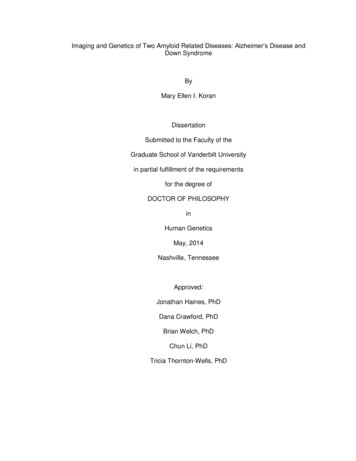
Transcription
Imaging and Genetics of Two Amyloid Related Diseases: Alzheimer’s Disease andDown SyndromeByMary Ellen I. KoranDissertationSubmitted to the Faculty of theGraduate School of Vanderbilt Universityin partial fulfillment of the requirementsfor the degree ofDOCTOR OF PHILOSOPHYinHuman GeneticsMay, 2014Nashville, TennesseeApproved:Jonathan Haines, PhDDana Crawford, PhDBrian Welch, PhDChun Li, PhDTricia Thornton-Wells, PhD
Copyright 2014 by Mary Ellen Irene KoranAll Rights Reserved
DEDICATIONTo my parents, sister, Cody, friends, and family for all of their encouragement andsupport throughout this adventure.iii
ACKNOWLEDGEMENTSFirst, I would like to thank Dr. Tricia Thornton-Wells, who has been an amazing mentor,incredibly supportive and encouraging, and from whom I have learned much aboutmentoring students. I would also like to thank my committee members, Drs. JonathanHaines, Dana Crawford, Chun Li, and Brian Welch whose constructive guidance helpedguide and shape this dissertation and my career as a scientist. Dr. Haines has providedvaluable insight into deciphering the genetics of Alzheimer’s Disease, which resulted insignificant contributions to Chapters 4 and 5 of this dissertation, and has been anindispensable mentor from the beginning, encouraging me to continue in the pursuit of ascientific career. Drs. Crawford and Li have substantially influenced the work describedin Chapters 4 and 5, providing expertise in the statistical interpretation of the results andthe construction of the applied methods, in addition to their encouraging reflections. Dr.Welch’s contributions to Chapters 2 and 3 were indispensable: his knowledge ofmagnetic resonance physics, and more importantly, his ability and willingness to teachand share this knowledge were vital throughout the application and optimization of thesequences, the implementation of post-processing analyses, and the interpretation ofthe results. He was always supportive and positive, and has the rare talent of being ableto turn extremely difficult theories into easily digestible concepts.I would like to thank others beyond my committee as well. Dr. Timothy J. Hohman hasapplied his considerable expertise in neuroscience, imaging, statistics, and programmingto this entire dissertation; I honestly would not have been able to accomplish as much asI did without his help and guidance. He is always willing to drop what he is doing to helpothers, and for this I am eternally grateful. R. Michael Sivley has applied his extensiveiv
knowledge in programming to this entire dissertation as well, particularly Chapter 2 andAppendix A. Both Mike and Tim have become friends to me, and lab would not be thesame without them here.I would like to thank all the study volunteers for their time and willingness to participate inthis research; without them this body of work would not exist.I would like to thank my friends and family. From the beginning, they have beenextremely loving and supportive. My mother has provided the encouragement to pursuewhatever interests I could possibly imagine, my father has instilled the drive, ambition,and perseverance that continue to shape my personality and decisions, and my sisterkeeps me in check and brings me “back to earth” when I get too far away. Finally, I mustthank my partner in life, Cody Goodwin. I honestly don’t think I would have made itthrough the tedium that is the first year of medical school without him. He provides mewith balance and a healthy perspective on life and work, stimulates me intellectually, andmost importantly, loves me unconditionally.v
TABLE OF CONTENTSPageDEDICATION . iiiACKNOWLEDGEMENTS . ivLIST OF TABLES .xLIST OF FIGURES . xiiChapter1. IMAGING GENETICS OF ALZHEIMER’S DISEASE AND DOWN SYNDROME:TWO AMYLOID BASED DISEASES . 1Alzheimer’s Disease . 1Down Syndrome . 3Imaging Phenotypes in Alzheimer’s Disease and Down Syndrome . 6Structural Imaging . 6Amyloid Imaging. 8Utilizing Imaging Modalities in Genetic Studies . 9Imaging Genetics . 10Imaging Genetics in LOAD . 10Gaps in Knowledge Addressed . 152. VALIDATION OF T1Ρ MAGNETIC RESONANCE IMAGING IN HUMAN ADULTSWITH AMYLOID BETA PLAQUE DEPOSITION . 17Introduction . 17Methods and Materials . 20Subjects . 20MRI . 23Imaging Analysis . 24Results. 34Discussion . 37vi
3. DIFFERENCES IN AGE-RELATED EFFECTS ON BRAIN VOLUME IN DOWNSYNDROME AS COMPARED TO WILLIAMS SYNDROME AND TYPICALDEVELOPMENT . 42Introduction . 42Etiology and Presentation of Down Syndrome and WilliamsSyndrome. 42Brain Morphometry in Down Syndrome and Williams Syndrome . 44Study Aims . 45Methods . 45Study Participants . 45Magnetic Resonance Imaging Acquisition . 48Neuroimaging Analysis. 48Genotyping. 52Cognitive Testing . 52Statistical Analysis . 53Secondary Exploratory Analyses . 54Results. 56Discussion . 654. GENETIC INTERACTIONS ASSOCIATED WITH LONGITUDINAL CHANGES INVENTRICLE SIZE IN ALZHEIMER’S DISEASE . 70Introduction . 70Materials and Methods . 73Subjects . 74Genotyping. 75Analysis of Imaging Data . 75SNP-SNP Interaction Analysis . 76Results. 79Pathway Based Interaction Analysis: . 79Discussion . 87Conclusions . 89Supporting Information . 915. GENETIC INTERACTIONS FOUND BETWEEN CALCIUM CHANNEL GENESMODULATE AMYLOID LOAD MEASURED BY POSITRON EMISSIONTOMOGRAPHY . 92vii
Introduction . 92Methods . 95Subjects . 95Genotyping . 96Effects of interactions on amyloid deposition. 97Quantification of amyloid deposition . 97SNP-SNP interaction analysis: Discovery. 98SNP-SNP interaction analysis: Stage 1 Validation . 101SNP-SNP interaction analysis: Stage 2 Validation . 101Results. 102Discovery Dataset . 102Stage 1 Validation Dataset . 102Stage 2 Validation Dataset . 103Discussion . 106Calcium homeostasis and its relationship to amyloidogenesis. 106RYR3 and CACNA1C. 107RYR3 in Alzheimer’s Disease pathogenesis . 108CACNA1C in Alzheimer’s Disease pathogenesis . 109RYR3-CACNA1C interaction and amyloid load . 109Conclusion . 110Supporting Information . 1126. CONCLUSIONS AND FUTURE DIRECTIONS . 113Summary and Future Directions for Chapter 2 . 114Summary and Future Directions for Chapter 3 . 115Summary and Future Directions for Chapter 4 . 116Summary and Future Directions for Chapter 5 . 117Conclusions . 120AppendixA. SUPPORTING INFORMATION FOR CHAPTER 2 . 121Other images acquired/analyzed . 121T1 and T2 . 121Region of Interest Based Analysis . 124FreeSurfer Log Creation Code . 124FreeSurfer Template and Recon-All code . 125FreeSurfer Post-Processing and Analysis Template . 125MATLAB and SPM Code for T1ρ, T1, and T2 calculation . 128Voxel Based Analysis . 135Analysis Template . 135MATLAB and SPM Code for T1ρ, T1, and T2 calculation . 136viii
Quantifying Images . 139Quantification of T1ρ-weighted Images . 139Quantification of T1 Images . 142B. SUPPORTING INFORMATION FOR CHAPTER 3 . 146C. SUPPORTING INFORMATION FOR CHAPTER 4 . 159D. SUPPORTING INFORMATION FOR CHAPTER 5 . 161Code for InterSNP program used in gene-gene interaction analysis . 161REFERENCES . 163ix
LIST OF TABLESTablePage1 Demographics for community-based recruitment. .222 Demographics for subjects with T1ρ analyzed. .253 Regions of interest (ROIs) tested in T1ρ validation analysis. .354 Demographics for volume analysis .475 Regions of interest included in volume analysis .506 Regions of interest not included in volume analysis .517 Relationship between volume and DLD cognitive scores or APOE in brain regionsshowing a correlation with age in Down Syndrome participants .558 Brain regions where volume had a significant relationship with age betweenparticipants with Down Syndrome and typically developing controls in the modelVolume of ROI Age Sex Diagnosis Diagnosis x Age. .569 Brain regions where volume had a significant relationship with age betweenparticipants with Down Syndrome and Williams Syndrome in the model Volume ofROI Age Sex Diagnosis Diagnosis x Age. .5610 Brain regions where volume had a significant relationship with age betweenparticipants with Down Syndrome and typically developing controls after outliersremoved. .6011 Brain regions where volume had a significant relationship with age betweenparticipants with Down Syndrome and Williams Syndrome after outliers removed. .6012 Demographic data for genetic interaction association with longitudinal inferiorlateral ventricle volume change .74x
13 Contingency tables for significant SNP-SNP interactions from genetic interactionassociation with longitudinal inferior lateral ventricle volume change .7814 Contingency tables for SNP-SNP interaction between SYNJ2 and PI4KA forassociation study with longitudinal inferior lateral ventricle volume change. .8015 Full results with Bonferroni corrected significant SNP-SNP interactions forgenetic interaction association with longitudinal inferior lateral ventricle volumechange. .8616 Demographic data for genetic interaction association with PET amyloid load .9617 Genes included in discovery dataset of the genetic interaction association withPET amyloid load. .10018 Significant SNP-SNP Interactions in RYR3-CACNA1C from discovery andvalidation analyses from genetic interaction association with PET amyloid load .10419 SNP-SNP Interactions in RYR3-CACNA1C from Stage 2 validation of geneticinteraction association with PET amyloid load .10420 Results from analysis of relationship between volume and age using typicallydeveloping controls as the control group. .14621 Results from analysis comparing relationship with volume and age betweenparticipants with Down Syndrome and Williams Syndrome. .15322 Post-hoc analysis of effect of significant interactions between SYNJ2-PI4KA,PARD3-MYH2, PDE3A- ABHD12B, and OR2L13-PRKG1 on the volume of RILVand LILV combined.15923 Mapping of Single Nucleotide Polymorphisms from significant interactions togene and base pair location. .161xi
LIST OF FIGURESFigurePage1 T1ρ-weighted MRI validated in a mouse model of Alzheimer’s Disease. .182 T1ρ- weighted MRI maps in human subjects with Alzheimer’s Disease. .203 Example of image acquisition for T1ρ validation study. .244 Quantification of T1ρ weighted MRI and the T1ρ dispersion curve .285 Exchange rate contrast (ERC) equation and calculation for the T1ρvalidation study.296 Region of interest (ROI) based analysis pipeline using FreeSurfer ROI parcellationand segmentation for the T1ρ validation study.317 Voxel-based analysis pipeline for the T1ρ validation study .328 Overview of both region of interest (ROI) and voxel-based pipelines for the T1ρvalidation study.339 Example calculated T1ρ maps for human subjects. .3410 Example of quantified T1ρ dispersion curves plotted in human subjects. .3611 Example of quantified exchange rate contrast (ERC) values of the lefthippocampus plotted within groups.3712 Representative histograms of the T1ρ values in AD-related regions of interest. .4013 Relationship between inferior lateral ventricle volume and age in DownSyndrome, Williams Syndrome, and typically developing controls. .58xii
14 Relationship between regional brain volumes and age in Down Syndrome,Williams Syndrome, and typically developing controls. .5915 Relationship between regional brain volumes and age in Down Syndrome,Williams Syndrome, and typically developing controls after outliers were removed. .6116 Regional brain volumes in relation to Dementia Questionnaire for People withLearning Disabilities-sum of cognitive scores (DLD-SCS) in the Down Syndromecohort. .6317 Regional brain volumes in relation to Dementia Questionnaire for People withLearning Disabilities-sum of social scores (DLD-SOS) in the Down Syndrome cohort. .6418 Relationship between age and brain region volumes stratified by APOE risk allelecarrier status.6519 Effect of SYNJ2 and PI4KA interaction on right inferior lateral ventricle volumechange. .8120 Effect of SYNJ2 and PI4KA interaction on left inferior lateral ventricle volumechange. .8221 Effect of SYNJ2 and PI4KA interaction on right inferior lateral ventricle volumechange across diagnoses. .8322 Effect of SYNJ2 and PI4KA interaction on left inferior lateral ventricle volumechange across diagnoses. .8423 Phosphatidylinositol and Akt/PKB Survival Signaling Pathway. .8824 Discovery dataset results from study of gene-gene interactions associated withamyloid load. .10525 Stage 1 validation dataset results from study of gene-gene interactionsassociated with amyloid load. .10526 Stage 2 validation dataset results from study of gene-gene interactionsassociated with amyloid load. .106xiii
27 Dispersion curves with quantified T1 images included at 2000 Hz. .12228 Example of discrepancy between expected and actual dispersion curve when R2(1/T2) was used.12329 Dispersion curve with both R2 and R1ρ collected at 0Hz plotted.124xiv
Chapter 1IMAGING GENETICS OF ALZHEIMER’S DISEASE AND DOWN SYNDROME: TWOAMYLOID BASED DISEASESThe advancement of in vivo imaging modalities that detect the neuropathologiesassociated with both Down Syndrome and Alzheimer’s Disease present newopportunities to explore these diseases in living human subjects. Previously, theseneuropathologies could not be detected until after autopsy or in a living patient, with ararely taken brain biopsy. The use of quantitative traits derived from these imagingmodalities offers increased power to detect associations with large-scale genetic data,and these studies fall under the category of imaging genetics. Imaging genetics studiescan identify novel risk genes and elucidate gene function and novel mechanisms ofdisease pathology and etiology. Recent imaging genetics studies of theneuropathologies of Alzheimer’s Disease and Down Syndrome have attempted to obtaina more complete and in depth understanding of the underlying genetic etiology of theirpathologies. Here, we will briefly overview both diseases, the neuropathologiesassociated with each, the imaging modalities used to detect these pathologies, andfinally, the studies combining these imaging modalities with genetic data.Alzheimer’s DiseaseAlzheimer’s Disease (AD) is an irreversible, degenerative disease of the brain thataccounts for an estimated 55-75% of dementia cases each year 1. This disorder resultsin a gradual loss of memory, judgment and cognitive function along with the massive1
death of brain cells. While AD usually appears in individuals 65 years of age and older,less common forms do affect individuals earlier in adulthood. In 2000, there were 4.8million people in the United States with AD , and that number is expected to increase to13.2 million by 2050 2, prompting many researchers throughout the scientific communityto eagerly research ways in which the disease can be detected and prevented, orslowed.Late onset Alzheimer’s disease (LOAD) accounts for about 96% of AD cases anddevelops in patients over the age of 65, with early-onset AD affecting subjects less than65 years of age and accounting for 4% of the AD population 3. Clinical diagnosis ofLOAD is based on a patient’s symptoms and cognitive function assessed using a batteryof neuropsychological tests 4. When an individual is in the early-stages of AD,characteristic symptoms include problems with thinking, memory and concentration thatmay be attributed to normal aging and stress. As the disease progresses into mildcognitive impairment (MCI) and various stages of dementia, cognitive functions continueto decline. Most noticeably, short term memory becomes highly impaired 5. In addition,language abilities, motor skills, perception and attention deteriorate eventually leavingmany people unable to care for themselves and completely dependent on others 6. Evenwith neuropsychological testing of these features of disease, LOAD cannot bedefinitively diagnosed until autopsy, when the neuropathological hallmarks can bedetected. These hallmarks include amyloid beta plaques that can beimmunohistochemically analyzed in brain sections 4. Large amounts of amyloid betapeptide, a toxic protein fragment, are produced in the brain and as this peptideaccumulates in the brain, extracellular senile amyloid beta plaques form 7. Although stilldebated, these plaques may lead to nerve cell death and the progressive symptoms of2
this disorder 8. The brain of a patient with AD is marked by profound atrophy of thehippocampus and cerebral cortex 9.LOAD has a complex genetic etiology that has proven difficult to unravel, involvingheterogeneity and gene-gene and gene-environment interactions 1. LOAD is notinherited in a Mendelian (dominant or recessive) manner, but the disease does cluster infamilies 1. The heritability estimates of LOAD are high, with h2 between 60 and 80%, butmuch of the genetic contribution to this condition remains unexplained 10,11.Early work investigating the genetic contributions to LOAD found associations betweendisease status and the gene encoding apolipoprotein-E (APOE) 12,13. Genetic studies inLOAD have continued with single marker genetic associations, or genome wideassociation studies (GWAS), in which disease status is the outcome variable. In thesestudies, 21 genes/genomic loci that have been confirmed to be associated with LOAD,but only APOE has a large effect size, with an odds ratio of about 3.7 for one copy of thehigh risk e4 allele 11,14. The remaining 20 loci exhibit small effect sizes, with odds ratioshovering between 0.73 and 1.22 (these include: BIN1, CLU, ABCA7, CR1, PICALM,MS4A6A, CD33, MS4A4E, CD2AP, EPHA1, HLA-DRB5–HLA-DRB1, PTK2B, SORL1,SLC24A4-RIN3, DSG2, INPP5D, MEF2C, NME8, ZCWPW1, CELF1, FERMT2, andCASS411,14. In depth reviews discussing the genetics of LOAD have been publishedelsewhere 15–17.Down SyndromeDown syndrome (DS; trisomy 21) is a neurodevelopmental disorder that is caused by thepresence of three copies of chromosome 21. It occurs in 1 in every 691 live births in theUS 18 and is the most common genetic cause of intellectual disability 19,20. DS is typically3
identified at birth or shortly thereafter and can be diagnosed prenatally using acombination of ultrasound, blood tests, amniocentesis, or chorionic villus sampling.Postnatally, the diagnosis of DS is initially based on common physical characteristicsthat can be observed in infancy, such as low muscle tone, a single crease across thepalm of the hand, brachycephaly, and upward slanting palpebral fissures. Diagnosis canthen be confirmed with karyotype analysis. The degree of cognitive impairmentassociated with DS ranges from mild to severe, with the mean IQ of 50 20. Individualswith DS exhibit deficits in language, verbal short term-memory, and explicit long-termmemory; whereas visuospatial short-term memory, associative learning, and implicitmemory are relatively preserved 21. Common medical comorbidities include heartdefects, digestive malformations, cataracts, seizures, leukemia, and sleep apnea.Advances in the treatment of these medical comorbidities have resulted in dramaticimprovements in lifespan in the U.S., rising from 9 years in the early-twentieth century 22to nearly 60 years in 2010 23. With this substantial increase in life expectancy forindividuals with DS has come the need for an increased focus on health issues in agingpeople with DS. Most prominently, aging in individuals with DS is associated with anincreased risk of developing AD, with up to 70 percent developing dementia by age 70 24.Adults with DS account for up to 60% of individuals with developmental disabilities whoexhibit signs of AD 25. The clinical presentation of AD in individuals with DS can becomplex and less straightforward due to pre-existing intellectual disability. Furthercomplicating the diagnostic picture is that the most commonly used neuropsychologicalbatteries for assessing dementia in the general population are not effective in measuringcognitive dysfunction in people with intellectual disabilities because these subjects tendto score at or near the bottom of the normative range, exhibiting floor effects. Therefore,AD in subjects with DS is clinically diagnosed through specially designed4
neuropsychological tests like the Dement
Mary Ellen I. Koran Dissertation Submitted to the Faculty of the Graduate School of Vanderbilt University in partial fulfillment of the requirements for the degree of DOCTOR OF PHILOSOPHY in Human Genetics May, 2014 Nashville, Tennessee Approved: Jonathan Haines, PhD Dana Crawford, PhD Brian Welch, PhD Chun Li, PhD Tricia Thornton-Wells, PhD