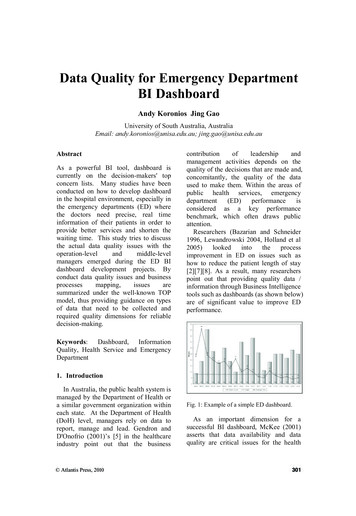
Transcription
Data Quality for Emergency DepartmentBI DashboardAndy Koronios Jing GaoUniversity of South Australia, AustraliaEmail: andy.koronios@unisa.edu.au; jing.gao@unisa.edu.auAbstractAs a powerful BI tool, dashboard iscurrently on the decision-makers' topconcern lists. Many studies have beenconducted on how to develop dashboardin the hospital environment, especially inthe emergency departments (ED) wherethe doctors need precise, real timeinformation of their patients in order toprovide better services and shorten thewaiting time. This study tries to discussthe actual data quality issues with theoperation-levelandmiddle-levelmanagers emerged during the ED BIdashboard development projects. Byconduct data quality issues and businessprocessesmapping,issuesaresummarized under the well-known TOPmodel, thus providing guidance on typesof data that need to be collected andrequired quality dimensions for dmanagement activities depends on thequality of the decisions that are made and,concomitantly, the quality of the dataused to make them. Within the areas ofpublic health services, emergencydepartment(ED)performanceisconsidered as a key performancebenchmark, which often draws publicattention.Researchers (Bazarian and Schneider1996, Lewandrowski 2004, Holland et al2005)lookedintotheprocessimprovement in ED on issues such ashow to reduce the patient length of stay[2][7][8]. As a result, many researcherspoint out that providing quality data /information through Business Intelligencetools such as dashboards (as shown below)are of significant value to improve EDperformance.Keywords: Dashboard, InformationQuality, Health Service and EmergencyDepartment1. IntroductionIn Australia, the public health system ismanaged by the Department of Health ora similar government organization withineach state. At the Department of Health(DoH) level, managers rely on data toreport, manage and lead. Gendron andD'Onofrio (2001)’s [5] in the healthcareindustry point out that the business Atlantis Press, 2010Fig. 1: Example of a simple ED dashboard.As an important dimension for asuccessful BI dashboard, McKee (2001)asserts that data availability and dataquality are critical issues for the health301
The 2010 International Conference on E-Business Intelligencesystems performance measures [10]. Itmust be noted that a number of studieshave investigated the data qualityindicators in the ED such as [6][9]. Bybringing the data quality studies into BI,this study tries to adopt a processoriented approach to understand how dataquality issues emerged through the EDdata collection processes in order toprovide guidance in ED BI projects.Fig. 2: Example of a complex ED dashboard.2. Health Service Performance Quality IndicatorsBI dashboard tool is often regarded as auseful tool to monitor organization / department performance. The performanceis measurable through key performanceindicators (KPIs). KPIs are very important to management decision-making andare relied upon by all levels of an organization to measure success in achievingoutcomes. The public health servicesneed to identify its high-value high-riskdata quality issues that support each KPI.However, in practice, this process is oftenconducted unsatisfactorily. For example,in public organizations, Theurer (1998)indicates that there are several “pitfalls”of performance measurement (and the useof KPIs) [17], closely related to the dataquality management: Data by themselves have no meaning There is often lack of a strongcommitment from leaders to move302toward measuring performance andnot just collecting data on effort Employees may not have the capacity to develop measures, so they usewhatever ‘measures’ are alreadyavailable If measurement focuses on negativeaccountability, managers and employees will seek to avoid accountability when things go wrongIn health services, the review of bothnational and international health qualityindicators suggests that the most commonKPIs dimensions for Health services are[13]: Effectiveness: the degree of achieving desirable outcomes, given thecorrect provision of evidence-basedhealthcare services to all who couldbenefit, but not to those who wouldnot benefit; safety: the degree to which healthcare processes avoid, prevent, andameliorate adverse outcomes or injuries that stem from the processesof health care itself; Responsiveness: how a systemtreats people to meet their legitimate non-health expectations (oroftendiscussedaspatientexperience); Accessibility: the ease with whichhealth services are reached. Accesscan be physical, financial or psychological, and requires that healthservices are a priori available; Equity: defines the extent to whicha system deals fairly with all concerned; and Efficiency: is the system’s optimaluse of available resources to yieldmaximum benefits or results.These dimensions of KPIs will directlyinfluence the data quality requirements inthe ED processes and are considered inthe design of this study.3. Data Quality
The 2010 International Conference on E-Business IntelligenceManagers intuitively differentiate information from data, and describe information as data that has been processed.However, data and information are oftenused synonymously in practice, particularly when addressing quality issues.Therefore, this paper uses “data” interchangeably with “information”, as well asusing “data quality” (DQ) interchangeably with “information quality” (IQ).The quality of data is nderstandability,conciseness,andusefulness [1[18][19]. It is also suggestedthat the quality of data is dependent onhowthedatawillbeused[1][4][12][14][15][16]. This fitness foruse can be defined as the intersection ofthe quality dimension being considered,the use of data (purpose) and which datafields are used in order to fulfill thepurpose [12].When selecting appropriate dataquality dimensions to investigate EDprocesses. This paper has adopted thefour key dimensions that have beenincluded in the Canadian Institution aqualityframework (CIHI 2009). In the healthdomain this is likely to include the mostimportant of all the dimensions of dataquality, that of: accuracy, timeliness,comparability, and usability of clinicaldata. These dimensions of data quality,once defined need to be used consistentlyacross units of health care and across timeif comparisons and benchmarking are tobe meaningful. The relevance dimension(the degree to which a database orregistry meets the current and potentialfuture needs of users) of the CIHI dataquality framework is not fully consideredas this study will not look into the actualdatabase design of various ED systems,however, a recommendation has beenmade on how this can be included.The above dimensions can be furtherdecomposed into a number of elements.This will enable health institutions toadopt the definition that suits their dataquality strategy most closely. Withrespects to the Canadian Institution forHealthInformationdataqualityframework and other data qualityliterature, the decomposed data qualitydimensions for this study are in Table 2.DescriptionsThe degree to which data are the correct values.The degree to which all data required by standards are captured;The degree to which the documentation underlying the datais complete.The difference between the end of the reference period andwhen the data for that period is made available.The difference between the end of the reference period andwhen the data for that period is made available in a formatreport.The degree to which standards and definitions are comparable over time.Changes are authorized and reasons are legitimate and recordedData entered as a result of correct interpretation of policesand standards, thus meeting the analysis and reporting requirementsTable 1: the decomposed data quality dimensions for this study.303
The 2010 International Conference on E-Business Intelligence4. Research DesignThis study tries to adopt a processoriented approach to understand how dataquality issues emerged through the EDdata collection process in order to providea solid foundation for BI dashboard.Based on the literature, the following research model was developed to guide thisstudy.Fig. 3: Research Model (PI: Performance Indicator).The data quality issues are categorizedunder the well-known TOP (Technology,Organization and People) framework.Mitroff and Linstone (1993) argue thatany phenomenon, subsystem or systemcan be analyzed from what they call aMultiple Perspective method – employingdifferent ways of seeing, to seekperspectives on the problem.This study consists of two stages: Stage One: Primary data collection was conducted through observation in various ED departments, interviews with ED staffand non-ED staff who have rolesof data stewards, data custodians,data users and data collectors inrelation to the ED data management. Overall, more than 30stakeholders were interviewedthrough individual or group face304 to-face meetings and teleconferencing.Stage Two: The ED data collection and submission process flowcharts were created. Data qualityissues were summarized againstthe critical tasks within theprocesses and presented by usingthe TOP model.5. FindingsThe performance of Emergency Department is as a key performance indicator inthe public health services. The waitingtime in emergency department is oftenused for benchmarking purposes. Theflow charts below provide the descriptions for major tasks of the ED visits andassociated data quality issues. However,these tasks are not designed as a linearprocess. Thus, the process model is at-
The 2010 International Conference on E-Business Intelligencetached at the end to indicate the ssuesDQ DimensionsThe current policy does not take the actual waiting timebefore triage into consideration.CompletenessConsistencyTable 2: Arrival in person – Data Quality e ambulance information system is not linked to theED systems.The current policy does not take the actual waiting timebefore triage into consideration.There is an ambulance entry timestamp (when the ambulance staff clicks the button), but this data is not usedas the starting time for ED visits.DQ Table 3: Arrive in Ambulance – Data Quality Issues.PerspectivesIssuesDQ DimensionsOrganizationalDue the lack of resources, the triage can take a longtime to complete.TimelinessPeopleThe triage is conducted based on the triage staff's expertise, which may have mistakes.AccuracyThe patient's situation may change over time (whichmay require changes in the triage category).TimelinessIntegrityTechnicalTable 4: Triage – Data Quality suesThe current ED system user-interface is not welldesigned to neither embed the data verification rulesnor highlight critical data items.The current process does not track those (patients) whohas submitted the form to be entered to the system, butdecided to leave the ED due to long waiting.The patient may never complete the form as they havebeen called for the treatment.The clerk may misread the patient’s hand-writing andnot enter the correct data into the system.DQ yCompletenessAccuracyCompletenessAccuracyTable 5: Initial registration – Data Quality Issues.305
The 2010 International Conference on E-Business Intelligence6. Data Collection Process6.1. Arrival in PersonThe majority of patients arrives the hospital in person. It must be noted that someof these patients may not necessarily seekurgent assistance, but rather require simple clinical procedures due the inability toaccess these services from the generalpracticians.The arrival time does not start from themoment that these patients enters the ED.Instead, it starts from the moment that he/ she has been registered in the system bya triage nurse.6.4. Initial RegistrationOnce the patient fills the form, they willsubmit the form to a data entry / adminclerk. The clerk will enter the patient information into the ED system.It must note that the system maycontain an incomplete record, which isdifficult to differentiate from anincomplete record (the system hasn’trecorded the patient departure time)6.5. TreatmentA critical patient may arrive ED in ambulance. They will be transferred to anambulance waiting area accompanied byambulance staff. These patients will beseen by a triage nurse in the first instance.The patient receives the treatment fromnurses, NPs and doctors. Some emergency departments have allocated fast-trackareas for simple procedures. During thetreatment, nurses, NPS and doctors willrecord the procedures in the ED system(with or without the need of individuallogins depending on the actual implementof ED systems).6.3. Triage6.6. AdmittedTriage is officially considered as the startof ED visit. A triage nurse will ask forpatient details and assign a triage category for the patient. This task usually takes2 3 minutes. The acquired data is enteredinto the system and a start time has beenrecorded. Once the triage is completed,the patient will be given a paper form tofill and asked to wait for the treatment.It must be noted that in some instances,triage can take an average of 29 minutesin one state public hospital. Due the lackof resources, the patients cannot be seenand treated by a nurse or nursepractitioner (NP) or a doctor within theallowed time for the specific triagecategory that they have been assigned.Thus, instead of keeping the patientwaiting, the triage nurse takes an effort toconduct an extensive examination of thepatient which is beyond of the scope ofhis / her duties.A proportion of patients will be admittedto the hospital. Once they have beenphysically transfer to the ward, the exittime is recorded as the end of ED visit.Their data will be transferred either manually or electronically to the PatientAdmission System (PAS) systems.6.2. Arrive in Ambulance3066.7. DischargeSome patients will be discharged fromthe hospital. A nurse, NP, doctor willrecord the departure time and dischargethe patient. In some cases, admin clerkswill check whether the patient data anddeparture time has been recorded properly.If the patient arrives in ambulance,regardless whether he / she is admitted ordischarged, the ambulance departure timeis also recorded (the ambulance staff
The 2010 International Conference on E-Business Intelligenceneeds to click a button before leaving thehospital).It is noted that there are inconsistencyin recording the departure time in thesystem as it is not the exact moment thatpatient leaves the ED. The actual timecan be when the ED staff has time toenter the data.PerspectivesTechnicalOrganizationalPeople7. Conclusion and RecommendationThese analysis of data quality issues willnot only serve as a roadmap of where tolook for data quality problems but wouldalso allow for possible rationalization andoptimization of information resources todevelop a meaningful and accurate BIdashboard in the ED environment.IssuesWhen the ED and Patient Admission System (PAS)systems are not developed by one vendor, the data integration issue occurs (often requires a manualprocess).Different area of services / hospital may use differentED and PAS systems.The patient may not provide all required data. For example, the insurance details were not carried.DQ ble 6: Treatment – Data Quality Issues.PerspectivesTechnicalIssuesOne implementation of ED systems does not have individual accounts for audit trails when a change hasbeen made. Changes can be made at any time.DQ DimensionsIntegrityDuplicationOrganizationalThe use of SNOMED CT system for clinical coding isover-complicated in the ED environment.It is not the first priority of individuals who updatesthe system record especially the staff are busy in treating the grityThere is a possibility for individuals to change the datato meet the benchmark - e.g. assign a lower triage categoryAccuracyIntegrityPeopleTable 7: Admitted– Data Quality suesDQ DimensionsThe departure time in the is not the exact moment thatpatient leaves the ED. The actual exit time can be thetime when the ED staff has time to enter the data.AccuracyTimelinessTable 8: Discharge – Data Quality Issues.307
The 2010 International Conference on E-Business IntelligenceAs much as possible, peoples’ attitudestowards the care processes andparticularly the IT systems which supportthese processes should also be recordedand analyzed as they would provide cluesabout possible ‘workarounds’ and alsothe motivations that may or may not drivethem to have commitment to high levelsof data quality. If for example staffexpress dissatisfaction with IT systems ascumbersome and unresponsive to theirneeds, it is likely to point to them nottaking the requisite care in data entry forexample or point to a practice of themmaintaining their own locally basedsystems. Although such practices may betolerated, proper governance of such dataresources would be critical.Organizational issues such as localculture for example must also bethoroughlyexaminedtoidentifyvulnerabilities such as duplication of data,contradictory classifications of data ordiffering interpretations of mandated oradvisory departmental standards.This study also indicates thatunderstanding and mapping the clinicalprocesses and associated ‘data andinformation path’ would be critical inestablishing the points for assessing thedata quality along the various businessprocesses. With this understanding, theBI developers together with the businessprocess owners (e.g. doctors) will be ableto define performance standards for keyoperational processes, and the BI systemwill monitor events, analyze patterns, andhelp users determine what actions to takein a reliable way.References[1] Ballou, D.P. & Pazer, H.L. (1995)Designing Information Systems toOptimize the Accuracy-timelinessTradeoff. Information Systems Research. 6(1), 51-72308[2] Bazarian JJ, Schneider SM et al. Doadmitted patients held in the emergency department impair throughputof treat and release patients? AcadEmerg Med. 1996; 3(12): 1113-1118.[3] Canadian Institute for Health Information (CIHI), (2005), The CIHI Data Quality Framework 2009, URL:http://www.cihi.ca/cihiweb/en/downloads/data quality framework 2009e.pdf, online accessed [1/6/2010][4] English, L.P., (1999) Improving DataWarehouse and Business InformationQuality: Methods for reducing costsand increasing Profits, Willey & Sons[5] Gendron S. M. and D'Onofrio, J. M.(2001), Data Quality in the Healthcare Industry, Data Quality, 7:1[6] Graff L, Stevens C, et al. Measuringand Improving Quality in EmergencyMedicine. Acad Emerg Med. 2002; 9(11): 1091-1107.[7] Holland L, Smith L et al., (2005) Reducing Laboratory Turnaround TimeOutliers Can Reduce Emergency Department Patient Length of Stay. AmJ Clin Pathol. 2005; 125(5) 672-674.[8] Lewandrowski K. (2004), How theclinical laboratory and the emergencydepartment can work together tomove patients through quickly. Clinical Leadership Management Review,2004; 18(3): 155-9.[9] Lindsay P, Schull M, et al. (2002),The Development of Indicators toMeasure the Quality of Clinical Carein EDs Following the ModifiedDelphi Approach. Academic Emergency Medicine, 2002; 9(11): 11311139.[10]McKee, M. (2001): Measuringthe efficiency of health systems. British Medical Journal, 323(7308): 295296.[11]Mitroff, I. I. and H. A. Linstone,(1993), The Unbounded Mind: Breaking the Chains of Traditional Busi-
The 2010 International Conference on E-Business Intelligenceness Thinking, New York, OxFordUniversity Press, 1993.[12]Neely, M.P. (2001) A ProposedFramework for the Analysis ofSource Data in a Data Warehouse,Proceedings of the Sixth InternationalConference on Information Quality,November 2001, Cambridge, MA,USA, MIT[13]OECD, (2006), "Health CareQuality Indicators Project ConceptualFramework Paper", Editors: EdwardKelley and Jeremy Hurst, OECDHealth Working Paper, DELSA/HEA/WD/HWP(2006)3[14]Orr, K. (1998) Data Quality andSystem Theory. Communications ofthe ACM. 41(2), 66-71[15]Salaun, Y., and Flores, K.,(2001), “Information Quality: Meeting the Needs of the Consumer”, In-ternational Journal of InformationManagement, 21(1), 2001, pp. 21-37.[16]Strong, D.M. (1997) IT Processdesigns for Improving InformationQuality and reducing Exception Handling: A Simulation Experiment. Information and Management. 31, 251263[17]Theurer, J., (1998), Seven pitfalls to avoid when establishing performance measures. Public Management, 1998. 80(7): p. 21-24.[18]Wand, Y. & Wang, R.Y. (1996)Anchoring Data Quality Dimensionsin Ontological Foundations. Communications of the ACM. 39(11), 86-95[19]Wang, R.Y., and Strong, D.M.,(1996) “Beyond Accuracy: What Data Quality Means to Data Consumers”,Journal of Management InformationSystems, 12(4), 1996, pp. 5-33.309
Keywords: Dashboard, Information Quality, Health Service and Emergency Department 1. Introduction In Australia, the public health system is managed by the Department of Health or a similar government organization within each state. At the Department of Health (DoH) level, managers rely on data to report, manage and lead. Gendron and