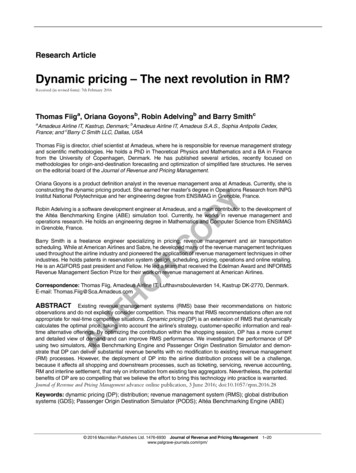
Transcription
Research ArticleDynamic pricing – The next revolution in RM?Received (in revised form): 7th February 2016Thomas Fiiga, Oriana Goyonsb, Robin Adelvingb and Barry SmithcaAmadeus Airline IT, Kastrup, Denmark; bAmadeus Airline IT, Amadeus S.A.S., Sophia Antipolis Cedex,France; and cBarry C Smith LLC, Dallas, USAThomas Fiig is director, chief scientist at Amadeus, where he is responsible for revenue management strategyand scientific methodologies. He holds a PhD in Theoretical Physics and Mathematics and a BA in Financefrom the University of Copenhagen, Denmark. He has published several articles, recently focused onmethodologies for origin-and-destination forecasting and optimization of simplified fare structures. He serveson the editorial board of the Journal of Revenue and Pricing Management.PYOriana Goyons is a product definition analyst in the revenue management area at Amadeus. Currently, she isconstructing the dynamic pricing product. She earned her master’s degree in Operations Research from INPGInstitut National Polytechnique and her engineering degree from ENSIMAG in Grenoble, France.ORobin Adelving is a software development engineer at Amadeus, and a main contributor to the development ofthe Altéa Benchmarking Engine (ABE) simulation tool. Currently, he works in revenue management andoperations research. He holds an engineering degree in Mathematics and Computer Science from ENSIMAGin Grenoble, France.ORCBarry Smith is a freelance engineer specializing in pricing, revenue management and air transportationscheduling. While at American Airlines and Sabre, he developed many of the revenue management techniquesused throughout the airline industry and pioneered the application of revenue management techniques in otherindustries. He holds patents in reservation system design, scheduling, pricing, operations and online retailing.He is an AGIFORS past president and Fellow. He led a team that received the Edelman Award and INFORMSRevenue Management Section Prize for their work on revenue management at American Airlines.THCorrespondence: Thomas Fiig, Amadeus Airline IT, Lufthavnsboulevarden 14, Kastrup DK-2770, Denmark.E-mail: Thomas.Fiig@Sca.Amadeus.comAUABSTRACT Existing revenue management systems (RMS) base their recommendations on historicobservations and do not explicitly consider competition. This means that RMS recommendations often are notappropriate for real-time competitive situations. Dynamic pricing (DP) is an extension of RMS that dynamicallycalculates the optimal price, taking into account the airline’s strategy, customer-specific information and realtime alternative offerings. By optimizing the contribution within the shopping session, DP has a more currentand detailed view of demand and can improve RMS performance. We investigated the performance of DPusing two simulators, Altéa Benchmarking Engine and Passenger Origin Destination Simulator and demonstrate that DP can deliver substantial revenue benefits with no modification to existing revenue management(RM) processes. However, the deployment of DP into the airline distribution process will be a challenge,because it affects all shopping and downstream processes, such as ticketing, servicing, revenue accounting,RM and interline settlement, that rely on information from existing fare aggregators. Nevertheless, the potentialbenefits of DP are so compelling that we believe the effort to bring this technology into practice is warranted.Journal of Revenue and Pricing Management advance online publication, 3 June 2016; doi:10.1057/rpm.2016.28Keywords: dynamic pricing (DP); distribution; revenue management system (RMS); global distributionsystems (GDS); Passenger Origin Destination Simulator (PODS); Altéa Benchmarking Engine (ABE) 2016 Macmillan Publishers Ltd. 1476-6930 Journal of Revenue and Pricing Managementwww.palgrave-journals.com/rpm/1–20
Fiig et alIndustry-changingDP will revolutionize RM in two significantways. First, DP will change RMS from a purelyoffline process involving processing of historicaldata into a dynamic, real-time pricing engine.Second, DP will disrupt the current distributionprocesses. With DP, fares and taxes filed to fareaggregators such as ATPCO are no longersufficient for price computation. This affects allshopping and downstream processes (for example, ticketing, servicing, revenue accounting,RM and interline settlement) that rely onexisting information from fare aggregators.While DP seemed farfetched in the past, theadvent of the New Distribution Capabilitystandard has made DP both realistic and desirable for airlines.AUTHORCOThe Internet has fundamentally transformedhow airline customers shop, bringing unprecedented price transparency to the marketplaceand making revenue management (RM) decisions more dependent on competition. Existingrevenue management systems (RMS), however,are blind to real-time competitive situations.The RMS uses historical data to perform forecasting and optimization and then relies onan offline upload of bid prices to the onlineInventory system. RMS takes competition intoaccount only indirectly (and in an averagedway) through its effect on bid prices. Hence, anRMS will provide identical recommendationsregardless of the real-time competitive scenario.The current RMS paradigm, which relies onthe process of fare filing and then having RMSopening or closing fares, is limited in two ways.First, fares are static and published before beingoffered publicly. Second, these fares and availability controls are applied in an identical fashion across multiple shopping sessions, duringwhich the optimal price may vary significantly.We believe that in the current businessenvironment, dynamic pricing (DP) can improveRMS performance. DP provides benefits in thefollowing areas:Real-time responsiveness: The choice set withina shopping session depends on competitors’schedules and available prices. While schedulesand prices are relatively static, availabilitychanges frequently. DP allows airlines to adjustprices in real time during the shopping session(without the need to file fares in advance) basedon the competitors’ offers.Customer segmentation: DP can observe customer characteristics during the shopping processand may improve its estimate of a customer’swillingness to pay (WTP), expectations ofservice quality or preferred time of day. Withthis ability, the airline can improve its productofferings to the customer.Pricing precision: Even though airlines maypublish prices several times per day, they offer arelatively fixed set of prices for each market,with gaps between prices. The best prices for anairline’s products during a given shopping session may lie between or outside of the range ofpublished fares. DP improves pricing precisionby offering a continuous price.PYINTRODUCTION ANDMOTIVATION2 2016 Macmillan Publishers Ltd. 1476-6930ContributionTo our knowledge, no published work providesan investigation of the practical aspects, deployment or operation of DP, nor are we aware of anyDP implementation within the airline industry.Our goal is to investigate DP’s behaviorand anticipate its likely revenue performanceto supplement existing theoretical work.We demonstrate consistent benefits for earlyadopters of DP through simple examples andrealistic simulations. We also show that DP canbenefit multiple airlines in a single market.Significant work remains to be done to bringDP into everyday use. In this article we providecontext and motivation for these next steps.OrganizationThis article is organized as follows: After aliterature review, we define DP and discuss theJournal of Revenue and Pricing Management1–20
Dynamic pricing – The next revolution in RM?OnlineOfflineDistributionMarketRevenue ricesRetail onlineOptimize MSPricesRMS onlineOptimize conversionRMS offlineOptimize airlinerevenueOptimize airlinerevenueCompetitor MatchingAgent Content andPricing Management Smith et al (2007) Rao and Smith(2006) Lua (2007)PricingFare managementOptimize airlinerevenueDynamic Availability Fare Matching Vinod (2010)Ryzin (1994) Zhao and ZhengStrategic Pricing Vinod et al (2009)(2000) Westermann (2006) Ratliff (2015) Isler and D'Souza(2009) Gallego and vanGame theory (OALresponsive) Gallego et al (2006) Isler and Imhof(2008) Gallego and Hu(2014)Competition in RMS(OAL unresponsive) D’Huart (2010) Zhang and Kallesen(2008)Figure 1: Literature review – Competitive awareness.PYOThe airline deregulation process began with theenactment in the US of the Airline DeregulationAct of 1978. Since then, deregulation has beenimplemented to various degrees elsewhere in theworld. Deregulation has resulted in price volatilityand a dramatic increase in the number of fares.To remain competitive, airlines had to updatefares more frequently in response to competitors’ actions. In fare-matching, which is still aprevalent form of competitive response, airlinesuse monitoring tools to identify competitors’fare changes and, based on preset rules, automatically match the competitor’s fares (for moreinformation on the fare-matching practice, seeVinod, 2010).Fare-matching is insufficient for the competitive awareness because it does not respond inreal time and ignores availability and differencesin schedule quality.RLITERATURE REVIEWFare-matchingCfundamental difference between DP and RMS.This is followed by the presentation of revenueresults from two simulators, Altéa BenchmarkingEngine (ABE) and Passenger Origin DestinationSimulator (PODS). Finally, we discuss limitations and ideas for future research, and presentour conclusions.AUTHOFigure 1 shows how a customer request for anitinerary is passed through the retailer, distributorand to the airline’s Inventory system for evaluation. The Inventory system performs the onlineRMS control that calculates availability, which,via the pricing system, determines price quotesthat are communicated back to the customer.RMS offline processes, which include forecastingand optimization, calculate bid prices that areused in online RMS control. Pricing is used bothby RMS for yield estimates and by distributors(for example, issuers of tickets).We have categorized references (and theirimplied solutions) by actor (retailer, distributor,airline), further split into online or offline.Identifying the actors is important becausethe objective of each is different, and hence,the proposed models and solutions cited in theliterature reflect these differences. 2016 Macmillan Publishers Ltd. 1476-6930Strategic pricingUnlike fare-matching, which is reactive, strategic pricing proactively files prices that wouldoptimize an airline’s expected revenue by takinginto account customers’ scheduling preferences(for example, number of connections, durationor fare restrictions).Journal of Revenue and Pricing Management1–203
Fiig et alcommercial value, however, it does not consider competitors’ prices, schedule quality orcustomer segmentation. Further, by beingrestricted to published price points, it also lackspricing precision. These deficiencies should notbe seen as limitations of dynamic availabilitybut, rather, as a generic flaw of the existingRMS paradigm.Dynamic availabilityCompetition in RMSInternet accessibility enabled the low-cost carrier (LCC) business model because fenceless farestructures were better suited for distributionover the Internet. In the absence of rules andrestrictions, fare classes act as price pointsthat the Inventory system opens and closesdynamically, depending on demand andcustomers’ WTP. Historically, this has beencalled DP. As prices remain static, however, werefer to this approach as dynamic availability.Gallego and van Ryzin (1994) developed thetheoretical foundation for dynamic availability.They modeled demand as a homogenous(time-invariant) Poisson process. By controllingthe price, the airline controlled the intensity ofthe demand. They derived structural propertiesfor the lowest available price, inventory monotonicity (lowest available price increases withless inventory) and time monotonicity (lowestavailable price decreases with less time todeparture).Zhao and Zheng (2000) generalized theseresults by modeling the demand as a nonhomogeneous Poisson process. They showedthat inventory monotonicity continues to hold,while time monotonicity in general will not.Westermann (2006) and Isler and D’Souza(2009) discuss how airlines that use a globaldistribution system (GDS) can apply dynamicavailability. RMS online controls are enhancedby comparing origin and destination (O&D)marginal revenue against the sum of bid price(BP) for the traversed legs.Dynamic availability has real-time responsiveness (hence the term dynamic), and isconsistent with RMS. Despite its importantTo avoid the complexity of game theory (seebelow), competition-based approaches to RMStraditionally assume that the competitor is unresponsive. By incorporating competitive pricinginformation into demand forecast and optimization, the idea is to improve RMS performance.d’Huart (2010) uses PODS simulations tocalculate spill-in/spill-out by comparing a competitor’s current lowest available booking classat a given number of days before departure withthe corresponding historical distribution of thecompetitor’s lowest available booking classes.Assuming steady-state conditions and that thecompetitor does not apply user overrides, thisapproach tells us whether the competitor wasmore open (spill-out) or more closed (spill-in)than typical. PODS simulations show that thisapproach provides revenue gain.Zhang and Kallesen (2008) formulate adynamic programming model for a single legwith one competitor, for a fenceless fare structure with discrete price points. The competitor’sprice information is incorporated by assuminga predefined price curve that follows a stochasticprocess (Markov chain) generated by the competitor’s price-transition matrix. They demonstrate that their model provides positive revenuegain versus traditional approaches (for example,EMSR) that ignore competition. Althoughthese approaches were revenue-positive, theirpracticality is questionable, given the assumptions of steady-state conditions.Though modeling that indirectly accountsfor competitors’ influences can be incorporatedinto offline RMS processes, this approach hasno real-time component. Consequently, thereAUTHORCOPYVinod et al (2009) and Ratliff (2015) describehow to set strategic prices by modeling acustomer’s scheduling preferences through theuse of a discrete choice model.While strategic pricing is an improvementover fare-matching, it still suffers from lackof responsiveness in real time and ignores theavailability of the airline and its competitors.4 2016 Macmillan Publishers Ltd. 1476-6930Journal of Revenue and Pricing Management1–20
Dynamic pricing – The next revolution in RM?is no accounting for availability or potentialdifferences in schedule quality of the airlineand its competitors.Game theory offers a more accurate modelof the competitive environment and as suchprovides valuable insight. To our knowledge,however, it has never been used in real-worldRMS applications because of its complexity.Game theoryCompetitor matchingCOPYIn competitor matching, the airline monitors,possibly in real time, competitors’ prices andavailability and responds by opening or closingbooking classes based on simple market-specificbusiness rules (for example, expected load factoror days before departure).Lua (2007) tested these ideas by performingPODS simulations. Results were generallynegative for the airline performing the matching and positive for the competitor. This wasbecause competitor matching fails to accountfor the airline’s own RMS strategy. In addition,the method ignores differences in schedulequality between the airline and its competitors.Competitor matching improves the onlineRMS process through real time, competitivepricing responsiveness. However, in additionto the previously mentioned limitations, competitor matching lacks consistency and pricingprecision.AUTHORIn game theory, the objective is to optimizerevenue by considering competitors’ strategies.In principle, this would require the airline tomodel a competitor’s RMS system, calibratingthe competitor’s demand forecast as well ashaving perfect knowledge of its inventory soas to predict its strategy across the bookinghorizon. Obviously, this approach is impossible,and the standard solution is to analyze theexistence and stability of a Nash equilibrium(no player can gain by unilaterally changing strategy).Gallego et al (2006) show that a unique Nashequilibrium exists for games with a multinomiallogit (MNL) demand model under mild andrealistic assumptions associated with the utilityand cost functions.Isler and Imhof (2008) consider a single flightleg with two competitors, each with fencelessfare structures, assuming perfect knowledge ofthe competitor’s inventory. They model customer arrival as a homogeneous Poisson process.Through simplified demand assumptions, theyanalyze the Nash equilibrium using dynamicprogramming techniques and show that theequilibrium leads to complete spiral-down forlarge capacities (zero bid price).Gallego and Hu (2014) consider an O&Dmarket with multiple competitors but aremore general about the product definition.They formulate a differential game, assumingdemand is deterministic. Assuming further thatcompetitors are unresponsive, they prove theexistence of a Nash equilibrium as well asconditions for its uniqueness (the MNL demandmodel satisfies these conditions). The equilibrium state may be characterized by a relativelysimple structure (time-dependent equilibriumprices and time-independent BP representingcompetitors’ capacity pressure). This structureallows them to conclude that this equilibriummay also be applicable to responsive competitors. 2016 Macmillan Publishers Ltd. 1476-6930Agent content and pricingmanagementThe business model for an online travel agent(OLTA) can be either an agency model or amerchant model, each of which has differentimplications for revenue maximization.In the agency model, suppliers set prices andpay agent’s commissions and/or override incentives, provided that certain volume targets aremet. The agent may also charge customersa service fee to offset costs of doing business,such as marketing campaigns (for example, costof Google’s AdWords).Rao and Smith (2006) and Smith et al (2007)describe how Travelocity, under an agencybusiness model, applies revenue planning inresponse to customers’ request, thus controllingJournal of Revenue and Pricing Management1–205
Fiig et alTable 1: Suppliers business needs and existing solutions within RMSRMS onlineBusiness needsCompetitors' pricesReal-time responsivenessSchedule qualityCustomer segmentationPrice precisionRMS labilityCompetition selectionNoYesYesNoYesN/AN/AYesFare YesFare rules Fare sPricing precision is limited by the frequency of fare filing. Real-time responsiveness – to respond in realtime to the context of the session Schedule quality – to account for customerschedule preferences Customer segmentation – to price differentiateby customer segment Price precision – to price in between publishedprice points (continuous prices) RMS consistency – to account for RMSrecommendationsAUTHORCOwhich itineraries to display and in which order.The objective is to maximize revenue fromairline and market commissions, performancespecific incentives and service fees.In the merchant model, the agent accessesthe supplier’s content at a discounted rate,leaving the agent free to mark it up. This modelcan be used by airlines that seek an alternativedistribution channel for distressed inventory.Opaque products, in which the customer is notgiven specific information about the airline orschedule until after the booking, provide anexample of how this model can be used.Smith et al (2007) explain how Travelocity,under the merchant business model, dynamically optimizes the markup during the shoppingsessions. The optimization includes both a conversion model (customer buys either opaque ornon-opaque) and a share model (mix of opaqueand non-opaque), considering competitiveprices for the non-opaque product.These models share many of the facets of theDP model considered in this article. However,for a travel agent, the optimization problem issimplified because the agent does not own theinventory and, hence, can ignore the RMSaspects.To summarize our literature review, Table 1describes how different business needs are metby each solution. These needs are categorizedby:PYaRMS offline Competitors’ prices – to use competitors’ priceinformation, online/offline6 2016 Macmillan Publishers Ltd. 1476-6930DP FUNDAMENTALSDP is a new and innovative concept that, to ourknowledge, has not been implemented in theairline industry. Even the definition of DP is notfully established, as different definitions exist.This section will define what we mean by DPand compare and contrast it with existing RMSterminology. A summary is provided in Table 2.RMS objectiveThe first RMS, based on leg control, emergedin the 1980s. The objective was to maximizerevenue from each flight leg separately. Thisrequired demand forecasts as well as optimization at the leg level. In the 1990s, O&D RMSstarted to emerge. In these systems, the objective was to maximize revenue for the entirenetwork, considering all future revenue streamsfrom different O&D traffic flows. As a result,this required demand forecasting at the levelJournal of Revenue and Pricing Management1–20
Dynamic pricing – The next revolution in RM?Table 2: Comparison of O&D RMS with DPObjectiveDemand assumptionsInput data by O&DflowaO&D RMSDP Maximize revenue, across thebooking horizon O&D traffic flows are assumedindependenta Demand forecast are averages overshopping sessions Volume forecasts Sell-up/buy-down Yields Maximize contribution within thesession O&D traffic flows are assumeddependent Demand forecast are session specific,depending on the context Choice model parameters All airline offers (schedules, prices ) Bid prices from O&D RMSO&D dependency possible, but not normal practice.RMS optimizationTHORCORMS demand assumptionsIn RMS, the prevailing assumption, whichwe will take, is to consider demand for eachO&D traffic flow independent of one another.Recently, however, Vulcano et al (2012) proposed demand models that overcome thislimitation.In the RMS view, demand forecasts areaverages over multiple shopping sessions acrosscustomer segments and competitive situations.Without loss of generality, we have chosento illustrate the concepts using a fenceless farestructure, with price pi 0, in decreasing orderi 1, , n. The objective of optimization is tofind the fare pi that maximizes revenue to go.Let the choice probability (quantity) Qt ðpi Þ andtotal revenue TRt ðpi Þ ¼ pi Qt ðpi Þ be expressedas a function of time and price only, clearlyindicating that we are not considering individual customers, but rather averages over allshopping sessions.The Bellman recursion equation for theoptimal revenue to go is then given by:PYof O&D traffic flow, along with networkoptimization algorithms.AUIt is useful to review the optimization problemfor a single leg from the RMS perspective (seeTalluri and van Ryzin, 2004; Fiig et al, 2010),as we will expand on this when discussing DP.The optimization problem for a single leg withno overbooking and over a finite bookinghorizon [0;T ] can be formulated for an arbitrarydemand model using dynamic programming.We proceed by considering the revenue to go,Jt ðxÞ at time t and remaining capacity x. Theboundary conditions on Jt ðxÞ require that therevenue to go is 0 at the end of the bookinghorizon JT ðxÞ ¼ 0 and when there is noremaining capacity Jt ð0Þ ¼ 0. 2016 Macmillan Publishers Ltd. 1476-6930Jt - 1 ðxÞ ¼ Jt ðxÞ λt max½TRt ðpi Þ - Qt ðpi ÞBPt ðxÞ :piHere, λt 1 is the volume component ofthe demand forecast at the given departure dateand time step, and the bid price is defined asBPt(x) Jt(x) Jt(x 1). To maximize the objective function, we have to maximize thecontribution ct(pi) TRt(pi) Qt(pi)BPt(x) ineach time step. In the special case of static anddeterministic demand, this corresponds withthe characterization of equilibrium prices considered in Gallego and Hu (2014).Maximization of the contribution is equivalent to the standard acceptance criterion used inonline RMS: p BPt(x) FMt(p). To see this,note that maximization is obtained by settingΔct ðpÞ ΔQ ¼ 0 ) MRt ðpÞ - BPt ðxÞ ¼ 0, whereMRt ðpÞ ¼ ΔTRt ðpÞ ΔQ is the marginal revenue.Journal of Revenue and Pricing Management1–207
Fiig et alRMS input dataCOThe input data to O&D RMS is defined bythe requirements of network optimization.We need a valuation and a demand forecast atthe level of O&D traffic flows.The valuation, or yield, is the airline’s netrevenue after commission, taxes and proration.This is obtained from flown coupons (revenueaccounting) and published fares.The O&D demand forecast is obtained fromthe RMS forecasting system, which estimatesforecast parameters based on historic observations (that is, bookings, fares and availabilities)and apply these parameters to produce ademand forecast for future departure dates (foradditional details, see Fiig et al, 2014).the discrete choice models’ parameters, however, we need information not only about thechoice selected but also about the alternativesnot chosen. The fact that this informationnormally is not readily available has prohibitedthe use of discrete choice models. However,technological advancements and radical growthin online sales have made choice-related information more accessible.A wide range of choice models is available(see Garrow, 2010 for an extensive review).In practice, the models that have attracted themost attention are MNL and nested logit models.These models lead to a closed-form expressionfor the choice probability, which greatly simplifies both interpretation and computation.Applying the notation common in choicemodels, we define the choice probabilityQt(i pi, xni, β) for an individual customer n having a choice set i Cn, where pi is the price, xniis the vector of non-price attributes associatedwith individual n and alternative i and parameter estimates β (time-dependence throughthe parameters).PYFinally, substituting the fare modifierFMt(p) p MRt(p) into the equation providesthe RMS criterium. The criterium is applicableto both leg-based RMS and O&D-based RMS(for additional details, see Fiig et al, 2010).DP definitionTHORWe define DP as dynamic calculation of the optimalprice, taking into account the airline’s strategy,customer-specific information, and real-time alternative offerings.DP demand assumptionsAUIn contrast to an averaged view of demand,DP considers individual customer characteristics(for example, segmentation) and the actualchoice sets available to customers during shopping sessions. For a customer who makes traveldecisions, searches or purchases using an OLTA,he or she must prioritize among potentiallyhundreds of itineraries, with different pricesand product characteristics across multipleairlines.To model an individual customer’s decisionmaking process, discrete choice models havebeen found to be superior to traditional forecasting methodologies (Garrow, 2010). To estimate8 2016 Macmillan Publishers Ltd. 1476-6930DP objectiveWhile RMS has a holistic objective – to maximize revenue for the entire network considering all future revenue streams from differentO&D traffic flows – DP has a more modestobjective: to calculate the optimal price for agiven shopping session.In analogy with RMS, DP seeks to maximizethe contribution ct in each time step. UnlikeRMS, however, DP takes the BP as an input(from RMS) and, therefore, the volume component of the demand forecast λt becomesirrelevant.This simplification allows us to expand thechoice probability beyond the limitations ofRMS (independence and averaging), so as to consider choice models that incorporate customerspecific identification and segmentation andreal-time alternative competitive offerings.To illustrate this, let us denote the airline thatimplements DP as the DP airline. Then, forJournal of Revenue and Pricing Management1–20
Dynamic pricing – The next revolution in RM?customer n, we can calculate the contributionfor the alternative i Cn, ct(i) TRt(i) BPt(x) Qt(i pi, xni, β). Therefore, the objective for DP isto determine the prices that maximize the sum ofcontributions of the alternatives across the DPairline’s subset SAL Cn:Xct ðiÞmaxpii2SALAverageacross sessionsIt should be noted that DP and RMS’scontribution become identical if we restrictDP to the underlying assumptions of RMS(independence among alternatives, average choiceprobability and selection among published pricepoints only).Figure 2: DP example. DP view: Market share versusprice is given at the session level, for fixed competitor (OA)prices. Illustrated for AL2 price 75, 100, 125 (solid lines).RM view: The consolidated RM view of the market shareversus price (dashed line) shows lower price sensitivity.ORDP exampleCTo model session-specific customer choices,input data should include the choice modelparameters (by customer segment) and thechoice set available to the customer. Thiscomprises all of the product and price characteristics (for example, schedules, out-of-pocketprices, product quality) of the available offers.Figure 2 illustrates the market share for AL1versus p1 for each possible value of p2 (solidcurves). As the RMS is based on a consolidationof booking history across multiple sessions, ittends to see a different averaged relationship.This is illustrated by the dashed curve. Theapparent impact of price (or other non-pricefactors) tends to be diminis
Market Revenue Management Customer Pricing Retail online Optimize retailers revenue Distributor Optimize conversion RMS online Optimize airline revenue RMS offline Optimize airline revenue Fare management Optimize airline revenue Agent Content and Pricing Management † Smith et al (2007) † Rao and Smith (2006) Competitor Matching † Lua (2007)