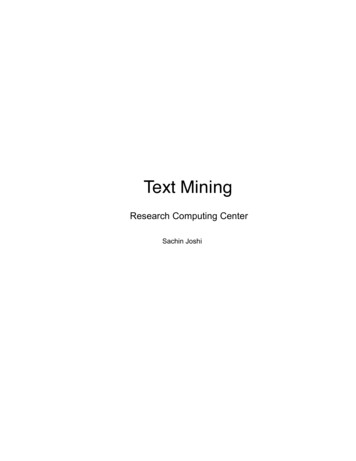
Transcription
Text MiningResearch Computing CenterSachin Joshi
8/27/16Logistics Course outcomes: Python programming proficiency (to the extend of stand-alone ones);Linguistics basics;Mining algorithm in real world applications;The ability to tackle more challenging NLP and text mining problems;Awareness of the values locked up in text data;Both computation- and data-driven thinking (good for big data andanalytics jobs). Some running and cool projects to brag about!CSE 398/4987What is text miningText data TweetsReviewsGovernment reportsScientific papersNewsBooksMessagesData Mining (Data Science)Non-text data CSE 398/498ImagesVideosTemperaturesTime seriesLocationGraph/networks84
What is text miningReal worldHuman beingsText dataMining as reverse engineering Infer what the real world is; Infer what the human beings are thinking; Infer the language itself.Decision making!What is text mining A more practical viewReal worldComputingModelingLinguistics
What is text mining ExampleText dataKnowledgeAttractive Nakiri.(double bevel) I have been using ShunClassic Santokus and utility knives for almost everythingso I felt a need to try something "new." This was it. Thisblade is quite thin and based on the specs it is made of agood quality steel. It isn't at the very top in terms ofhardness, but I hope that also means that it will be lessbrittle and potentially easier to sharpen.AttractiveBlade - ThinSteel - Good qualityBlade - less brittleBlade - Easy to sharpenDecision Prob(buying) 95%Text mining vs. other approaches Data Science: focus on data processing, with simple miningmodels. Data Mining: focus on general mining techniques for moregeneral data formats. AI: a general area, providing some techniques for text mining. Database: focuses on structured data, while text data are highlyunstructured. traditional NLP: some techniques can be used for text mining,but it focuses more on text analysis (beat a sentence to death).
Why text mining Texts are every where! Texts have valuable but hidden knowledge. Many useful and real-world applications Stock market (deep)Customer survey (Amazon.com)Policy and government (opengov.com)Question and answering systems (Baidu’s medical QA)Many more Stock market prediction Predict whether a stock will go up or down using opinionexpressed in public forum and news.Entities: companies,countries, people, etc.Opinions: positive,negative, neutral, etc.
Customer relation managementScientific literal management Categorization of publications; Information retrieval; Discovering scientific hypothesis; Influential paper discovery; Trending topics for research Know what the customers likeand don’t like about a product. Possibly recommend alternativeproducts. Sway customers opinions viaincentive. Retain leaving customers.
Question answeringHow to do text mining Tools:Computers: store and process big text data. (programmingand data structures)Linguistics: human knowledge about syntax, semantics, etc.(of English)Statistical and machine learning models: infer the hiddenknowledge about the real world in the text data. (Calculus,linear algebra, probability and statistics.)
Text MiningIntroductionOutline Sentence segmentation. Word tokenization. Word normalization:§ case folding§ lemmatization§ stemming. Text representations.
A big picture Decide the tokens/vocabulary of your corpus. Further tasks:§ word collocation (phrase level)§ classification, clustering, topic models (document level)§ syntax parsing (sentence level)§ semantic analysis: entity resolution, relation detection (all levels)§ sentiment analysis (all levels).Text processing pipeline Get the tokens!Vector space modelIndexing and IRTopic modeling& normalizationClusteringClassificationSequential model
Sentence segmentation Why: we want to study properties of sentences sometimes. What are the boundaries between sentences? Punctuations:§ Question mark: “?”§ Exclamation (!)§ Semi-colon (;)§ Period (.): 500.00 dollars, Ph.D,§ Comma (,): 10,500 dollars§ Quotation (“): “Bye”, I said§ Ampersand (&): AT&T, Barnes & Nobel More advanced methods are based on machine learning: checkthe surrounding of a punctuation to decide whether it is aboundary or not. NLTK uses Punkt sentence segmenter.Word tokenization A sequence of characters - sequence of meaningful tokens. Example:From IIR:From FSNLP:Notice theirdifference?
Tokenization How to define a valid token is task-dependent. A simple space separator is not enough: “San” and “Francisco”? “Mar 2015”?Do we care about phrases? “San Francisco”? “New York”?Special characters like “ 10”? Or hashtag on Twitter “#LU”Apostrophes (’): “doesn’t” or “does” and “n’t”? In sentiment analysis, negative isinformative. “rock ‘n’ roll”, “Tom’s place”§ Commas: “100, 000 dollars” or (“100” “000” “dollars”)§ Hyphens: “soon-to-be” or (“soon” “to” “be”), “Hewlett-Packard”§ Email addresses, dates, URLs (Usually treated separately).§ In practice, no tokenization is perfect.§ Instead, it is usually via fast programmed automata via regularexpressions.Stop word removal Stop word list To remove or not to remove, that’s question: Some stop words have no meaning: “the”, “a”, “for” Stop word removal can reduce data size. But stop word are critical elements in syntax and semantics. NLPusually keeps the stop words to facilitate the analysis of a wholesentence.
Word normalization After tokenization, we may have two words that can belong tothe same class. Turn multiple words into a single class (may be incorrect). Examples: (“is” and “was” may be considered equivalent). USA and U.S.A have the same meaning There are various kinds of word normalization:§ case folding.§ lemmatization.§ stemming.§ semantic links (“auto” and “car”).Case folding Usually we want lower-case the capital letters at the beginningof a sentence. Example: “He went to church” - “he”, “went”, “to”, “church” Counter-examples: “Kennedy was shot”? “USA” - “usa”? Case can be informative. “US” - “us”: country name vs. a pronoun, big loss of information. “C.A.T” - “cat”: company name vs. an animal Rule-based: Only lower case the first letter of a sentence and allwords in titles, leaving other things un-touched. Machine learning: sequence model with rich features.
Lemmatization Lemma - lemmatization A lemma is a major entry or base form in an English dictionary. Examples:§ “is”, “are”, “were” share the lemma “be”.§ “dinner” and “dinners” share the lemma “dinner”“He is reading detective stories.”Some information is lost.“He be read detective story.”Lemmatization More formally, we want to break a word into a few parts torecover the most basic component in the word (morphology). A word consists of morphemes /mofims/§ stem morphemes: the basic meaning of the word;§ affix morphemes: added meanings§ Example: “dog” - “dog”, “cats” - “cat” “s”§ “organization” - “organize” - “organ Morphological Parsing is the technical term for this wordbreaking process
Stemming (Porter Stemmer) A simple but crude rule-based lemmatization method. A word is passed through the stemmer multiple times, with theoutput of the last pass as the input to the current pass.a small test Can have a lot of errors: “organization” - “organize” - “organ” “noisy” - “noise”Example of different stemmers
Stemming vs. lemmatization Stemming is crude and rule-based. Lemmatization involves a dictionary and morphological analysisof words. (requiring more linguistic knowledge). Example: Stemming: “saw” - “s” Lemmatization: “saw” - “see” (verb) or “saw” (noun) When is stemming useful and harmful? Examples?Text representation Vector space (or bag-of-words) models:§ Word order does not matter (or lost).§ Boolean.§ Term-frequency.§ Term-frequency inverse-document-frequencyAll linear algebraconcepts andoperations hold in thisvector space. Sequences of tokens (after text pre-processing). Word order matters and shall be modeled.Requires a set of math toolsbeyond linear algebra.
A corpus as a Boolean matrixDocumentsTokensAntony and CleopatraJulius CaesarThe 1111worser101110Each document is represented by a binary vector {0,1} V Issues with such text representation?Think about finding relevant docs using keyword “Antony”Term frequency matrix Consider the number of occurrences of a term in a document: Each document is a count vector in ℕv: a column belowAntony and CleopatraAntonyJulius CaesarThe rcy203551worser201110Relevance information is now better preserved: try to find docs containing “Antony”Usually we take the log the frequencies to avoid scaling problem.
Bag of words model Vector representation doesn’t consider the ordering of words ina document John is quicker than Mary and Mary is quicker than John havethe same vectors This is called the bag of words model. In a sense, this is a step back: The positional index was able todistinguish these two documents.Document frequency Rare terms are more informative than frequent terms Recall stop words Consider a term (e.g., “Calpurnia”) in the query that is rare inthe collection.Antony and CleopatraAntonyJulius CaesarThe rcy203551worser201110Think about finding docs relevant to the query “Caesar” & “Calpurnia”.
Document frequency Frequent terms are less informative than rare terms Consider a query term that is frequent in the collection (e.g., high,increase, line) A document containing such a term is more likely to be relevant thana document that doesn’t But it’s not a sure indicator of relevance. For frequent terms, we want high positive weights for words likehigh, increase, and line Given equal term frequencies, want lower weights for rare terms. We will use document frequency (df) to capture this.idf weight dft is the document frequency of t: the number of documentsthat contain t dft is an inverse measure of the informativeness of t dft N We define the idf (inverse document frequency) of t byidft log10 ( N/dft ) We use log (N/dft) instead of N/dft to “dampen” the effect of idf. (Thinkabout N 1M, df 100 and 10.
idf example, suppose N 1 million There is one idf value for each term t in a 00fly10,000under100,000the1,000,000idft log10 ( N/dft )tf-idf weighting The tf-idf weight of a term is the product of its tf weight and itsidf weight.w t ,d log(1 tft ,d ) log10 ( N / df t ) Best known weighting scheme in information retrieval Note: the “-” in tf-idf is a hyphen, not a minus sign! Alternative names: tf.idf, tf x idf Increases with the number of occurrences within a document Increases with the rarity of the term in the collection
Binary count weight matrixAntony and CleopatraJulius CaesarThe worser1.3700.114.150.251.95Each document is now represented by a real-valuedvector of tf-idf weights R V Documents as vectors So we have a V -dimensional vector space Terms are axes of the space Documents are points or vectors in this space Very high-dimensional: tens of millions of dimensions when youapply this to a web search engine These are very sparse vectors - most entries are zero.
Documents in vector space with two termsFinanceGreatAll concepts and operations in linear algebra apply Distance of two vectors. Angle between two vectors (cos similarity).
Distance vs. similarityNotation: di and q are all documents.Summary Low level text processing. Bag-of-words or vector space text representations. Distance/similarity measures. Coming up: Classification based on the vector space of terms.
Text MiningText ClassificationText classification Why text classification§ Spam detection;§ Finding relevant documents;§ Sentiment analysis
Formulation (Supervised learning)§ Given:§ A document d (usually in a vector space).§ A fixed set of classes:C {c1, c2, , cJ}§ A training set D of documents each with a label in C§ Determine:§ A learning method or algorithm which will enable us to learn a classifier f§ For a test document d, we assign it the class f(d) CClassifiers§ Supervised learning§ Naive Bayes (simple, common).§ k-Nearest Neighbors (simple, powerful)§ Support-vector machines (new, generally more powerful)§ plus many other methods§ No free lunch: requires hand-classified training data§ But data can be built up (and refined) by amateurs§ Many commercial systems use a mixture of methods
Classification using bag-of-wordsf(I love this movie! It's sweet,but with satirical humor. Thedialogue is great and theadventure scenes are fun Itmanages to be whimsical andromantic while laughing at theconventions of the fairy talegenre. I would recommend it tojust about anyone. I've seen itseveral times, and I'm alwayshappy to see it again wheneverI have a friend who hasn't seenit yet.) cClassification using bag-of-wordsf(greatlove22recommend 1laughhappy.11.) c
Features§ Features axes in the vector space.§ Supervised learning classifiers can use any sort of feature§ URL, email address, punctuation, capitalization, dictionaries, networkfeatures§ In the bag of words view of documents§ We use only word features§ we use all of the words (vocabulary) in the text (not a subset)Feature Selection: Why?§ Text collections have a large number of features§ 10,000 – 1,000,000 unique words and more
Feature Selection: Why?§ Selection may make a particular classifier feasible§ Some classifiers can’t deal with 1,000,000 features§ Reduces training time§ Training time for some methods is quadratic or worse in the number of features§ Makes runtime models smaller and faster§ Can improve generalization (performance)§ Eliminates noise features§ Avoids overfittingEvaluating§ Evaluation must be done on test data that are independent ofthe training data§ Sometimes use cross-validation (averaging results over multipletraining and test splits of the overall data)§ Easy to get good performance on a test set that was availableto the learner during training (e.g., just memorize the test set)§ Measures: precision, recall, F1, classification accuracy§ Classification accuracy: r/n where n is the total number of test docs and ris the number of test docs correctly classified
A running example§ Classify webpages from CS departments into:§ student, faculty, course, project§ Train on 5,000 hand-labeled web pages§ Cornell, Washington, U.Texas, Wisconsin§ Crawl and classify a new site (CMU) using Naïve Bayes§ Results
Classification Using Vector Spaces§ In vector space classification, training set corresponds to alabeled set of points (equivalently, vectors)§ Premise 1: Documents in the same class form a contiguousregion of space§ Premise 2: Documents from different classes don’t overlap(much)§ Learning a classifier: build surfaces to delineate classes in thespaceSec.14.1Documents in a Vector SpaceGovernmentScienceArts
Sec.14.1Test Document of what class?GovernmentScienceArtsSec.14.1Test Document GovernmentIs thissimilarityhypothesistrue ingeneral?GovernmentScienceArtsOur focus: how to find good separators
Rocchio Classifier Training: Use standard tf-idf weighted vectors to represent text documents For training documents in each category, compute a prototype vector bysumming the vectors of the training documents in the category. Prototype centroid of members of class Where Dc is the set of all documents that belong to class c and v(d) is the vectorspace representation of d. Testing: assign test documents to the category with the closest prototypevector based on cosine similarity.Illustration of Rocchio Text Categorization
9/20/16Rocchio Properties Forms a simple generalization of the examples in each class (aprototype).may be problematic Prototype vector does not need to be averaged or otherwisenormalized for length since cosine similarity is insensitive tovector length. Classification is based on similarity to class prototypes. Does not guarantee classifications are consistent with the giventraining data.Why not?CSE 398/49819Rocchio Anomaly Prototype models have problems with polymorphic (disjunctive)categories.CSE 398/4982010
k Nearest Neighbor Classification kNN k Nearest Neighbor It is a supervised learning method. Training: storing the representations of the training examples in D. Testing: classify a document d into class c:§ Define k-neighborhood N as k nearest neighbors of d§ Count number of documents i in N that belong to c§ Estimate P(c d) as i/k§ Choose as class argmaxc P(c d) [ majority class]Example: k 6 (6NN)P(science )?GovernmentScienceArts
Properties of kNN kNN performance sensitive to k. When k 1? Noise (i.e., an error) in the category label of a single training example. More robust alternative is to find the k most-similar examplesand return the majority category of these k examples. When k the number of all documents? Value of k is typically odd to avoid ties; 3 and 5 are mostcommon. Time complexity when testing:𝑂(𝑛 𝑉 ) where 𝑛 is the number oftraining documents and 𝑉 is the vocabulary size.Properties of kNN Nearest neighbor method depends on a similarity (or distance)metric. Simplest for continuous m-dimensional instance: space isEuclidean distance. Simplest for m-dimensional binary instance space: is Hammingdistance (number of feature values that differ). For text, cosine similarity of tf.idf weighted vectors is typicallymost effective.
9/20/16Illustration of 3 Nearest Neighbor for Text VectorSpaceCSE 398/49825kNN vs. Rocchio Nearest Neighbor tends to handle polymorphic categories betterthan Rocchio.Why kNN can handle this?CSE 398/4982613
Optimization for logistic regressionLikelihood function:Log-likelihood function:Gradient of log-likelihoodLinear classification Many common text classifiers are linear classifiers Naïve BayesPerceptronRocchioLogistic regressionSupport vector machines (with linear kernel)Linear regression with threshold Despite this similarity, noticeable performance differences For separable problems, there is an infinite number of separating hyperplanes. Whichone do you choose? What to do for non-separable problems? Different training methods pick different hyperplanes Classifiers more powerful than linear often don’t perform better on text problems.
Linear classification Can find separating hyperplane by linear programming(or can iteratively fit solution via perceptron): separator can be expressed as ax by cFind a,b,c, such thatax by c for red pointsax by c for blue points.Example linear text classifier Class: “interest” (as in interest rate) Example features of a linear classifier wi ti0.70 prime0.67 rate0.63 interest0.60 rates0.46 discount0.43 bundesbankwi ti 0.71 dlrs 0.35 world 0.33 sees 0.25 year 0.24 group 0.24 dlr To classify, find dot product of feature vector and weights
Which hyperplane? Lots of possible solutions for a,b,c. Some methods find a separatinghyperplane, but not the optimal one[according to some criterion of expected goodness] E.g., perceptron Most methods find an optimal separatinghyperplane Which points should influence optimality? All points Linear/logistic regression Naïve Bayes Only “difficult points” close to decisionboundary Support vector machinesProperties of text classification High dimensional data: thousands or millions of features, somerelevant, many are irrelevant Documents are zero along almost all axes Most document pairs are very far apart (i.e., not strictlyorthogonal, but only share very common words and a fewscattered others) In classification terms: often document sets are separable, formost any classification This is part of why linear classifiers are quite successful in thisdomain
More than one class Multi-labeled classification A document can belong to 0, 1, or 1 classes. Decompose into n binary problems Quite common for documents Multi-class classification Classes are mutually exclusive. Each document belongs to exactly one class E.g., digit recognition. Digits are mutually exclusive
One-vs-all classificaiton Build a separator between each class and its complementaryset (docs from all other classes). Given test doc, evaluate it for membership in each class. Assign document to class with: maximum score(s) maximum confidence(s) maximum probability (probabilities)?
Text MiningClusteringTopics Clustering. Motivation Quality of clustering Clustering methods. Flat clustering (K-means)
What is clustering Clustering: the process of grouping a set of objects into classesof similar objects Documents within a cluster should be similar. Documents from different clusters should be dissimilar. The commonest form of unsupervised learning Unsupervised learning learning from raw data, as opposed tosupervised data where a classification of examples is givenA data set with clear cluster structure Grouping the following points into 3 groups (clusters), based onsimilarity/distance.
Motivating example Document clusteringWords having multiple meaningsMultiple meaningsof the word“Cluster”, eachmeaning isrepresented bya set of documents6
Helping information retrieval Cluster hypothesis - Documents in the same cluster behave similarly with respect torelevance to information needs Therefore, to improve search recall: Cluster docs in corpus a priori When a query matches a doc D, also return other docs in the cluster containing D Hope if we do this: The query “car” will also return docs containing automobile Because clustering grouped together docs containing car with those containingautomobile.Cluster for “car” and “automobile”Motivating example Word clustering grouping words withsimilar topictogether 5 topics: shopping,tech, tagging, rdf,firefox8
Issues of clustering How many clusters? Do you know that before clustering? Too many? Too few? Which distance measure to adopt? e.g. cosine vs. Euclidean?
Categorization of clustering algorithms Based on methodology. Flat algorithms Usually start with a random (partial) partitioning Refine it iteratively K means clustering (Model based clustering) Hierarchical algorithms Bottom-up, agglomerative (Top-down, divisive)Categorization of clustering algorithms Based on results. Hard clustering: Each document belongs to exactly one cluster More common and easier to do Soft clustering: A document can belong to more than one cluster. Makes more sense for applications like creating browsable hierarchies You may want to put a pair of sneakers in two clusters: (i) sports apparel and(ii) shoes You can only do that with a soft clustering approach.
Partitioning Algorithms Partitioning method: Construct a partition of n documentsinto a set of K clusters Given: a set of documents and the number K Find: a partition of K clusters that optimizes the chosenpartitioning criterion Globally optimal Intractable for many objective functions Ergo, exhaustively enumerate all partitions Effective heuristic methods: K-means and K-medoidsalgorithmsK-means Assumes documents are real-valued vectors. Clusters based on centroids (aka the center of gravity or mean)of points in a cluster, c:!!1xµ(c) c x! c Reassignment of instances to clusters is based on distance tothe current cluster centroids. (Or one can equivalently phrase it in terms of similarities)
K-Means Algorithm Select K random docs {s1, s2, sK} as seeds. Until clustering converges (or other stopping criterion): For each doc di: Assign di to the cluster cj such that dist(xi, sj) is minimal. (Next, update the seeds to the centroid of each cluster) For each cluster cj sj µ(cj)A running example (K 2)Pick seedsReassign clustersCompute centroidsxxxReassign clustersxCompute centroidsReassign clustersConverged!
When to stop? Several possibilities, e.g., A fixed number of iterations. Doc partition unchanged. Centroid positions don’t change. Are the last two conditions the same?Convergence Convergence? Why should the K-means algorithm ever reach a fixedpoint? A state in which clusters don’t change. K-means is a special case of a general procedure known asthe Expectation Maximization (EM) algorithm. EM is known to converge. Number of iterations could be large. But in practice usually isn’t!
Convergence Define goodness measure of cluster k as sum of squareddistances from cluster centroid: Gk Σ i (di – ck )2(sum over all di in cluster k) G Σk Gk Reassignment monotonically decreases G since eachvector is assigned to the closest centroid.Convergence of K-Means Recomputation monotonically decreases each Gk since(mk is number of members in cluster k): Σ (di – a)2 reaches minimum for: Σ –2(di – a) 0 Σ di Σ a mK a Σ di a (1/ mk ) Σ di ck K-means typically converges quickly
Sensitivity to seed set selection Results can vary based on randomseed selection. Some seeds can result in poorconvergence rate, or convergenceto sub-optimal clusterings. Select good seeds using a heuristic(e.g., doc least similar to any existingmean) Try out multiple starting points Initialize with the results of anothermethod.Example showingsensitivity to seedsIn the above, if you startwith B and E as centroidsyou converge to {A,B,C}and {D,E,F}If you start with D and Fyou converge to{A,B,D,E} {C,F}
How many clusters? Number of clusters K is given Partition n docs intopredetermined number ofclusters Finding the “right” number ofclusters is part of the problem Given docs, partition into an“appropriate” number of subsets. E.g., for Google news - we knowthe number of clusters (sports,politics, finance).
Text MiningWordCollocationWhat is word collocation “an expression consisting of two or more words that correspondto some conventional way of saying things.” “Collocations of a given word are statements of the habitual orcustomary places of that word” Examples: “stiff breeze”, “strong tea”, “powerful drug”, “broaddaylight”, “weapons of mass destruction”, “make up”, “check in”
What is word collocation (Choueka, 1988)[A collocation is defined as] “a sequence of two or more consecutive words, thathas characteristics of a syntactic and semantic unit, and whose exact andunambiguous meaning or connotation cannot be derived directly from themeaning or connotation of its components." Criteria: abilitynon-translatable word for wordWord collocation A phrase is compositional if its meaning can be predicted fromthe meaning of its parts Collocations have limited compositionality there is usually an element of meaning added to the combination Ex: strong tea Idioms are the most extreme examples of non-compositionality Ex: to hear it through the grapevine
Word collocation We cannot substitute near-synonyms for the components of acollocation. Strong is a near-synonym of powerful strong tea?powerful tea yellow is as good a description of the color of white wines white wine?yellow wine Many collocations cannot be freely modified with additionallexical material or through grammatical transformations weapons of mass destruction -- ?weapons of massive destruction to be fed up to the back teeth -- ?to be fed up to the teeth in the backTypes of collocations Verb particle/phrasal verb constructions to go down, to check out, Proper nouns John Smith Terminological expressions concepts and objects in technical domains hydraulic oil filter Idioms to hear it through the grapevines.
Why study word collocation In natural language generation The output should be natural make a decision?take a decision In lexicography Identify collocations to list them in a dictionary To distinguish the usage of synonyms or near-synonyms In parsing To give preference to most natural attachments plastic (can opener)? (plastic can) opener In corpus linguistics and psycholinguists Ex: To study social attitudes towards different types of substances strong cigarettes/tea/coffee powerful drug(Near-)Synonyms To determine if 2 words are synonyms-- Principle of substitutability: 2 words are synonym if they can be substituted for one another insome?/any? sentence without changing the meaning or acceptability of thesentence How big/large is this plane? Would I be flying on a big/large or small plane? Miss Nelson became a kind of big / ? large sister to Tom. I think I made a big / ? large mistake.
Frequency based method Justeson and Katz’s filter Hypothesis:§ if 2 words occur together very often, they must be interestingcandidates for a collocation Method:§ Select the most frequently occurring bigrams (sequence of 2 adjacentwords)Example Except for “New York”, all bigrams arepairs of function wordsNeed some additional information to filterthese outTag PatternExampleANlinear functionNNregression coefficientAA NGaussian random variableANNcumulative distribution functionNANmean squared errorNNNclass probability functionN PNdegrees of freedom
Example Based on POS tags and frequency Simple method that works very well.“The portfolio is fine except for the fact that the lastmovement of sonata #6 is missing .”[(’The’, ’DT’), (’portfolio’, ’NN’), (’is’, ’VBZ’), (’fine’, ’JJ’),(’except’, ’IN’), (’for’, ’IN’), (’the’, ’DT’), (’fact’, ’NN’), (’that’,’IN’), (’the’, ’DT’), (’last’, ’JJ’), (’movement’, ’NN’), (’of’,’IN’), (’sonata’, ’NN’), (’#6’, ’CD’), (’is’, ’VBZ’), (’missing’,’VBG’), (’.’, ’.’)]S
Text mining vs. other approaches Data Science: focus on data processing, with simple mining models. Data Mining: focus on general mining techniques for more general data formats. AI: a general area, providing some techniques for text mining. Database: focuses on structured data, while text data are highly unstructured.