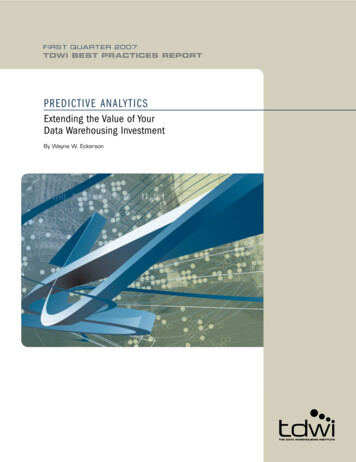
Transcription
FIRST QUARTER 2007TDWI BEST PRACTICES REPORTPREDICTIVE ANALYTICSExtending the Value of YourData Warehousing InvestmentBy Wayne W. Eckerson
Research SponsorsMicroStrategy, Inc.OutlookSoft CorporationSASSPSSSybase, Inc.Teradata, a division of NCR
FIRST QUARTER 2007TDWI BEST PRACTICES REPORTPREDICTIVE ANALYTICSExtending the Value of YourData Warehousing InvestmentBy Wayne W. EckersonTable of ContentsResearch Methodology and Demographics . . . . . . . . . . . . . . . . . . . . . . . . . . . . 3What Is Predictive Analytics? . . . . . . . . . . . . . . . . . . . . . . . . . . . . . . . . . . . . . . 4Definitions . . . . . . . . . . . . . . . . . . . . . . . . . . . . . . . . . . . . . . . . . . . . . . . . . . . . . 5The Business Value of Predictive Analytics . . . . . . . . . . . . . . . . . . . . . . . . . . 8Measuring Value . . . . . . . . . . . . . . . . . . . . . . . . . . . . . . . . . . . . . . . . . . . . . . . . 8How Do You Deliver Predictive Analytics? . . . . . . . . . . . . . . . . . . . . . . . . . . 10The Process of Predictive Modeling. . . . . . . . . . . . . . . . . . . . . . . . . . . . . . . . 111. Defining the Project. . . . . . . . . . . . . . . . . . . . . . . . . . . . . . . . . . . . . . . . . . . 122. Exploring the Data . . . . . . . . . . . . . . . . . . . . . . . . . . . . . . . . . . . . . . . . . . . 123. Preparing the Data . . . . . . . . . . . . . . . . . . . . . . . . . . . . . . . . . . . . . . . . . . . 134. Building Predictive Models . . . . . . . . . . . . . . . . . . . . . . . . . . . . . . . . . . . . . 145. Deploying Analytical Models . . . . . . . . . . . . . . . . . . . . . . . . . . . . . . . . . . . 156. Managing Models . . . . . . . . . . . . . . . . . . . . . . . . . . . . . . . . . . . . . . . . . . . . 18Trends in Predictive Analytics . . . . . . . . . . . . . . . . . . . . . . . . . . . . . . . . . . . . . 19Analytics Bottleneck . . . . . . . . . . . . . . . . . . . . . . . . . . . . . . . . . . . . . . . . . . . . 19Advances in Predictive Analytics Software . . . . . . . . . . . . . . . . . . . . . . . . . 20Database-Embedded Analytics . . . . . . . . . . . . . . . . . . . . . . . . . . . . . . . . . . . 22BI-Enabled Analytics and Applications . . . . . . . . . . . . . . . . . . . . . . . . . . . . . 24Industry Standards for Interoperability . . . . . . . . . . . . . . . . . . . . . . . . . . . . . 26Recommendations . . . . . . . . . . . . . . . . . . . . . . . . . . . . . . . . . . . . . . . . . . . . . . . 271. Hire business-savvy analysts to create models. . . . . . . . . . . . . . . . . . . . . 272. Nurture a rewarding environment to retain analytic modelers . . . . . . . . 283. Fold predictive analytics onto the information management team . . . . . 294. Leverage the data warehouse to prepare and score the data . . . . . . . . . 305. Build awareness and confidence in the technology . . . . . . . . . . . . . . . . . 31Conclusion . . . . . . . . . . . . . . . . . . . . . . . . . . . . . . . . . . . . . . . . . . . . . . . . . . . . . . 32www.tdwi.org1
PREDIC T I V E A N ALY T IC SAbout the AuthorWAYNE W. ECKERSON is director of research and services for The Data Warehousing Institute(TDWI), a worldwide association of business intelligence and data warehousing professionalsthat provides education, training, research, and certification. Eckerson has 17 years of industryexperience and has covered data warehousing and business intelligence since 1995.Eckerson is the author of many in-depth reports, a columnist for several business and technologymagazines, and a noted speaker and consultant. He authored the book Performance Dashboards:Measuring, Monitoring, and Managing Your Business, published by John Wiley & Sons in October2005. He can be reached at weckerson@tdwi.org.About TDWITDWI, a division of 1105 Media, Inc., is the premier provider of in-depth, high-quality educationand research in the business intelligence and data warehousing industry. Starting in 1995 with asingle conference, TDWI is now a comprehensive resource for industry information and professionaldevelopment opportunities. TDWI sponsors and promotes quarterly World Conferences, regionalseminars, onsite courses, a worldwide Membership program, business intelligence certification,resourceful publications, industry news, an in-depth research program, and a comprehensive Website: www.tdwi.org.About TDWI ResearchTDWI Research provides research and advice for BI professionals worldwide. TDWI Researchfocuses exclusively on BI/DW issues and teams up with industry practitioners to deliver bothbroad and deep understanding of the business and technical issues surrounding the deployment ofbusiness intelligence and data warehousing solutions. TDWI Research offers reports, commentary,and inquiry services via a worldwide Membership program and provides custom research,benchmarking, and strategic planning services to user and vendor organizations.AcknowledgmentsTDWI would like to thank many people who contributed to this report. First, we appreciate themany users who responded to our survey, as well as those who responded to our requests for phoneinterviews. We would also like to recognize TDWI’s account and production team: Jennifer Agee,Bill Grimmer, Denelle Hanlon, Deirdre Hoffman, and Marie McFarland.SponsorsMicroStrategy, Inc., OutlookSoft Corporation, SAS, SPSS Inc, Sybase, Inc., and Teradata,a division of NCR, sponsored the research for this report.2TDWI RESE A RCH 2006 by 1105 Media, Inc. All rights reserved. Printed in the United States. TDWI is a trademark of 1105 Media, Inc. Other product and companynames mentioned herein may be trademarks and/or registered trademarks of their respective companies. TDWI is a division of 1105 Media, Inc.,based in Chatsworth, CA.
Research MethodologyResearch MethodologyFocus. This report is designed for the business ortechnical manager who oversees a BI environmentand wishes to learn the best practices and pitfalls ofimplementing a predictive analytics capability. While itdiscusses some technical issues, it is designed to educatebusiness managers about how to drive greater valuefrom their existing investments in data warehousing andinformation delivery systems.DemographicsPositionMethodology. The research for this report is based ona survey that TDWI conducted in August of 2006, aswell as interviews with BI and analytics practitioners,consultants, and solution providers. To conduct thesurvey, TDWI sent e-mail messages to IT professionals inTDWI’s and 1105 Media’s databases. (TDWI is a businessunit of 1105 Media.) A total of 888 people responded tothe survey, including 55 people whose responses we didnot count since they work for a BI vendor in a sales ormarketing capacity, or are professors or students. Thus,our analysis was based on responses from 833 people. Ofthis group, 168 had either partially or fully implementeda predictive analytics solution. Most of our survey analysisis based on the answers provided by these 168 respondents.Percentages may not always add up to 100% due torounding or questions that allow respondents to selectmore than one answer.RoleLocationRespondent Profile. A majority of the 833 qualifiedsurvey respondents (61%) are corporate IT professionalswho serve as mid-level managers in the United States andwho work for large organizations. (See charts.)Company Profile. A majority (58%) work in groupsthat support the entire enterprise, while 20% support abusiness unit, and 16% support multiple departments.The industries with the highest percentage are consultingand professional services (13%), financial services(12%), software/internet (9%), and insurance (8%.)Respondents work for companies of various sizes. Onefifth of respondents (21%) hail from companies with lessthan 100 million in revenue a year, while another 26%of respondents come from companies that earn less than 1 billion, while 15% come from companies with annualrevenues of between 1 billion and 5 billion.IndustryBased on 750 qualified respondents.www.tdwi.org3
PREDIC T I V E A N ALY T IC SWhat Is Predictive Analytics?Consider the power of predictive analytics: A Canadian bank uses predictive analytics to increase campaign response rates by 600%,cut customer acquisition costs in half, and boost campaign ROI by 100%. A large state university predicts whether a student will choose to enroll by applyingpredictive models to applicant data and admissions history. A research group at a leading hospital combined predictive and text analytics to improve itsability to classify and treat pediatric brain tumors. An airline increased revenue and customer satisfaction by better estimating the number ofpassengers who won’t show up for a flight. This reduces the number of overbooked flightsthat require re-accommodating passengers as well as the number of empty seats.As these examples attest, predictive analytics can yield a substantial ROI. Predictive analyticscan help companies optimize existing processes, better understand customer behavior, identifyunexpected opportunities, and anticipate problems before they happen. Almost all of TDWI’sLeadership Award1 winners in the past six years have applied predictive analytics in some form oranother to achieve breakthrough business results.High Value, Low Penetration. With such stellar credentials, the perplexing thing about predictiveanalytics is why so many organizations have yet to employ it. According to our research, only 21%of organizations have “fully” or “partially” implemented predictive analytics, while 19% have aproject “under development” and a whopping 61% are still “exploring” the issue or have “no plans.”(See Figure 1.)Status of Predictive AnalyticsFigure 1. Predictive analytics is still in an early-adopter phase. Based on 833 respondents to a TDWI surveyconducted August 2006.Predictive analytics is also an arcane set of techniques and technologies that bewilder many businessand IT managers. It stirs together statistics, advanced mathematics, and artificial intelligence andadds a heavy dose of data management to create a potent brew that many would rather not drink!They don’t know if predictive analytics is a legitimate business endeavor or an ivory tower scienceexperiment run wild.4TDWI RESE A RCH1For many years, TDWI recognized the top overall applicant to its Best Practices Awards program with the TDWILeadership Award for excellence in data warehousing and business intelligence. For more information on this program,visit www.tdwi.org/Education and click on Best Practices.
What Is Predictive Analytics?Where Do You Start? But once managers overcome their initial trepidation, they encounter anotherobstacle: how to apply predictive analytics optimally in their company. Most have only a vaguenotion about the business areas or applications that can benefit from predictive analytics. Second,most don’t know how to get started: whom to hire, how to organize the project, or how to architectthe environment.DefinitionsBefore we address those questions, it’s important to define what predictive analytics is and is not.Predictive analytics is a set of business intelligence (BI) technologies that uncovers relationships andpatterns within large volumes of data that can be used to predict behavior and events.2 Unlike otherBI technologies, predictive analytics is forward-looking, using past events to anticipate the future.(See Figure 2.)Applications. Predictive analytics can identify the customers most likely to churn next month orto respond to next week’s direct mail piece. It can also anticipate when factory floor machines arelikely to break down or figure out which customers are likely to default on a bank loan. Today,marketing is the biggest user of predictive analytics with cross-selling, campaign management,customer acquisition, and budgeting and forecasting models top of the list, followed by attritionand loyalty applications. (See Figure 3.)The Spectrum of BI TechnologiesFigure 2. Among business intelligence disciplines, prediction provides the most business value but is also the mostcomplex. Each discipline builds on the one below it—these are additive, not exclusive, in practice2 TDWI defines business intelligence as the tools, technologies, and processes required to turn data into information andinformation into knowledge and plans that optimize business actions. In short, business intelligence makes the businessesrun more intelligently. It encompasses data integration, data warehousing, and reporting and analysis tools. Colloquially,most people use the term “BI tools” to refer to reporting and OLAP tools, not the full spectrum of BI capabilities.www.tdwi.org5
PREDIC T I V E A N ALY T IC SApplications for Predictive AnalyticsFigure 3. Based on 167 respondents who have implemented predictive analytics. Respondents could select multipleanswers.Versus BI Tools. In contrast, other BI technologies—such as query and reporting tools, onlineanalytical processing (OLAP), dashboards, and scorecards—examine what happened in thepast. They are deductive in nature—that is, business users must have some sense of the patternsand relationships that exist within the data based on their personal experience. They use query,reporting, and OLAP tools to explore the data and validate their hypotheses. Dashboards andscorecards take deductive reasoning a step further: they present users with a de facto set ofhypotheses in the form of metrics and KPIs that users examine on a regular basis.Predictive analytics letsdata lead the way.Predictive analytics works the opposite way: it is inductive. It doesn’t presume anything about thedata. Rather, predictive analytics lets data lead the way. Predictive analytics employs statistics,machine learning, neural computing, robotics, computational mathematics, and artificialintelligence techniques to explore all the data, instead of a narrow subset of it, to ferret outmeaningful relationships and patterns. Predictive analytics is like an “intelligent” robot thatrummages through all your data until it finds something interesting to show you.No Silver Bullet. However, it’s important to note that predictive analytics is not a silver bullet.Practitioners have learned that most of the “intelligence” in these so-called decision automationsystems comes from humans who have a deep understanding of the business and know whereto point the tools, how to prepare the data, and how to interpret the results. Creating predictivemodels requires hard work, and the results are not guaranteed to provide any business value. Forexample, a model may predict that 75% of potential buyers of a new product are male, but if 75%of your existing customers are male, then this prediction doesn’t help the business. A marketingprogram targeting male shoppers will not yield any additional value or lift over a more generalizedmarketing program.Predictive analytics isstatistics on steroids.6TDWI RESE A RCHMore Than Statistics. It’s also important to note that predictive analytics is more than statistics.Some even call it statistics on steroids. Linear and logistic regressions—classic statistical
What Is Predictive Analytics?techniques—are still the workhorse of predictive models today, and nearly all analytical modelersuse descriptive statistics (e.g., mean, mode, median, standard deviation, histograms) to understandthe nature of the data they want to analyze.However, advances in computer processing power and database technology have made it possibleto employ a broader class of predictive techniques, such as decision trees, neural networks, geneticalgorithms, support vector machines, and other mathematical algorithms. These new techniquestake advantage of increased computing horsepower to perform complex calculations that oftenrequire multiple passes through the data. They are designed to run against large volumes of datawith lots of variables (i.e., fields or columns.) They also are equipped to handle “noisy” data withvarious anomalies that may wreak havoc on traditional models.Terminology. Predictive analytics has been around for a long time but has been known by othernames. For much of the past 10 years, most people in commercial industry have used the term“data mining” to describe the techniques and processes involved in creating predictive models.However, some software companies—in particular, OLAP vendors—began co-opting the term inthe late 1990s, claiming their tools allow users to “mine” nuggets of valuable information withindimensional databases. To stay above the fray, academics and researchers have used the term“knowledge discovery.”Predictive analyticsversus data mining.Today, the term data mining has been watered down so much that vendors and consultants nowembrace the term “predictive analytics” or “advanced analytics” or just “analytics” to describethe nature of the tools or services they offer. But even here the terminology can get fuzzy. Not allanalytics are predictive. In fact, there are two major types of predictive analytics, (1) supervisedlearning and (2) unsupervised learning.Training Models. Supervised learning is the process of creating predictive models using a set ofhistorical data that contains the results you are trying to predict. For example, if you want to predictwhich customers are likely to respond to a new direct mail campaign, you use the results of pastcampaigns to “train” a model to identify the characteristics of individuals who responded to thatcampaign. Supervised learning approaches include classification, regression, and time-series analysis.Classification techniques identify which group a new record belongs to (i.e., customer or event)based on its inherent characteristics. For example, classification is used to identify individuals ona mailing list that are likely to respond to an offer. Regression uses past values to predict futurevalues and is used in forecasting and variance analysis. Time-series analysis is similar to regressionanalysis but understands the unique properties of time and calendars and is used to predict seasonalvariances, among other things.Unsupervised Learning. In contrast, unsupervised learning does not use previously knownresults to train its models. Rather, it uses descriptive statistics to examine the natural patternsand relationships that occur within the data and does not predict a target value. For example,unsupervised learning techniques can identify clusters or groups of similar records within a database(i.e., clustering) or relationships among values in a database (i.e., association.) Market basketanalysis is a well-known example of an association technique, while customer segmentation is anexample of a clustering technique.Whether the business uses supervised or unsupervised learning, the result is an analytic model.Analysts build models using a variety of techniques, some of which we have already mentioned:neural networks, decision trees, linear and logistic regression, naive Bayes, clustering, association,www.tdwi.org7
PREDIC T I V E A N ALY T IC Sand so on. Each type of model can be implemented using a variety of algorithms with uniquecharacteristics that are suited to different types of data and problems. Part of the skill in creatingeffective analytic models is knowing which models and algorithms to use. Fortunately, manyleading analytic workbenches now automatically apply multiple models and algorithms to a problemto find the combination that works best. This advance alone has made it possible for non-specialiststo create fairly effective analytical models using today’s workbenches.The Business Value of Predictive AnalyticsOrganizations with a“strike-it-rich” mentalityare likely to get frustratedand give up.Incremental Improvement. Although organizations occasionally make multi-million dollardiscoveries using predictive analytics, these cases are the exception rather than the rule.Organizations that approach predictive analytics with a “strike-it-rich” mentality will likely becomefrustrated and give up before reaping any rewards. The reality is that predictive analytics providesincremental improvement to existing business processes, not million-dollar discoveries.“We achieve success in little percentages,” says a technical lead for a predictive analytics team in amajor telecommunications firm. She convinced her company several years ago to begin buildingpredictive models to identify customers who might cancel their wireless phone service. “Our modelshave contributed to lowering our churn rate, giving us a competitive advantage.”The company’s churn models expose insights about customer behavior that the business uses toimprove marketing or re-engineer business processes. For example, salespeople use model output tomake special offers to customers at risk of churning, and the managers to change licensing policiesthat may be affecting churn rates.Measuring ValueOur survey reinforces the business value of predictive analytics. Among respondents who haveimplemented predictive analytics, two-thirds (66%) say it provides “very high” or “high” businessvalue. A quarter (27%) claim it provides moderate value and only 4% admit it provides “low” or“very low” value. (See Figure 4.) 3What Is the Business Value of Predictive Analytics to Your Organization?Figure 4. Based on 166 respondents who have implemented predictive analytics.8TDWI RESE A RCH3 Our respondents are generally individuals who create predictive models or manage analytic teams, so their perceptions ofthe business value they provide may be biased or differ from what business executives or managers might say. Nonetheless, Ibelieve the responses generally align with my understanding of the success rates of predictive analytics in most organizationsand other research conducted by vendors and research providers like International Data Corp.
The Business Value of Predictive AnalyticsHow Do You Measure Success?Figure 5. Based on 110 users who have implemented predictive analytics initiatives that offer “very high” or “ high”value. Respondents could select multiple choices.Respondents who selected “very high” or “high” in Figure 4 say they measure the success of theirpredictive analytics efforts with several criteria, starting with “meets business goals” (mentioned by57% of respondents.) Other success criteria include “model accuracy” (56%), “ROI” (40%), “lift”(35%), and “adoption rate by business users” (34%.) (See Figure 5.)Minimizing Churn. Brian Siegel is vice president of marketing analytics at TN Marketing, a firmthat produces and distributes books and videos for its clients. He uses “lift” to measure the successof his predictive models. In a marketing campaign, lift measures the difference in customer responserates between customer lists created with and without a predictive model. As a one-man predictiveanalytics shop at his company, Siegel identifies people from client customer lists and outside listswho are likely to respond to a marketing campaign that his company conducts on behalf of a client.“We have some cases where we don’t need a whole lot of lift to achieve the ROI our president islooking for,” says Siegel, who further states that he is successful eight out of ten times in achievingthe response rates established by the marketing team. When he’s not successful, Siegel says it’susually because the data set is too small or responses too random to offer predictive value.Siegel is quick to translate the lift of his campaigns to business value. “Our response modelingefforts are worth millions,” he says. “There have been a number of occasions where we wouldnot have been able to acquire a new client and make the investment required to run a marketingcampaign without the lift provided by our predictive models. So, I’m part of the sales process.”Siegel is successfuleight out of ten times inachieving the desiredresponse rates.ROI. Interestingly, only a quarter of companies (24%) that have implemented predictive analyticshave conducted a formal ROI study. This is about average for most BI projects based on past TDWIresearch. Companies with high-value analytic programs that have calculated ROI invest on average 1.36 million and receive a payback within 11.2 months. (These results are based on responses fromonly 37 survey respondents.)The survey also asked respondents how much their group invests annually to support its predictiveanalytics practice, including hardware, software, staff, and services. The median investment is 600,000 for all respondents that have implemented predictive analytics, but 1 million forrespondents with programs delivering “very high” or “high” business value. These results suggestthat you get what you pay for. (See Table 1.)Companies withsuccessful analyticsprograms invest 1 millionannually.www.tdwi.org9
PREDIC T I V E A N ALY T IC SMedian Investments in Predictive AnalyticsIN V E S T ME N TAll Companies 600,000Companies with “high value programs” 1 millionTable 1. Companies whose predictive analytics practice delivers “very high” or “ high” business value (see Figure4) invest more money than companies whose programs deliver “moderate” or lower value. Based on 166 and 110respondents, respectively.Drilling down more, the survey asked respondents to report their investments in predictive analyticsby staff, software, hardware, and external services. Not surprisingly, staff costs consume the lion’sshare of expenses, followed by software and hardware. Organizations spend only 10% of their totalbudget on external service providers, either consultants or service bureaus. (See Figure 6.)Median Breakdown of Expenses on Predictive AnalyticsFigure 6. Median numbers are based on 166 respondents whose groups have implemented predictive analytics.How Do You Deliver Predictive Analytics?What Now? While some organizations have discovered the power of predictive analytics to reducecosts, increase revenues, and optimize business processes, the vast majority are still looking to getin the game. Today, most IT managers and some business managers understand the value thatpredictive analytics can bring, but most are perplexed about where to begin.“We are sitting on amountain of gold butwe’re not mining it aseffectively as we could.”“We are sitting on a mountain of gold but we’re not mining it as effectively as we could,” saysMichael Masciandaro, director of business intelligence at Rohm & Haas, a global specialty materialsmanufacturer. “We say we do analytics, but it’s really just reporting and OLAP.”Rohm & Haas has hired consultants before to build pricing models that analyze and solve specificproblems, but these models lose their usefulness once the consultants leave. Masciandaro saysbuilding an internal predictive analytics capability could yield tremendous insights and improve theprofitability of key business areas, but he struggles to understand how to make this happen.“How do you implement advanced analytics so they are not a one-off project done by an outsideconsultancy?” says Masciandaro. “How do you bring this functionality in house and use it to delivervalue every day? And where do you find people who can do this? There are not too many of themout there.”10TDWI RESE A RCH
How Do You Deliver Predictive Analytics?The Process of Predictive ModelingMethodologies. Although most experts agree that predictive analytics requires great skill—andsome go so far as to suggest that there is an artistic and highly creative side to creating models—most would never venture forth without a clear methodology to guide their work, whether explicitor implicit. In fact, process is so important in the predictive analytics community that in 1996several industry players created an industry standard methodology called the Cross IndustryStandard Process for Data Mining (CRISP-DM.) 4Most analytic modelersadhere to a methodologyto ensure success.CRISP-DM. Although only 15% of our survey respondents follow CRISP-DM, it embodies acommon-sense approach that is mirrored in other methodologies. (See Figure 7.) “Many people,including myself, adhere to CRISP-DM without knowing it,” says Tom Breur, principal of XLNTConsulting in the Netherlands. Keith Higdon, vice president and practice leader for businessintelligence at Sedgwick Claims Management Services, Inc. (CMS), adds, “CRISP-DM is a goodplace to start because it’s designed to be cross-industry. But then you have to think, ‘What makesmy world unique?’”What Methodology Does Your Group Use?Figure 7. Based on 167 respondents who have implemented predictive analytics.Regardless of methodology, most processes for creating predictive models incorporate the followingsteps:1. Project Definition: Define the business objectives and desired outcomes for the project andtranslate them into predictive analytic objectives and tasks.2. Exploration: Analyze source data to determine the most appropriate data and modelbuilding approach, and scope the effort.3. Data Preparation: Select, extract, and transform data upon which to create models.4. Model Building: Create, test, and validate models, and evaluate whether they will meetproject metrics and goals.5. Deployment: Apply model results to business decisions or processes. This ranges fromsharing insights with business users to embedding models into applications to automatedecisions and business processes.6. Model Management: Manage models to improve performance (i.e., accuracy), control access,promote reuse, standardize toolsets, and minimize redundant activities.Most experts say the data preparation phase of creating predictive models is the most timeconsuming part of the process, and our survey data agrees. On average, preparing the data4 The impetus for CRISP-DM came from NCR, Daimler Chrysler, and SPSS, who in 1997 formed an industry consortiumand obtained funding from the European Commission to establish an industry-, tool-, and application-neutral standardprocess for data mining. Today, an open special interest group of more t
Figure 1. Predictive analytics is still in an early-adopter phase. Based on 833 respondents to a TDWI survey conducted August 2006. Predictive analytics is also an arcane set of techniques and technologies that bewilder many business and IT managers. It stirs together statistics, advanced mathematics, and artificial intelligence and