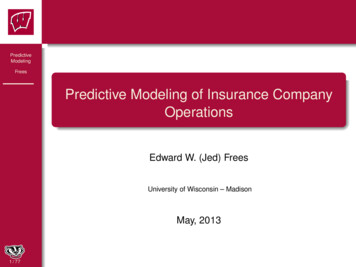
Transcription
PredictiveModelingFreesPredictive Modeling of Insurance CompanyOperationsEdward W. (Jed) FreesUniversity of Wisconsin – MadisonMay, 20131 / 77
OutlinePredictiveModelingFrees1Predictive Modeling2Two-Part Models3Multivariate Regression4Multivariate Two-Part Model5Gini IndexMEPS Model Validation62 / 77Concluding Remarks
An Actuary Is .PredictiveModelingFreesPredictiveModelingTwo-Part ModelsMultivariateRegressionMultivariateTwo-Part ModelGini IndexMEPS ValidationConcludingRemarks3 / 77
Predictive wo-Part ModelsMultivariateRegressionMultivariateTwo-Part ModelGini IndexMEPS ValidationConcludingRemarks4 / 77Predictive analyticsis an area of statistical analysis that deals withextracting information from data andusing it to predict future trends and behavior patterns.relies on capturing relationships between explanatoryvariables and the predicted variables from past occurrences,and exploiting it to predict future outcomes.is used in financial services, insurance, telecommunications,retail, travel, healthcare, pharmaceuticals and other fields.
Predictive Modeling and InsurancePredictiveModelingFreesInitial UnderwritingPredictiveModelingTwo-Part ModelsMultivariateRegressionMultivariateTwo-Part ModelGini IndexMEPS ValidationConcludingRemarksOffer right price for the right riskAvoid adverse selectionRenewal Underwriting/Portfolio ManagementRetain profitable customers longerClaims ManagementManage claims costsDetect and prevent claims fraudUnderstand excess layers for reinsurance and retentionReservingProvide management with an appropriate estimate of futureobligationsQuantify the uncertainty of the estimates5 / 77
Business Analytics and Two-Part ModelsMultivariateRegressionMultivariateTwo-Part ModelGini IndexMEPS ValidationConcludingRemarksSales and MarketingPredict customer behavior and needs, anticipate customerreactions to promotionsReduce acquisition costs (direct mail, discount programs)Compensation AnalysisIncent and reward employee/agent behavior appropriatelyProductivity AnalysisAnalyze production of employees, other units of businessSeek to optimize productionFinancial Forecasting6 / 77
Predictive -Part ModelsMultivariateRegressionMultivariateTwo-Part ModelGini IndexMEPS ValidationConcludingRemarks7 / 77Here are some useful skills/topicsTwo-Part? For example, loss or no lossLoss distributions are typically skewed and heavy-tailed
Predictive -Part ModelsMultivariateRegressionMultivariateTwo-Part ModelGini IndexMEPS ValidationConcludingRemarksHere are some useful skills/topicsTwo-Part? For example, loss or no lossLoss distributions are typically skewed and heavy-tailedCensored?Losses censored by amounts through deductibles or policylimitsLoss censored by time, e.g., claim trianglesInsurance data typically has lots of explanatory variables.Lots.7 / 77
Predictive Modeling and gTwo-Part ModelsMultivariateRegressionMultivariateTwo-Part ModelGini IndexMEPS ValidationConcludingRemarksI think about predictive modeling as a subset of businessanalytics, although many use the terms interchangeablyFor some, predictive modeling means advanced data-miningtools as per Hastie, Tibshirani and Friedman (2001). TheElements of Statistical Learning: Data Mining, Inference andPrediction.These tools include neural networks, classification trees,nonparametric regression and so forthOthers think about the traditional triad of statistical inference:EstimationHypothesis TestingPredictionI fall in this latter camp8 / 77
Some ngTwo-Part ModelsMultivariateRegressionMultivariateTwo-Part ModelGini IndexMEPS ValidationConcludingRemarks9 / 77See my latest book: Frees (2010), Regression Modeling withActuarial and Financial Applications, Cambridge UniversityPress.As indicated by the title, the focus here is on regression
Thanks to .PredictiveModelingFreesPredictiveModelingTwo-Part ModelsMultivariateRegressionMultivariateTwo-Part ModelGini IndexMEPS ValidationConcludingRemarks10 / 77Collaborators:Emiliano Valdez, Katrien Antonio, Margie RosenbergPeng Shi, Yunjie (Winnie) SunGlenn Meyers, A. David CummingsXipei Yang, Zhengjun Zhang, Xiaoli Jin, Xiao (Joyce) Lin
Motivating Two-Part -Part ModelsMultivariateRegressionMultivariateTwo-Part ModelGini IndexMEPS ValidationConcludingRemarks11 / 77Insurance and healthcare data often feature a largeproportion of zeros, where zero values can represent:Individual’s lack of utilizationNo expenditure (e.g., no claim)Non-participation in a program
Motivating Two-Part -Part ModelsMultivariateRegressionMultivariateTwo-Part ModelGini IndexMEPS ValidationConcludingRemarks11 / 77Insurance and healthcare data often feature a largeproportion of zeros, where zero values can represent:Individual’s lack of utilizationNo expenditure (e.g., no claim)Non-participation in a programHow to model zero expenditures?Ignore their existenceThrow them out and condition that usage is greater than zeroDo something else
Two-Part -Part ModelsMultivariateRegressionMultivariateTwo-Part ModelGini IndexMEPS ValidationConcludingRemarks12 / 77Economists use the term ’two-part models’ (First part whether zero, or 0; Second part Amount)Actuaries refer to these as frequency and severity models andintroduced in Bowers et al. (Chapter 2)Let ri 1, if claim, 0 otherwiseyi amount of the claim.(Claim recorded)i ri yiTwo-part models include covariates in each part.
Two-Part -Part ModelsMultivariateRegressionMultivariateTwo-Part ModelGini IndexMEPS ValidationConcludingRemarksEconomists use the term ’two-part models’ (First part whether zero, or 0; Second part Amount)Actuaries refer to these as frequency and severity models andintroduced in Bowers et al. (Chapter 2)Let ri 1, if claim, 0 otherwiseyi amount of the claim.(Claim recorded)i ri yiTwo-part models include covariates in each part.I will use data from the Medical Expenditure Panel Survey(MEPS) to illustrate a few ideasy Medical Expenditure, many x’s to explain/predict12 / 77
Inpatient Expenditures Summary aphyAGEAge in years between 18 to 65 (mean: 39.0)GENDER1 if female52.7GENDER1 if male47.3ASIAN1 if Asian4.3BLACK1 if Black14.8NATIVE1 if Native1.1WHITEReference level79.9NORTHEAST1 if Northeast14.3MIDWEST1 if Midwest19.7SOUTH1 if South38.2WESTReference level27.91 if college or higher degree27.2COLLEGEHIGHSCHOOL 1 if high school degree43.3Reference level is lower than high school degree29.5POOR1 if poor3.8FAIR1 if fair9.9GOOD1 if good29.9VGOOD1 if very good31.1Reference level is excellent health25.4MNHPOOR1 if poor or fair7.50 if good to excellent mental health92.5ANYLIMIT1 if any functional or activity limitation22.30 if otherwise77.7HINCOME1 if high income31.6MINCOME1 if middle income29.9LINCOME1 if low income15.8NPOOR1 if near poor5.8Reference level is poor/negative17.0INSURE1 if covered by public or private health77.8insurance in any month of 20030 if have not health insurance in nTwo-Part wo-Part ModelSelf-ratedphysical healthGini IndexMEPS ValidationConcludingRemarksSelf-ratedmental healthAny activitylimitationIncome comparedto poverty lineInsurancecoverageTotalDescriptionPercentof dataMEPS Data: Random sample of 2,000 individuals aged 18 - 64 from first panel in 2003.13 / 77AverageExpendPercentPositiveExpendAverageof 68.738.758.878.198.188.680.230.673.17.97.438.32
Inpatient Expenditures Summary gCategoryVariableDemographyAGEGENDERGENDERTwo-Part ModelsMultivariateRegressionMultivariateTwo-Part ModelGini IndexTotalDescriptionPercentof dataAverageExpendPercentPositiveExpendAge in years between 18 to 65 (mean: 39.0)1 if female52.70.9110.71 if male47.30.404.7100.00.677.9Averageof PosExpend8.538.668.32MEPS ValidationConcludingRemarks14 / 77MEPS Data: Random sample of 2,000 individuals aged 18 - 64 from first panel in 2003.
Bias Due to Limited Dependent VariablesPredictiveModelingEither excluding or ignoring zeros induces a biasLeft-hand panel: When individuals do have health expenditures,they are recorded as y 0 expenditures. (Censored)Right-hand panel: If the responses below the horizontal line aty d are omitted, then the fitted regression line is very differentfrom the true regression line. (Truncated)FreesPredictiveModelingTwo-Part ModelsMultivariateRegressionMultivariateTwo-Part ModelTRUELINEGini IndexMEPS ValidationConcludingRemarksyFITTEDLINEyd0x15 / 77x
Tobit ModelPredictiveModelingFreesPredictiveModelingHow do we estimate model parameters?Use maximum likelihood. Standard calculations show ln L as:Two-Part ModelsMultivariateRegressionMultivariateTwo-Part ModelGini IndexMEPS ValidationConcludingRemarksln L 0xi β diln1 Φ σi:yi di)(1(yi (x0i β di ))22 ln 2πσ 2 i:yi diσ2where {i : yi di } Sum of censored observationsand {i : yi di } Sum over non-censored observations.16 / 77
Definition of Two-Part -Part ModelsDenote the corresponding set of regression coefficients as β1 .Typical models include the linear probability, logit and -Part Model2Gini IndexMEPS ValidationConcludingRemarksUse a binary regression model with ri as the dependentvariable and x1i as the set of explanatory variables.Conditional on ri 1, specify a regression model with yi asthe dependent variable and x2i as the set of explanatoryvariables.Denote the corresponding set of regression coefficients as β2 .Typical models include the linear regression and gammaregression models.17 / 77
Full and Reduced Two-Part ModelsFull Part ModelsMultivariateRegressionMultivariateTwo-Part ModelGini IndexMEPS ValidationConcludingRemarks18 / UREScale σ 1.6541.8120.1613.944Reduced 245-0.6401.0521.3971.3334.195
Two-Part Part ModelsMultivariateRegressionMultivariateTwo-Part ModelGini IndexMEPS ValidationConcludingRemarks The outcome of interest is y 0y r 0r 1r indicates if a claim has occurred and,conditional on claim occurrence (r 1), y is the claimamount.Part 1. The distribution of r can be written as Fr (θ r ), where theparameter vector depends on explanatory variables θ r θ r (x).Part 2. Similarly, the distribution of y can be written as Fy (θ y ),where θ y θ y (x).When θ r and θ y are functionally independent, we canoptimize each part in isolation of one another and thus, treatthe likelihood process in “two parts.”19 / 77
Alternatives to the Two-Part Part ModelsMultivariateRegressionMultivariateTwo-Part ModelGini IndexMEPS ValidationConcludingRemarks20 / 77Tobit model. A related model used extensively ineconometrics, where y max(0, y ). This is a censoredregression model.The tobit regression model typically assumes normality. Incontrast, the two-part model retains flexibility in the specificationof the amount distribution.
Alternatives to the Two-Part Part ModelsMultivariateRegressionMultivariateTwo-Part ModelGini IndexMEPS ValidationConcludingRemarks20 / 77Tobit model. A related model used extensively ineconometrics, where y max(0, y ). This is a censoredregression model.The tobit regression model typically assumes normality. Incontrast, the two-part model retains flexibility in the specificationof the amount distribution.Tweedie GLM. Compared to the two-part model, a strength ofthe Tweedie approach is that both parts are estimatedsimultaneously; this means fewer parameters, making thevariable selection process simpler.The Tweedie distribution is a Poisson sum of gamma randomvariables.Thus, it has a mass at zero as well as a continuous component.It is used to model “pure premiums,” where the zeroscorrespond to no claims and the positive part is used for theclaim amount.
Aggregate Loss Part ModelsMultivariateRegressionMultivariateTwo-Part ModelGini IndexMEPS ValidationConcludingRemarks21 / 77For an aggregate loss model, we observe y (N, SN ) and xN describes the number of claimsSN is the aggregate claim amount.As with the two-part model, we separate the count (N) andseverity portions (SN ).Alternatively, we may observe (N, y 1 , . . . , y N , x).y j describes the claim amount for each event/episode.SN y 1 · · · y N is the aggregate claim amount.
Predictive ModelsPredictiveModelingHere are some skills/topics useful in predictive modelingFreesPredictiveModelingTwo-Part ModelsMultivariateRegressionMultivariateTwo-Part ModelGini IndexMEPS ValidationConcludingRemarks22 / 77Two-Part? For example, loss or no lossLoss distributions are typically skewed and heavy-tailedCensored?Losses censored by amounts through deductibles or policylimitsLoss censored by time, e.g., claim trianglesInsurance data typically has lots of explanatory variables.Lots.
Predictive ModelsPredictiveModelingHere are some skills/topics useful in predictive modelingFreesPredictiveModelingTwo-Part ModelsMultivariateRegressionMultivariateTwo-Part ModelGini IndexMEPS ValidationConcludingRemarks22 / 77Two-Part? For example, loss or no lossLoss distributions are typically skewed and heavy-tailedCensored?Losses censored by amounts through deductibles or policylimitsLoss censored by time, e.g., claim trianglesInsurance data typically has lots of explanatory variables.Lots.Multivariate responses, e.g., types of coverages, perils,bundling of insurancesLongitudinal (panel)? Are you following the contract overtime?Losses credible? we often wish to incorporate externalknowledge into our analysis
Advertising 2PredictiveModelingFreesPredictiveModelingTwo-Part ModelsMultivariateRegressionMultivariateTwo-Part ModelGini IndexMEPS ValidationConcludingRemarks23 / 77See, for example, my 2004 book.
Multivariate gTwo-Part ModelsMultivariateRegressionMultivariateTwo-Part ModelGini IndexMEPS ValidationConcludingRemarks24 / 77In multivariate analysis, there are several outcomes of interest(multivariate), yWith regression, there are several variables available toexplain/predict these outcomes, xMultivariate regression provides the foundation for severalstatistical methodologies.Structural Equations Modeling (SEM)Longitudinal Data ModelingHierarchical Linear Modeling
Multivariate RegressionPredictiveModelingFrees y1 Now suppose the outcome of interest is y . .ypPredictiveModelingTwo-Part ModelsMultivariateRegressionMultivariateTwo-Part ModelGini IndexMEPS ValidationConcludingRemarks25 / 77Use the notation Fj (θ j ) for the distribution function of yj ,j 1, . . . , p.
Multivariate RegressionPredictiveModelingFrees y1 Now suppose the outcome of interest is y . .ypPredictiveModelingTwo-Part ModelsMultivariateRegressionMultivariateTwo-Part ModelGini IndexUse the notation Fj (θ j ) for the distribution function of yj ,j 1, . . . , p.The joint distribution function can be expressed using acopula C asF C(F1 , . . . , Fp ).MEPS ValidationConcludingRemarks25 / 77The set of parameters is θ θ 1, . . . , θ p, α ,where α is the set of parameters associated with the copula C.
Multivariate RegressionPredictiveModelingFrees y1 Now suppose the outcome of interest is y . .ypPredictiveModelingTwo-Part ModelsMultivariateRegressionMultivariateTwo-Part ModelGini IndexUse the notation Fj (θ j ) for the distribution function of yj ,j 1, . . . , p.The joint distribution function can be expressed using acopula C asF C(F1 , . . . , Fp ).MEPS ValidationConcludingRemarks25 / 77The set of parameters is θ θ 1, . . . , θ p, α ,where α is the set of parameters associated with the copula C.Copula functions work particularly well with continuousvariables. There is less evidence about their utility for fittingdiscrete outcomes (or mixtures).It is customary, although not necessary, to let θ j depend onexplanatory variables x and to use constant α.
Student ngTwo-Part ModelsMultivariateRegressionMultivariateTwo-Part ModelGini IndexMEPS ValidationConcludingRemarksWe now have a way to assess the joint distribution of thedependent variables by (1) specifying the marginal distributionsand (2) the copulaConsider a regression context of student assessmentData from the 1988 NELS. We consider a random sample ofn 1, 000 studentsY1 math scoreY2 science scoreY3 reading scoreexplanatory variables: minority, ses (socio-economic status),female, public, schoolsize, urban, and ruralSome Summary Statisticsreadmathscisesschoolsize26 / 77meanSummary medSummary sdSummary minSummary 400.00
Student ngStudent achievement scores are slightly right-skewed.Consider gamma e :700.010.000.000.000ConcludingRemarks27 / 770.04DensityDensity0.0200.02MEPS Validation0.010MultivariateTwo-Part Model0.020.030MultivariateRegressionGini Index0.08Two-Part Models5101520sci25303510203040readStudent Achievement Scores. Somewhat skewed.50
Comparing Achievement ScoresPredictiveModelingEven after controlling for explanatory variables, scores are highlyrelated.FreesPredictiveModelingTwo-Part ModelsMultivariateRegression round(cor(cbind(umath,usci,uread),method c("spearman")),digits 3)umath usci ureadumath 1.000 0.651 0.646usci 0.651 1.000 0.650uread 0.646 0.650 1.0000.00.20.40.60.81.01.0MultivariateTwo-Part Model0.80.6umathGini Index0.4MEPS igure :28 / 770.20.40.60.81.0 0.00.20.40.60.81.00.00.20.40.60.81.0Scatterplot matrix of Prob Int Transformed student math, science and reading scores.
Likelihood wo-Part ModelsMultivariateRegressionMultivariateTwo-Part ModelGini IndexMEPS ValidationConcludingRemarksWe wish to estimate the full likelihood simultaneouslyUsing the chain-rule from calculus, we have 2F(y1 , y2 ) y1 y2 2C(F1 (y1 ), F2 (y2 )) y1 y2f1 (y1 )f2 (y2 )c(F1 (y1 ), F2 (y2 )),where fj and c are densities corresponding to the distributionfunctions Fj and C.Taking logs, we haveL ln f1 (y1 ) ln f2 (y2 ) ln c(F1 (y1 ), F2 (y2 ))F1 - math - set of beta’s, F2 - sci - set of beta’sone parameter for the copula29 / 77
Comparison of Independence to ingTwo-Part ModelsMultivariateRegressionMultivariateTwo-Part ModelGini IndexMEPS ValidationConcludingRemarks30 / 77CoefficientCopula ParameterMath 7Science .611-0.297-0.7440.789
Multivariate gTwo-Part ModelsMultivariateRegressionMultivariateTwo-Part ModelGini IndexMEPS ValidationConcludingRemarks31 / 77Several outcomes of interest (multivariate), several variablesavailable to explain/predict these outcome (regression)Why multivariate regression?Sharing of information - as with SUR (seemingly unrelatedregressions). This is an efficiency argument - most helpful forsmall data sets.
Multivariate gTwo-Part ModelsMultivariateRegressionMultivariateTwo-Part ModelGini IndexMEPS ValidationConcludingRemarksSeveral outcomes of interest (multivariate), several variablesavailable to explain/predict these outcome (regression)Why multivariate regression?Sharing of information - as with SUR (seemingly unrelatedregressions). This is an efficiency argument - most helpful forsmall data sets.Scientific interest. The main purpose is to understand howoutcomes are related. For example, when I control forclaimant’s age, gender, use of lawyer and so forth, how arelosses and expenses related?Prediction. Assessing association is particularly important forthe tails.In the school example, the interest is in predicting the tails ofthe joint distribution. Which children are performing poorly(well) in math, science, and reading (simultaneously)?31 / 77
Special Case: Longitudinal art ModelsMultivariateRegressionMultivariateTwo-Part ModelGini IndexMEPS ValidationConcludingRemarks32 / 77Here, we think of y (y1 , . . . , yT )0 as a short time series froman outcome of interest, e.g., commercial auto claims from abattery company.There is an extensive literature on linear longitudinal datamodels and their connections to credibility theory.More recently, many are working on generalized linear modeloutcomes with random effects (GLMMs) to handle extensionsto medium/thick tail distributions.
Example. Massachusetts Auto Claims,NAAJ (2005), IME -Part ModelsMultivariateRegressionMultivariateTwo-Part ModelGini IndexMEPS ValidationConcludingRemarks33 / 77Consider claims arising from bodily injury liability.We have annual data from n 29 towns over T 6 years,1993-1998, inclusive.On the margin, we used gamma regressions.Two explanatory variables used for premium rating were (a)population per square mile (log units) and (b) per capita incomeA Gaussian copula was used for time dependencies
A More Complex Example. Singapore AutoClaims, JASA (2008), Astin sider claims arising from three types of auto coverages.bodily injuryown damagethird party claimsEach is skewed and heavy-tailedTwo-Part Models4e 05MultivariateRegressionMEPS Validationown damagethird party property0e 00ConcludingRemarksDensityGini Indexthird party injury2e 05MultivariateTwo-Part Model0200000400000600000Predicted Losses34 / 77Figure :Density by Coverage Type800000
Singapore Auto ClaimsPredictiveModelingFreesData FeaturesPredictiveModelingTwo-Part ModelsMultivariateRegressionMultivariateTwo-Part ModelGini IndexMEPS ValidationConcludingRemarksEach policyholder may have 0, 1 or more (up to 5) claims.Each claim yields one of 7 ( 23 1) combinations of the threecoveragesLots of variables to explain the presence and extent of a claim(age, sex, driving history and so on)Model FeaturesUsed a random effects Poisson for claim countsA multinomial logit for claim typeA copula model with GB2 marginal regressions for claimsseverityResults - Important associations among coverage severities35 / 77
Advertising 3PredictiveModelingFreesPredictiveModelingTwo-Part ModelsMultivariateRegressionMultivariateTwo-Part ModelGini IndexMEPS ValidationConcludingRemarks36 / 77You can learn more about copula regression at ourTechnology Enhanced Learning AELearn/default.aspx
Why Multivariate Two-Part ModelsMultivariateRegressionMultivariateTwo-Part ModelGini IndexMEPS ValidationConcludingRemarks37 / 77For some products, insurers must track payments separatelyby component to meet contractual obligations.In automobile coverage, deductibles and limits depend on thecoverage type, e.g., bodily injury, damage to one’s own vehicleor to another party.In medical insurance, there are often co-pays for routineexpenditures such as prescription drugs.In personal lines umbrella insurance, there are separate limitsfor homeowners and auto coverages, as well overall limits forlosses from all sources.
Why Multivariate Two-Part ModelsMultivariateRegressionMultivariateTwo-Part ModelGini IndexMEPS ValidationConcludingRemarks37 / 77For some products, insurers must track payments separatelyby component to meet contractual obligations.In automobile coverage, deductibles and limits depend on thecoverage type, e.g., bodily injury, damage to one’s own vehicleor to another party.In medical insurance, there are often co-pays for routineexpenditures such as prescription drugs.In personal lines umbrella insurance, there are separate limitsfor homeowners and auto coverages, as well overall limits forlosses from all sources.For other products, there may be no contractual reasons todecompose but insurers do so anyway to better understandthe risk, e.g., homeowners insurance.Multivariate models need not be restricted to only insurancelosses, e.g., Example 3 study of term and whole life insuranceownership, or assets such as stocks and bonds.Commonly understood thatUncertainty(Z1 Z2 ) 6 Uncertainty(Z2 ) Uncertainty(Z2 ).Need to understand the joint behavior of risks (Z1 , Z2 ).
Basic Notation of a Multivariate lingTwo-Part ModelsMultivariateRegressionMultivariateTwo-Part ModelGini IndexMEPS ValidationConcludingRemarksUse a multivariate outcome of interest y where each element of thevector consists of two parts. Thus, we observe y1r1 y . as well as r . yprpand potentially observe y 1 . y . .y p r - the frequency vector, y as the amount, or severity, vector.Decompose the overall likelihood into frequency and severitycomponentsf(r, y ) f1 (r) f2 (y r)38 / 77Let’s look at some Actuarial Applications.
Example 1. Health Care Expenditures,NAAJ (2011)PredictiveModelingFreesMedical Expenditure Panel SurveyPredictiveModelingTwo-Part ModelsMultivariateRegressionMultivariateTwo-Part ModelGini IndexMEPS ValidationConcludingRemarks39 / 779,472 participants from 2003 for in-sample, 9,657 participantsfrom 2004 for validationp 2 Outcomes of Interest - Inpatient (Hospital) and OutpatientExpendituresExplanatory Variables - About 30. Includes demography (age,sex, ethnicity), socio-economic (education, marital status,income), health status, employment (status, industry), healthinsuranceFrequency Model - Logistic, Negative Binomial modelsSeverity Model - Gamma regression, mixed linear models
Example 2. Multi-Peril veModelingTable : Summarizing 404,664 Policy-Years, p 9 PerilsPeril (j)Two-Part ModelsMultivariateRegressionMultivariateTwo-Part ModelGini IndexMEPS ValidationConcludingRemarks40 / LiabilityOtherTheft-VandalismTotalFrequency(in 8125.889 Numberof 3,834 1191,661
Example 2. Multi-Peril veModelingTwo-Part ModelsMultivariateRegressionMultivariateTwo-Part ModelGini IndexMEPS ValidationConcludingRemarksWork appeared in Astin Bulletin (2010) and Variance 2013We drew two random samples from a homeowners databasemaintained by the Insurance Services Office.This database contains over 4.2 million policyholder years.Policies issued by several major insurance companies in theUnited States, thought to be representative of most geographicareas in the US.Our in-sample, or “training,” dataset consists of arepresentative sample of 404,664 records taken from thisdatabase.We estimated several competing models from this datasetWe use a held-out, or “validation” subsample of 359,454records, whose claims we wish to predict.41 / 77
Multi-Peril Homeowners Insurance ingTwo-Part ModelsMultivariateRegressionMultivariateTwo-Part ModelGini IndexMEPS Validatio
Predictive Modeling and Statistics I think about predictive modeling as a subset of business analytics, although many use the terms interchangeably For some, predictive modeling means advanced data-mining tools as per Hastie, Tibshirani and Friedman (2001). The Elements of Sta