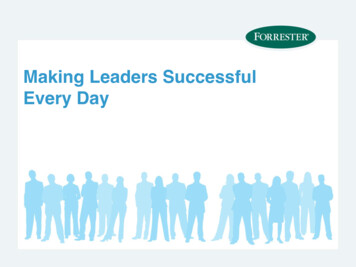
Transcription
Making Leaders SuccessfulEvery Day
The Patterns Of Big DataA Data Management Playbook ToolkitForrester ResearchBrian Hopkins, Principal AnalystJune 11, 2013
Table of contents: examples by patternPatternFirm (industry)/vendor — slide numbersEnterprise data warehouseaugmentationPharmaceutical company/Cloudera.21-23Data refinery plus datawarehouse (DW) / businessintelligence (BI) databasemanagement system (DBMS)edo interactive/Pentaho.31-33Wealth management firm (financial services)/Composite Software.24-26Vestas Wind Systems (manufacturing)/IBM.34-36NK (social media)/Actian.37-39Opera Solutions (IT)/LexisNexis.40-42Rubicon (digital marketing)/MapR.43-45Razorfish (digital marketing)/Teradata Aster.45-48All-in-oneSears (retail)/Datameer.54-57Telecommunications Company/Datameer.58-59Hub-and-spokePharmaceutical company/Cloudera .64-65Internet analytics firm (telecommunications)/Hortonworks.66-68 2013 Forrester Research, Inc. Reproduction Prohibited3
Table of contents: examples by company and industryIndustryEnd user firmsPattern (vendor) — slidesDigital marketingedo interactiveData refinery plus DW / BI DBMS(Pentaho).31-33RazorfishData refinery plus DW / BI DBMS (TeradataAster).46-48RubiconData refinery plus DW / BI DBMS(MapR).43-45Financial servicesWealth management firmEDW augmentation (Composite).24-26PharmaceuticalPharmaceutical companyEDW augmentation (Cloudera).21-23Hub-and-spoke (Cloudera).64-65ITOpera SolutionsData refinery plus DW / BI DBMS(LexisNexis).40-42ManufacturingVestas Wind SystemsData refinery plus DW / BI DBMS(IBM).34-36RetailSearsAll-in-one (Datameer).54-57Social mediaNKData refinery plus DW / BI ionscompanyAll-in-one (Datameer).57-59Internet analytics firmHub-and-spoke (Hortonworks).66-68 2013 Forrester Research, Inc. Reproduction Prohibited4
Table of contents: examples by technology vendorVendor — productPatterns/industry — slide numbersActian — VectorwiseData refinery plus DW / BI DBMS (socialmedia).37-39Composite Software — ServerEDW augmentation (financial services).24-26Cloudera — Cloudera HadoopDistributionEDW augmentation and hub-and-spoke (healthcare).21-23 . . 64-65DatameerAll-in-one (retail and telecommunications).54-59Hortonworks — Hortonworks DataPlatformHub-and-spoke (telecommunications).66-68IBM — InfoSphere BigInsightsData refinery plus DW / BI DBMS(manufacturing).34-36LexisNexis — HPCC SystemsData refinery plus DW / BI DBMS(IT).40-42MapR — M5Data refinery plus DW / BI DBMS (digitalmarketing).43-45PentahoData refinery plus DW / BI DBMS (digitalmarketing).31-33Teradata — AsterData refinery plus DW / BI DBMS (digitalmarketing).46-48 2013 Forrester Research, Inc. Reproduction Prohibited5
Big data patterns research methodology› This toolkit is a companion to our data management playbook strategic plan report. SeeForrester’s June 12, 2013, “Deliver On Big Data Potential With A Hub-And-Spoke Architecture”report to understand how firms are leveraging big data technology to solve problems.› The objective of this research is to see what early adopters have actually done. Many thinkbig data is synonymous with huge volumes of exotic new external data like mobile, social,machine, and log files. But the reality is that firms are taking a pragmatic approach focused onwringing value from internal data first.› We interviewed 11 firms with production implementations. We worked with vendors to identify11 firms we could talk to about their experience with big data implementations. We analyzed 12examples and present the results here.› We uncovered four patterns in big data production implementations. The companionresearch piece identifies a total of seven technology patterns, but some are only now emergingand we did not find examples of clients willing to speak to us. The four patterns we found all leadto a new data management approach that Forrester calls “hub-and-spoke,” which delivers on thehyperflexibility your business needs to be successful in the digital age. 2013 Forrester Research, Inc. Reproduction Prohibited6
Purpose of this toolkitCLARIFY AND ILLUMINATE THE MOST COMMON BIG DATA PATTERNS› Use this research as a basis for business conversations. Study the problems we identifiedand the results firms told us about. Use these examples in your business strategy conversation tostimulate discussions about what is really possible.› Use this research to understand technology architecture patterns. In formulating strategiesto provide more flexibility and lower data cost to your business, study these patterns and lessonslearned to identify the data management technology building blocks your firm really needs.› Use these patterns as part of your vendor selection and solution design. We attempted to bevery broad in the types of technologies we evaluated as part of the patterns. We want to thank thevendors and users that cooperated in providing this information. We have included one page forproduct information from each participating vendor; use these to engage in your investigations. 2013 Forrester Research, Inc. Reproduction Prohibited7
Key takeaways› Big data is about dealing with more data with greater agility and cost-effective performance.› None of our examples used social or pure unstructured, external content, despite the hype.› We found that production implementations generally follow four of the seven patterns we identifiedin our report.› These patterns illustrate the evolving hub-and-spoke data management architecture with an“extract-load-transform” approach.› Improvements in Hadoop, streaming platforms, and in-memory data technology will have aprofound impact on the future of big data solutions. 2013 Forrester Research, Inc. Reproduction Prohibited8
Forrester defines “big data” as techniques andtechnologies that make handling data at extreme scaleaffordable.Source: September 30, 2011, “Expand Your Digital Horizon With Big Data” Forrester reportSo what? When the unaffordable becomes affordable, theimpossible becomes possible.
Financial, customer, and transactional data in coresystems is most important to business strategyPlanning, budgeting, forecasting53%Transactional-corporate apps31%44%Customer38%41%Transactional-custom apps27%36%Spreadsheets31%26%37%Unstructured internal22%29%Product22%28%System logsScientific15%17%3rd party11%Partner12%Video, imagery, audioSensor21%18%Very ial network7%14%Consumer mobile8%13%Unstructured external 5% 10%Base: 603 global decision-makers involved in business intelligence, data management, and governance initiativesSource: Forrsights Strategy Spotlight: Business Intelligence And Big Data, Q4 2012 2013 Forrester Research, Inc. Reproduction Prohibited10
Top performers (firms with greater than 15% annualgrowth) utilize more diverse data sourcesPlanning, budgeting, forecasting53%Transactional-corporate apps31%44%Customer38%41%Transactional-custom apps27%36%Spreadsheets31%26%37%Unstructured internal22%29%Product22%28%System logsScientific15%3rd party11%Partner12%Video, imagery, ocial network7%14%Consumer mobile8%13%Very importantImportantTop performersare 32% morelikely to utilizeexternal datasourcesTop performersare 24% morelikely to expandbeyondcustomer andproduct dataUnstructured external 5% 10%Base: 603 global decision-makers involved in business intelligence, data management, and governance initiativesSource: Forrsights Strategy Spotlight: Business Intelligence And Big Data, Q4 2012 2013 Forrester Research, Inc. Reproduction Prohibited11
Top performers (greater than 15% annual growth)realize they need more“What best describes your firm’s current usage/plans to adopt bigdata technologies and solutions?”Averageperformers arethinking about bigdataTop performersare expandingtheir big dataimplementationsRest oforganizations( 15% growth)(N 482)High performance( 15% growth)(N 58)19%8%7%13%Planning to implementin more than 1 yearPlanning to implementin the next 12 monthsImplemented, notexpanding14% 3%7%21%Expanding/upgradingimplementationBase: 603 global decision-makers involved in business intelligence, data management, and governance initiativesSource: Forrsights Strategy Spotlight: Business Intelligence And Big Data, Q4 2012 2013 Forrester Research, Inc. Reproduction Prohibited12
Big data technology patternsWe spoke to early adoption leaders with productionbig data experienceOUR INTERVIEWS UNCOVERED MULTIPLE EXAMPLES THAT WE GROUPED INTO FOURPATTERNSDescriptionTechnology considerationsEDWaugmentationAn enterprise data warehouse (EDW) remains thelocus of analytic data architecture, but cold data isoffloaded to hub. High-volume data that is not costeffective to move into a warehouse is added andanalyzed using new or existing tools, but primaryanalytics remain in the data warehouse and marts.Distributed data hub options: HDFS, HBaseExisting BI tools are used against the EDW/marts, datavirtualization may be used to integrate NoSQL data with existingBI tools; specialized analytic packages may be added foranalytics of hub data directly.All-in-oneA distributed data system is implemented for longterm, high-detail big data persistence in the hub andanalytics without employing a business intelligencedatabase for analytics. Low level code is written orbig data packages are added that integrate directlywith the distributed data store for extreme-scaleoperations and analytics.Distributed data hub options: Hadoop, HBase, Cassandra,MongoDB, LexisNexisBI tools specifically integrated with or designed for distributeddata access and manipulation are needed. Data operationseither use BI tools that provide NoSQL capability or low levelcode is required (e.g., MapReduce or Pig script). May use datavirtualization technology to integrate other enterprise data andbig data data with existing BI tools.Data refineryplus DW / BIDBMSThe distributed hub is used as a data staging andextreme-scale data transformation platform, butlong-term persistence and analytics is performed bya BI DMBS using SQL analytics.Distributed data hub options: Hadoop, LexisNexis, CassandraBI database is biggest choice: See Forrester’s June 2, 2011,“It’s The Dawning Of The Age Of BI DBMS” report. BI tools withHadoop integration may be used for data manipulation or maywrite low level scripts (Pig), or code (MapReduce).Hub-and-spokeAn evolution of the EDW augmentation, all-in-one,and data refinery plus DW / BI DBMS pattern thatprovides multiple options for both hub-and-spoketechnologies. Data may be harmonized andanalyzed in the hub or moved out to spokes whenmore quality and performance is needed, or whenusers simply want control.All the options in the previous three patterns, plus the data hubmay shift from one physical hub to a logical or distributed one, inwhich different data platforms work together seamlessly tocapture raw data and maintain it in a minimally harmonized anduseful stage. For example, EMC, IBM, Microsoft, and Oracle arebeginning to provide tightly integrated data warehouseappliances and distributed data store (like Hadoop). If the flowand query of data is seamless, we consider this to be a datahub, even though the hub contains a BI DBMS. 2013 Forrester Research, Inc. Reproduction Prohibited13
Big data technology patternsEmerging patterns we did not findWE HAVE SEEN EXAMPLES OF THESE THREE PATTERNS BUT DID NOT FIND PRODUCTIONEXAMPLES THAT MET OUR CRITERIA FOR THIS TOOLKITOur criteria for this research was that we could speak with a user that has a production solution.We may update this toolkit in the future with examples of these three immature patterns as wefind firms willing to talk with us.DescriptionTechnology considerationsStandalonepackageBuy a packaged big data analytic tool to meetdepartment needs rapidly. Uses are generallylimited to the capabilities of the tools. Most arefocused on customer intelligence andmarketing use cases.Examples of packaged big data applications: KXEN, nPario,and NGDataStreaming analyticsA streaming analytics package solution isdeployed to capture and analyze high-velocitydata as it “streams” through the system.Distributed data hub options: none initially, but may add later aspart of path to hub-and-spokeStreaming package examples: IBM InfoSphere Streams,SQLstream, Apache S4, and StormHub-and-spoke plusin-memoryVery early pattern emerging that extends huband-spoke with in-memory and with an elasticcaching or data grid technology to provide veryhigh performance, embedded, or interactiveanalytics without using a BI DBMSHub options are the same as for the hub-and-spoke pattern.In-memory data grid platforms examples: Platfora, ScaleOutSoftware, Tibco SoftwareNote: The June 12, 2013, “Deliver On Big Data Potential With A Hub-And-Spoke Architecture” Forrester reportdefines the seven patterns, but three of them are immature or nascent without production examples we could find.The four patterns on the previous slide are covered in detail in this toolkit. 2013 Forrester Research, Inc. Reproduction Prohibited14
Big data technology patternsBasic pattern building blocksWE IDENTIFIED SIX BUILDING BLOCKS IN THE PATTERNSBuilding blockDescriptionConsiderationsDistributed datahubThe center of the architecture;provides a low-cost data persistencecapability that meets minimumrequirements for availability, security,and recovery, while exposing data forlow-level transformation and analyticsTechnology choices: Hadoop, other NoSQL, open source, orvendor supported, use of advanced technologies such as inmemory data grids, incorporation of mainframe data,integrated unstructured and structured data platforms, loadingand disposal processes, harmonization standards, metadata,cloud versus dedicated optionsIncludes both contextual servicessuch as data quality, master datamanagement, metadata andmodeling, and delivery services suchas federation/virtualization,transformation, movement, andsecurity services that operate on thehub and on the spokesMaster data management strategy and technology choices,integration approach and technology choices, level of quality,service performance, service availability to hub or spokes,vertical and horizontal scaling, cloud service utilizationHigh-performance BI databaseappliances and/or homegrown datawarehouse solutions that areappropriately spokes. Provides highavailability, low latency SQLanalytics.Enterprise versus departmental implementations, data storagecost, analytics requirements for latency and user access, BItool integrations, loading technology and performance, skills ofusers, volume and velocity projects versus tool performancecharacteristics. Examples: Greenplum, Netezza, TeradataAster, and Exadata.HubData servicesSVCEnterprise datawarehouse/departmentalBI databasesDW/BI DBNote: These building blocks emerged from our assessment of the big data implementations. 2013 Forrester Research, Inc. Reproduction Prohibited15
Big data technology patternsBasic pattern building blocks (cont.)WE IDENTIFIED SIX BUILDING BLOCKS IN THE PATTERNSBuilding blockBig dataanalyticspackagesBI and analyticspackagesData ionConsiderationsPackages applications that providedata operations and analytic tools thatinteract directly with hub dataIntegration with NoSQL vendors, needs and skills of users,volume and velocity projects versus tool performancecharacteristics. Examples: Datameer, Pentaho*Traditional business intelligence andanalytics packages that do notaccess, analyze, or operate on datain the hub. Instead they accessprocessed data in an operational orstructured analytic data store.What packages to buy for the functionality needed, how it getssupported, how data is sourced into the package. Examplesinclude BusinessObjects, Cognos, Tableau Software,QlikView. As more vendors add Hadoop integration to theircapabilities, the distinction between these tools and big dataanalytics packages will blur.Tools used by data scientists toexplore, manage hub data, stage fordata mining, and model development,management, and deploymentType of operations, data requirements, departmental versusenterprise team, sandbox and staging area needs, model toapplication integration, model to BI DB integration, analyticand exploration tool needs, operating procedures, security.Example technology: SPSS, R, SAS, Mahout, MapReduce*Note:Pentaho can function as a big data analytics package ora BI and analytics package, depending on how it’s employed.Note: These building blocks emerged from our assessment of the big data implementations. 2013 Forrester Research, Inc. Reproduction Prohibited16
KeyWE USE NUMBERED CIRCLES TO MAP HUB-AND-SPOKE COMPONENTS TO PATTERNS ANDEXAMPLES1 data hub2 data services3 enterprise data warehouse/departmental BI database4 big data aware analytics packages5 standalone BI and analytics packages6 data science workbenchHub 2013 Forrester Research, Inc. Reproduction ProhibitedSVCDW/BI DBBigdataStandaloneWorkbench17
Hub-and-spoke architectureTHE BUILDING BLOCKS CREATE A HUB-AND-SPOKE DATA MANAGEMENT ARCHITECTUREOperational systems (afew examples)6Data science workbench facilitates dataexploration and discoveryMany datawarehouses and BIdatabases moved outto spokes.Traditional extract,transfer, load (ETL) indata warehousessupports quality andstructure needs.53253153Extract-load-transform (notETL!) means data istransformed and loadedinto “spokes” wheneverappropriate.534Some datawarehouse and BI DBappliances have builtin Hadoop integration,so they may beconsidered part of thehub or a spoke.Big-data-aware BI packages can operateagainst schema-less data in the hub directly;standard BI packages operate against relationaldata warehouses and BI databases.In our June 12, 2013, “Deliver On Big Data Potential With A Hub-And-Spoke Architecture” report, we present a more abstract picture of thehub-and-spoke. This diagram reduces that picture to a more concrete level.123456 2013 Forrester Research, Inc. Reproduction ProhibitedHubSVCDW/BI DBBigdataStandaloneWorkbench18
Pattern: enterprise data warehouse augmentationPattern: enterprise data warehouseaugmentationPrimary purpose: make existing data warehouse environment more cost effectiveSecondary purpose: add more data and conduct rapid analysis in the hubExamples:Pharmaceutical company . 21-23Wealth management firm (financial services) .24-26 2013 Forrester Research, Inc. Reproduction Prohibited19
Pattern: enterprise data warehouse augmentationEnterprise data warehouse augmentation patternUsers employ the same BI tools they are used to. Note: some BItools have integrations with data hub platforms, others do not andneed an intermediary such as a data virtualization layerThe main feature of this pattern is that some datawarehouse loads are rerouted to use the big datahub’s data services.or452 or 413VirtualizationThe dirty DW or operational datastore (ODS) contains lightlyharmonized data that can bequeried using structured query orvia an API (e.g., HBase). 2013 Forrester Research, Inc. Reproduction ProhibitedCan be used as a dataservice to expediteintegration of data notsourced to the hub.Virtualization supportstraditional, non-big-dataaware, BI tools.123456HubSVCDW/BI DBBigdataStandaloneWorkbench20
Pattern: enterprise data warehouse augmentationExample: pharmaceutical companyEXAMPLE — ENTERPRISE DATA WAREHOUSE AUGMENTATION PATTERNNeed›››Regulations (e.g., HIPAA)require healthcare orgs to storeelectronic data interchange(EDI) data for extended periodsof timeTrouble meeting seven-yeardata retention requirementwhile processing millions ofclaims every dayExisting system was storingdata as character large objectsin clustered, high-availabilityrelational databasemanagement system (RDBMS)Solution›››››Implemented Hadoop(Cloudera) as an augmentationto existing data warehousesolutions for EDI data archivalImplemented customeringestion process thataggregates all EDI files for theday and loads HadoopResults››Ten times lower total cost ofownership (TCO) whileenabling analytics on storeddataImplemented Hadoop solutionfor about half the cost of otheroptionsParses files into HBase forfaster data accessAs part of ingestion processing,does some data enrichment tosupport downstream analyticsUsed Flume to ingest data fromother transactional systemsDevelopment: four months (initial)Note: The pharmaceutical company appears twice, illustrating a firm initially pursuing one pattern then evolving tohub-and-spoke. 2013 Forrester Research, Inc. Reproduction Prohibited21
Pattern: enterprise data warehouse augmentationPharmaceutical company — conceptual solutionarchitectureWrote custom loader toaggregate one day of data inAvrio file formatData operations andharmonization usingMapReduce and loading toHBaseThe combination of enterpriseanalytics and a data warehouse is stillused but augmented with Hadoop,initially for lower-cost data retention25SqoopCustom Inges onLEGACY FILE BASED SYSTEMS1Flume (1TB/day)SqoopPAYER DATA, TRANSACTIONALSYSTEMS, ETC.Chose to learn low-levelMapReduce coding versususing a packaged toolSource: pharmaceutical company 2013 Forrester Research, Inc. Reproduction ProhibitedORACLE,IBM NETEZZAThis part is addressed the inhub-and-spoke pattern, slides63 to 65.123456HUBSVCDW/BI DBBigdataStandaloneWorkbench22
Pattern: enterprise data warehouse augmentationVendor informationCLOUDERASource: Cloudera 2013 Forrester Research, Inc. Reproduction Prohibited23
Pattern: enterprise data warehouse augmentationExample: wealth management firm (financial services)EXAMPLE — ENTERPRISE DATA WAREHOUSE AUGMENTATIONNeed›››This capital investment andwealth management firm hadstrong business demand forrisk data from many differentsystems.IT was taking a month or two toproduce new reports.There was no way to get all theinformation. Capturing all thehistorical trade data would havecost millions using a“traditional” approach.Solution›››››››Implement Apache Hadoopdistribution (pure open source);a “do it yourself” approachLoad historical trade dataIntegrate this data with othersystems via data virtualizationExpose to existing BI toolsChose this as opposed to usingits existing data warehouseConsidering changing over to asupported Hadoop distributionand adding more tools (likeHBase)Results›››››More than 100 million recordsin Hadoop todayImplemented at a fraction of thecost of a relational databaseapproachCan produce reports in daysBusiness can access big datain small chunks for self-serviceanalytics using SpotfireTremendous data growth,expect over 1 PB next yearImplemented with three fulltime equivalents (FTEs)internallyDevelopment: three FTEs 2013 Forrester Research, Inc. Reproduction Prohibited24
Wealth management firm — conceptual solutionarchitectureVisualization/analysis toolStatisticalmodeling5Custom analysisjobs6ViewViewExample of how avirtualization serviceexposes big data in smallviews to other toolsViewData virtualizationWebservice2Hadoop and extreme-scale ELTused as a cost-effective way toaugment the data warehousewith cost-effective datapersistence and ubSVCDW/BI DBBigdataStandaloneWorkbenchFor detailed discussion of the impact data virtualization is having on firm’s data architectures, see the June 15, 2011,“Data Virtualization Reaches Critical Mass” Forrester report. 2013 Forrester Research, Inc. Reproduction Prohibited25
Pattern: enterprise data warehouse augmentationVendor informationCOMPOSITE gementGovernance, risk,and complianceHuman capitalmanagementMergers andacquisitionsSingle view ofenterprise dataSupply chainmanagementSAP dataintegrationComposite data virtualization platformDevelopmentenvironmentRuntime agerComposite information serverStudioMonitorPerformance plusadaptersActive clusterXMLPackaged appsRDBMSExcel filesData warehouseOLAP cubesHadoop/big dataXML docsFlat filesWeb servicesSource: Composite Software 2013 Forrester Research, Inc. Reproduction Prohibited26
Pattern: enterprise data warehouse augmentationForrester’s point of view› The enterprise data warehouse augmentation pattern is the easiest to fund when big data isperceived as an “IT thing.”› The benefits are tangible and immediately realized, the business impacts are manageable, andthe upside is huge.› We suggest: Start by doing a five-year TCO calculation on all data in your data warehouse or data mart environments.Include the cost of integrating all that data. Do an analysis of how much data in your data warehouse environment has no or low analytic usage. Determine if any of this cold data has retention requirements that drive its storage. Develop a five-year TCO for an open source distributed data hub. See if a business case can be made.› Your biggest technology strategic decisions are: How to enable analytics on data in the hub. Data virtualization and BI tools with big data tool integrationcapability can help. What distributed data hub technology to choose, and how to acquire the skills. The approach to data movement and the level to which hub data is harmonized. 2013 Forrester Research, Inc. Reproduction Prohibited27
Pattern: enterprise data warehouse augmentationLessons learned from users› Dealing with raw data is messy. This is a new way of thinking. You need a data harmonization andintegration approach that delivers a minimum quality level. Think minimum viable quality, notcompletely clean data. An enterprise data model is essential for semantic consistency, but thedata doesn’t have to conform completely to the model to be useable — this is one reason big datadelivers hyperflexibility.› Data access will be challenging, both politically and technically. Ensure your strategy andbusiness case is strong enough to overcome these challenges. Be sure you have support fromthe top to overcome parochial concerns. Define who owns the data once you have sourced andharmonized it.› Tool selection makes all the difference. The devil is in the details — understand what “integrateswith Hadoop” really means in terms of specific versions of Hadoop components, the specificintegration functionality, and quality of community and vendor support available. 2013 Forrester Research, Inc. Reproduction Prohibited28
Pattern: data refinery plus DW / BI DBMSPattern: data refinery plus DW / BIDBMSPrimary purpose: lower the cost of data capture and operations while loading astructured business intelligence database for low latency structured analyticsExamples:edo interactive . . .31-33Vestas (manufacturing) . . .34-36NK (social media) . .37-39Opera Solutions (IT) . 40-42Rubicon (digital marketing) .43-45Razorfish (digital marketing) .46-48 2013 Forrester Research, Inc. Reproduction Prohibited29
Pattern: data refinery plus DW / BI DBMSData refinery plus DW / BI DBMSData science work is primarily developingmodels and attributes for deployment toembedded analytics and BI DBMS.The primary feature of this pattern is theloading of an EDW or other BI DBMS froma distributed hub.6Distributed data hub anddata services’ primarypurpose is extreme-scaledata operations.Sourcesystems5Raw data is typicallypurged after harmonizationand archival.2 or 4231Sourcesystems 2013 Forrester Research, Inc. Reproduction ProhibitedData may be archivedafter harmonization, butthe BI DBMS remains theprimary place foranalytics.123456HubSVCDW/BI DBBigdataStandaloneWorkbench30
Pattern: data refinery plus DW / BI DBMSExample: edo interactiveNeed››››B2B electronic marketing firmwas projecting rapid datagrowth, needed to rethinkinfrastructure. Currentlyproviding 120 million offers amonth and more than 25 milliontransactions a day, producingas much as 50 terabytes ofdata and growing.Wanted an affordable and easyto use extract, load, andtransform tool (not ETL) tohandle massive scaleaffordablySolution›››Implemented a Hadoop(Cloudera 3) data refineryplatform to load massiveamounts of data t
An enterprise data warehouse (EDW) remains the locus of analytic data architecture, but cold data is offloaded to hub. High-volume data that is not cost effective to move into a warehouse is added and analyzed using new or existing tools, but primary analytics remain in the data warehouse and marts. D