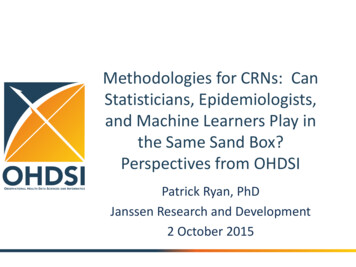
Transcription
Methodologies for CRNs: CanStatisticians, Epidemiologists,and Machine Learners Play inthe Same Sand Box?Perspectives from OHDSIPatrick Ryan, PhDJanssen Research and Development2 October 2015
Introducing OHDSI The Observational Health Data Sciences andInformatics (OHDSI) program is a multistakeholder, interdisciplinary collaborative tocreate open-source solutions that bring outthe value of observational health data throughlarge-scale analytics OHDSI has established an internationalnetwork of researchers and observationalhealth databases with a central coordinatingcenter housed at Columbia Universityhttp://ohdsi.org
OHDSI: a global communityOHDSI Collaborators: 100 researchers in academia, industry and government Statisticians, Epidemiologists, Informaticists, MachineLearners, Clinical Scientists all playing in the same sandbox 10 countriesOHDSI Distributed Data Network: 40 databases standardized to OMOP common data model 500 million patients
OHDSI ongoing collaborative gical researchObservationaldata management Data quality assessmentCommon Data Model evaluationATHENA for standardizedvocabularies WhiteRabbit for CDM ETLUsagi for vocabulary mappingHERMES for vocabulary explorationACHILLES for database profiling Phenotype evaluation CIRCE for cohort definitionCALYPSO for feasibility assessmentHERACLES for cohortcharacterization Chronic disease therapy pathways Empirical calibrationLAERTES for evidence synthesis CohortTemporalPatternDiscovery HOMER for causality assessment Evaluation framework andbenchmarking PatientLevelPredictionAPHRODITE for predictivephenotyping PENELOPE for patient-centeredproduct timationPatient-levelpredictionClinical applicationshttp://ohdsi.org
Evolution of the OMOP Common data modelOMOP CDMv2OMOP CDM now Version 5, followingmultiple iterations of implementation,testing, modifications, and expansionbased on the experiences of the OMOPcommunity who bring on a growinglandscape of research use cases.OMOP CDMv4OMOP CDMv5http://omop.org/CDMPage 5
Drug safety surveillanceDevice safety surveillanceVaccine safety surveillanceComparative effectivenessHealth economicsuse casesOne model, multipleClinical researchQuality of carePersonObservation periodStandardized health system dataLocationCare siteStandardized meta-dataCDM sourceSpecimenProviderDeathVisit occurrenceProcedure occurrenceProcedure costDrug exposureDrug costDevice exposureDomainConcept classConcept relationshipRelationshipConcept synonymDevice costConcept ancestorCohortMeasurementCohort attributeNoteCondition eraObservationDrug eraFact relationshipDose eraStandardizedderived elementsCondition occurrenceSource to concept mapDrug strengthCohort definitionAttribute definitionStandardized vocabulariesVisit costVocabularyStandardized healtheconomicsStandardized clinical dataPayer plan periodConcept
Patients with total knee replacementAdministrative claims: TruvenMarketScan CCAEElectronic health records: UKCPRDHospital billing records:Premier
Evidence OHDSI seeks to generate fromobservational data Clinical characterization:– Natural history: Who are the patients who have exposure to cranialperforaters?– Quality improvement: what proportion of patients who have exposureto cranial perforaters experience seizure? Population-level estimation– Safety surveillance: Do cranial perforaters cause seizure?– Comparative effectiveness: Do cranial perforaters cause seizure morethan non-perforated craniectomy? Patient-level prediction– Precision medicine: Given everything you know about me and mymedical history, if I have exposure to a cranial perforator, what is thechance that I am going to have seizure in the next year?– Disease interception: Given everything you know about me, what isthe chance I will develop the need for a cranial perforater?
‘Cranial perforator’ in the OHDSI vocabularyas a device!.but none of our source datamap to ithttp://ohdsi.org/web/atlas
Causal inference problem:?ExposureCovariates?OutcomeProblem: The data I have doesn’t allowme to answer the question I wantStop and donothingWait to getnew dataChange yourquestion
Opportunities for changing the question Clinical characterization:– Natural history: Who are the patients who have exposure to cranialperforaters a craniectomy with burr hole procedure?– Quality improvement: what proportion of patients who have exposure tocranial perforaters a craniectomy with burr hole procedure experienceseizure?Population-level estimation– Safety surveillance: Does cranial perforaters a craniectomy with burr holeprocedure cause seizure?– Comparative effectiveness: Do cranial perforaters a craniectomy with burrhole procedure cause seizure more than non-perforated craniectomy?Patient-level prediction– Precision medicine: Given everything you know about me and my medicalhistory, if I have exposure to a cranial perforaters a craniectomy with burr holeprocedure what is the chance that I am going to have seizure in the next year?– Disease interception: Given everything you know about me, what is thechance I will develop the need for a cranial perforaters a craniectomy withburr hole procedure?
Feasibility assessment: cohortdefinitionhttp://ohdsi.org/web/calypso
Cohort characterization
Cohort exploration
What can we learn:Scenario 1 : mature technology Clinical characterization of indicated population andusers of mature technology Population-level estimation of new users of maturetechnology vs. alternative treatments or non-exposure Patient-level prediction to summary past experience of‘patients like me’
What can we learn:Scenario 2: New technology Clinical characterization of indicated population andusers of current treatments PLUS new technology Population-level estimation of new users of newtechnology vs. alternative treatments or non-exposure Patient-level prediction to summary past experience of‘patients like me’
Concluding thoughts We need to do more to understand what we can learnfrom the data we’ve already got (changing the questionas necessary) and get more data to address what weknow we’re missing Product lifecycle continuum (new - mature product)can fit with same evidence generation continuum:clinical characterization population-level estimation patient-level prediction .same analytics but different use cases The entire evidence lifecycle (methods research open-source analytics development clinicalapplication) needs to be cultivated to support robustproduct surveillance and evaluation
Join the journeyInterested inOHDSI?Questions orcomments?Contact:ryan@ohdsi.org19
The Observational Health Data Sciences and Informatics (OHDSI) program is a multi-stakeholder, interdisciplinary collaborative to create open-source solutions that bring out the value of observational health data through large-scale analytics OHDSI has established an international network of researchers and observational