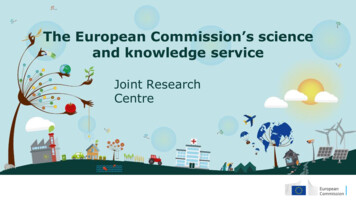
Transcription
The European Commission’s scienceand knowledge serviceJoint ResearchCentre
Economics of AI: prediction, labourmarkets, and data economicsBertin Martens, Songül TolanHUMAINT Winter School; JRC Sevilla February 8,2019
AI Prediction MachinesAjay Agrawal, Joshua Gans and Avi Goldfarb (2018) “Prediction Machines: The Simple Economics of Artificial Intelligence”, Harvard Business Review Press
Better Predictions: good for policy Prediction Policy Problems: survival probabilities for joint replacement unemployment spell length for job search strategies highest risk youth for targeting interventions Beyond prediction: varying costs of interventions and treated cases effects of interventionsJon Kleinberg, Jens Ludwig, Sendhil Mullainathan, and Ziad Obermeyer (2018) “Prediction Policy Problems”, American Economic Review, 105(5), 491-95.Susan Athey (2017) “Beyond Prediction: Using Big Data for Policy Problems”, Science, 355.6324: 483-485
Cheaper Predictions: good for business1.2.Ajay Agrawal, Joshua Gans and Avi Goldfarb (2018) “Prediction Machines: The Simple Economics of Artificial Intelligence”, Harvard Business Review Press
But what about jobs? 47% of all jobs will be gone! Or maybe only 9%? 2 Issues: Only focus on substitution Are occupations the right level at which we measuresubstitution?Frey, Carl Benedikt, and Michael A. Osborne. 2017. “The Future of Employment: How Susceptible Are Jobs to Computerisation?” Technological Forecasting and SocialChange. tz, Melanie, Terry Gregory, and Ulrich Zierahn. 2016. “The Risk of Automation for Jobs in OECD Countries: A Comparative Analysis.” OECD Social, Employment andMigration Working Papers 2 (189): 47–54. https://doi.org/10.1787/5jlz9h56dvq7-en
Economic effects beyond substitution Price effects Demand effectsComplementarityIncome effectsWage effects and labour supplyLong-run changes in businessprocessesAjay Agrawal, Joshua Gans and Avi Goldfarb (2018) “Prediction Machines: The Simple Economics of Artificial Intelligence”, Harvard Business Review PressBrynjolfsson, E., & Mitchell, T. (2017). What can machine learning do? Workforce implications. Science, 358(6370), 1530-1534.Martens, B., & Tolan, S. (2018). Will This Time Be Different? A Review of the Literature on the Impact of Artificial Intelligence on Employment, Incomes and Growth.
Substitution: from occupations to tasks andskillsProblems: Arbitrary occupation labels Different sets of tasks under the sameoccupation level Variation over time, culture, sector. Discretisation of tasks Level of granularity Specificity/generalityBrynjolfsson, E., Mitchell, T., & Rock, D. (2018). What Can Machines Learn, and What Does It Mean for Occupations and the Economy?. In AEA Papers and Proceedings (Vol.108, pp. 43-47).Fernández-Macías, E., Gómez, E., Hernández-Orallo, J., Loe, B. S., Martens, B., Martínez-Plumed, F., & Tolan, S. (2018). A multidisciplinary task-based perspective forevaluating the impact of AI autonomy and generality on the future of work. arXiv preprint arXiv:1807.02416.
What we learn from robots and othertechnologies Industrial robots jobs and wages: USA: Negative employment and wage effects EU: Negative employment, no wage effects Ger: No employment but wage effects Skill/routine-biased technological change and labour marketpolarisation and tech-driven inequality Potential policy measures Education Redistributive policies (from winners to losers, from capital tolabour) Labour market policies and unionizationAcemoglu, D., & Restrepo, P. (2018). The Race between Man and Machine: Implications of Technology for Growth, Factor Shares, and Employment. American EconomicReview, 108(6), 1488-1542.Autor, D. H., Levy, F., & Murnane, R. J. (2003). The skill content of recent technological change: An empirical exploration. The Quarterly journal of economic s, 118(4), 12791333.Martens, B., & Tolan, S. (2018). Will This Time Be Different? A Review of the Literature on the Impact of Artificial Intelligence on Employment, Incomes and Growth.
How AI could be different AI/ML as a general-purpose-technology AI could lead to the creation of new tasks that benefitlow-skill workers in the long run AI could learn high-skill tasksBrynjolfsson, E., Rock, D., & Syverson, C. (2018). Artificial intelligence and the modern productivity paradox: A clash of expectations and statistics. In The Economics ofArtificial Intelligence: An Agenda. University of Chicago Press.
Bertin MartensHumaint and Digital Economy research projectsJoint Research Centre of the European Commission
Digital technology increased the supply of dataBut human data (information) processing capacity constantResult 1: Inefficient use of available informationAI/ML reduces the relative price of machine to human decision-makingMore human decisions are outsourced to machinesResult 2: More efficient use of available informationWho gains, who loses? Depends on two trade-offs: Access to data: open versus restricted Objectives of algorithm operator: ethics versus incentives Joint Research Centre (2018) "Artificial Intelligence: A European Perspective", chapter 12.
No data ownership laws in the EU (or elsewhere)De facto control: Technical Protection Measures & Bilateral ContractsLower data access costs increase the pressure on ownership Ex. 1: digital media piracy debate copyright law Ex. 2: personal data privacy debate GDPR Ex. 3: non-personal data ? No laws?Trade-off between private costs and social benefits: Social benefits of open access: more users, more benefits Private costs of open access: loss of privacy, confidentiality, value N. Duch, B. Martens, F. Müller (2017) "The economics of ownership, access and trade in digital data"JRC Digital Economy working paper 2017-01.
Economic incentives that push towards data openness: Individual consumer incentives to open access: Ex.: fitness & health data, car insurance, consent notice Social incentives: externalities of data sharing Ex.: behavioural scores in sharing platforms, credit scores Industry incentives: Economies of scale and scope in dataaggregation improve the performance of AI/ML algorithms
Economies of scale in data feed into algorithms:Ex.: Click-through rates on rare search terms in Bing and GoogleSource: McAfee et al (2015) Measuring Scale Economies in Search, Microsoft Inc.
Data (mileage) and performance of autonomous driving algorithmsCompanyMiles per Disengagement2015Google / 75,5961,254283208444132225321Total 11,82421,5985055744,2283,663Source: California Department of Motor Vehicles, 2018
Pre-digital "model" of information distribution in human societies: Decentralised storage/learning, specialisation and exchangeDigital AI/ML model: Centralisation of information in large data pools, non-rival algorithms More unequal distribution of information in societyWho gains, who loses? Do less good to some to avoid more harm to others? Ex.: Hotel booking platform 2-sided market: hotels, touristsSantos, B and S Koulayev (2014) Optimizing Click-through in Online Rankings for Anonymous ConsumersUrsu, R (2016) The Power of Ranking: Quantifying the Effects of Rankings on Online Consumer Search and Choice
Thank you!Questions?Contact: t
Susan Athey (2017) "Beyond Prediction: Using Big Data for Policy Problems", Science, 355.6324: 483-485 Cheaper Predictions: good for business Ajay Agrawal, Joshua Gans and Avi Goldfarb (2018) "Prediction Machines: The Simple Economics of Artificial Intelligence", Harvard Business Review Press