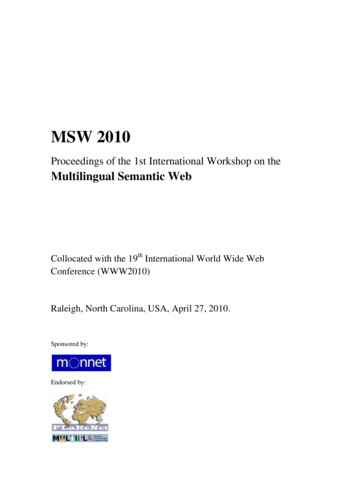
Transcription
MSW 2010Proceedings of the 1st International Workshop on theMultilingual Semantic WebCollocated with the 19th International World Wide WebConference (WWW2010)Raleigh, North Carolina, USA, April 27, 2010.Sponsored by:Endorsed by:
PrefaceAlthough knowledge processing on the Semantic Web is inherently languageindependent, human interaction with semantically structured and linked data will remaininherently language-based as this will be done preferably by use of text or speech input,in many different languages. Semantic Web development will therefore be increasinglyconcerned with knowledge access to and generation in/from multiple languages.Multilinguality can be therefore considered an emerging challenge to Semantic Webdevelopment and to its global acceptance – across language communities around theworld. The MSW workshop was concerned with discussion of new infrastructures,architectures, algorithms, etc., whose goal is to enable an easy adaptation of SemanticWeb applications to multiple languages, addressing issues in representation, extraction,integration, presentation, and so on. This workshop brought together researchers fromseveral distinct communities, including natural language processing, computationallinguistics, human-computer interaction, artificial intelligence and the Semantic Web.There were 12 submissions to the workshop, from which the program committeeaccepted 4 as full papers, 2 as short papers, and 2 as position papers. Taking intoaccount only the full and short papers the selection rate amounts to 50%. The acceptedpapers cover a variety of topics regarding the use of multilingual ontologies withdifferent purposes that range from information extraction and data querying to userprofile enrichment, as well as multilingualism modeling issues, controlled languages ormultilingual ontology mapping for the future Semantic Web. The MSW Workshopprogram also included a keynote talk by Professor Sergei Nirenburg entitled “TheOntology-Lexicon Space for Dealing with Meaning in Natural Language(s): Extraction,Manipulation, Acquisition, Uses, Needs, Lessons Learned, Hopes”.We would like to thank the authors for providing the content of the program. We wouldlike to express our gratitude to the program committee for their work on reviewingpapers and providing interesting feedback to authors. We would also like to thankBehrang Qasemizadeh for his technical support. And finally, we kindly acknowledgethe European Union and the Science Foundation Ireland for their support of theworkshop through research grants for Monnet (FP7-248458) and Lion2(SFI/08/CE/I1380), and to the European Project FlareNet (ECP-2007-LANG-617001)and the German Project Multipla (DFG-38457858) for their endorsement.Paul BuitelaarPhilipp CimianoElena Montiel-PonsodaApril, 2010ii
Table of ContentsOntologies for Multilingual Extraction 1Deryle W. Lonsdale, David W. Embley and Stephen W. LiddleCLOVA: An Architecture for Cross-Language Semantic Data Querying . 5John McCrae, Jesús R. Campaña and Philipp CimianoCross-Lingual Ontology Mapping and Its Use on the Multilingual Semantic Web . 13Bo Fu, Rob Brennan and Declan O'SullivanRivière or Fleuve? Modelling Multilinguality in the Hydrographical Domain 21Guadalupe Aguado-de-Cea, Asunción Gómez-Pérez, Elena Montiel-Ponsoda and LuisM. Vilches-BlázquezWord Order Based Analysis of Given and New Information in Controlled SyntheticLanguages . 29Normunds GruzitisMetadata Synchronization between Bilingual Resources: Case Study in Wikipedia . 35Eun-kyung Kim, Matthias Weidl and Key-Sun ChoiModeling Wordlists via Semantic Web . 39Shakthi Poornima and Jeff GoodMultilingual Ontology-based User Profile Enrichment . 41Ernesto William De Luca, Till Plumbaum, Jérôme Kunegis, Sahin Albayrakiii
MSW 2010 OrganizationOrganizing CommitteePaul BuitelaarUnit for Natural Language Processing, DERI - National University of Ireland, Galwayhttp://www.paulbuitelaar.net/Philipp CimianoSemantic Computing Group, Cognitive Interaction Technology Excellence Cluster (CITEC)Bielefeld University, Germanyhttp://www.cimiano.deElena Montiel-PonsodaOntology Engineering Group, Departamento de Inteligencia ArtificialUniversidad Politécnica de Madrid, Españaemontiel(at)delicias.dia.fi.upm.esProgram CommitteeGuadalupe Aguado de Cea, Universidad Politécnica de Madrid - Artificial IntelligenceDepartment, SpainNathalie Aussenac-Gilles, IRIT, Toulouse - Knowledge Engineering, Cognition andCooperation, FranceTimothy Baldwin, Univ. of Melbourne - Language Technology Group, AustraliaRoberto Basili, Universita Tor Vergata, Rome - Artificial Intelligence group, ItalyChris Bizer, Freie Universität Berlin - Web-based Systems Group, GermanyFrancis Bond, NICT - Language Infrastructure Group, JapanChristopher Brewster, Aston University - Operations and Information Management Group,UKDan Brickley, Vrije Universiteit & FOAF project, the NetherlandsNicoletta Calzolari, ILC-CNR - Computational Linguistics Institute, ItalyManuel Tomas Carrasco Benitez, European Commission, LuxembourgJeremy Carroll, TopQuadrant Inc., USAKey-Sun Choi, KAIST - Semantic Web Research Center, South-KoreaThierry Declerck, DFKI - Language Technology Lab, GermanyAldo Gangemi, ISTC-CNR - Semantic Technology Laboratory, ItalyAsuncion Gómez Pérez, Universidad Politécnica de Madrid - Artificial IntelligenceDepartment, SpainGregory Grefenstette, Exalead, FranceSiegfried Handschuh, DERI, Nat. Univ. of Ireland, Galway - Semantic Collaborative SoftwareUnit, IrelandMichael Hausenblas, DERI, Nat. Univ. of Ireland, Galway - Data Intensive InfrastructuresUnit, IrelandIvan Herman, CWI & W3C, the NetherlandsChu-Ren Huang, Hong Kong Polytechnic University - Dept. of Chinese and Bilingual Studies,Hong KongAntoine Isaac, Vrije Universiteit - Web and Media Group, the NetherlandsErnesto William De Luca, Universität Magdeburg - Data and Knowledge Engineering Group,GermanyPaola Monachesi, Universiteit Utrecht - Institute of Linguistics, the NetherlandsSergei Nirenburg, University of Maryland - Institute for Language and InformationTechnologies, USAiv
Alessandro Oltramari, ISTC-CNR - Laboratory for Applied Ontology & Univ. of Padua, ItalyJacco van Ossenbruggen, CWI - Semantic Media Interfaces & VU - Intelligent Systems, theNetherlandsWim Peters, University of Sheffield - Natural Language Processing group, UKLaurette Pretorius, University of South Africa - School of Computing, South-AfricaJames Pustejovsky, Brandeis University – CS Dept., Lab for Linguistics and Computation,USAMaarten de Rijke, Univ. van Amsterdam - Information and Language Processing Systems, theNetherlandsFelix Sasaki, W3C deutsch-österr. Büro & FH Potsdam, GermanyMartin Volk, Universität Zürich - Institute of Computational Linguistics, SwitzerlandPiek Vossen, Vrije Universiteit - Dept. of Language, Cognition and Communication, theNetherlandsYong Yu, Shanghai Jiao Tong University - APEX Data & Knowledge Management Lab, Chinav
Ontologies for Multilingual ExtractionDeryle W. LonsdaleDavid W. EmbleyStephen W. LiddleLinguistics & English Lang.Brigham Young UniversityComputer ScienceBrigham Young UniversityInformation SystemsBrigham Young eduABSTRACTunderstanding the eventual need to extend OntoES toother languages. This appears to be an opportune timefor our group to enter the area of multilingual information extraction and show how the DEG infrastructureis poised to make significant contributions in this areaas it has already has in extracting English information.There are currently a few efforts in the area of multilingual information extraction. Some focus on verynarrow domains, such as technical information for oildrilling and exploration in Norwegian and English. Others are more general but involve more than two languages, such as accessing European train system schedules. The U.S. government (NIST TREC), the European Union (7th Framework CLEF), and Japan (NTCIR) all have initiatives to help further the developmentand evaluation of multilingual information retrieval anddata extraction systems. Of course, Google and othercompanies interested in web content and market shareare enabling multilingual access to the Internet.Almost all of the existing efforts involve a typical scenario that might include: collecting a query in the user’slanguage, translating that query into the language ofthe web pages to be searched, locating the answers, andthen returning the relevant results to the user or tosomeone who can help the user understand their content. This approach is fraught with problems since machine translation (MT), a core component in the process, is still a developing technology.For reasons discussed below, we believe that our approach has technical and linguistic merit, and can introduce a fresh perspective on multilingual informationextraction. Our ontology-based techniques are ideal forextracting content in various languages without having to rely directly on MT. By carefully developing theknowledge resources necessary, we can extend DEGtype processing to other languages in a modular fashion.In our global society, multilingual barriers sometimesprohibit and often discourage people from accessing awider variety of goods and services. We propose multilingual extraction ontologies as an approach to resolving these issues. Our ontologies provide a conceptualframework for a narrow domain of interest. Groundingnarrow-domain ontologies linguistically enables them tomap relevant utterances and text to meaningful concepts in the ontology. Our prior work includes leveraging large-scale lexicons and terminology resources forgrounding and augmenting ontological content [14]. Linguistically grounding ontologies in multiple languagesenables cross-language communication within the scopeof the various ontologies’ domains. We quantify the success of linguistically grounded ontologies by measuringprecision and recall of extracted concepts, and we cangauge the success of automated cross-linguistic-mappingconstruction by measuring the speed of creation and theaccuracy of generated lexical resources.1. INTRODUCTIONThough English has so far served as the principallanguage for Internet use (with currently 28.7% of allusers), its relative importance is rapidly diminishing.Chinese users, for example, comprise 21.7% of Internetusers and their growth in numbers between 2000 and2009 has been 1,018.7%; the growth in Spanish usershas been 631.3% over the last decade. Since more people want to access web information in more languages,this poses a substantial challenge and opportunity forresearch and business organizations whose interest is inproviding multilingual access to web content.The BYU Data Extraction research Group (DEG)1has worked for years on tools—such as its OntologyExtraction System (OntoES)—to enable access to webcontent of various types: car advertisements, obituaries, clinical trial data, and biomedical information. Thegroup to date has focused on English web data, while2. THE ONTOLOGY-BASED APPROACH2.1 Extraction Ontologies1Just over a decade ago, the BYU Data-Extractionresearch Group (DEG) began its work on informationextraction. In a 1999 paper, DEG researchers describedan efficacious way to combine ontologies with simplenatural language processing [5].2 The idea is to de-This work was funded in part by U.S. National Science Foundation grants for the TIDIE (IIS-0083127)and TANGO (IIS-0414644) projects.Copyright is held by the author/owner(s).WWW2010, April 26-30, 2010, Raleigh, North Carolina.21Recently, others have begun to combine ontologies with
clare a narrow domain ontology for an application ofinterest and augment its concepts with linguistic recognizers. Coupling recognizers with conceptual modelingturns a conceptual ontology into an extraction ontology. When applied to data-rich semi-structured text, anextraction ontology recognizes linguistic elements thatidentify concept instances for the object and relationship sets in the ontology’s conceptual model. We callour system OntoES, Ontology-based Extraction System.Consider, for example, a typical car ad. Its contentcan be modeled with a conceptual ontology such as thatshown in Figure 1. With linguistic recognizers added forconcepts such Make, Model, Year, Price, and Mileage,the domain ontology becomes an extraction ontology.Figure 1: Extraction Ontology for Car Ads.We have developed a form-based tool [15] that helpsusers to develop ontologies including declaring recognizers and associating them with ontological concepts.It also permits users to specify regular expressions thatrecognize traditional value phrases for car prices such as“ 15,900”, “7,595”, and “ 9500”—with optional dollarsigns and commas. Users can also declare additional recognizers for other expected price expressions such as “15grand”. To help make recognizers more precise, userscan declare exception expressions, left and right context expressions, units expressions, and even keywordphrases such as “MSRP” and “our price” to help sortout various prices that might appear. Figure 2 showssnippets from recognizer declarations for car ads data.Applying the recognizers of all the concepts in a carads extraction ontology to a car ad annotates, extracts,and organizes the facts from that ad. The result is amachine-readable cache of facts that users can query oruse to perform data analysis or other automated tasks.3To verify that a carefully designed extraction ontology for car ads can indeed annotate, extract, and organize facts for query and analysis, DEG researchers havenatural language processing [11, 2]. The combinationhas been called “linguistically grounding ontologies.”3See http://deg.byu.edu for a working online demonstration of the system.Priceinternal representation: Integerexternal representation: \ [1-9]\d{0,2},?\d{3} \d?\d [Gg]rand .context keywords: price asking obo neg(\. otiable) .LessThan(p1: Price, p2: Price) returns (Boolean)context keywords: (less than under .)\s*{p2} .Make.external representation: CarMake.lexicon.Figure 2: Sample Recognizer Declarations forCar Ads.conducted experiments with hundreds of car ads fromvarious on-line sources containing thousands of fact instances. In one experiment, when an existing OntoEScar ads ontology was hand-tuned on a corpus of 100development documents and then tested on an unseencorpus of about 110 car ads, the system extracted 1003attributes with with recall measures of 94% and precision measures nearing 100% [6].Recently, DEG researchers have experimented withinformation extraction in Japanese. Figure 3 shows anOntoES extraction ontology that can extract information from Japanese car ads analogous to the Englishone shown earlier. The concept names are in Japaneseas are the regular-expression recognizers. Yen amountsrange from 10,000 yen to 9,999,999 yen whereas dollaramounts range from 100 to 99,999. The critical observation is that the structure of the Japanese ontologyis identical to the structure of the English ontology.This type of ontology-based matching across languagesat the lexical level indicates a possible strategy for providing a cross-linguistic bridge through concepts ratherthan relying on traditional means of translation. Similar approaches have been tried in such areas as machinetranslation (e.g. [4]) and cross-linguistic information retrieval [12].Figure 3: Japanese Extraction Ontology for CarAds.2
As currently implemented, OntoES extraction ontologies can “read” and “write” in any single language. Thecar-ad examples here are in English and Japanese, butextraction ontologies work the same for all languages.To “read” means to recognize instance values for ontological concepts, to extract them, and to appropriatelylink related values together based on the associated conceptual relationships and constraints. To “write” meansto list the facts recorded in the ontological structure.Having “read” a typical car ad, OntoES might write:patterns [8], we expect to fully exploit patterns in text.2.2 Multilingual MappingsWe are extending in a principled way the cross-linguistic effectiveness of our OntoES system by adapting it for use in processing data-rich documents in languages other than English. Though the OntoES systemwas originally designed to handle English-language documents, it was implemented according to standard webrelated software engineering principles and best practices: version control, integrated development enviroments, standardized data markup and encoding (XML,RDF, and OWL), Unicode character representation, andtractability (SWRL rules and Pellet-based reasoning).Consequently, we anticipate that internationalization ofthe system should be relatively straightforward, not requiring wholesale rewrites of crucial components. Thisshould allow us to handle web pages in any language,given appropriate linguistic knowledge sources. SinceOntoES does not need to parse out the grammaticalstructure of webpage text, only lower-level lexical (wordbased) information is necessary for linguistic processing.The system’s lexical knowledge is highly modular,with specific resources encoded as user-selectable lexicons. The information used to build up existing content for the English lexicons includes a mix of implicitknowledge and existing resources. Some lexicon entrieswere created by students during class and project work;other entries were developed from existing lexical resources (e.g. the US Census Bureau for personal names,the World Factbook for country names, Ethnologue forlanguage names, etc.). We are developing analogous lexicons for other languages, and adapting OntoES as necessary to accommodate them in its processing. As wasthe case for English, this involves some hand-crafting ofrelevant material, as well as finding and converting existing data sources in other languages for targeted typesof lexical information. Often this is relatively straightforward: for example, WordNet is a sizable and important component for English OntoES, and similar andcompatible resources exist for other languages. However, we also need to rely on linguistic knowledge andexperience to find, convert, and implement appropriatecross-linguistic lexical resources.In the realm of cross-linguistic extraction systems,OntoES has a clear advantage. We claim that ontologies, which lie at the crux of our extraction approach,can serve as viable interlinguas. We are currently substantiating this claim. Since an ontology represents aconceptualization of items and relationships of interest(e.g. interesting properties of a car, information neededto set up a doctor’s appointment, etc.), a given ontologyshould be appropriate cross-linguistically with perhapsoccasionally some slight cultural adaptation. For example, in our prior work on extraction from obituaries [5]we found that worldwide cultural and dialect differenceswere readily apparent even in English material. Certainterms for events like “tenth day kriya”, “obsequies”,and “cortege” were found only in English obituaries announcing events outside of America. Since our lexicalresources serve as a “grounding” of the lowest-level concepts from ontologies with the lexical content of the webYear: 1984Make: DodgeModel: W100Price: 2,000Feature: 4x4Feature: PickupAccessory: 12.5x35” mud tiresIn addition, based on the constraints, OntoES knowsand can write several meta statements about an ontology. Examples: “an Accessory is a F eature” (whitetriangles denote hyponym/hypernym is-a constraints);“T rim is part of M odelT rim” (black triangles denotemeronym/holonym is-part-of constraints), “Car has atmost one M ake” (the participation constraint 0:1 onCar for M ake denotes that Car objects in car ads associate with M ake names between 0 and 1 times, or “atmost once”).As currently implemented, however, OntoES cannotread in one language and write in another. This crosslingui
The MSW Workshop program also included a keynote talk by Professor Sergei Nirenburg entitled “The Ontology-Lexicon Space for Dealing with Meaning in Natural Language(s): Extraction, Manipulation, Acquisition, Uses, Needs, Lessons Learned, Hopes”. We would like to thank the authors for provid