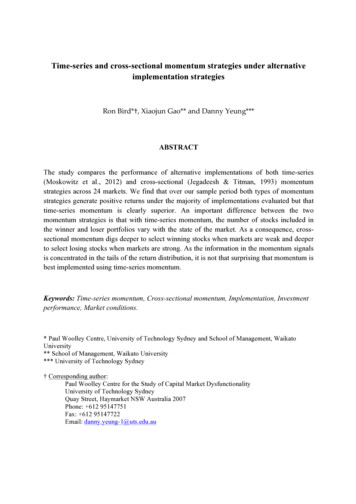
Transcription
Time-series and cross-sectional momentum strategies under alternativeimplementation strategiesRon Bird*†, Xiaojun Gao** and Danny Yeung***ABSTRACTThe study compares the performance of alternative implementations of both time-series(Moskowitz et al., 2012) and cross-sectional (Jegadeesh & Titman, 1993) momentumstrategies across 24 markets. We find that over our sample period both types of momentumstrategies generate positive returns under the majority of implementations evaluated but thattime-series momentum is clearly superior. An important difference between the twomomentum strategies is that with time-series momentum, the number of stocks included inthe winner and loser portfolios vary with the state of the market. As a consequence, crosssectional momentum digs deeper to select winning stocks when markets are weak and deeperto select losing stocks when markets are strong. As the information in the momentum signalsis concentrated in the tails of the return distribution, it is not that surprising that momentum isbest implemented using time-series momentum.Keywords: Time-series momentum, Cross-sectional momentum, Implementation, Investmentperformance, Market conditions.* Paul Woolley Centre, University of Technology Sydney and School of Management, WaikatoUniversity** School of Management, Waikato University*** University of Technology Sydney† Corresponding author:Paul Woolley Centre for the Study of Capital Market DysfunctionalityUniversity of Technology SydneyQuay Street, Haymarket NSW Australia 2007Phone: 612 95147751Fax: 612 95147722Email: danny.yeung-1@uts.edu.au
1. IntroductionNumerous studies have found that profits that can be realised from following a momentumbased strategy of buying recent outperforming stocks (winners) and selling recentunderperforming stocks (losers) (Jegadeesh & Titman, 1993, 2002). The fact that thismomentum strategy has proved robust across time, countries and asset classes has led Fama(1998) to observe that momentum remains the “premier unexplained anomaly”.1The majority of momentum studies have used cross-sectional momentum as the basis forsecurity selection, choosing stocks on the basis of their relative performance over some priorperiod (Jegadeesh & Titman, 1993).2 In a recent study, Moskowitz et al. (2012) proposedtime-series momentum as providing an alternative framework for security selection wheresecurities are chosen on the basis of their absolute performance over some prior period.Moskowitz et al. (2012) found that time-series momentum performed well both in absoluteterms and relative to cross-sectional momentum, across futures markets in equity indices,bonds, currencies and commodities. Baltas and Kosowski (2013) confirmed the strongperformance of time-series momentum strategies and highlighted that they drove theperformance of many hedge funds. In contrast, Menkhoff et al. (2012) when examiningcurrency markets found that cross-sectional momentum outperformed time-series momentum.Although previous studies have evaluated the momentum strategy in numerous marketssettings, by far the bulk of these studies have concentrated on equity markets. Therefore it issomewhat surprising that we are yet to see a comprehensive study that compares cross1The momentum strategy has been documented in US stock market (Jegadeesh & Titman, 1993), Europeanstock markets (Bird & Casavecchia, 2006; Rouwenhorst, 1998), emerging stock markets (Hameed & Kusnadi,2002), international stock markets (Gupta et al., 2010), industries (Moskowitz & Grinblatt, 1999), currencies(Menkhoff et al., 2012) and futures markets (Asness et al., 2013).2The cross-sectional stock selection criteria based on recent returns has been investigated broadly acrossinternational stock markets (Rouwenhorst, 1998), industry markets (Moskowitz & Grinblatt, 1999), currencyand futures markets (Asness et al., 2013; Menkhoff et al., 2012) as well as the selection criteria on the basis of52 week high stock prices(George & Hwang, 2004).2
sectional and time-series momentum in this arena. The main objective of this study is toredress this deficiency by undertaking a study that evaluates the absolute and relativeperformance of these two momentum strategies applying multiple implementations across 24major equity markets3. By evaluating multiple implementations, we not only obtain valuableinsights into the performance of the two momentum strategies but also how they might bestbe implemented. Further, we investigate several factors that may give rise to differences inthe performance of the two strategies such as differences in the characteristics of the stockselected and the performance of the strategies under different markets conditions.We find that time-series momentum generates profits for the majority of the implementationsevaluated in all 24 markets and that that these profits are significant in the vast majority ofinstances. Cross-sectional momentum also proves to be a highly profitable strategy althougha comparison of the performance of the two momentum strategies that time-series momentumis superior particularly under the better implementations. We confirm that both momentumstrategies perform best in up markets and that the superiority of time-series momentum islargely due to its excellent performance in such markets. Indeed, we find that a most likelyexplanation for the better performance of time-series momentum is that it is a consequence ofthe approach to stock selection embedded in time-series momentum being more in tune withmarket conditions. Two of the more interesting markets evaluated in our study are Japan andthe US. Although confirming that cross-sectional momentum is not a good investmentstrategy to pursue in Japan, we find that the same is not true for time-series momentum whichwould seem to offer profitable opportunities under the majority of implementations. Overallwe find that neither form of momentum performs particularly well in the US market over our3The countries examined included the 23 countries included in the MSCI World Index (Australia, Austria,Belgium, Canada, Denmark, Finland, France, Germany, Hong Kong, Ireland, Israel, Italy, Japan, theNetherlands, New Zealand, Norway, Portugal, Singapore, Spain, Sweden, Switzerland, the UK and the US) plusGreece.3
sample period. Digging deeper, we find that this is due to their performance in the US beingmuch worse during the GFC than was the case for the other markets.The remainder of the study is organised as follows. In Section 2, we compare the crosssectional and time-series momentum strategies. Section 3 and 4 discuss the data and thevarious implementations of the momentum trading strategies. In Section 5, we analyse theresults of both momentum strategies while Section 6 provides us with the opportunity of tosummarise our findings.2. Cross-sectional versus time-series momentumCross sectional and time-series momentum both select stocks on the basis of theirperformance over some prior (formation) period. The only difference between the twoapproaches being that cross sectional momentum assigns stocks to the winner and loserportfolio on the basis of their relative performance while time-series momentum assignsstocks on the basis of their absolute performance. With cross-sectional momentum, all stocksare ranked on the basis of their performance over the formation period with the bestperforming stocks (e.g. top 20%) being assigned to the winner portfolio and the worstperforming stocks (e.g. bottom 20%) being assigned to the loser portfolio. With time-seriesmomentum, stocks that realised a return over the formation period above a certain level (e.g. 5%) are assigned to the winner portfolio and those that realise a return below a certain level(e.g. -3%) are assigned to the loser portfolio. In both cases, the profitability of the strategy ismeasured by the aggregate of the return from of a zero-investment strategy of taking a longposition in the winner portfolio and a short position in the loser portfolio.Given that both strategies identify winner and loser stocks based on their past performance, atany point in time they will hold many stocks in common. However more importantly, the4
different stock selection rules embedded in each of the momentum strategies will ensure thatthat there will be differences in their holdings. As we will demonstrate, time-seriesmomentum will assign more stocks to the winner than to the loser portfolio when markets arestrong, with the opposite being the case during periods when markets have been experiencingweak performance. In contrast, cross sectional momentum will always assign the samenumber of stocks to each portfolio irrespective of how the market is performing. Hence thereis a timing element in the selection of stocks embedded in time-series momentum which doesnot exist in cross sectional momentum. An interesting question to ask is whether this keydifference between the two strategies translates into any significant difference in performance(and why), especially in the light of the findings of Cooper et al. (2004) that show theperformance of cross sectional analysis is conditioned by the performance of the market.A major contribution of this paper is to provide evidence on the relative and absoluteperformance of cross sectional and time-series momentum across 24 developed marketsutilising numerous implementations. In particular by comparing “like-for-like” across 768implementations and 24 markets, we will provide the most comprehensive evidence to date asto which of cross sectional and time-series momentum realises the best investment outcomesin equity markets and insights into why the performance of the two strategies differ. Inaddition, we provide important insights into the absolute performance of each of themomentum strategies during a period when markets experienced both rapid growth and rapiddecline and thus encompass conditions when momentum should both thrive and struggle.Jegadeesch and Titman (1993) was the first academic paper to highlight the profitability ofmomentum as an investment strategy when they examined the performance of equallyweighted portfolios of stocks chosen on the basis of their performance over severalcombinations of formation periods and held for several holding periods. They found evidencethat the stocks with best past performance (top 10%) outperformed those stocks with the5
worst past performance (bottom 10%) although the extent of this outperformance was verymuch a function of how the strategy was implemented. They concluded that these anomalousfindings could not be explained by risk factors and suggested the need for a more“sophisticated model of investor behaviour”. Rouwenhorst (1998) conducted the firstinternational study of momentum and found that it delivered outperformance in 11 out of 12European markets. Rouwenhorst (1999) subsequently extended this study to 20 emergingmarkets with similar (albeit slightly weaker) findings. Jegadeesch and Titman (2001) reexamined the momentum strategy over a longer time period and found little evidence of anydeterioration in its performance. They also noted at the time that the available evidencedsupported the persistence of the performance of the over relatively long periods and across allof the developed markets, with the exception of Japan (Asness, 2011; Hanauer, 2014). Themore recent evidence is still strongly supportive of the continuation of the momentumanomaly although there is some suggestion that it might be waning in the US market (Hwangand Rubesam, 2013). The Australian evidence is largely supportive of the momentumstrategy performing well in this market with Gaunt and Gay (2003), Haun and Pavlov (2003),and Demir et al. (2006) all finding momentum profits ranging between 5%pa and 16%pa.However, there is some counterevidence with both Durand et al. (2006) and Brailsford et al.(2012) failing to identify any significant momentum profits.The pervasiveness of the empirical findings on momentum has stimulated numerous studiesseeking an explanation for its apparent continued profitability. These studies can broadly besplit into two camps. One group that proposes more traditional explanations aimed atestablishing that the findings are due to methodological flaws in the research design (e.g.failure to control for risk, transaction costs). A second group who argue that the momentumprofits are attributable to irrational behaviour of investors that results in stocks prices bothunder- and over-reacting to information signals. As the focus of this paper is not on providing6
explanations for past findings, we will not go further with this debate other than to observethat the success of a momentum investment strategy is dependent on stocks trending for asustained period in both directions.3. DataThe sample covers the 24 major stock markets mentioned previously. The period coveredextends from 1990 to 2012 and covers both bull and bear markets. The daily and monthlyreturns and the market value for all active and dead stocks were obtained from ThomsonReuters DataStream 4 .Following Ince and Porter (2006), we apply several screeningprocedures to our sample stocks. For a stock to be included in our analysis in a particularmonth, it must have both return and market capitalization data available. In accordance withChui et al., (2010), monthly returns will be trimmed if the market capitalization of a stock isbelow the bottom fifth percentile of all stocks within a given country in any month.Following McLean et al. (2009), we winsorise daily and monthly returns within a givencountry at the top and bottom 1% to minimise the effects of outliers.Table 1: Summary StatisticsThis table reports for each market the total number of stocks, the average number of stocks eachmonth, the average monthly returns of a portfolio consisting of all stocks in our universe based onequal weight (EW), market weight (MW) and inversed-volatility weight (INVSTD) calculated overvarious periods, the average monthly market value and monthly book-to-market value. We leave outthe first two year as preparation for the momentum strategies and therefore all results in this paper arefrom 1992 to 20124We also conducted the analysis for the U.S. market using CRSP data and obtained substantially the sameresults.7
VOL12marketvaluebook- ‐stocksmonthly(localto- .62The first two columns of Table 1 display the total number of stocks, and the average numberof stocks per month, included for each of our 24 markets. The total number of stocksaggregated to 34,697 across 24 developed markets with the U.S. market having the largestnumber of stocks with 10,041 stocks and Ireland having the smallest number with 131 stocks.We also report the average monthly returns of a portfolio consisting of all stocks availableeach month weighted using one of several weighting schemes: equal weights (EW), marketvalue weights (MW) and four sets of returns for inverse volatility weights (IVOL) dependingon the period over which volatility is measured. Finally, we report the average market valueand book-to market ratio across all of the stocks in each of the 24 markets. These numbers8
will provide a useful reference when examining the characteristics of the winner and loserportfolios in each country.4. Momentum Implementation StrategiesThe success of the momentum strategies depends on stocks trending for sustained periods inboth directions. This is best articulated in the momentum life cycle of Lee and Swaminathan(2000) which suggests that stock prices typically oscillate around their fair value. In such aworld, the success of any momentum strategy will depend on it being based onimplementation rules that are in harmony with the periodicity of the (mis)pricing cycles. Asmomentum trading signals are based on recent pricing movements, they will always be late inidentifying winning and losing securities. However, the more successful momentumstrategies will be those based on implementation rules that results in identifying winners(losers) early in their up-(down) cycle and reversing these positions in (close to) an optimumfashion.There are very limited insights that we get from theory as to what implementation rules willproduce the best outcomes, leaving it largely as an empirical question. In this paper weprovide comprehensive evidence on the absolute and relative performance of time-series andcross-sectional momentum under (almost) all of the implementation rules that have been usedin previous studies and in so doing show how sensitive this performance is to theimplementations chosen and also provide insights into what might be the optimumimplementations. The various implementation rules are discussed below in terms of thecontribution that they make to the two components of the investment process: stock selectionand portfolio construction.9
4.1. Stock SelectionStock selection involves identifying the stocks in which to take both long and short positionsinvest or short. For both momentum strategies, stock selection has the following components:4.1.1.Specifying the prior period over which to measure stock returns (the formationperiod):The importance of this decision is to set the formation period long enough to identify theestablishment of true trends in markets but not too long so as to leave the identification of thetrend until too late in the cycle. In this study we examine four formation periods (J) of three,six, nine and 12 months.4.1.2.Specifying the cut-off rule that identifies stocks as being either winners or losers:With cross-sectional momentum, this involves ranking stocks on the basis of theirperformance over the previous J months and then identifying as winners those stocks thatrank in the top x% of the distribution, and as losers those stocks that rank in the bottom x%.One value for x that we examined in this study was 50% which results in all stocks beingdesignated as either winners or losers. The use of this cut-off would suggest that pastperformance provides a good signal of future performance across the whole range ofperformance outcomes. Dating back to the original study of Jegadeesh and Titman (1993),the strength of the information signal has been shown to degrade as one proceeds down therankings. In order to demonstrate this, we also examine the situation where we set the cut-offat 30% and 16%.With time-series momentum, the cut-off for identifying winners and losers is an absolutenumber(s). Moskowitz et al. (2012) used a method where all stocks that realise a positive pastreturn were identified as winners and those that realise a negative return were identified as10
losers. Another similar method that we use with time-series momentum is to set the cut-off asthe market return over the formation period. Of course, each of these rules results in everystock in the investment universe being classified as either a winner or a loser. In order tomatch the situation where the cut-offs under cross-sectional momentum are set at 30% (16%),we set symmetric upper and lower cut-offs for time-series momentum which result onaverage in 60% (32%) of the investment universe being classified as either a winner or a loserwhen measured across the whole sample period.54.2. Portfolio ConstructionThe portfolio construction decisions involve determining at the time of each rebalancing, theproportion of the portfolio (weights) that are allocated to the winning stocks and the losingstocks in their respective portfolios. There are three separate decisions that in combinationdetermine these weights:4.2.1.The holding periodThis is a rule common to both momentum strategies that determines the period that aparticular stock is held once it is included in either the winner or the loser portfolio. Forexample, if the holding period is six months, then a stock acquisition will be reversed sixmonths after the stock has been acquired. As mentioned earlier, the implementation ruleshave to be in harmony with the periodicity of the oscillations of the typical stock. In otherwords, the aggregate of the formation and holding periods should approximate the period ofthe upward and downward cycles for the typical stock. In this study we examine holdingperiods (H) of three, six, nine and 12 months.5The cut-offs were chosen because they equate with cut-offs which are determined as being 0.5 (1.0)standard deviations from the mean in order over the same to allocate 60% (32%) of the stocks eitherthe winner or loser portfolios11
4.2.2.The period for portfolio rebalancing:One portfolio rebalancing strategy that we examine is a buy and hold strategy where theportfolio is rebalanced at the end of each holding period (BHAR). For example, if the holdingperiod is six months then the portfolio is rebalanced every six months with the portfolioacquired six months ago being sold and replaced by a new portfolio. The alternative weconsider is that the portfolio is rebalanced every month irrespective of the holding period forthe stocks (CAR). Again assume a holding period of six months which means that withmonthly rebalancing, the portfolio holdings acquired six months will be replaced with newholdings which means that approximately one-sixth of the portfolio will be turned over eachmonth. Jegadeesh and Titman (1993) considered these two strategies for rebalancing andfound monthly rebalancing to be superior.A further matter to take into account is the role that the bid-ask spread takes in explainingmomentum. It is quite possible that stocks that have performed well (poorly) over some priorperiod are near the top (bottom) of the bid-ask spread. This being the case, particularly shortterm future performance may be eroded by pricing moving back towards the midpoint of thebid-ask spread. This raises the possibility that better performance might be realised bydelaying trading for a short time after a stock has been identified as a winner or a loser. Inthis study we also look at buy-and-hold strategies and monthly rebalancing where trading isdelayed by one month. This means that in total we consider four rebalancing strategies:BHAR (0), BHAR (1), CAR (0) and CAR (1).4.2.3.The determination of the weights assigned to stocks:Once it is determined what assets to include in a portfolio, it is then necessary to allocateportions of the total funds available in specific stocks. The two most common methods12
evaluated in the academic literature are to equally weight each stock (EW) or to apportionfunds to stocks based on the market weight of the stock’s equity (MW). An importantdifference between these two methods being that by equally weighting the portfolio holdingsare more skewed towards stock in smaller companies. A third method of weighting stocksused in this study is to base the proportion of funds allocated to each stock on the inverse ofthe volatility of the returns of the stocks to be included in each portfolio (I-VOL)6. This issimilar to the method employed in Moskowitz et al. (2012) and tilts the portfolios towardsstocks with lower volatility and so produces investment portfolios with lower risk.A summary of the 768 implementations that are examined for each of cross-sectional andtime-series momentum implementation strategies to be examined is set out in Table 2.Table 2. Implementation Options for Time-Series and Cross-Sectional MomentumStock selection criteriaFormation periodsInvest inwholesampleStockselectionCut-offPointInvest in32% (60%)of sampleRebalancing regime7PortfolioConstructionPortfolio weightsHolding periodsCross-sectionalTime-series momentummomentumJ 3, 6, 9 and 12 monthsThe absolute cut off point forWinner/Loser portfoliowinner and loser portfolios iscontains top/bottom 50%a 0% return (or alternatively,stocks in the entire marketthe market return for theperiod)Winner/Loser portfoliocontains top/bottom 16%(30%) of stocks in theentire marketAbsolute cut off points ofwinner (loser) portfolios arecalculated as the averagemonthly return on all stocksover the entire sample periodplus/minus approximately one(half) standard deviation.CAR(0) and CAR(1)BHAR(0) and BHAR(1)Equal weight (EW)Market value weight (MW)Inversed-volatility weight (I-VOL)H 3, 6, 9 and 12 months6The volatilities are estimated using daily returns over the formation period used in the particularimplementation.7To be consistent with Fama (1998), BHAR indicates momentum return has been calculated by buy-and holdconstruction and CAR indicates momentum return has been calculated by monthly rebalancing construction.BHAR (0)/BARH (1) present BHAR with zero/1 month gap between formation and holding periods.13
5. The ResultsTable 3 reports the average monthly returns for 16 (J x H) time-series momentum strategiesacross the 24 markets along with an indication of whether the returns are significant at the 1%(1), 5% (5) and 10% (10) levels8. The specific strategy being to form a long portfolioconsisting of the identified winning stocks and a short portfolio consisting of the identifiedlosing stocks with the average monthly returns reported being the aggregate of the monthlyreturns on the two portfolios. The reported results all relate to the implementation where thecut-offs for selecting the stocks to be included in the cross-sectional momentum portfolios areset at 16%.9 There were 192 implementations evaluated for each time-series and crosssectional momentum but we report only on the returns on what we call the “best”, “median”and “worst” implementations.10 In order to identify these three sets of results we aggregatethe monthly returns for the time-series and cross- sectional momentum for each of theimplementations, rank them and then choose the best (highest ranking), the median (middleranking) and the worst (lowest ranking). A consideration of the results for theseimplementations provides useful insights into the absolute and relative performance of thetwo momentum strategies, how this profitability is conditioned by the implementation used,and so what implementation strategies are best to apply.8Newey-West t-statistics were calculated in order to determine the significance of the average monthly returns.In order to determine the cut-offs for time-series momentum for each market, we calculated the mean andstandard deviation of the returns for all stocks across the total sample period in each market and set the cut-offsat one standard deviation above, and one-standard deviation below, the mean. For example, the cut-offs set forAustralia were 5.99%% for the winner stocks and -4.14%% for the loser stocks. This is an effective “in-sample”means of calculating the cut-offs as they are based on the mean and standard deviations of the returns of thestocks in our universe over the entire sample period. We also determined and applied cut-offs determined “outof-sample” by setting new cut-offs each calendar year based on the history of stock returns realised in pastperiod. When applying these “out-of-sample” cut-offs, we obtained results almost identical to those reported inthis paper10Simply presenting the tables for the performance of all the implementations considered would take 36 pagesso we have chosen to encapsulate the insights provided across all the implementations by largely restricting ourreporting to these three implementations.914
Table 3: Momentum Performance under Best, Median and Worst ImplementationThis table reports the average monthly returns of losers (L), winners (W), momentum (W-L) portfolios for timeseries (TSM) and cross-sectional (CSM) momentum strategies and the return difference between TSM and CSMfor the best, median and worst implementation in each of the markets. These implementations are determined byadding the average monthly returns of the two momentum strategies under each of the implementations, rankingthem and the identifying the best, median and BestMedianWorstImplementationTime- ‐series(%)JxHWeightConstructionWLW- ‐L119x3MWBHAR(0)2.75- AR(1)0.981.38- ‐0.401112x3IVOLCAR(0)1.21- ‐0.361.5751012x3MWCAR(0)0.86- ‐0.161.0112x12MWBHAR(1)- ‐0.050.53- ‐0.571112x3IVOLCAR(1)1.19- ‐0.601.791112X9EWBHAR(1)0.76- ‐0.200.973x9MWBHAR(0)0.330.36- ‐0.03119x3MWBHAR(0)3.04- )1.721.480.24119x3IVOLBHAR(1)1.30- )0.780.580.191112x6MWBHAR(0)3.000.012.98
strategies perform best in up markets and that the superiority of time-series momentum is largely due to its excellent performance in such markets. Indeed, we find that a most likely explanation for the better performance of time-