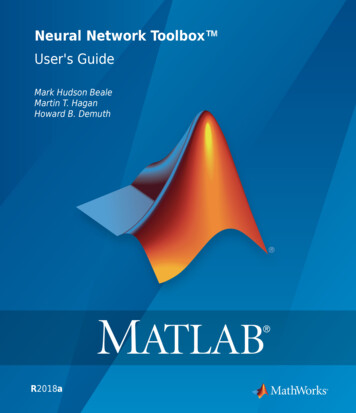
Transcription
Neural Network Toolbox User's GuideMark Hudson BealeMartin T. HaganHoward B. DemuthR2018a
How to Contact MathWorksLatest news:www.mathworks.comSales and services:www.mathworks.com/sales and servicesUser community:www.mathworks.com/matlabcentralTechnical support:www.mathworks.com/support/contact usPhone:508-647-7000The MathWorks, Inc.3 Apple Hill DriveNatick, MA 01760-2098Neural Network Toolbox User's Guide COPYRIGHT 1992–2018 by The MathWorks, Inc.The software described in this document is furnished under a license agreement. The software may be usedor copied only under the terms of the license agreement. No part of this manual may be photocopied orreproduced in any form without prior written consent from The MathWorks, Inc.FEDERAL ACQUISITION: This provision applies to all acquisitions of the Program and Documentation by,for, or through the federal government of the United States. By accepting delivery of the Program orDocumentation, the government hereby agrees that this software or documentation qualifies as commercialcomputer software or commercial computer software documentation as such terms are used or defined inFAR 12.212, DFARS Part 227.72, and DFARS 252.227-7014. Accordingly, the terms and conditions of thisAgreement and only those rights specified in this Agreement, shall pertain to and govern the use,modification, reproduction, release, performance, display, and disclosure of the Program andDocumentation by the federal government (or other entity acquiring for or through the federal government)and shall supersede any conflicting contractual terms or conditions. If this License fails to meet thegovernment's needs or is inconsistent in any respect with federal procurement law, the government agreesto return the Program and Documentation, unused, to The MathWorks, Inc.TrademarksMATLAB and Simulink are registered trademarks of The MathWorks, Inc. Seewww.mathworks.com/trademarks for a list of additional trademarks. Other product or brandnames may be trademarks or registered trademarks of their respective holders.PatentsMathWorks products are protected by one or more U.S. patents. Please seewww.mathworks.com/patents for more information.
Revision HistoryJune 1992April 1993January 1997July 1997January 1998September 2000June 2001July 2002January 2003June 2004October 2004October 2004March 2005March 2006September 2006March 2007September 2007March 2008October 2008March 2009September 2009March 2010September 2010April 2011September 2011March 2012September 2012March 2013September 2013March 2014October 2014March 2015September 2015March 2016September 2016March 2017September 2017March 2018First printingSecond printingThird printingFourth printingFifth printingSixth printingSeventh printingOnline onlyOnline onlyOnline onlyOnline onlyEighth printingOnline onlyOnline onlyNinth printingOnline onlyOnline onlyOnline onlyOnline onlyOnline onlyOnline onlyOnline onlyOnline onlyOnline onlyOnline onlyOnline onlyOnline onlyOnline onlyOnline onlyOnline onlyOnline onlyOnline onlyOnline onlyOnline onlyOnline onlyOnline onlyOnline onlyOnline onlyRevised for Version 3 (Release 11)Revised for Version 4 (Release 12)Minor revisions (Release 12.1)Minor revisions (Release 13)Minor revisions (Release 13SP1)Revised for Version 4.0.3 (Release 14)Revised for Version 4.0.4 (Release 14SP1)Revised for Version 4.0.4Revised for Version 4.0.5 (Release 14SP2)Revised for Version 5.0 (Release 2006a)Minor revisions (Release 2006b)Minor revisions (Release 2007a)Revised for Version 5.1 (Release 2007b)Revised for Version 6.0 (Release 2008a)Revised for Version 6.0.1 (Release 2008b)Revised for Version 6.0.2 (Release 2009a)Revised for Version 6.0.3 (Release 2009b)Revised for Version 6.0.4 (Release 2010a)Revised for Version 7.0 (Release 2010b)Revised for Version 7.0.1 (Release 2011a)Revised for Version 7.0.2 (Release 2011b)Revised for Version 7.0.3 (Release 2012a)Revised for Version 8.0 (Release 2012b)Revised for Version 8.0.1 (Release 2013a)Revised for Version 8.1 (Release 2013b)Revised for Version 8.2 (Release 2014a)Revised for Version 8.2.1 (Release 2014b)Revised for Version 8.3 (Release 2015a)Revised for Version 8.4 (Release 2015b)Revised for Version 9.0 (Release 2016a)Revised for Version 9.1 (Release 2016b)Revised for Version 10.0 (Release 2017a)Revised for Version 11.0 (Release 2017b)Revised for Version 11.1 (Release 2018a)
ContentsNeural Network Toolbox Design Book1Neural Network Objects, Data, and Training StylesWorkflow for Neural Network Design . . . . . . . . . . . . . . . . . . . .1-2Four Levels of Neural Network Design . . . . . . . . . . . . . . . . . . .1-4Neuron Model . . . . . . . . . . . . . . . . . . . . . . . . . . . . . . . . . . . . . . .Simple Neuron . . . . . . . . . . . . . . . . . . . . . . . . . . . . . . . . . . . .Transfer Functions . . . . . . . . . . . . . . . . . . . . . . . . . . . . . . . . .Neuron with Vector Input . . . . . . . . . . . . . . . . . . . . . . . . . . . .1-51-51-61-7Neural Network Architectures . . . . . . . . . . . . . . . . . . . . . . . . .One Layer of Neurons . . . . . . . . . . . . . . . . . . . . . . . . . . . . . .Multiple Layers of Neurons . . . . . . . . . . . . . . . . . . . . . . . . .Input and Output Processing Functions . . . . . . . . . . . . . . . .1-111-111-131-15Create Neural Network Object . . . . . . . . . . . . . . . . . . . . . . . . .1-17Configure Neural Network Inputs and Outputs . . . . . . . . . . .1-21Understanding Neural Network Toolbox Data Structures . . .Simulation with Concurrent Inputs in a Static Network . . . . .Simulation with Sequential Inputs in a Dynamic Network . . .Simulation with Concurrent Inputs in a Dynamic Network . .1-231-231-241-26Neural Network Training Concepts . . . . . . . . . . . . . . . . . . . . .Incremental Training with adapt . . . . . . . . . . . . . . . . . . . . . .Batch Training . . . . . . . . . . . . . . . . . . . . . . . . . . . . . . . . . . .1-281-281-31v
Training Feedback . . . . . . . . . . . . . . . . . . . . . . . . . . . . . . . .2Deep NetworksDeep Learning in MATLAB . . . . . . . . . . . . . . . . . . . . . . . . . . . . .What Is Deep Learning? . . . . . . . . . . . . . . . . . . . . . . . . . . . . .Try Deep Learning in 10 Lines of MATLAB Code . . . . . . . . . . .Start Deep Learning Faster Using Transfer Learning . . . . . . .Train Classifiers Using Features Extracted fromPretrained Networks . . . . . . . . . . . . . . . . . . . . . . . . . . . . .Deep Learning with Big Data on CPUs, GPUs, in Parallel, and onthe Cloud . . . . . . . . . . . . . . . . . . . . . . . . . . . . . . . . . . . . . .viContents1-342-22-22-52-72-82-8Try Deep Learning in 10 Lines of MATLAB Code . . . . . . . . . .2-10Deep Learning with Big Data on GPUs and in Parallel . . . . .Training with Multiple GPUs . . . . . . . . . . . . . . . . . . . . . . . . .Fetch and Preprocess Data in Background . . . . . . . . . . . . . .Deep Learning in the Cloud . . . . . . . . . . . . . . . . . . . . . . . . .2-132-152-162-17Construct Deep Network Using Autoencoders . . . . . . . . . . . .2-18Pretrained Convolutional Neural Networks . . . . . . . . . . . . . .Download Pretrained Networks . . . . . . . . . . . . . . . . . . . . . .Transfer Learning . . . . . . . . . . . . . . . . . . . . . . . . . . . . . . . . .Feature Extraction . . . . . . . . . . . . . . . . . . . . . . . . . . . . . . . .2-212-222-242-25Learn About Convolutional Neural Networks . . . . . . . . . . . . .2-27List of Deep Learning Layers . . . . . . . . . . . . . . . . . . . . . . . . . .2-31Specify Layers of Convolutional Neural Network . . . . . . . . . .Image Input Layer . . . . . . . . . . . . . . . . . . . . . . . . . . . . . . . .Convolutional Layer . . . . . . . . . . . . . . . . . . . . . . . . . . . . . . .Batch Normalization Layer . . . . . . . . . . . . . . . . . . . . . . . . . .ReLU Layer . . . . . . . . . . . . . . . . . . . . . . . . . . . . . . . . . . . . .Cross Channel Normalization (Local Response Normalization)Layer . . . . . . . . . . . . . . . . . . . . . . . . . . . . . . . . . . . . . . . .Max- and Average-Pooling Layers . . . . . . . . . . . . . . . . . . . . .2-362-372-372-392-392-402-40
Dropout Layer . . . . . . . . . . . . . . . . . . . . . . . . . . . . . . . . . . .Fully Connected Layer . . . . . . . . . . . . . . . . . . . . . . . . . . . . .Output Layers . . . . . . . . . . . . . . . . . . . . . . . . . . . . . . . . . . .Set Up Parameters and Train Convolutional NeuralNetwork . . . . . . . . . . . . . . . . . . . . . . . . . . . . . . . . . . . . . . . . .Specify Solver and Maximum Number of Epochs . . . . . . . . .Specify and Modify Learning Rate . . . . . . . . . . . . . . . . . . . .Specify Validation Data . . . . . . . . . . . . . . . . . . . . . . . . . . . . .Select Hardware Resource . . . . . . . . . . . . . . . . . . . . . . . . . .Save Checkpoint Networks and Resume Training . . . . . . . . .Set Up Parameters in Convolutional and Fully ConnectedLayers . . . . . . . . . . . . . . . . . . . . . . . . . . . . . . . . . . . . . . .Train Your Network . . . . . . . . . . . . . . . . . . . . . . . . . . . . . . e Training from a Checkpoint Network . . . . . . . . . . . .2-51Define Custom Deep Learning Layers . . . . . . . . . . . . . . . . . . .Layer Templates . . . . . . . . . . . . . . . . . . . . . . . . . . . . . . . . . .Layer Architecture . . . . . . . . . . . . . . . . . . . . . . . . . . . . . . . .Check Validity of Layer . . . . . . . . . . . . . . . . . . . . . . . . . . . . .Output Layer Architecture . . . . . . . . . . . . . . . . . . . . . . . . . .2-562-572-602-642-66Define a Custom Deep Learning Layer with LearnableParameters . . . . . . . . . . . . . . . . . . . . . . . . . . . . . . . . . . . . . . .Layer with Learnable Parameters Template . . . . . . . . . . . . .Name the Layer . . . . . . . . . . . . . . . . . . . . . . . . . . . . . . . . . .Declare Properties and Learnable Parameters . . . . . . . . . . .Create Constructor Function . . . . . . . . . . . . . . . . . . . . . . . .Create Forward Functions . . . . . . . . . . . . . . . . . . . . . . . . . .Create Backward Function . . . . . . . . . . . . . . . . . . . . . . . . . .Completed Layer . . . . . . . . . . . . . . . . . . . . . . . . . . . . . . . . .GPU Compatibility . . . . . . . . . . . . . . . . . . . . . . . . . . . . . . . .Check Validity of Layer Using checkLayer . . . . . . . . . . . . . . .Include User-Defined Layer in Network . . . . . . . . . . . . . . . e a Custom Regression Output Layer . . . . . . . . . . . . . . .Regression Output Layer Template . . . . . . . . . . . . . . . . . . . .Name the Layer . . . . . . . . . . . . . . . . . . . . . . . . . . . . . . . . . .Declare Layer Properties . . . . . . . . . . . . . . . . . . . . . . . . . . .Create Constructor Function . . . . . . . . . . . . . . . . . . . . . . . .Create Forward Loss Function . . . . . . . . . . . . . . . . . . . . . . .Create Backward Loss Function . . . . . . . . . . . . . . . . . . . . . .2-872-872-882-892-892-902-92vii
viiiContentsCompleted Layer . . . . . . . . . . . . . . . . . . . . . . . . . . . . . . . . .GPU Compatibility . . . . . . . . . . . . . . . . . . . . . . . . . . . . . . . .Include Custom Regression Output Layer in Network . . . . . .2-922-932-94Define a Custom Classification Output Layer . . . . . . . . . . . . .Classification Output Layer Template . . . . . . . . . . . . . . . . . .Name the Layer . . . . . . . . . . . . . . . . . . . . . . . . . . . . . . . . . .Declare Layer Properties . . . . . . . . . . . . . . . . . . . . . . . . . . .Create Constructor Function . . . . . . . . . . . . . . . . . . . . . . .Create Forward Loss Function . . . . . . . . . . . . . . . . . . . . . .Create Backward Loss Function . . . . . . . . . . . . . . . . . . . . .Completed Layer . . . . . . . . . . . . . . . . . . . . . . . . . . . . . . . .GPU Compatibility . . . . . . . . . . . . . . . . . . . . . . . . . . . . . . .Include Custom Classification Output Layer in Network . . ck Custom Layer Validity . . . . . . . . . . . . . . . . . . . . . . . . .List of Tests . . . . . . . . . . . . . . . . . . . . . . . . . . . . . . . . . . . .Diagnostics . . . . . . . . . . . . . . . . . . . . . . . . . . . . . . . . . . . .Check Validity of Layer Using checkLayer . . . . . . . . . . . . . .2-1072-1072-1092-114Long Short-Term Memory Networks . . . . . . . . . . . . . . . . . . .LSTM Network Architecture . . . . . . . . . . . . . . . . . . . . . . . .Layers . . . . . . . . . . . . . . . . . . . . . . . . . . . . . . . . . . . . . . . .Classification and Prediction . . . . . . . . . . . . . . . . . . . . . . . .Sequence Padding, Truncation, and Splitting . . . . . . . . . . .LSTM Layer Architecture . . . . . . . . . . . . . . . . . . . . . . . . . .2-1162-1162-1192-1202-1202-122Preprocess Images for Deep Learning . . . . . . . . . . . . . . . . . .Resize Images . . . . . . . . . . . . . . . . . . . . . . . . . . . . . . . . . .Augment Images for Training . . . . . . . . . . . . . . . . . . . . . . .Advanced Image Preprocessing . . . . . . . . . . . . . . . . . . . . .2-1272-1272-1282-129Develop Custom Mini-Batch Datastore . . . . . . . . . . . . . . . . .Overview . . . . . . . . . . . . . . . . . . . . . . . . . . . . . . . . . . . . . .Implement MiniBatchable Datastore . . . . . . . . . . . . . . . . . .Add Support for Shuffling . . . . . . . . . . . . . . . . . . . . . . . . . .Add Support for Parallel and Multi-GPU Training . . . . . . . .Add Support for Background Dispatch . . . . . . . . . . . . . . . .Validate Custom Mini-Batch Datastore . . . . . . . . . . . . . . . .2-1312-1312-1322-1352-1362-1372-138
34Deep Learning in the CloudScale Up Deep Learning in Parallel and in the Cloud . . . . . . . .Deep Learning on Multiple GPUs . . . . . . . . . . . . . . . . . . . . . .Deep Learning in the Cloud . . . . . . . . . . . . . . . . . . . . . . . . . .Advanced Support for Fast Multi-Node GPUCommunication . . . . . . . . . . . . . . . . . . . . . . . . . . . . . . . . .3-23-23-43-5Deep Learning with MATLAB on Multiple GPUs . . . . . . . . . . . .Select Particular GPUs to Use for Training . . . . . . . . . . . . . . .Train Network in the Cloud Using Built-in Parallel Support . . .3-73-73-8Multilayer Neural Networks and BackpropagationTrainingMultilayer Neural Networks and BackpropagationTraining . . . . . . . . . . . . . . . . . . . . . . . . . . . . . . . . . . . . . . . . . .4-2Multilayer Neural Network Architecture . . . . . . . . . . . . . . . . . .Neuron Model (logsig, tansig, purelin) . . . . . . . . . . . . . . . . . .Feedforward Neural Network . . . . . . . . . . . . . . . . . . . . . . . . .4-44-44-5Prepare Data for Multilayer Neural Networks . . . . . . . . . . . . .4-8Choose Neural Network Input-Output ProcessingFunctions . . . . . . . . . . . . . . . . . . . . . . . . . . . . . . . . . . . . . . . . .Representing Unknown or Don't-Care Targets . . . . . . . . . . .4-94-11Divide Data for Optimal Neural Network Training . . . . . . . . .4-12Create, Configure, and Initialize MultilayerNeural Networks . . . . . . . . . . . . . . . . . . . . . . . . . . . . . . . . . .Other Related Architectures . . . . . . . . . . . . . . . . . . . . . . . . .Initializing Weights (init) . . . . . . . . . . . . . . . . . . . . . . . . . . .4-144-154-15Train and Apply Multilayer Neural Networks . . . . . . . . . . . . .Training Algorithms . . . . . . . . . . . . . . . . . . . . . . . . . . . . . . .4-174-18ix
5Training Example . . . . . . . . . . . . . . . . . . . . . . . . . . . . . . . . .Use the Network . . . . . . . . . . . . . . . . . . . . . . . . . . . . . . . . .4-204-22Analyze Shallow Neural Network Performance AfterTraining . . . . . . . . . . . . . . . . . . . . . . . . . . . . . . . . . . . . . . . . .Improving Results . . . . . . . . . . . . . . . . . . . . . . . . . . . . . . . .4-244-29Limitations and Cautions . . . . . . . . . . . . . . . . . . . . . . . . . . . . .4-30Dynamic Neural NetworksIntroduction to Dynamic Neural Networks . . . . . . . . . . . . . . . .5-2How Dynamic Neural Networks Work . . . . . . . . . . . . . . . . . . . .5-3Feedforward and Recurrent Neural Networks . . . . . . . . . . . . .5-3Applications of Dynamic Networks . . . . . . . . . . . . . . . . . . . .5-10Dynamic Network Structures . . . . . . . . . . . . . . . . . . . . . . . .5-10Dynamic Network Training . . . . . . . . . . . . . . . . . . . . . . . . . .5-11xContentsDesign Time Series Time-Delay Neural Networks . . . . . . . . .Prepare Input and Layer Delay States . . . . . . . . . . . . . . . . . .5-135-17Design Time Series Distributed Delay Neural Networks . . . .5-19Design Time Series NARX Feedback Neural Networks . . . . .Multiple External Variables . . . . . . . . . . . . . . . . . . . . . . . . . .5-225-29Design Layer-Recurrent Neural Networks . . . . . . . . . . . . . . .5-30Create Reference Model Controller with MATLAB Script . . .5-33Multiple Sequences with Dynamic Neural Networks . . . . . . .5-40Neural Network Time-Series Utilities . . . . . . . . . . . . . . . . . . .5-41Train Neural Networks with Error Weights . . . . . . . . . . . . . . .5-43Normalize Errors of Multiple Outputs . . . . . . . . . . . . . . . . . . .5-46
Multistep Neural Network Prediction . . . . . . . . . . . . . . . . . . .Set Up in Open-Loop Mode . . . . . . . . . . . . . . . . . . . . . . . . . .Multistep Closed-Loop Prediction From Initial Conditions . . .Multistep Closed-Loop Prediction Following KnownSequence . . . . . . . . . . . . . . . . . . . . . . . . . . . . . . . . . . . . .Following Closed-Loop Simulation with Open-LoopSimulation . . . . . . . . . . . . . . . . . . . . . . . . . . . . . . . . . . . .675-515-515-525-525-53Control SystemsIntroduction to Neural Network Control Systems . . . . . . . . . .6-2Design Neural Network Predictive Controller in Simulink . . .System Identification . . . . . . . . . . . . . . . . . . . . . . . . . . . . . . .Predictive Control . . . . . . . . . . . . . . . . . . . . . . . . . . . . . . . . . .Use the Neural Network Predictive Controller Block . . . . . . . .6-46-46-56-6Design NARMA-L2 Neural Controller in Simulink . . . . . . . . .Identification of the NARMA-L2 Model . . . . . . . . . . . . . . . . .NARMA-L2 Controller . . . . . . . . . . . . . . . . . . . . . . . . . . . . . .Use the NARMA-L2 Controller Block . . . . . . . . . . . . . . . . . .6-146-146-166-18Design Model-Reference Neural Controller in Simulink . . . .Use the Model Reference Controller Block . . . . . . . . . . . . . .6-236-24Import-Export Neural Network Simulink Control Systems . .Import and Export Networks . . . . . . . . . . . . . . . . . . . . . . . .Import and Export Training Data . . . . . . . . . . . . . . . . . . . . .6-316-316-35Radial Basis Neural NetworksIntroduction to Radial Basis Neural Networks . . . . . . . . . . . . .Important Radial Basis Functions . . . . . . . . . . . . . . . . . . . . . .7-27-2xi
8Radial Basis Neural Networks . . . . . . . . . . . . . . . . . . . . . . . . . .Neuron Model . . . . . . . . . . . . . . . . . . . . . . . . . . . . . . . . . . . .Network Architecture . . . . . . . . . . . . . . . . . . . . . . . . . . . . . . .Exact Design (newrbe) . . . . . . . . . . . . . . . . . . . . . . . . . . . . . .More Efficient Design (newrb) . . . . . . . . . . . . . . . . . . . . . . . .Examples . . . . . . . . . . . . . . . . . . . . . . . . . . . . . . . . . . . . . . . .7-37-37-47-67-77-8Probabilistic Neural Networks . . . . . . . . . . . . . . . . . . . . . . . . . .Network Architecture . . . . . . . . . . . . . . . . . . . . . . . . . . . . . . .Design (newpnn) . . . . . . . . . . . . . . . . . . . . . . . . . . . . . . . . .7-97-97-10Generalized Regression Neural Networks . . . . . . . . . . . . . . . .Network Architecture . . . . . . . . . . . . . . . . . . . . . . . . . . . . . .Design (newgrnn) . . . . . . . . . . . . . . . . . . . . . . . . . . . . . . . . .7-127-127-14Self-Organizing and Learning Vector QuantizationNetworksIntroduction to Self-Organizing and LVQ . . . . . . . . . . . . . . . . .Important Self-Organizing and LVQ Functions . . . . . . . . . . . . .8-28-2Cluster with a Competitive Neural Network . . . . . . . . . . . . . . .Architecture . . . . . . . . . . . . . . . . . . . . . . . . . . . . . . . . . . . . . .Create a Competitive Neural Network . . . . . . . . . . . . . . . . . .Kohonen Learning Rule (learnk) . . . . . . . . . . . . . . . . . . . . . . .Bias Learning Rule (learncon) . . . . . . . . . . . . . . . . . . . . . . . . .Training . . . . . . . . . . . . . . . . . . . . . . . . . . . . . . . . . . . . . . . . .Graphical Example . . . . . . . . . . . . . . . . . . . . . . . . . . . . . . . . .8-38-38-48-58-58-68-8Cluster with Self-Organizing Map Neural Network . . . . . . . . .Topologies (gridtop, hextop, randtop) . . . . . . . . . . . . . . . . . .Distance Functions (dist, linkdist, mandist, boxdist) . . . . . . .Architecture . . . . . . . . . . . . . . . . . . . . . . . . . . . . . . . . . . . . .Create a Self-Organizing Map Neural Network(selforgmap) . . . . . . . . . . . . . . . . . . . . . . . . . . . . . . . . . . .Training (learnsomb) . . . . . . . . . . . . . . . . . . . . . . . . . . . . . .Examples . . . . . . . . . . . . . . . . . . . . . . . . . . . . . . . . . . . . . . .xiiContents8-98-118-148-178-188-198-22
Learning Vector Quantization (LVQ) Neural Networks . . . . .Architecture . . . . . . . . . . . . . . . . . . . . . . . . . . . . . . . . . . . . .Creating an LVQ Network . . . . . . . . . . . . . . . . . . . . . . . . . . .LVQ1 Learning Rule (learnlv1) . . . . . . . . . . . . . . . . . . . . . . .Training . . . . . . . . . . . . . . . . . . . . . . . . . . . . . . . . . . . . . . . .Supplemental LVQ2.1 Learning Rule (learnlv2) . . . . . . . . . . .9Adaptive Filters and Adaptive TrainingAdaptive Neural Network Filters . . . . . . . . . . . . . . . . . . . . . . . .Adaptive Functions . . . . . . . . . . . . . . . . . . . . . . . . . . . . . . . . .Linear Neuron Model . . . . . . . . . . . . . . . . . . . . . . . . . . . . . . .Adaptive Linear Network Architecture . . . . . . . . . . . . . . . . . .Least Mean Square Error . . . . . . . . . . . . . . . . . . . . . . . . . . . .LMS Algorithm (learnwh) . . . . . . . . . . . . . . . . . . . . . . . . . . . .Adaptive Filtering (adapt) . . . . . . . . . . . . . . . . . . . . . . . . . . . vanced TopicsNeural Networks with Parallel and GPU Computing . . . . . . .Deep Learning . . . . . . . . . . . . . . . . . . . . . . . . . . . . . . . . . . .Modes of Parallelism . . . . . . . . . . . . . . . . . . . . . . . . . . . . . .Distributed Computing . . . . . . . . . . . . . . . . . . . . . . . . . . . . .Single GPU Computing . . . . . . . . . . . . . . . . . . . . . . . . . . . . .Distributed GPU Computing . . . . . . . . . . . . . . . . . . . . . . . . .Parallel Time Series . . . . . . . . . . . . . . . . . . . . . . . . . . . . . .Parallel Availability, Fallbacks, and Feedback . . . . . . . . . . .10-210-210-210-310-510-810-1010-10Optimize Neural Network Training Speed and Memory . . . .Memory Reduction . . . . . . . . . . . . . . . . . . . . . . . . . . . . . . .Fast Elliot Sigmoid . . . . . . . . . . . . . . . . . . . . . . . . . . . . . . .10-1210-1210-12Choose a Multilayer Neural Network Training Function . . .SIN Data Set . . . . . . . . . . . . . . . . . . . . . . . . . . . . . . . . . . .PARITY Data Set . . . . . . . . . . . . . . . . . . . . . . . . . . . . . . . . .10-1610-1710-19xiii
ENGINE Data Set . . . . . . . . . . . . . . . . . . . . . . . . . . . . . . . .CANCER Data Set . . . . . . . . . . . . . . . . . . . . . . . . . . . . . . .CHOLESTEROL Data Set . . . . . . . . . . . . . . . . . . . . . . . . . .DIABETES Data Set . . . . . . . . . . . . . . . . . . . . . . . . . . . . . .Summary . . . . . . . . . . . . . . . . . . . . . . . . . . . . . . . . . . . . . .Improve Neural Network Generalization and Avoid Overfitting .Retraining Neural Networks . . . . . . . . . . . . . . . . . . . . . . . .Multiple Neural Networks . . . . . . . . . . . . . . . . . . . . . . . . .Early Stopping . . . . . . . . . . . . . . . . . . . . . . . . . . . . . . . . . .Index Data Division (divideind) . . . . . . . . . . . . . . . . . . . . . .Random Data Division (dividerand) . . . . . . . . . . . . . . . . . . .Block Data Division (divideblock) . . . . . . . . . . . . . . . . . . . .Interleaved Data Division (divideint) . . . . . . . . . . . . . . . . . .Regularization . . . . . . . . . . . . . . . . . . . . . . . . . . . . . . . . . .Summary and Discussion of Early Stopping andRegularization . . . . . . . . . . . . . . . . . . . . . . . . . . . . . . . .Posttraining Analysis (regression) . . . . . . . . . . . . . . . . . . . 3510-3610-3710-3710-3710-3810-3810-4110-43Edit Shallow Neural Network Properties . . . . . . . . . . . . . . . .Custom Network . . . . . . . . . . . . . . . . . . . . . . . . . . . . . . . .Network Definition . . . . . . . . . . . . . . . . . . . . . . . . . . . . . . .Network Behavior . . . . . . . . . . . . . . . . . . . . . . . . . . . . . . .10-4610-4610-4710-57Custom Neural Network Helper Functions . . . . . . . . . . . . . .10-60Automatically Save Checkpoints During Neural NetworkTraining . . . . . . . . . . . . . . . . . . . . . . . . . . . . . . . . . . . . . . . .10-61Deploy Trained Neural Network Functions . . . . . . . . . . . . . .Deployment Functions and Tools for Trained Networks . . . .Generate Neural Network Functions for ApplicationDeployment . . . . . . . . . . . . . . . . . . . . . . . . . . . . . . . . . .Generate Simulink Diagrams . . . . . . . . . . . . . . . . . . . . . . .10-6310-6310-6410-67Deploy Training of Neural Networks . . . . . . . . . . . . . . . . . . .10-68
1112Historical Neural NetworksHistorical Neural Networks Overview . . . . . . . . . . . . . . . . . . .11-2Perceptron Neural Networks . . . . . . . . . . . . . . . . . . . . . . . . . .Neuron Model . . . . . . . . . . . . . . . . . . . . . . . . . . . . . . . . . . .Perceptron Architecture . . . . . . . . . . . . . . . . . . . . . . . . . . . .Create a Perceptron . . . . . . . . . . . . . . . . . . . . . . . . . . . . . . .Perceptron Learning Rule (learnp) . . . . . . . . . . . . . . . . . . . .Training (train) . . . . . . . . . . . . . . . . . . . . . . . . . . . . . . . . . .Limitations and Cautions . . . . . . . . . . . . . . . . . . . . . . . . . .11-311-311-511-611-811-1011-15Linear Neural Networks . . . . . . . . . . . . . . . . . . . . . . . . . . . . .Neuron Model . . . . . . . . . . . . . . . . . . . . . . . . . . . . . . . . . .Network Architecture . . . . . . . . . . . . . . . . . . . . . . . . . . . . .Least Mean Square Error . . . . . . . . . . . . . . . . . . . . . . . . . .Linear System Design (newlind) . . . . . . . . . . . . . . . . . . . . .Linear Networks with Delays . . . . . . . . . . . . . . . . . . . . . . .LMS Algorithm (learnwh) . . . . . . . . . . . . . . . . . . . . . . . . . .Linear Classification (train) . . . . . . . . . . . . . . . . . . . . . . . .Limitations and Cautions . . . . . . . . . . . . . . . . . . . . . . . . . al Network Object ReferenceNeural Network Object Properties . . . . . . . . . . . . . . . . . . . . . .12-2General . . . . . . . . . . . . . . . . . . . . . . . . . . . . . . . . . . . . . . . .12-2Architecture . . . . . . . . . . . . . . . . . . . . . . . . . . . . . . . . . . . . .12-2Subobject Structures . . . . . . . . . . . . . . . . . . . . . . . . . . . . . .12-6Functions . . . . . . . . . . . . . . . . . . . . . . . . . . . . . . . . . . . . . . .12-8Weight and Bias Values . . . . . . . . . . . . . . . . . . . . . . . . . . . .12-12Neural Network Subobject Properties . . . . . . . . . . . . . . . . . .Inputs . . . . . . . . . . . . . . . . . . . . . . . . . . . . . . . . . . . . . . . .Layers . . . . . . . . . . . . . . . . . . . . . . . . . . . . . . . . . . . . . . . .Outputs . . . . . . . . . . . . . . . . . . . . . . . . . . . . . . . . . . . . . . .Biases . . . . . . . . . . . . . . . . . . . . . . . . . . . . . . . . . . . . . . . .Input Weights . . . . . . . . . . . . . . . . . . . . . . . . . . . . . . . . . . .Layer Weights . . . . . . . . . . . . . . . . . . . . . . . . . . . . . . . . . .12-1412-1412-1612-2212-2412-2512-27xv
13BibliographyNeural Network Toolbox Bibliography . . . . . . . . . . . . . . . . . . .AMathematical NotationMathematics and Code Equivalents . . . . . . . . . . . . . . . . . . . . . .Mathematics Notation to MATLAB Notation . . . . . . . . . . . . . .Figure Notation . . . . . . . . . . . . . . . . . . . . . . . . . . . . . . . . . . .BCContentsA-2A-2A-2Neural Network Blocks for the Simulink EnvironmentNeural Network Simulink Block Library . . . . . . . . . . . . . . . . . .Transfer Function Blocks . . . . . . . . . . . . . . . . . . . . . . . . . . . .Net Input Blocks . . . . . . . . . . . . . . . . . . . . . . . . . . . . . . . . . .Weight Blocks . . . . . . . . . . . . . . . . . . . . . . . . . . . . . . . . . . . .Processing Blocks . . . . . . . . . . . . . . . . . . . . . . . . . . . . . . . . .B-2B-3B-3B-3B-4Deploy Neural Network Simulink Diagrams . . . . . . . . . . . . . . .Example . . . . . . . . . . . . . . . . . . . . . . . . . . . . . . . . . . . . . . . . .Suggested Exercises . . . . . . . . . . . . . . . . . . . . . . . . . . . . . . .Generate Functions and Objects . . . . . . . . . . . . . . . . . . . . . . .B-5B-5B-7B-8Code NotesNeural Network Toolbox Data Conventions . . . . . . . . . . . . . . . .Dimensions . . . . . . . . .
Create Neural Network Object. 1-17 Configure Neural Network Inputs and Outputs. 1-21 Understanding Neural Network Toolbox Data Structures. 1-23 Simulation with Concurrent Inputs in a Static Network. 1-23 Simulation with Sequential Inputs in a Dynamic Network. 1-24 Simulation with Concu