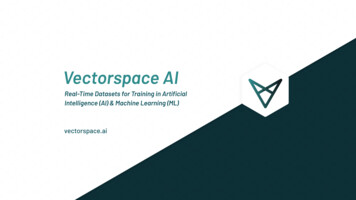
Transcription
Vectorspace AIReal-Time Datasets for Training in ArtificialIntelligence (AI) & Machine Learning (ML)vectorspace.ai
What Do We Do?1Vectorspace AI builds real-time on-demand datasets that are used to train the worlds AI & Machine Learning (ML) systems via tiered subscriptions2Customer subscriptions are converted to BTC/ETH then to our VXV utility token on the exchange3This VXV is placed in a wallet which doubles as a what we call a “VXV wallet-enabled API key”4Datasets are distributed using our VXV wallet-enabled API services5Datasets are tracked using our VXV Data Provenance Pipeline (DPP) hashReddit AMA on mments/9k5i8u/askscience ama series were team vectorspace ai/
What Our Customers Are Saying“Really great work - just readthe details. Will you be writingan academic paper based onyour findings? “”You guys are working on“Requesting API Access. Reallysome really incredible stuff.intrigued by the amazing workCan’t wait to get my hands onyour team has done aroundsome code especially aftercontent summarization. WasPJ Hampton, PhD Researcher, NLPlooking at those links nowwondering what is the processwow.this is more of what wefor applying for API access.need in the world, this willIs there an open source“Hello, I’m pretty curious andchange everything :D Cool.implementation that we canamazed by how powerful youryou’re based in SF would lovecontribute towards?”summarization of articlesR. Tewarito meetup and grab a coffeeis ! I’d like to try it out on asometime and tell you what I’mside project so that’s why I’mworking on.”“I tried it with the 50 Shadessending you this e-mail :)”N. SherriffN. Hardyof Grey text (btw, impressivetext generation work) and I“I do a lot of hobby orfound the results to be just“Great work with the contextexperimental projects usingOk. However, I then tried itsummarization! May I requestNLP, I’d love a chance to usewith a new text related to ana copy of the code base? Iyour system. Is this something app description and found thewon’t use for commercialresult to be quite impressive,purposes, or anything like that. you think you may release asopen source?”both the summarization andJust academic insight.”T. Leleluthe keywords extraction. WellH. Petersondone!”“That’s actually really cool.Now you’ve got me looking intothese algorithms.”bcolb“That’s. incredible. Wow.”chrismbarrcarlos argueta“I’m really impressed by howaccurate your algorithm is,and I would like to try it outsummarizing some newsconcerning the same topic(in different languages). Mygoal is to create an algorithmthat can merge some textsspeaking about the samesubject, so that’s why I willread your mathematicalexplanations on the API page.FYI I tried it on French newsarticles, and it seems to beworking almost as good asenglish. (it may work better onlatin-based languages right?)”“Amazing work! Is there arepository I can have accessto? These are excitingtimes for aspiring amateuracademics like myself. Publicknowledge of this calibre isexactly what humanity needsto overcome the debt of ourmyriad lapses in good faith.”W. Sahatdjian“Is there somewhere I can usethis same software/algorithmto actually summarize otherthings? I am thoroughlyimpressed.”“I’m comparing this algorithmwith the one i wrote forwingztv.com (shameless plug)i am really shocked by howmuch better these guys algo is.although mine was just kindawas hacked up. it just seessentences with more wordsin common and ranks then byit, and then i just grab the top5. this algo looks a lot morefancy O.o probably why it’s alot better lol.”primary action itemsmoridin007Anon
Current Partners & Collaborators
Market Opportunity“Ten million dollars for a data set might seem likea lot, but if you can spend 10 million to make ahundred, 10 million suddenly doesn’t seem like a lotof money anymore.”Kevin McPartland, researcher at Greenwich AssociatesCitation“Some investment firms are hiring individuals in theemerging role of Head of Data It may possibly bethe next frontier for funds looking for an investmentedge” where the new norm is a “continuousinformation arbitrage.”CME GroupCitation
Technology & Commercially Released Products“Coming up with features is difficult, time-consuming,requires expert knowledge. ‘Applied machine learning’is basically feature engineering.”Patents, Awards & PapersWinner R&D100 Award - Lawrence Berkeley National LaboratoryAndrew Ng, Google Brain Deep Learning founder, former Baidu AI ChiefCitation“In machine learning and deep learning we can’t doanything without data. So the people that createdatasets for us to train our models are the (oftenunder-appreciated) heroes.”fast.aiCitation“In the future AI will be diffused into every aspect of theeconomy.”Nils J. Nilsson, Founding researcher, Artificial Intelligence & ComputerScience, Stanford UniversityCitationSystem and method for generating a relationship network - K Franks, CA Myers,RM Podowski - US Patent 7,987,191, 2011Inter-term relevance analysis for large librariesMatching and recommending relevant videos and media to individual search engine resultsMedia discovery and playlist generationSystem and method for summarizing search resultsDiscovering and scoring relationships extracted from human generated listsStatistical modeling of biomedical corpora: mining the Caenorhabditis Genetic CenterBibliography for genes related to life span - Blei DM, Franks K, Jordan MI, Mian IS.
Vectorspace AI (VXV)Science & Data EngineeringBusiness UnitsVXV Wallet-Enabled APISmart BasketsNLP/ML/AI & AnalyticsVXV Wallet-Enabled APIVXV Life Sciences FundCustomersRevenue, Profit & Funding:(Cryptocurrency, Stocks, Assets)- Subscriptions- Licensing- 20% Net- Revenue Sharing- Transaction FeesAlternative Data ServicesFor:Streaming Real-Time On-Demand Datasets(Triangulated Sentiment/Alternative & Proprietary)QuantBotFeature Vector Supercolumns(automated feature engineering)On-Demand Datasets(Triangulated Sentiment/Alternative)Data Engineering, Algorithms & Analytics (Advanced NLP/ML/AI)- AI & Data Operations- Exchanges- Trading Desks- Funds- Data Vendors- Partners
VXV Wallet-Enabled API KeyTransaction Network (General)Science & Data EngineeringBusiness UnitsCustomersVXV Wallet-Enabled APIRequired VXV per Wallet-Enabled API Keyat any given timeFinancial Product Developers (3)Smart Basket ETF API42.9 nges (2)QuantBot API153.1 VXV0x32Be343B94f860124dC4fEe278FDCBD38C102D88VXV WalletValidatorCurrent Partners (3)VXV API KeyPricing AlgorithmNLP API153.1 mand Datasets API153.1 VXV0x32Be343B94f860124dC4fEe278FDCBD38C102D88JSON API Endpoint: GET ending smart basketsParams: vxv token addr 0xC2A568489BF6AAC5907fa69f8FD4A9c04323081DData Engineering, Algorithms & Analytics (Advanced NLP/ML/AI)Data Vendors, ML/AI & Funds (3)
VXV Wallet-Enabled API KeysSubscription-to-Exchange On-Demand Order ExecutionScience & Data EngineeringBusiness UnitsCustomersVXV Smart Contract(Subscription Handler)SubscriptionsFinancial Product DevelopersAll Industry Sectors: Data/ML/AIBTCETHUSDVXVExchangeBTCPython Trade Execution ModuleETHUSDUSD to BTC/ETHVXV Wallet-Enabled APIKey Generator & PopulatorCustomer Activation Module(Tier 1 Services)VXVVXV API KeyPricing AlgorithmExchange APIAutomated BuyOrder Execution:VXVVXV Wallet-Enabled API Key: 1.000 VXV / 0xC2A568489BF6AAC5907fa69f8FD4A9c04323081DJSON API Endpoint: GET ending smart basketsParams: vxv token addr 0xC2A568489BF6AAC5907fa69f8FD4A9c04323081DData Engineering, Algorithms & Analytics (Advanced NLP/ML/AI)Active VXV Customer Pool(API Calls per Day)
VXV Data Engineering PipelineRevenue Architecture DiagramEng Group 1Real-TimeData Acquisition:CrawlersEng Group 2Crawl SnapNewsCrawl SnapProfiles (Crypto)Crawl SnapProfiles (Stocks)Static Data Sources- Dictionaries- Encyclopedias- PatentsEng Group 3StorageGoogle CloudAWSVXV Data Provenance Pipeline (DPP) Hash:44a173f6b45d74355fd28e3ff392b07e06f8855fEng Group 2Eng Group 4Dataset BuilderAlgo 1: VXV-LBNLAlgo 2: Word2VecAlgo 3: GloVeVector IndexerIngest (Last 24hrs)VisualizationsDataset A: Context-Controlled ClusteringDataset B: Context-Controlled Sentiment- Near Real-Time (1,440 calls per day per customer)- Correlation Matrix- Trends, Topics, Entities- Near Real-Time (1,440 calls per day per customer)- Correlation Matrix- Trends, Topics, EntitiesEng Group 1, 2VXVWallet-Enabled APISubscription LayerStripe/Uphold.comto Smart ContractVXV Smart Contract(Subscription Handler)BTCETHUSDData Engineering, Algorithms & Analytics (Advanced NLP/ML/AI)VXV* See previous slideUSD to BTC/ETH
VXV Streaming Real-Time On-Demand DatasetsWith VXV Wallet-Enabled API SubscriptionsCustomersStreaming Real-Time On-Demand Dataset(100,000 Datasets per Day)Financial Product DevelopersAll Industry Sectors: Data/ML/AISubscriptionsBTCETHUSDVXVVXV Data Provenance Pipeline (DPP) ngeExchange asourcesAutomated BuyOrder Execution:VXV- Real-Time Search Trends:Google, Bing, FB, Twitter- Emerging Technologies- Bitcoin, Price Relationships- Chemicals- Pharmaceuticals- Context-Controlled Sentiment- Top Gainers, Top Losers- Metals- Foods- Human Genes- Real Estate- Commodities- Global Geography,Cities- Botanicals,Phytochemicals,MicronutrientsTopic- Data Publishers- Data Vendors- Public- Proprietory- OntologiesTopic- Reports- Filings- News- SocialData Engineering, Algorithms & Analytics (Advanced NLP/ML/AI)
Investment & Growth StrategyVectorspace AI is raising growth capital in 50k tranches up to 150kin exchange for equity. Use of funds will go towards:Support for our current customers & customer discovery processFund will be used to convert pilot programs with current customers to full-fledged revenue-generating partnershipsAugmenting our Silicon Valley based team of engineers & customer support staffBuild sales & marketing organization to capture business globallyLegal opinions
On-Demand Price TiersTier 1 – Free LimitedTier 2 – 0.99 per On-Demand UpdateTier 3 – 1,950.00/Mo 0.99 per ODUFreeData VendorInstitutionalUpdates1 Free On-Demand Update100 Free On-Demand Updates10,000 Free On-Demand UpdatesEquity TypesNYSE stocksBitcoin & Cryptocurrencies, NYSE,Nasdaq, OTCAnyData StreamsFeatured onlyAnyAnyCustom FeaturesYesYesSupportYesYes
Revenue ModelYear 1Year 2Year 24252365252365252365On-Demand Updates per 73,600262,800,000Number of Custom Data Sources555555 0.99 0.99 0.99 0.99 0.99 0.99 44,906,400 195,129,000 98,794,080 676,447,200 170,644,320 1,300,860,000CustomersTrading hours per dayTrading days per year (avg.)Cost per On-Demand UpdateRevenueTotal Revenue 240,035,400 775,241,280.00 1,471,504,320.00
Vectorspace CYM Short/Long BasketsThe ApproachThe Story & TeamIt is well-established that companies participate in The CYM Team is lead by Kasian Franks, who hasan economic ecosystem that has parallels to thebeen working with artificial intelligence for morenatural world: sympathetic, symbiotic, and parasitic than 15 years. While at Lawrence Berkeley Nationalrelationships can all be found among and betweenLaboratory, he led a team of researchers incorporations. The Efficient Market Hypothesisdeveloping NLP/NLU algorithms to extract hiddensuggests that all of these potential relationshipsconnections between human genes involved inbetween companies are known by the entireextending human lifespan under the direction ofmarketplace at the same time and, thus, they areMina Bissell, director of the Life Sciences division.already factored into company stock prices.NLP/NLU based pattern recognition and matchingtechnologies were necessary to interpret thisIn reality, few investors have the proper tools togenetic data and map it to medical health data anduncover these hidden corporate relationships andprotein functionality. The team then developedaccurately project the directional knock-on impactthe first NLP/NLU system to uncover hiddenof a change in one company in such a relationship.relationships between proteins, disease states,and biological functions in the area of spaceThe CYM System utilizes cutting-edge artificialbiosciences for the purpose of LET radiation repairintelligence technology based on patentedVectorspace techniques to identify and understand and protection during extended human space travel.hidden relationships between companies to enableinvestors to get ahead of the broader impacts ofbreaking news. Strategies can be tailored based oncustomer needs.The heart of Kasian’s NLP/NLU technology was asystem designed to understand and read scientificabstracts, medical journals and updating newswhile uncovering hidden relationships among thebiological components studied. In 2008, the teamadapted this technology to the investment worldand created CYM.The TechnologyThe CYM System draws in a massive amount oftriangulated and alternative data. The System thenuses proprietary, patented algorithms and softwareto detect hidden relationships within a givencontext.Based on a global event occurring, the CYM systemthen automatically generates short baskets ofequities by leveraging context controlled NLP/NLU.The result is an ability to spot information arbitrageanomalies based on global events.System TestingThe CYM System has been tested by outsideauditors. Historical performance is based on theanalysis of human language surrounding publiccompanies located in public and private data pools.
Vectorspace CYM Short/Long Basket ReturnsS&P 500 Stocks (ETFs not included)Hidden Relationship ReturnsS&P 66%13.46%1.20%2015-04/166.79%-2.93%9.72%
CYM Data 2008-2015
CYM Data 2008-2015 (cont.)
CYM Data 2010-2015
CYM Data 2010-2015 (cont.)
CYM Data 2012-2015
CYM Data 2012-2015 (cont.)
Trade File FormatOne file (csv) per symbol, 18 columns per fileNo header row, 1 row per tradeColumn #FieldDetails1Trade reference numberCount of the trade for a given dateReset to 1 for the first trade of each dateSuffix “Ref ” string to trade counter2DateYYYYMMDD format3Position typeLong / Short4Profit (in )Profit per position per share in s5Symbol6Entry priceAssume top-ask for buy and top-bid for sell7Exit priceAssume top-ask for buy and top-bid for sell8Position sizeNumber of shares / contracts traded9Entry valueColumn 6 x Column 810Exit valueColumn 7 x Column 811Profit (in bips)Profit per position in basis points12Entry timeTime in HH:MM format – in Eastern time (US)13Exit timeTime in HH:MM format – in Eastern time (US)14Hold TimeTime from entry to exit (in minutes)Minimum profit (in bips)Minimum bps seen during the life of the tradesMeasured from time of entry - granularity of atleast once a minute – can be more granular(Once every order-book change / execute)16Maximum profit (in bips)Maximum bps seen during the life of the tradesMeasured from time of entry - granularity of atleast once a minute – can be more granular(Once every order-book change / execute)17Minimum profit timeTime in HH:MM format – in Eastern time (US)18Maximum profit timeTime in HH:MM format – in Eastern time (US)15
Daily File FormatOne file (csv) per symbol, 10 columns per fileNo header row, 1 row per trading day – if there were no trades on atrading day, populate all fields (except Date) with “0”Column #FieldDetails1DateYYYYMMDD format2Positive positionsCount of positions 03Total positionsCount of positions for the day4Long positionsCount of long positions for the day5Short positionsCount of short positions for the day6Daily bpsCumulative bps for all trades for the symbol for the date7Positive longsCount of long positions 08Negative longsCount of long positions 09Positive shortsCount of short positions 010Negative shortsCount of short positions 0
TeamKasian FranksMike MuldoonA 25 year Silicon Valley veteran and pioneer in digital contentstreaming much before Netflix and Amazon entered the space.Franks started as a software engineer working for companiessuch as Genentech, Sun, Oracle, Cisco, Motorola and Morningstar.In 2005, as a genomic research scientist at Lawrence BerkeleyNational Laboratory, he was the lead inventor of new vector spacerepresentations of hidden relationship networks in data along withpattern recognition systems aiming to mimic portions of humancognition. While at the Lab, he co-authored a paper with Michael I.Jordan (machine learning maestro and doctoral advisor to AndrewNg) titled “Statistical modeling of biomedical corpora: mining theCaenorhabditis Genetic Center Bibliography for genes related to lifespan - Blei DM1, Franks K, Jordan MI, Mian IS.”. Following this, he cofounded SeeqPod in partnership with Lawrence Berkley NationalLaboratory of U.S . Department of Energy that was then headed bySteven Chu, Energy Secretary in President Obama’s first term andwinner of Nobel Prize in Physics (1997). SeeqPod was a consumerfacing streaming data search/discovery/recommendationplatform originally powering Spotify and others while attracting 50million monthly active users and 250 million monthly search andrecommendation queries. In 2008, his team won the R&D100 award.The company was acquired in 2009. He continues to spend his timementoring startup founders and hedge funds on Machine Learning,Natural Language Processing (NLP), Artificial Intelligence and datascience strategies.Mike’s first program was an ad-lib game, which he wrote in 5th gradeon a TRS-80 owned by the school’s computer club. He has sinceestablished a track record of leading large projects from concept todelivery, and brings over 20 years of experience to Starmine.ai. Asemployee #1 at SeeqPod, he took the product from whiteboard to50M monthly active users, delivering an architecture that deployedhundreds of servers across seven different data centers globallypushing 1.6Gb/s of traffic.Caleb PateCaleb is currently working in Data Science, AI & Machine Learningwith a focus on feature engineering and cryptocurrencies whilecontinuing to define, explore and solve problems related torecommendation systems. As a member of the founding teamat SeeqPod, he built the core Music Recommendation & Curationstrategy. He played in a band with an international following and ranan independent music label and continues to create new musicalworlds as a Producer, Musician and DJ.Team Continued: https://vectorspace.ai/index.html#Team
vectorspace.ai
“I tried it with the 50 Shades of Grey text (btw, impressive text generation work) and I found the results to be just Ok. However, I then tried it with a new text related to an app description and found the result to be quite impressive, both the summarization and the keywords extract