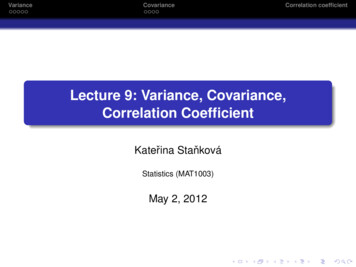
Transcription
VarianceCovarianceCorrelation coefficientLecture 9: Variance, Covariance,Correlation CoefficientKateřina StaňkováStatistics (MAT1003)May 2, 2012beamer-tu-log
VarianceCovarianceCorrelation coefficientOutline1VarianceDefinitionStandard DeviationVariance of linear combination of RV2CovarianceMeaning & DefinitionExamples3Correlation coefficientbook: Sections 4.2, 4.3beamer-tu-log
VarianceCovarianceCorrelation coefficientAnd now . . .1VarianceDefinitionStandard DeviationVariance of linear combination of RV2CovarianceMeaning & DefinitionExamples3Correlation coefficientbeamer-tu-log
VarianceCovarianceCorrelation coefficientDefinitionVarianceLet X be an RV with µx E(X ). Then the variance of X isgiven byV (X ) E{(X µX )2 }beamer-tu-log
VarianceCovarianceCorrelation coefficientDefinitionVarianceLet X be an RV with µx E(X ). Then the variance of X isgiven byV (X ) E{(X µX )2 }Notation: V (X ), Var(X ), σ 2 , σx2 {z }bookbeamer-tu-log
VarianceCovarianceCorrelation coefficientDefinitionVarianceLet X be an RV with µx E(X ). Then the variance of X isgiven byV (X ) E{(X µX )2 }Notation: V (X ), Var(X ), σ 2 , σx2 {z }bookAlternative formulaV (X ) E{(X µX )2 } E(X 2 2 µX X µ2X ) E(X 2 ) 2 µX E(X ) µ2X E(X 2 ) 2 µ2X µ2X E(X 2 ) µ2Xbeamer-tu-log
VarianceCovarianceCorrelation coefficientDefinitionVarianceLet X be an RV with µx E(X ). Then the variance of X isgiven byV (X ) E{(X µX )2 }Notation: V (X ), Var(X ), σ 2 , σx2 {z }bookAlternative formulaV (X ) E{(X µX )2 } E(X 2 2 µX X µ2X ) E(X 2 ) 2 µX E(X ) µ2X E(X 2 ) 2 µ2X µ2X E(X 2 ) µ2XObservationVariance is always nonnegative!beamer-tu-log
VarianceCovarianceCorrelation coefficientStandard DeviationStandard deviation σXspSquare root of V (X ), i.e., σX V (X ) E(X 2 ) µ2X{z} V (X )beamer-tu-log
VarianceCovarianceCorrelation coefficientStandard DeviationStandard deviation σXspSquare root of V (X ), i.e., σX V (X ) E(X 2 ) µ2X{z} V (X )Example 1X : ] eyes on a diebeamer-tu-log
VarianceCovarianceCorrelation coefficientStandard DeviationStandard deviation σXspSquare root of V (X ), i.e., σX V (X ) E(X 2 ) µ2X{z} V (X )Example 1X : ] eyes on a die2V (X ) E(X ) µ2X91 6 2735 212beamer-tu-log
VarianceCovarianceCorrelation coefficientStandard DeviationStandard deviation σXspSquare root of V (X ), i.e., σX V (X ) E(X 2 ) µ2X{z} V (X )Example 1X : ] eyes on a die2V (X ) E(X ) Standard deviation: σX µ2Xq91 6 2735 2123512beamer-tu-log
VarianceCovarianceCorrelation coefficientStandard DeviationStandard deviation σXspSquare root of V (X ), i.e., σX V (X ) E(X 2 ) µ2X{z} V (X )Example 1X : ] eyes on a die2V (X ) E(X ) Standard deviation: σX µ2Xq91 6 2735 2123512Example 2n : a real numberbeamer-tu-log,
VarianceCovarianceCorrelation coefficientStandard DeviationStandard deviation σXspSquare root of V (X ), i.e., σX V (X ) E(X 2 ) µ2X{z} V (X )Example 1X : ] eyes on a die2V (X ) E(X ) Standard deviation: σX µ2Xq91 6 2735 2123512Example 2n : a real numberbeamer-tu-logV (n) E(n2 ) µ2n n2 n2 0,
VarianceCovarianceCorrelation coefficientStandard DeviationStandard deviation σXspSquare root of V (X ), i.e., σX V (X ) E(X 2 ) µ2X{z} V (X )Example 1X : ] eyes on a die2V (X ) E(X ) Standard deviation: σX µ2Xq91 6 2735 2123512Example 2n : a real numberbeamer-tu-logV (n) E(n2 ) µ2n n2 n2 0,σn 0
VarianceCovarianceCorrelation coefficientStandard DeviationVariance & standard deviationpV (X ) E{(X µX )2 } E(X 2 ) µ2X , σX V (X )beamer-tu-log
VarianceCovarianceCorrelation coefficientStandard DeviationVariance & standard deviationpV (X ) E{(X µX )2 } E(X 2 ) µ2X , σX V (X )Example 3Let X be a continuous random variable with PDFg(x) 1010 4x x ,330 x 1(0 elsewhere)E(X ) E(X 2 ) V (X ) ,σX beamer-tu-log
VarianceCovarianceCorrelation coefficientStandard DeviationVariance & standard deviationpV (X ) E{(X µX )2 } E(X 2 ) µ2X , σX V (X )Example 3Let X be a continuous random variable with PDFg(x) 1010 4x x ,330 x 1(0 elsewhere)1ZE(X ) x g(x)dx0E(X 2 ) V (X ) ,σX beamer-tu-log
VarianceCovarianceCorrelation coefficientStandard DeviationVariance & standard deviationpV (X ) E{(X µX )2 } E(X 2 ) µ2X , σX V (X )Example 3Let X be a continuous random variable with PDFg(x) 1010 4x x ,331ZE(X ) 1Zx g(x)dx 0 x00 x 1(0 elsewhere) 1010 4x x dx332E(X ) V (X ) ,σX beamer-tu-log
VarianceCovarianceCorrelation coefficientStandard DeviationVariance & standard deviationpV (X ) E{(X µX )2 } E(X 2 ) µ2X , σX V (X )Example 3Let X be a continuous random variable with PDFg(x) 1010 4x x ,331ZE(X ) 1Zx g(x)dx 0 x00 x 1(0 elsewhere) 1010 45x x dx 3392E(X ) V (X ) ,σX beamer-tu-log
VarianceCovarianceCorrelation coefficientStandard DeviationVariance & standard deviationpV (X ) E{(X µX )2 } E(X 2 ) µ2X , σX V (X )Example 3Let X be a continuous random variable with PDFg(x) 1010 4x x ,331ZE(X ) 021Zx g(x)dx x01Z0 x 1(0 elsewhere) 1010 45x x dx 3392x g(x)dxE(X ) 0V (X ) ,σX beamer-tu-log
VarianceCovarianceCorrelation coefficientStandard DeviationVariance & standard deviationpV (X ) E{(X µX )2 } E(X 2 ) µ2X , σX V (X )Example 3Let X be a continuous random variable with PDFg(x) 1010 4x x ,33110 x 1(0 elsewhere) 1010 45x x dx 33900 Z 1Z 11010 4x 2 g(x)dx x2x x dxE(X 2 ) 3300ZE(X ) V (X ) Zx g(x)dx x,σX beamer-tu-log
VarianceCovarianceCorrelation coefficientStandard DeviationVariance & standard deviationpV (X ) E{(X µX )2 } E(X 2 ) µ2X , σX V (X )Example 3Let X be a continuous random variable with PDFg(x) 1010 4x x ,33110 x 1(0 elsewhere) 1010 45x x dx 33900 Z 1Z 11010 45x 2 g(x)dx x2x x dx E(X 2 ) 331400ZE(X ) V (X ) Zx g(x)dx x,σX beamer-tu-log
VarianceCovarianceCorrelation coefficientStandard DeviationVariance & standard deviationpV (X ) E{(X µX )2 } E(X 2 ) µ2X , σX V (X )Example 3Let X be a continuous random variable with PDFg(x) 1010 4x x ,33110 x 1(0 elsewhere) 1010 45x x dx 33900 Z 1Z 11010 45x 2 g(x)dx x2x x dx E(X 2 ) 331400ZE(X ) Zx g(x)dx V (X ) E(X 2 ) (E(X ))2 x,σX beamer-tu-log
VarianceCovarianceCorrelation coefficientStandard DeviationVariance & standard deviationpV (X ) E{(X µX )2 } E(X 2 ) µ2X , σX V (X )Example 3Let X be a continuous random variable with PDFg(x) 1010 4x x ,33110 x 1(0 elsewhere) 1010 45x x dx 33900 Z 1Z 11010 45x 2 g(x)dx x2x x dx E(X 2 ) 331400 255V (X ) E(X 2 ) (E(X ))2 , σX 149ZE(X ) Zx g(x)dx xbeamer-tu-log
VarianceCovarianceCorrelation coefficientStandard DeviationVariance & standard deviationpV (X ) E{(X µX )2 } E(X 2 ) µ2X , σX V (X )Example 3Let X be a continuous random variable with PDFg(x) 1010 4x x ,33110 x 1(0 elsewhere) 1010 45x x dx 33900 Z 1Z 11010 45x 2 g(x)dx x2x x dx E(X 2 ) 331400 25555V (X ) E(X 2 ) (E(X ))2 , σX 1491134ZE(X ) Zx g(x)dx xbeamer-tu-log
VarianceCovarianceCorrelation coefficientStandard DeviationVariance & standard deviationpV (X ) E{(X µX )2 } E(X 2 ) µ2X , σX V (X )Example 3Let X be a continuous random variable with PDFg(x) 1010 4x x ,33110 x 1(0 elsewhere) 1010 45x x dx 33900 Z 1Z 11010 45x 2 g(x)dx x2x x dx E(X 2 ) 331400r 2555555V (X ) E(X 2 ) (E(X ))2 , σX 14911341134ZE(X ) Zx g(x)dx xbeamer-tu-log
VarianceCovarianceCorrelation coefficientStandard DeviationVariance & standard deviationV (X ) E{(X µX )2 } E(X 2 ) µ2XpσX V (X )beamer-tu-log
VarianceCovarianceCorrelation coefficientStandard DeviationVariance & standard deviationV (X ) E{(X µX )2 } E(X 2 ) µ2XpσX V (X )Example 4Leth(y ) 5 y 4 ,0 y 1(0 elsewhere),beamer-tu-log
VarianceCovarianceCorrelation coefficientStandard DeviationVariance & standard deviationV (X ) E{(X µX )2 } E(X 2 ) µ2XpσX V (X )Example 4Leth(y ) 5 y 4 ,0 y 1(0 elsewhere)E(Y ) E(Y 2 ) V (Y ) , σY beamer-tu-log
VarianceCovarianceCorrelation coefficientStandard DeviationVariance & standard deviationV (X ) E{(X µX )2 } E(X 2 ) µ2XpσX V (X )Example 4Leth(y ) 5 y 4 ,Z0 y 1(0 elsewhere)1y h(y )dyE(Y ) 0E(Y 2 ) V (Y ) , σY beamer-tu-log
VarianceCovarianceCorrelation coefficientStandard DeviationVariance & standard deviationV (X ) E{(X µX )2 } E(X 2 ) µ2XpσX V (X )Example 4Leth(y ) 5 y 4 ,Z10 y 11Zy h(y )dy E(Y ) 0(0 elsewhere)5 y 5 dy0E(Y 2 ) V (Y ) , σY beamer-tu-log
VarianceCovarianceCorrelation coefficientStandard DeviationVariance & standard deviationV (X ) E{(X µX )2 } E(X 2 ) µ2XpσX V (X )Example 4Leth(y ) 5 y 4 ,Z10 y 11Zy h(y )dy E(Y ) 00(0 elsewhere)5 y 5 dy 5 6y6 1y 0E(Y 2 ) V (Y ) , σY beamer-tu-log
VarianceCovarianceCorrelation coefficientStandard DeviationVariance & standard deviationV (X ) E{(X µX )2 } E(X 2 ) µ2XpσX V (X )Example 4Leth(y ) 5 y 4 ,Z10 y 11Zy h(y )dy E(Y ) 00(0 elsewhere)5 y 5 dy 5 6y6 1 y 056E(Y 2 ) V (Y ) , σY beamer-tu-log
VarianceCovarianceCorrelation coefficientStandard DeviationVariance & standard deviationV (X ) E{(X µX )2 } E(X 2 ) µ2XpσX V (X )Example 4Leth(y ) 5 y 4 ,Z11Zy h(y )dy E(Y ) 0E(Y 2 ) 0 y 1Z01(0 elsewhere)5 y 5 dy 5 6y6 1 y 056y 2 h(y )dy0V (Y ) , σY beamer-tu-log
VarianceCovarianceCorrelation coefficientStandard DeviationVariance & standard deviationV (X ) E{(X µX )2 } E(X 2 ) µ2XpσX V (X )Example 4Leth(y ) 5 y 4 ,Z10 y 10E(Y 2 ) Z0V (Y ) 1Zy h(y )dy E(Y ) 5 y 5 dy 01y 2 h(y )dy 1Z(0 elsewhere) 5 6y6 1 y 0565 y 6 dy0, σY beamer-tu-log
VarianceCovarianceCorrelation coefficientStandard DeviationVariance & standard deviationV (X ) E{(X µX )2 } E(X 2 ) µ2XpσX V (X )Example 4Leth(y ) 5 y 4 ,Z10 y 10E(Y 2 ) Z0V (Y ) 1Zy h(y )dy E(Y ) (0 elsewhere)5 y 5 dy 01y 2 h(y )dy 1Z05 y 6 dy 5 6y6 1 y 05657, σY beamer-tu-log
VarianceCovarianceCorrelation coefficientStandard DeviationVariance & standard deviationV (X ) E{(X µX )2 } E(X 2 ) µ2XpσX V (X )Example 4Leth(y ) 5 y 4 ,Z10 y 10E(Y 2 ) 1Zy h(y )dy E(Y ) Z(0 elsewhere)5 y 5 dy 01y 2 h(y )dy 1Z0V (Y ) E(Y 2 ) (E(Y ))205 y 6 dy 5 6y6 1 y 05657, σY beamer-tu-log
VarianceCovarianceCorrelation coefficientStandard DeviationVariance & standard deviationV (X ) E{(X µX )2 } E(X 2 ) µ2XpσX V (X )Example 4Leth(y ) 5 y 4 ,Z10 y 10E(Y 2 ) 1Zy h(y )dy E(Y ) Z0(0 elsewhere)5 y 5 dy 01y 2 h(y )dy 1Z5 y 6 dy 0V (Y ) E(Y 2 ) (E(Y ))2 5 75 6y6 1 y 05657 256, σY beamer-tu-log
VarianceCovarianceCorrelation coefficientStandard DeviationVariance & standard deviationV (X ) E{(X µX )2 } E(X 2 ) µ2XpσX V (X )Example 4Leth(y ) 5 y 4 ,Z10 y 10E(Y 2 ) 1Zy h(y )dy E(Y ) Z0(0 elsewhere)5 y 5 dy 01y 2 h(y )dy 1Z5 y 6 dy 0V (Y ) E(Y 2 ) (E(Y ))2 5 75 6y6 1 y 05657 255 , σY 6252beamer-tu-log
VarianceCovarianceCorrelation coefficientStandard DeviationVariance & standard deviationV (X ) E{(X µX )2 } E(X 2 ) µ2XpσX V (X )Example 4Leth(y ) 5 y 4 ,Z10 y 10E(Y 2 ) 1Zy h(y )dy E(Y ) Z(0 elsewhere)5 y 5 dy 01y 2 h(y )dy 1Z05 y 6 dy 05V (Y ) E(Y ) (E(Y )) 7225 6y6 1 y 05657r 2555 , σY 6252252beamer-tu-log
VarianceCovarianceCorrelation coefficientVariance of linear combination of RVTheorem about V (a X b)beamer-tu-log
VarianceCovarianceCorrelation coefficientVariance of linear combination of RVTheorem about V (a X b)Let X be an RV with variance V (X ). Then:V (a X b) a2 V (X )beamer-tu-log
VarianceCovarianceCorrelation coefficientVariance of linear combination of RVTheorem about V (a X b)Let X be an RV with variance V (X ). Then:V (a X b) a2 V (X )Proof:beamer-tu-log
VarianceCovarianceCorrelation coefficientVariance of linear combination of RVTheorem about V (a X b)Let X be an RV with variance V (X ). Then:V (a X b) a2 V (X )Proof:V (a X b) E{(a X b µaX b )2 }beamer-tu-log
VarianceCovarianceCorrelation coefficientVariance of linear combination of RVTheorem about V (a X b)Let X be an RV with variance V (X ). Then:V (a X b) a2 V (X )Proof:V (a X b) E{(a X b µaX b )2 } E{(a X b (aµX b))2 }beamer-tu-log
VarianceCovarianceCorrelation coefficientVariance of linear combination of RVTheorem about V (a X b)Let X be an RV with variance V (X ). Then:V (a X b) a2 V (X )Proof:V (a X b) E{(a X b µaX b )2 } E{(a X b (aµX b))2 } E{(a X a µX )2 }beamer-tu-log
VarianceCovarianceCorrelation coefficientVariance of linear combination of RVTheorem about V (a X b)Let X be an RV with variance V (X ). Then:V (a X b) a2 V (X )Proof:V (a X b) E{(a X b µaX b )2 } E{(a X b (aµX b))2 } E{(a X a µX )2 } E{a2 (X µX )2 }beamer-tu-log
VarianceCovarianceCorrelation coefficientVariance of linear combination of RVTheorem about V (a X b)Let X be an RV with variance V (X ). Then:V (a X b) a2 V (X )Proof:V (a X b) E{(a X b µaX b )2 } E{(a X b (aµX b))2 } E{(a X a µX )2 } E{a2 (X µX )2 } a2 E{(X µX )2 }beamer-tu-log
VarianceCovarianceCorrelation coefficientVariance of linear combination of RVTheorem about V (a X b)Let X be an RV with variance V (X ). Then:V (a X b) a2 V (X )Proof:V (a X b) E{(a X b µaX b )2 } E{(a X b (aµX b))2 } E{(a X a µX )2 } E{a2 (X µX )2 } a2 E{(X µX )2 } a2 V (X )beamer-tu-log
VarianceCovarianceCorrelation coefficientVariance of linear combination of RVTheorem about V (a X b)Let X be an RV with variance V (X ). Then:V (a X b) a2 V (X )Proof:V (a X b) E{(a X b µaX b )2 } E{(a X b (aµX b))2 } E{(a X a µX )2 } E{a2 (X µX )2 } a2 E{(X µX )2 } a2 V (X ) beamer-tu-log
VarianceCovarianceCorrelation coefficientAnd now . . .1VarianceDefinitionStandard DeviationVariance of linear combination of RV2CovarianceMeaning & DefinitionExamples3Correlation coefficientbeamer-tu-log
VarianceCovarianceCorrelation coefficientMeaning & DefinitionWhat is covariance?Dependence of realizations of 2 (or more) different RVs.beamer-tu-log
VarianceCovarianceCorrelation coefficientMeaning & DefinitionWhat is covariance?Dependence of realizations of 2 (or more) different RVs.DefinitionLet X and Y be RVs with µX E(X ) and µY E(Y ). ThenCov(X , Y ) E{(X µX )(Y µY )}Notation: Cov(X , Y ), σX ,Y {z}bookbeamer-tu-log
VarianceCovarianceCorrelation coefficientMeaning & DefinitionWhat is covariance?Dependence of realizations of 2 (or more) different RVs.DefinitionLet X and Y be RVs with µX E(X ) and µY E(Y ). ThenCov(X , Y ) E{(X µX )(Y µY )}Notation: Cov(X , Y ), σX ,Y {z}bookRemarkCovariance can be positive and negative (variance is alwaysnonnegative)beamer-tu-log
VarianceCovarianceCorrelation coefficientMeaning & DefinitionDefinitionCov(X , Y ) E{(X µX )(Y µY )}Notation: Cov(X , Y ), σX ,Y {z}bookbeamer-tu-log
VarianceCovarianceCorrelation coefficientMeaning & DefinitionDefinitionCov(X , Y ) E{(X µX )(Y µY )}Notation: Cov(X , Y ), σX ,Y {z}bookAlternative formulabeamer-tu-log
VarianceCovarianceCorrelation coefficientMeaning & DefinitionDefinitionCov(X , Y ) E{(X µX )(Y µY )}Notation: Cov(X , Y ), σX ,Y {z}bookAlternative formulaCov(X , Y ) E{(X µX )(Y µY )} E (XY µY · X µX · Y µX µY ) E(XY ) µY · µX µX · µY µX µY E(XY ) µX µYbeamer-tu-log
VarianceCovarianceCorrelation coefficientExamplesCovarianceLet X and Y be RVs with µX E(X ) and µY E(Y ). ThenCov(X , Y ) E{(X µX )(Y µY )} E(XY ) µX µYNotation: Cov(X , Y ), σX ,Y {z}bookbeamer-tu-log
VarianceCovarianceCorrelation coefficientExamplesCovarianceLet X and Y be RVs with µX E(X ) and µY E(Y ). ThenCov(X , Y ) E{(X µX )(Y µY )} E(XY ) µX µYNotation: Cov(X , Y ), σX ,Y {z}bookExample 1X , Y independent RVsbeamer-tu-log
VarianceCovarianceCorrelation coefficientExamplesCovarianceLet X and Y be RVs with µX E(X ) and µY E(Y ). ThenCov(X , Y ) E{(X µX )(Y µY )} E(XY ) µX µYNotation: Cov(X , Y ), σX ,Y {z}bookExample 1X , Y independent RVsCov(X , Y )beamer-tu-log
VarianceCovarianceCorrelation coefficientExamplesCovarianceLet X and Y be RVs with µX E(X ) and µY E(Y ). ThenCov(X , Y ) E{(X µX )(Y µY )} E(XY ) µX µYNotation: Cov(X , Y ), σX ,Y {z}bookExample 1X , Y independent RVsCov(X , Y ) E(XY ) µX µYbeamer-tu-log
VarianceCovarianceCorrelation coefficientExamplesCovarianceLet X and Y be RVs with µX E(X ) and µY E(Y ). ThenCov(X , Y ) E{(X µX )(Y µY )} E(XY ) µX µYNotation: Cov(X , Y ), σX ,Y {z}bookExample 1X , Y independent RVsCov(X , Y ) E(XY ) µX µY E(X ) · E(Y ) µX · µYbeamer-tu-log
VarianceCovarianceCorrelation coefficientExamplesCovarianceLet X and Y be RVs with µX E(X ) and µY E(Y ). ThenCov(X , Y ) E{(X µX )(Y µY )} E(XY ) µX µYNotation: Cov(X , Y ), σX ,Y {z}bookExample 1X , Y independent RVsCov(X , Y ) E(XY ) µX µY E(X ) · E(Y ) µX · µY 0beamer-tu-log
VarianceCovarianceCorrelation coefficientExamplesCovarianceCov(X , Y ) E{(X µX )(Y µY )} E(XY ) µX µYbeamer-tu-log
VarianceCovarianceCorrelation coefficientExamplesCovarianceCov(X , Y ) E{(X µX )(Y µY )} E(XY ) µX µYExample 2X , Y independent RVs with joint PDFbeamer-tu-log
VarianceCovarianceCorrelation coefficientExamplesCovarianceCov(X , Y ) E{(X µX )(Y µY )} E(XY ) µX µYExample 2X , Y independent RVs with joint PDF 10x y 2 , 0 x y 1f (x, y ) .0, elswhereFind Cov(X , Y ), E(X , Y )beamer-tu-log
VarianceCovarianceCorrelation coefficientExamplesCovarianceCov(X , Y ) E{(X µX )(Y µY )} E(XY ) µX µYExample 2X , Y independent RVs with joint PDF 10x y 2 , 0 x y 1f (x, y ) .0, elswhereFind Cov(X , Y ), E(X , Y )1010 4x x , (0 x 1) (0 elsewhere)33h(y ) 5 y 4 , (0 y 1) (0 elsewhere)g(x) beamer-tu-log
VarianceCovarianceCorrelation coefficientExamplesCovarianceCov(X , Y ) E{(X µX )(Y µY )} E(XY ) µX µYExample 2X , Y independent RVs with joint PDF 10x y 2 , 0 x y 1f (x, y ) .0, elswhereFind Cov(X , Y ), E(X , Y )1010 4x x , (0 x 1) (0 elsewhere)33h(y ) 5 y 4 , (0 y 1) (0 elsewhere)g(x) E(X ) 59 , E(X 2 ) 5,14V (X ) 55,1134E(Y ) 56 , E(Y 2 ) 75 , V (Y ) 5252beamer-tu-log
VarianceCovarianceCorrelation coefficientExamplesCovarianceCov(X , Y ) E{(X µX )(Y µY )} E(XY ) µX µYExample 2X , Y independent RVs with joint PDF 10x y 2 , 0 x y 1f (x, y ) .0, elswhereFind Cov(X , Y ), E(X , Y )1010 4x x , (0 x 1) (0 elsewhere)33h(y ) 5 y 4 , (0 y 1) (0 elsewhere)g(x) 555E(X ) 59 , E(X 2 ) 14, V (X ) 1134, E(Y ) 56 , E(Y 2 ) 75 , V (Y ) R1R12E(X , Y ) 0 x x · y · 10 x y dy dx 10215252beamer-tu-log
VarianceCovarianceCorrelation coefficientExamplesCovarianceCov(X , Y ) E{(X µX )(Y µY )} E(XY ) µX µYExample 2X , Y independent RVs with joint PDF 10x y 2 , 0 x y 1f (x, y ) .0, elswhereFind Cov(X , Y ), E(X , Y )1010 4x x , (0 x 1) (0 elsewhere)33h(y ) 5 y 4 , (0 y 1) (0 elsewhere)g(x) 555E(X ) 59 , E(X 2 ) 14, V (X ) 1134, E(Y ) 56 , E(Y 2 ) 75 , V (Y ) R1R12E(X , Y ) 0 x x · y · 10 x y dy dx 1021105Cov(X , Y ) E(XY ) µX µY 21 59 56 3785252beamer-tu-log
VarianceCovarianceCorrelation coefficientAnd now . . .1VarianceDefinitionStandard DeviationVariance of linear combination of RV2CovarianceMeaning & DefinitionExamples3Correlation coefficientbeamer-tu-log
VarianceCovarianceCorrelation coefficientDefinition of correlation coefficient ρ(X , Y )The correlation coefficient of 2 RVs X and Y is defined asfollows:cov(X , Y )ρ(X , Y ) σX · σYbeamer-tu-log
VarianceCovarianceCorrelation coefficientDefinition of correlation coefficient ρ(X , Y )The correlation coefficient of 2 RVs X and Y is defined asfollows:cov(X , Y )ρ(X , Y ) σX · σYObservations 1 ρ(X , Y ) 1beamer-tu-log
VarianceCovarianceCorrelation coefficientDefinition of correlation coefficient ρ(X , Y )The correlation coefficient of 2 RVs X and Y is defined asfollows:cov(X , Y )ρ(X , Y ) σX · σYObservations 1 ρ(X , Y ) 1If X and Y are independent, then ρ(X , Y ) 0.beamer-tu-log
VarianceCovarianceCorrelation coefficientDefinition of correlation coefficient ρ(X , Y )The correlation coefficient of 2 RVs X and Y is defined asfollows:cov(X , Y )ρ(X , Y ) σX · σYObservations 1 ρ(X , Y ) 1If X and Y are independent, then ρ(X , Y ) 0.In the previous exampleqp55σX V (X ) 1134,q5378 ,Cov(X , Y ) pσY V (Y ) 5252beamer-tu-log
VarianceCovarianceCorrelation coefficientDefinition of correlation coefficient ρ(X , Y )The correlation coefficient of 2 RVs X and Y is defined asfollows:cov(X , Y )ρ(X , Y ) σX · σYObservations 1 ρ(X , Y ) 1If X and Y are independent, then ρ(X , Y ) 0.In the previous exampleqp55σX V (X ) 1134,q5378 ,Cov(X , Y ) pσY V (Y ) ρ(X , Y ) q52525378q555· 2521134 0.4264beamer-tu-log
beamer-tu-logo Variance CovarianceCorrelation coefficient Lecture 9: Variance, Covariance, Correlation Coefficient Kateˇrina Sta nkovᡠStatistics (MAT1003)