlag New York Berlin HeidelbergA member of BertelsmannSpringer Science Business Media GmbH
To our childrenStephen, Barbara, and FiaELLBenjamin and SarahGC
This page intentionally left blank
Preface to the Second EditionSince the publication in 1983 of Theory of Point Estimation, much new workhas made it desirable to bring out a second edition. The inclusion of the newmaterial has increased the length of the book from 500 to 600 pages; of theapproximately 1000 references about 25% have appeared since 1983.The greatest change has been the addition to the sparse treatment of Bayesianinference in the first edition. This includes the addition of new sections onEquivariant, Hierarchical, and Empirical Bayes, and on their comparisons. Othermajor additions deal with new developments concerning the information inequality and simultaneous and shrinkage estimation. The Notes at the end ofeach chapter now provide not only bibliographic and historical material but alsointroductions to recent development in point estimation and other related topicswhich, for space reasons, it was not possible to include in the main text. Theproblem sections also have been greatly expanded. On the other hand, to savespace most of the discussion in the first edition on robust estimation (in particular L, M, and R estimators) has been deleted. This topic is the subject of twoexcellent books by Hampel et al (1986) and Staudte and Sheather (1990). Otherthan subject matter changes, there have been some minor modifications in thepresentation. For example, all of the references are now collected together atthe end of the text, examples are listed in a Table of Examples, and equationsare references by section and number within a chapter and by chapter, sectionand number between chapters.The level of presentation remains the same as that of TPE. Students with athorough course in theoretical statistics (from texts such as Bickel and Doksum1977 or Casella and Berger 1990) would be well prepared. The second edition ofTPE is a companion volume to “Testing Statistical Hypotheses, Second Edition(TSH2).” Between them, they provide an account of classical statistics from aunified point of view.Many people contributed to TPE2 with advice, suggestions, proofreading andproblem-solving. We are grateful to the efforts of John Kimmel for overseeingthis project; to Matt Briggs, Lynn Eberly, Rich Levine and Sam Wu for proofreading and problem solving, to Larry Brown, Anirban DasGupta, PersiDiaconis, Tom DiCiccio, Roger Farrell, Leslaw Gajek, Jim Hobert, Chuckvii
viiiPREFACE TO THE SECOND EDITIONMcCulloch, Elias Moreno, Christian Robert, Andrew Rukhin, Bill Strawdermanand Larry Wasserman for discussions and advice on countless topics, and toJune Meyermann for transcribing most of TEP to LaTeX. Lastly, we thank AndyScherrer for repairing the near-fatal hard disk crash and Marty Wells for thealmost infinite number of times he provided us with needed references.E. L. LehmannBerkeley, CaliforniaGeorge CasellaIthaca, New YorkMarch 1998
Preface to the First EditionThis book is concerned with point estimation in Euclidean sample spaces.The first four chapters deal with exact (small-sample) theory, and their approachand organization parallel those of the companion volume, Testing StatisticalHypotheses (TSH). Optimal estimators are derived according to criteria such asunbiasedness, equivariance, and minimaxity, and the material is organizedaround these criteria. The principal applications are to exponential and groupfamilies, and the systematic discussion of the rich body of (relatively simple)statistical problems that fall under these headings constitutes a second majortheme of the book.A theory of much wider applicability is obtained by adopting a large sampleapproach. The last two chapters are therefore devoted to large-sample theory,with Chapter 5 providing a fairly elementary introduction to asymptotic concepts and tools. Chapter 6 establishes the asymptotic efficiency, in sufficientlyregular cases, of maximum likelihood and related estimators, and of Bayes estimators, and presents a brief introduction to the local asymptotic optimality theory of Hajek and LeCam. Even in these two chapters, however, attention isrestricted to Euclidean sample spaces, so that estimation in sequential analysis,stochastic processes, and function spaces, in particular, is not covered.The text is supplemented by numerous problems. These and references to theliterature are collected at the end of each chapter. The literature, particularlywhen applications are included, is so enormous and spread over the journals ofso many countries and so many specialties that complete coverage did not seemfeasible. The result is a somewhat inconsistent coverage which, in part, reflectsmy personal interests and experience.It is assumed throughout that the reader has a good knowledge of calculusand linear algebra. Most of the book can be read without more advanced mathematics (including the sketch of measure theory which is presented in Section1.2 for the sake of completeness) if the following conventions are accepted.1. A central concept is that of an integral such as f dP or f dµ. This coversboth the discrete and continuous case. In the discrete case f dP becomes Σf(xi)P(xi) where P(xi) P(X xi) and f dµ becomes Σf(xi). In the continuous case, f dP and f dµ become, respectively, f(x)p(x) dx and f(x) dx. Little is lostix
xPREFACE TO THE FIRST EDITION(except a unified notation and some generality) by always making these substitutions.2. When specifying a probability distribution, P, it is necessary to specify notonly the sample space X, but also the class of sets over which P is to bedefined. In nearly all examples X will be a Euclidean space and a large classof sets, the so-called Borel sets, which in particular includes all open and closedsets. The references to can be ignored with practically no loss in the understanding of the statistical aspects.A forerunner of this book appeared in 1950 in the form of mimeographedlecture notes taken by Colin Blyth during a course I taught at Berkeley; theysubsequently provided a text for the course until the stencils gave out. Somesections were later updated by Michael Stuart and Fritz Scholz. Throughout theprocess of converting this material into a book, I greatly benefited from thesupport and advice of my wife, Juliet Shaffer. Parts of the manuscript were readby Rudy Beran, Peter Bickel, Colin Blyth, Larry Brown, Fritz Scholz, and GeoffWatson, all of whom suggested many improvements. Sections 6.7 and 6.8 arebased on material provided by Peter Bickel and Chuck Stone, respectively. Veryspecial thanks are due to Wei-Yin Loh, who carefully read the complete manuscript at its various stages and checked all the problems. His work led to thecorrections of innumerable errors and to many other improvements. Finally, Ishould like to thank Ruth Suzuki for her typing, which by now is legendary,and Sheila Gerber for her expert typing of many last-minute additions and corrections.E.L. LehmannBerkeley, California,March 1983
ContentsPreface to the Second EditionviiPreface to the First EditionixList of TablesxivList of FiguresxvList of ExamplesxviiTable of Notationxxv1Preparations1The Problem2Measure Theory and Integration3Probability Theory4Group Families5Exponential Families6Sufficient Statistics7Convex Loss Functions8Convergence in Probability and in Law9Problems10 Notes2Unbiasedness1UMVU Estimators2Continuous One- and Two-Sample Problems3Discrete Distributions4Nonparametric Families5The Information Inequality6The Multiparameter Case and Other 100109113124129143
xiiCONTENTS[ 0.03Equivariance1First Examples2The Principle of Equivariance3Location-Scale Families4Normal Linear Models5Random and Mixed Effects Models6Exponential Linear Models7Finite Population 34Average Risk Optimality1Introduction2First Examples3Single-Prior Bayes4Equivariant Bayes5Hierarchical Bayes6Empirical Bayes7Risk 2823055Minimaxity and Admissibility1Minimax Estimation2Admissibility and Minimaxity in Exponential Families3Admissibility and Minimaxity in Group Families4Simultaneous Estimation5Shrinkage Estimators in the Normal Case6Extensions7Admissibility and Complete 206Asymptotic Optimality1Performance Evaluations in Large Samples2Asymptotic Efficiency3Efficient Likelihood Estimation4Likelihood Estimation: Multiple Roots5The Multiparameter Case6Applications7Extensions8Asymptotic Efficiency of Bayes Estimators9Problems10 1
0.0 ]CONTENTSxiiiAuthor Index565Subject Index574
List of Tables1.4.1 Location-Scale Families1.5.1 Some One- and Two-Parameter Exponential Families1.7.1 Convex Functions1825452.3.1 I J Contingency Table2.5.1 I [τ (θ )] for Some Exponential Families2.5.2 If for Some Standard Distributions2.6.1 Three Information Matrices1071181191274.6.1 Bayes and Empirical Bayes Risks4.6.2 Hierarchical and Empirical Bayes Estimates4.7.1 Poisson Bayes and Empirical Bayes Risks2652672795.5.1 Maximum Component Risk5.5.2 Expected Value of the Shrinkage Factor5.6.1 Minimax Robust Bayes Estimator364365372
List of Figures1.10.1A Curved Exponential Family802.8.1 Illustration of the information inequality1445.2.1 Risks of Bounded Mean Estimators5.5.1 Risks of James-Stein Estimators5.5.2 Maximum Component Risk of the James-Stein Estimator3283573646.2.1 Risks of a Superefficient Estimator443
This page intentionally left blank
List of ExamplesChapter 1: .26.36.46.66.76.8The measurement problemCounting measureLebesgue measureContinuation of Example 2.1Continuation of Example 2.2Borel setsSupportLocation-scale familiesContinuation of Example 4.1Multivariate normal distributionThe linear modelA nonparametric iid familySymmetric distributionsContinuation of Example 4.7Sampling from a finite populationNormal familyMultinomialCurved normal familyLogit ModelNormal sampleBivariate normalContinuation of Example 5.3Binomial momentsPoisson momentsNormal momentsGamma momentsStein’s identity for the normalPoisson sufficient statisticSufficient statistic for a uniform distributionSufficient statistic for a symmetric distributionContinuation of Example 6.2Normal sufficient statisticContinuation of Example 3031333434353636
138.158.1710.1List of ExamplesContinuation of Example 6.4Sufficiency of order statisticsDifferent sufficient statisticsLocation familiesMinimal sufficiency in curved exponential familiesLocation/curved exponential familyLocation ancillarityCompleteness in some one-parameter familiesCompleteness in some two-parameter familiesMinimal sufficient but not completeCompleteness in the logit modelDose-response modelConvex functionsEntropy distanceConvex combinationQuadratic lossSquared error lossAbsolute error lossNonconvex lossSubharmonic functionsSubharmonic lossConsistency of the meanConsistency of S 2Markov chainsDegenerate limit distributionLimit of binomialContinuation of Example 8.13Asymptotic distribution of S 2Curvature[ 55859596080Chapter 2: 2.63.1Nonexistence of unbiased estimatorThe jackknifeLocally best unbiased estimationContinuation of Example 1.5Nonexistence of UMVU estimatorBinomial UMVU estimatorUMVU estimator for a uniform distributionEstimating polynomials of a normal varianceEstimating a probability or a critical valueThe normal two-sample problemThe multivariate normal one-sample problemThe exponential one-sample problemComparing UMVU and ML estimatorsBinomial UMVU estmators83838486878889919395969898100
0.0 85.25.55.65.75.115.135.166.36.5List of ExamplesInverse binomial samplingSequential estimation of binomial pTwo sampling plansPoisson UMVU estimationMultinomial UMVU estimationTwo-way contingency tablesConditional independence in a three-way tableMisbehaved UMVU estimatorEstimating the distribution functionNonparametric UMVU estimation of a meanNonparametric UMVU estimation of a varianceNonparametric UMVU estimation of a second momentDegree of the varianceNonexistence of unbiased estimatorTwo-sample UMVU estimatorU-estimation of covarianceHammersley-Chapman-Robbins inequalityInformation in a gamma variableInformation in a normal variableInformation about a function of a Poisson parameterBinomial attainment of information boundPoisson attainment of information boundIntegrabilityMultivariate normal information matrixInformation in location-scale 112112113114117117118121121123126126Chapter 3: 1.262.22.32.62.92.112.122.14Estimating binomial pLocation equivariant estimators based on one observationContinuation of Example 1.9MRE under 0 1 lossNormalExponentialUniformContinuation of Example 1.19Double ation familyTwo-sample location familyContinuation of Example 2.3Conclusion of Example 2.3Binomial transformation groupOrbits of a scale 57158159162162163163164
.67.8List of ExamplesCounterexampleScale equivariant estimator based on one observationStandardized power lossContinuation of Example 3.2MRE for normal variance, known meanNormal scale estimation under Stein’s lossRisk-unbiasednessMRE for normal variance, unknown meanUniformMore normal variance estimationMRE for normal meanUniform location parameterExponentialOne-way layoutA simple regression modelContinuation of Example 4.1Simple linear regressionUnbalanced one-way layoutTwo-way layoutQuadratic unbiased estimatorsOne-way random effects modelRandom effects two-way layoutTwo nested random factorsMixed effects modelBest prediction of random effectsTwo-way contingency tableConditional independence in a three-way tableUMVU estimation in simple random samplingSum-quota samplingInformative labelsUMVU estimation in stratified random sampling[ ter 4: Average Risk ssonBinomialSequential binomial samplingNormal meanSample meansNormal Variance, known meanNormal Variance, unknown meanRandom effects one-way layoutImproper prior BayesLimit of Bayes estimatorsScale UniformMultiple normal model229230233233235236237237238239240242
0.0 5.96.16.26.46.56.66.76.87.17.37.47.67.77.8List of ExamplesContinuation of Example 3.4Conjugate gammaEquivariant binomialLocation groupScale groupLocation-scale groupContinuation of Example 4.3Continuation of Example 4.4Continuation of Example 4.5Invariance of induced measuresConjugate normal hierarchyConjugate normal hierarchy, continuedBeta-binomial hierarchyPoisson hierarchy with Gibbs samplingGibbs point estimationNormal hierarchyBinomial reference priorNormal empirical BayesEmpirical Bayes binomialNormal empirical Bayes, µ unknownHierarchical Bayes approximationPoisson hierarchyContinuation of Example 5.2Continuation of Example 3.1The James-Stein estimatorBayes risk of the James-Stein estimatorBayesian robustness of the James-Stein estimatorPoisson Bayes and empirical Bayes estimationEmpirical Bayes analysis of varianceAnalysis of variance with regression 0Chapter 5: Minimaxity and 32.52.7A first exampleBinomialRandomized minimax estimatorDifference of two binomialsNormal meanNonparametric meanSimple random samplingBinomial restricted Bayes estimatorNormalRandomized responseVariance componentsAdmissibility of linear estimatorsContinuation of Example 2.5309311313313317318319321321322323323324
07.217.227.23List of ExamplesAdmissibility of X̄Truncated normal meanLinear minimax riskLinear modelNormal varianceContinuation of Example 2.13Normal variance, unknown meanBinomialBinomial admissible minimax estimatorTwo binomialsFinite groupCircular location familyLocation family on the lineMRE not minimaxA random walkDiscrete location familySeveral normal meansMultinomial BayesMultinomial minimaxIndependent experimentsA variety of loss functionsAdmissibility of XProper Bayes minimaxLoss functions in the one-way layoutSuperharmonicity of the marginalSuperharmonic priorShrinking toward a common meanShrinking toward a linear subspaceCombining biased and unbiased estimatorsUnknown varianceMixture of normalsBayesian robustnessImproved estimation for independent PoissonsImproved negative binomial estimationMultivariate binomialUnreasonable admissible estimatorThe positive-part Stein estimatorNonexistence of a minimal complete classExponential families have continuous risksSquared error lossAdmissible negative binomial MLEBrown’s identityMultivariate normal meanContinuation of Example 7.20Tail minimaxityBinomial estimation[ 75375376377377378379380381383385385386387
0.0 ]7.247.259.1List of ExamplesWhen there is no Stein effectAdmissible linear estimatorsConditional biasxxiii388389421Chapter 6: Asymptotic e variance of a binomial UMVU estimatorApproximate variance of a normal probability estimatorLimiting variance in the exponential distributionContinuation of Example 1.6Asymptotic distribution of squared mean estimatorsA family of estimatorsLarge-sample behavior of squared mean estimatorsAsymptotically biased estimatorSuperefficient estimatorContinuation of Example 2.5Binomial MLENormal MLEAn inconsistent MLEMinimum likelihoodOne-parameter exponential familyTruncated normalDouble exponentialContinuation of Example 3.6Location parameterGrouped or censored observationsMixturesCensored data likelihoodEM in a one-way layoutContinuation of Example 4.9Weibull distributionLocation-scale familiesMultiparameter exponential familiesMultivariate normal distributionBivariate normal distributionContinuation of Example 6.5Efficiency of nonparametric UMVU estimatorNormal mixturesMultinomial experimentsEstimation of a common meanBalanced one-way random effects modelBalanced two-way random effects modelIndependent binomial experimentsTotal informationNormal autoregressive Markov seriesEstimation of a common 3474475476477478479479481482
xxiv7.107.117.127.137.147.158.18.48.58.6List of ExamplesRegression with both variables subject to errorUniform MLEExponential MLEPareto MLELognormal MLESecond-order mean squared errorLimiting binomialExponential familiesLocation familiesBinomial[ 0.0482485485486486487487491492493
Table of NotationThe following notation will be used throughout the book.We present this list for easy reference.QuantityNotationCommentRandom variableX, Y,uppercaseSample spaceX,Yuppercase scriptRoman lettersParameterθ, λlowercase Greek lettersParameter space,uppercase scriptGreek lettersRealized values(data)x, ylowercaseDistribution function(cdf)F (x), F (x θ), P (x θ)Fθ (x), Pθ , (x)continuousor discreteDensity function (pdf)f (x), f (x θ), p(x θ )fθ (x), Pθ (x)notation is “generic”,i.e., don’t assumef (x y) f (x z)Prior distribution (γ ), (γ λ)Prior densityπ(γ ), π(γ λ)may be improper(X , P, B)sample space, probabilitydistribution, andsigma-algebra of setsProbability triple
xxviTable of NotationQuantity[ 0.0NotationCommentVectorh (h1 , . . . , hn ) {hi }boldface signifiesvectorsMatrixH {hij } hij uppercase signifiesmatricesIdentity matrixvector of onesmatrix of onesI1J 11 Special matricesand vectorsDot notationGradienthi· h(x) HessianLaplacianEuclidean normIndicator functionBig ”Oh,” little ”oh”j 1hijaverage across thedotted subscript vector of partialderivatives h(x) xi h(x) Jacobian J h(x), . . . , x n h(x) x1 1J xj 2 xi xj h(x)matrix of partialsecond derivatives hi (x)matrix ofderivatives 2i xi2 h(x)sum ofsecond derivatives x ( xi2 )1/2IA (x), I (x A)or I (x a)equals 1 ifx A,0 otherwiseO(n), o(n) or Op (n), op (n)As n constant, o(n) 0nsubscript p denotesin probabilityO(n)n
CHAPTER 1Preparations1 The ProblemStatistics is concerned with the collection of data and with their analysis andinterpretation. We shall not consider the problem of data collection in this bookbut shall take the data as given and ask what they have to tell us. The answerdepends not only on the data, on what is being observed, but also on backgroundknowledge of the situation; the latter is formalized in the assumptions with whichthe analysis is entered. There have, typically, been three principal lines of approach:Data analysis. Here, the data are analyzed on their own terms, essentially withoutextraneous assumptions. The principal aim is the organization and summarizationof the data in ways that bring out their main features and clarify their underlyingstructure.Classical inference and decision theory. The observations are now postulatedto be the values taken on by random variables which are assumed to follow ajoint probability distribution, P , belonging to some known class P. Frequently,the distributions are indexed by a parameter, say θ (not necessarily real-valued),taking values in a set, , so that(1.1)P {Pθ , θ }.The aim of the analysis is then to specify a plausible value for θ (this is theproblem of point estimation), or at least to determine a subset of of which wecan plausibly assert that it does, or does not, contain θ (estimation by confidencesets or hypothesis testing). Such a statement about θ can be viewed as a summaryof the information provided by the data and may be used as a guide to action.Bayesian analysis. In this approach, it is assumed in addition that θ is itselfa random variable (though unobservable) with a known distribution. This priordistribution (specified according to the problem) is modified in light of the data todetermine a posterior distribution (the conditional distribution of θ given the data),which summarizes what can be said about θ on the basis of the assumptions madeand the data.These three methods of approach permit increasingly strong conclusions, butthey do so at the price of assumptions which are correspondingly more detailedand possibly less reliable. It is often desirable to use different formulations inconjunction; for example, by planning a study (e.g., determining sample size)under rather detailed assumptions but performing the analysis under a weaker setwhich appears more trustworthy. In practice, it is often useful to model a problem
2PREPARATIONS[ 1.1in a number of different ways. One may then be satisfied if there is reasonableagreement among the conclusions; in the contrary case, a closer examination ofthe different sets of assumptions will be indicated.In this book, Chapters 2, 3, and 5 will be primarily concerned with the secondformulation, Chapter 4 with the third. Chapter 6 considers a large-sample treatment of both. (A book-length treatment of the first formulation is Tukey’s classicExploratory Data Analysis, or the more recent book by Hoaglin, Mosteller, andTukey 1985, which includes the interesting approach of Diaconis 1985.) Throughout the book we shall try to specify what is meant by a “best” statistical procedurefor a given problem and to develop methods for determining such procedures.Ideally, this would involve a formal decision-theoretic evaluation of the problemresulting in an optimal procedure.Unfortunately, there are difficulties with this approach, partially caused by thefact that there is no unique, convincing definition of optimality. Compounding thislack of consensus about optimality criteria is that there is also no consensus aboutthe evaluation of such criteria. For example, even if it is agreed that squared errorloss is a reasonable criterion, the method of evaluation, be it Bayesian, frequentist(the classical approach of averaging over repeated experiments), or conditional,must then be agreed upon.Perhaps even more serious is the fact that the optimal procedure and its properties may depend very heavily on the precise nature of the assumed probabilitymodel (1.1), which often rests on rather flimsy foundations. It therefore becomesimportant to consider the robustness of the proposed solution under deviationsfrom the model. Some aspects of robustness, from both Bayesian and frequentistperspectives, will be taken up in Chapters 4 and 5.The discussion so far has been quite general; let us now specialize to pointestimation. In terms of the model (1.1), suppose that g is a real-valued functiondefined over and that we wou
Blom: Probability and Statistics: Theory and Applications Brockwell and Davis: An Introduction to Times Series and Forecasting Chow and Teicher: Probability Theory: Independence, Interchangeability, Martingales, Third Edition Christensen: Plane Answers to Complex Questions: The