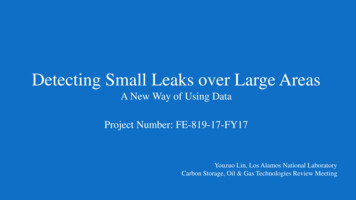
Transcription
Detecting Small Leaks over Large AreasA New Way of Using DataProject Number: FE-819-17-FY17Youzuo Lin, Los Alamos National LaboratoryCarbon Storage, Oil & Gas Technologies Review Meeting
AGENDA1. Technical Status2. Accomplishments to Date3. Lessons Learned4. Synergy Opportunities5. Summary & Path Forward6. Appendix
Technical Status
PROBLEM DESCRIPTIONWHYAn early assessment of CO2 storage requires a technique capable of detectionof small leaks in a large area without the need for extensive and expensive field datasetsCHALLENGES Small and useful events buried in noisy and large-scale datasetsEfficient method is required to allow early detectionFinancially effective methodSOLUTION Data-Driven Methods Multi-Physics Surface/Subsurface Measurements
Data-DrivenPAST & NEW risticsBasedSimulationNew DirectionsNew Directions Relevant information Computationally Efficient Financially CheapPrevious ProjectsPrevious Projects Detect Leak of CO2 Using Seismic Waveform Tomography Imaging Geologic Features using Seismic DataPhysics Based
Data-DrivenMulti-Physics-Guided Data-Driven MethodsMachine LearningHybrid MethodImprove Efficiency and AccuracyMulti-Physics Data SetsoData from Subsurface:o Seismico Gravityo Flowo PressureoData from Surface:o Unmanned Aerial Vehicle (UAV)2D ImageryCompensate Missing InfoEstimate UncertaintyHeuristicsBasedSimulationPrevious ProjectsPhysics Based
Advanced Seismic Imaging and Inversion TechniquesExample of Previous Effort
Advanced Seismic Imaging and Inversiono Novel full-waveform inversionand imaging methods foractive seismic data to obtainsubsurface fracture/fault zoneso Novel focal mechanism inversionmethods to reveal CO2-injectioninduced microseismic events inpre-existing fracture zones.
Physics-Guided CO2 Leakage DetectionExample of New Directions
Injection WellCHALLENGES Expensive costs in acquiring data Limited information out of data Time consuming to interpret the dataObs 1Obs 22Above ZoneCaprockPotentialLeak 1Potential ReservoirLeak 2SOLUTIONDetect Leakage Signatures from Pressure Data Pressure data from limited number of sensors Data-driven hybrid approach (Machine Learning FEHM) Dimensionality reductionLANL Team: Youzuo Lin, Dylan Harp, Bailian Chen, Rajesh Pawar, and George Guthrie.
Accurate Estimation of Unknown Leakso We employ the above training data to train oursupervised learning methods.o 500 unknown leak cases are created by varyingthe CO2 injection rate.o Prediction error is measured by Mean AbsoluteError (MAE): 𝐧𝐧𝐢𝐢 𝟏𝟏 𝐲𝐲𝐢𝐢𝐠𝐠𝐠𝐠 𝐲𝐲𝐢𝐢𝐩𝐩𝐩𝐩𝐩𝐩𝐩𝐩 𝐌𝐌𝐌𝐌𝐌𝐌 𝐧𝐧o Overall MAE 3 grido Detection error: within 1 grid point: 41.4% within 2 grid points: 60.6% within 3 grid points: 72.8%
Other Research Efforts and Data Types
Surface Feature Detection Permeability Estimation fromReal-Time GeologicFault/Fracture Detection from from UAV Hyperspectral Hydraulic Head DataImagerySeismic DataLearning-Based Inverse ModelingLANL Team: Youzuo Lin, Ellen Syracuse, Emily Schultz-Fellenz, David Coblentz, and George Guthrie.External Collaborators: Shusen Wang (UC, Berkeley), Jayaraman J. Thiagarajan (Center for Applied Scientific Computing, LLNL).
Accomplishments to Date
Hybrid Methodto EstimateUncertaintyHybrid Methodto Detect SmallEventsLeakage Detection andRisk AssessmentMicroseismic EventDetection UsingDeep Learning MethodReal-Time GeologicSurface/Subsurface FeatureDetectionLANL TeamYouzuo LinPaul JohnsonDavid CoblentzExternal CollaboratorsYue Wu (U. of Rochester)Ji Liu (U. of Rochester)Ming Yan (Michigan State)LANL TeamYouzuo LinEllen SyracuseEmily Schultz-FellenzDavid CoblentzGeorge GuthrieExternal CollaboratorsShusen Wang (UC, Berkeley)Jayaraman Thiagarajan (LLNL)LANL TeamYouzuo LinDylan HarpRajesh PawarGeorge GuthrieHybrid Methodto ImproveEfficiency& AccuracyHybrid Methodto CompensateMissing InfoLearning-BasedHydraulicInverse ModelingLANL TeamYouzuo LinDaniel O'MalleyBrendt WohlbergVelimir V. Vesselinov
Lessons Learned
Lesson LearnedLessons LearnedPhysics-BasedRelevant InformationMachine LearningXXComputationallyEfficientXXFinacially CheapXXRisks and ChallengesoooooHybrid MethodUseful training data sets to characterize the physicsSeamless fusion of multi-physics data setsSmall events buried among the noisy environmentComplexity of real world VS synthetic model: heterogeneity, scale,Early detection and warning
Synergy Opportunities
PROJECT TEAMLANL TeamYouzuo Lin, Dylan Harp, Ting Chen,Lianjie Huang, Paul Johnson, David Coblentz,Rajesh Pawar, and George GuthrieExternal CollaboratorsUniversity of RochesterDept. of Computer SciencesYue Wu, Zhen Zhou,and Dr. Ji LiuUniversity of California, BerkeleyDept. of StatisticsDr. Shusen WangMichigan State UniversityDept. of Computational SciencesDr. Ming YanPenn State UniversityDept. of GeosciencesDavid Chas BoltonLLNL Center for Applied ScientificComputingJayaraman J. ThiagarajanRESOURCESInternal LANL Program FundingLANL Center for Space and EarthScience (CSES) ProjectsPathfinderEnvironmental ProgramUNESE
Summary & Path Forward
SUMMARYSUMMARYo We have developed several seismic inversion and imaging techniqueso The method has been applied to Aneth CO2-EOR field datao Preliminary results to demonstrate the performance and feasibility of our hybrid machine learning methodsPATH FORWARDNear Futureo Add more physics to our current model and flow data: 2D- 3D, heterogeneityAlgorithms Development Based on Synthetic Data Testo Develop machine learning algorithms based on synthetic models and data setsPerformance Evaluation Based on Field Data Testo Acquire field data setso Acquire other types of data sets
Thank you!
Appendix
Benefit to the Programo Our techniques can detect small signals out of large noisy data.o Our techniques can extract useful information from different types of data sets.o All these techniques will be critical to early detection of CO2 leakage.
Project OverviewThe task is exploring related strategies for applying this approach to detection of a subsurfaceleak out of large area. It will leverage a combination of multiple types of field data andsubsurface emulators (which can rapidly reproduce detailed physics-based predictions).
Organization ChartU. C. BerkeleyUniversityCollaboratorsU. RochesterMichigan StatePenn StateLANLNational LabCollaboratorLLNL
Gantt ChatKick-offFY 17Milestone 12017OctAprAugOctFY 18Milestone 2AprAugOctMilestone 3AprAugOctSchedulingML AlgorithmDevelopment Defining tasksand locateresources Employ algorithms topressure data andothersDevelop ML methods formultiple le data setDevelop algorithmsObtain training datafrom synthetic model Use ML and pressure data to detectpotential leaks Develop multiphysics MLmethodsWill look intoseismic, UAVand otherdatasetsEndFY 19
Bibliography[1]. Youzuo Lin, Ellen B. Le, Daniel O'Malley, Velimir V. Vesselinov, and Tan Bui-Thanh, “Large-Scale Inverse Model Analyese EmployingFast Randomized Data Reduction”, Water Resources Research, 2017 (accepted).[2]. Youzuo Lin, and Lianjie Huang, “Building Subsurface Velocity Models with Sharp Interfaces Using Interface-Guided Seismic FullWaveform Inversion”, Pure and Applied Geophysics, 2017 (accepted).[3]. Youzuo Lin and Lianjie Huang, “Acoustic- and Elastic-Waveform Inversion Using a Modified Total-Variation RegularizationScheme,”Geophysical Journal International, 200 (1): 489-502, 2015[4]. Youzuo Lin and Lianjie Huang, “Quantifying Subsurface Geophysical Properties Changes Using Double-difference SeismicWaveform Inversion with a Modified Total-Variation Regularization Scheme,” Geophysical Journal International, 203 (3): 2125-2149,doi:10.1093/gji/ggv429, 2015.[5]. Youzuo Lin and Lianjie Huang, “Least-squares reverse-time migration with modified total-variation regularization,” SEG TechnicalProgram Expanded Abstracts 2015: 4264-4269.
The task is exploring related strategies for applying this approach to detection of a subsurface leak out of large area. It will leverage a combination of multiple types of field data and subsurface emulators (which can rapidly reproducedetailed physics-based predictions). Organization Chart. LANL. University