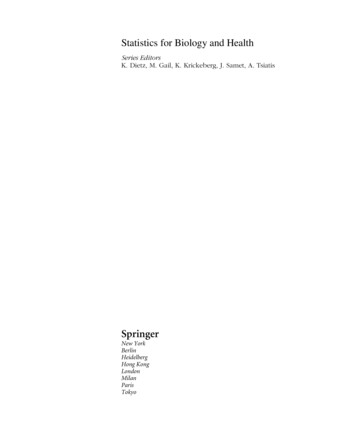
Transcription
Statistics for Biology and HealthSeries EditorsK. Dietz, M. Gail, K. Krickeberg, J. Samet, A. TsiatisSpringerNew YorkBerlinHeidelbergHong KongLondonMilanParisTokyo
SURVIVALANALYSISTechniques forCensored andTruncated DataSecond EditionJohn P. KleinMedical College of WisconsinMelvin L. MoeschbergerThe Ohio State University Medical CenterWith 97 Illustrations1Springer
John P. KleinDivision of BiostatisticsMedical College of WisconsinMilwaukee, WI 53226USASeries EditorsK. DietzInstitut für Medizinische BiometrieUniversität TübingenWestbahnhofstrasse 55D-72070 TübingenGermanyK. KrickebergLe ChateletF-63270 ManglieuFranceA. TsiatisDepartment of StatisticsNorth Carolina State UniversityRaleigh, NC 27695USAMelvin L. MoeschbergerSchool of Public HealthDivision of Epidemiology and BiometricsThe Ohio State University Medical CenterColumbus, OH 43210USAM. GailNational Cancer InstituteRockville, MD 20892USAJ. SametSchool of Public HealthDepartment of EpidemiologyJohns Hopkins University615 Wolfe St.Baltimore, MD 21205-2103USALibrary of Congress Cataloging-in-Publication DataKlein, John P., 1950–Survival analysis : techniques for censored and truncated data / John P. Klein, MelvinL. Moeschberger. — 2nd ed.p. cm. — (Statistics for biology and health)Includes bibliographical references and index.ISBN 0-387-95399-X (alk. paper)1. Survival analysis (Biometry) I. Moeschberger, Melvin L.II. Title. III. Series.R853.S7 K535 20032002026667610 .7 27–dc21ISBN 0-387-95399-XPrinted on acid-free paper. 2003, 1997 Springer-Verlag New York, Inc.All rights reserved. This work may not be translated or copied in whole or in part without the writtenpermission of the publisher (Springer-Verlag New York, Inc., 175 Fifth Avenue, New York, NY 10010,USA), except for brief excerpts in connection with reviews or scholarly analysis. Use in connection withany form of information storage and retrieval, electronic adaptation, computer software, or by similar ordissimilar methodology now known or hereafter developed is forbidden.The use in this publication of trade names, trademarks, service marks, and similar terms, even if they arenot especially identified as such, is not to be taken as an expression of opinion as to whether or not theyare subject to proprietary rights.Printed in the United States of America.9 8 7 6 5 4 3 2 1SPIN 10858633www.springer-ny.comSpringer-Verlag New York Berlin HeidelbergA member of BertelsmannSpringer Science Business Media GmbH
PrefaceThe second edition contains some new material as well as solutions tothe odd-numbered revised exercises. New material consists of a discussion of summary statistics for competing risks probabilities in Chapter 2and the estimation process for these probabilities in Chapter 4. A newsection on tests of the equality of survival curves at a fixed point intime is added in Chapter 7. In Chapter 8 an expanded discussion is presented on how to code covariates and a new section on discretizing acontinuous covariate is added. A new section on Lin and Ying’s additivehazards regression model is presented in Chapter 10. We now proceedto a general discussion of the usefulness of this book incorporating thenew material with that of the first edition.A problem frequently faced by applied statisticians is the analysis oftime to event data. Examples of such data arise in diverse fields suchas medicine, biology, public health, epidemiology, engineering, economics and demography. While the statistical tools we shall presentare applicable to all these disciplines our focus is on applications ofthe techniques to biology and medicine. Here interest is, for example,on analyzing data on the time to death from a certain cause, duration of response to treatment, time to recurrence of a disease, time todevelopment of a disease, or simply time to death.The analysis of survival experiments is complicated by issues of censoring, where an individual’s life length is known to occur only in acertain period of time, and by truncation, where individuals enter thestudy only if they survive a sufficient length of time or individuals arev
viPrefaceincluded in the study only if the event has occurred by a given date. Theuse of counting process methodology has, in recent years, allowed forsubstantial advances in the statistical theory to account for censoringand truncation in survival experiments. The book by Andersen et al.(1993) provides an excellent survey of the mathematics of this theory.In this book we shall attempt to make these complex methods moreaccessible to applied researchers without an advanced mathematicalbackground by presenting the essence of the statistical methods andillustrating these results in an applied framework. Our emphasis is onapplying these techniques, as well as classical techniques not basedon the counting process theory, to data rather than on the theoretical development of these tools. Practical suggestions for implementingthe various methods are set off in a series of practical notes at theend of each section. Technical details of the derivation of these techniques (which are helpful to the understanding of concepts, though notessential to using the methods of this book) are sketched in a series oftheoretical notes at the end of each section or are separated into theirown sections. Some more advanced topics, for which some additionalmathematical sophistication is needed for their understanding or forwhich standard software is not available, are given in separate chaptersor sections. These notes and advanced topics can be skipped withouta loss of continuity.We envision two complementary uses for this book. The first is asa reference book for investigators who find the need to analyze censored or truncated life time data. The second use is as a textbook fora graduate level course in survival analysis. The minimum prerequisitefor such course is a traditional course in statistical methodology. Thematerial included in this book comes from our experience in teachingsuch a course for master’s level biostatistics students at The Ohio StateUniversity and at the Medical College of Wisconsin, as well as from ourexperience in consulting with investigators from The Ohio State University, The University of Missouri, The Medical College of Wisconsin, TheOak Ridge National Laboratory, The National Center for ToxicologicalResearch, and The International Bone Marrow Transplant Registry.The book is divided into thirteen chapters that can be grouped intofive major themes. The first theme introduces the reader to basic concepts and terminology. It consists of the first three chapters which dealwith examples of typical data sets one may encounter in biomedicalapplications of this methodology, a discussion of the basic parametersto which inference is to be made, and a detailed discussion of censoringand truncation. New to the second edition is Section 2.7 that presents adiscussion of summary statistics for competing risks probabilities. Section 3.6 gives a brief introduction to counting processes, and is includedfor those individuals with a minimal background in this area who wishto have a conceptual understanding of this methodology. This sectioncan be omitted without jeopardizing the reader’s understanding of latersections of the book.
PrefaceviiThe second major theme is the estimation of summary survival statistics based on censored and/or truncated data. Chapter 4 discusses estimation of the survival function, the cumulative hazard rate, and measures of centrality such as the median and the mean. The construction ofpointwise confidence intervals and confidence bands is presented. Herewe focus on right censored as well as left truncated survival data sincethis type of data is most frequently encountered in applications. Newto the second edition is a section dealing with estimation of competingrisks probabilities. In Chapter 5 the estimation schemes are extendedto other types of survival data. Here methods for double and intervalcensoring; right truncation; and grouped data are presented. Chapter6 presents some additional selected topics in univariate estimation, including the construction of smoothed estimators of the hazard function,methods for adjusting survival estimates for a known standard mortalityand Bayesian survival methods.The third theme is hypothesis testing. Chapter 7 presents one-, two-,and more than two-sample tests based on comparing the integrateddifference between the observed and expected hazard rate. These testsinclude the log rank test and the generalized Wilcoxon test. Tests fortrend and stratified tests are also discussed. Also discussed are Renyitests which are based on sequential evaluation of these test statistics andhave greater power to detect crossing hazard rates. This chapter alsopresents some other censored data analogs of classical tests such as theCramer–Von Mises test, the t test and median tests are presented. Newto this second edition is a section on tests of the equality of survivalcurves at a fixed point in time.The fourth theme, and perhaps the one most applicable to appliedwork, is regression analysis for censored and/or truncated data. Chapter 8 presents a detailed discussion of the proportional hazards modelused most commonly in medical applications. New sections in this second edition include an expanded discussion of how to code covariatesand a section on discretizing a continuous covariate. Recent advancesin the methodology that allows for this model to be applied to lefttruncated data, provides the investigator with new regression diagnostics, suggests improved point and interval estimates of the predictedsurvival function, and makes more accessible techniques for handlingtime-dependent covariates (including tests of the proportionality assumption) and the synthesis of intermediate events in an analysis arediscussed in Chapter 9.Chapter 10 presents recent work on the nonparametric additive hazard regression model of Aalen (1989) and a new section on Lin andYing’s (1994) additive hazards regression models. One of these modelsmodel may be the model of choice in situations where the proportionalhazards model or a suitable modification of it is not applicable. Chapter11 discusses a variety of residual plots one can make to check the fit ofthe Cox proportional hazards regression models. Chapter 12 discussesparametric models for the regression problem. Models presented in-
viiiPrefaceclude those available in most standard computer packages. Techniquesfor assessing the fit of these parametric models are also discussed.The final theme is multivariate models for survival data. In Chapter13, tests for association between event times, adjusted for covariates,are given. An introduction to estimation in a frailty or random effectmodel is presented. An alternative approach to adjusting for associationbetween some individuals based on an analysis of an independentworking model is also discussed.There should be ample material in this book for a one or two semestercourse for graduate students. A basic one semester or one quarter coursewould cover the following rChapterChapter23, Sections 1–547, Sections 1–6, 889, Sections 1–41112In such a course the outlines of theoretical development of the techniques, in the theoretical notes, would be omitted. Depending onthe length of the course and the interest of the instructor, thesedetails could be added if the material in section 3.6 were coveredor additional topics from the remaining chapters could be addedto this skeleton outline. Applied exercises are provided at the endof the chapters. Solutions to odd numbered exercises are new tothe second edition. The data used in the examples and in most ofthe exercises is available from us at our Web site which is accessible through the Springer Web site at http://www.springer-ny.com ml.Milwaukee, WisconsinColumbus, OhioJohn P. KleinMelvin L. Moeschberger
ContentsPrefacevChapter 1 — Examples of Survival Data11.1 Introduction11.2 Remission Duration from a Clinical Trial for Acute Leukemia21.3 Bone Marrow Transplantation for Leukemia31.4 Times to Infection of Kidney Dialysis Patients61.5 Times to Death for a Breast-Cancer Trial71.6 Times to Infection for Burn Patients81.7 Death Times of Kidney Transplant Patients81.8 Death Times of Male Laryngeal Cancer Patients91.9 Autologous and Allogeneic Bone Marrow Transplants101.10 Bone Marrow Transplants for Hodgkin’s andNon-Hodgkin’s Lymphoma111.11 Times to Death for Patients with Cancer of the Tongue12ix
xContents1.12 Times to Reinfection for Patients with SexuallyTransmitted Diseases131.13 Time to Hospitalized Pneumonia in Young Children141.14 Times to Weaning of Breast-Fed Newborns141.15 Death Times of Psychiatric Patients151.16 Death Times of Elderly Residents of a RetirementCommunity161.17 Time to First Use of Marijuana171.18 Time to Cosmetic Deterioration of Breast Cancer Patients181.19 Time to AIDS19Chapter 2 — Basic Quantities and Models212.1 Introduction212.2 The Survival Function222.3 The Hazard Function272.4 The Mean Residual Life Function and Median Life322.5 Common Parametric Models for Survival Data362.6 Regression Models for Survival Data452.7 Models for Competing Risks502.8 Exercises57Chapter 3 — Censoring and Truncation633.1 Introduction633.2 Right Censoring643.3 Left or Interval Censoring703.4 Truncation723.5 Likelihood Construction for Censored and Truncated Data743.6 Counting Processes793.7 Exercises87
ContentsChapter 4 — Nonparametric Estimation of Basic Quantities forRight-Censored and Left-Truncated Dataxi914.1 Introduction914.2 Estimators of the Survival and Cumulative HazardFunctions for Right-Censored Data924.3 Pointwise Confidence Intervals for the Survival Function1044.4 Confidence Bands for the Survival Function1094.5 Point and Interval Estimates of the Mean andMedian Survival Time1174.6 Estimators of the Survival Function for Left-Truncatedand Right-Censored Data1234.7 Summary Curves for Competing Risks1274.8 Exercises133Chapter 5 — Estimation of Basic Quantities forOther Sampling Schemes1395.1 Introduction1395.2 Estimation of the Survival Function for Left, Double,and Interval Censoring1405.3 Estimation of the Survival Function forRight-Truncated Data1495.4 Estimation of Survival in the Cohort Life Table1525.5 Exercises158Chapter 6 — Topics in Univariate Estimation1656.1 Introduction1656.2 Estimating the Hazard Function1666.3 Estimation of Excess Mortality1776.4 Bayesian Nonparametric Methods1876.5 Exercises198
xiiContentsChapter 7 — Hypothesis Testing2017.1 Introduction2017.2 One-Sample Tests2027.3 Tests for Two or More Samples2057.4 Tests for Trend2167.5 Stratified Tests2197.6 Renyi Type Tests2237.7 Other Two-Sample Tests2277.8 Test Based on Differences in Outcome at aFixed Point in Time2347.9 Exercises238Chapter 8 — Semiparametric Proportional Hazards Regressionwith Fixed Covariates2438.1 Introduction2438.2 Coding Covariates2468.3 Partial Likelihoods for Distinct-Event Time Data2538.4 Partial Likelihoods When Ties Are Present2598.5 Local Tests2638.6 Discretizing a Continuous Covariate2728.7 Model Building Using the Proportional Hazards Model2768.8 Estimation of the Survival Function2838.9 Exercises287Chapter 9 — Refinements of the Semiparametric ProportionalHazards Model2959.1 Introduction2959.2 Time-Dependent Covariates2979.3 Stratified Proportional Hazards Models308
Contentsxiii9.4 Left Truncation3129.5 Synthesis of Time-varying Effects (Multistate Modeling)3149.6 Exercises326Chapter 10 — Additive Hazards Regression Models32910.1 Introduction32910.2 Aalen’s Nonparametric, Additive Hazard Model33010.3 Lin and Ying’s Additive Hazards Model34610.4 Exercises351Chapter 11 — Regression Diagnostics35311.1 Introduction35311.2 Cox–Snell Residuals for Assessing the Fit of a Cox Model35411.3 Determining the Functional Form of a Covariate:Martingale Residuals35911.4 Graphical Checks of the Proportional Hazards Assumption36311.5 Deviance Residuals38111.6 Checking the Influence of Individual Observations38511.7 Exercises391Chapter 12 — Inference for Parametric Regression Models39312.1 Introduction39312.2 Weibull Distribution39512.3 Log Logistic Distribution40112.4 Other Parametric Models40512.5 Diagnostic Methods for Parametric Models40912.6 Exercises419Chapter 13 — Multivariate Survival Analysis42513.1 Introduction42513.2 Score Test for Association427
xivContents13.3 Estimation for the Gamma Frailty Model43013.4 Marginal Model for Multivariate Survival43613.5 Exercises438Appendix A — Numerical Techniques for Maximization443A.1 Univariate Methods443A.2 Multivariate Methods445Appendix B — Large-Sample Tests Based on Likelihood Theory449Appendix C — Statistical Tables455C.1 Standard Normal Survival Function P [Z ⱖ z ]456C.2 Upper Percentiles of a Chi-Square Distribution457C.3a Confidence Coefficients c10 (aL , aU ) for 90% EPConfidence Bands459C.3b Confidence Coefficients c05 (aL , aU ) for 95% EPConfidence Bands463C.3c Confidence Coefficients c01 (aL , aU ) for 99% EPConfidence Bands465C.4a Confidence Coefficients k 10 (aL , aU ) for 90% Hall–WellnerConfidence Bands468C.4b Confidence Coefficients k 05 (aL , aU ) for 95% Hall–WellnerConfidence Bands471C.4c Confidence Coefficients k 01 (aL , aU ) for 99% Hall–WellnerConfidence Bands474C.5 Survival Function of the Supremum of the AbsoluteValue of a Standard Brownian Motion Process overthe Range 0 to 1477C.6 Survival Function of W 0 [B(t)]2 dt, where B(t) isa Standard Brownian Motion478C.7 Upper Percentiles of R is a Brownian Bridge479 k0 B o (u) du, where B o (u)
ContentsxvAppendix D — Data on 137 Bone Marrow Transplant Patients483Appendix E — Selected Solutions to Exercises489Bibliography515Author Index527Subject Index531
1Examples ofSurvival Data1.1 IntroductionThe problem of analyzing time to event data arises in a number ofapplied fields, such as medicine, biology, public health, epidemiology,engineering, economics, and demography. Although the statistical toolswe shall present are applicable to all these disciplines, our focus is onapplying the techniques to biology and medicine. In this chapter, wepresent some examples drawn from these fields that are used throughout the text to illustrate the statistical techniques we shall describe.A common feature of these data sets is they contain either censoredor truncated observations. Censored data arises when an individual’slife length is known to occur only in a certain period of time. Possiblecensoring schemes are right censoring, where all that is known is thatthe individual is still alive at a given time, left censoring when all that isknown is that the individual has experienced the event of interest priorto the start of the study, or interval censoring, where the only information is that the event occurs within some interval. Truncation schemesare left truncation, where only individuals who survive a sufficient timeare included in the sample and right truncation, where only individualswho have experienced the event by a specified time are included inthe sample. The issues of censoring and truncation are defined morecarefully in Chapter 3.1
2Chapter 1 Examples of Survival Data1.2 Remission Duration from a Clinical Trialfor Acute LeukemiaFreireich et al. (1963) report the results of a clinical trial of a drug6-mercaptopurine (6-MP) versus a placebo in 42 children with acuteleukemia. The trial was conducted at 11 American hospitals. Patientswere selected who had a complete or partial remission of their leukemiainduced by treatment with the drug prednisone. (A complete or partialremission means that either most or all signs of disease had disappearedfrom the bone marrow.) The trial was conducted by matching pairs ofpatients at a given hospital by remission status (complete or partial) andrandomizing within the pair to either a 6-MP or placebo maintenancetherapy. Patients were followed until their leukemia returned (relapse)or until the end of the study (in months). The data is reported inTable 1.1.TABLE 1.1Remission duration of 6 -MP versus placebo in children with acute leukemiaPairRemission Status atRandomizationTime to Relapse forPlacebo PatientsTime to Relapse for6 -MP Patients123456789101112131415161718192021Partial RemissionComplete RemissionComplete RemissionComplete RemissionComplete RemissionPartial RemissionComplete RemissionComplete RemissionComplete RemissionComplete RemissionComplete RemissionPartial RemissionComplete RemissionComplete RemissionComplete RemissionPartial RemissionPartial RemissionComplete RemissionComplete RemissionComplete RemissionComplete Remission1223128172118122541582351141810732 232261634 32 25 11 20 19 617 35 6139 6 10 Censoredobservation
1.3 Bone Marrow Transplantation for Leukemia3This data set is used in Chapter 4 to illustrate the calculation ofthe estimated probability of survival using the product-limit estimator,the calculation of the Nelson-Aalen estimator of the cumulative hazardfunction, and the calculation of the mean survival time, along with theirstandard errors. It is further used in section 6.4 to estimate the survivalfunction using Bayesian approaches. Matched pairs tests for differencesin treatment efficacy are performed using the stratified log rank testin section 7.5 and the stratified proportional hazards model in section9.3.1.3 Bone Marrow Transplantation for LeukemiaBone marrow transplants are a standard treatment for acute leukemia.Recovery following bone marrow transplantation is a complex process.Prognosis for recovery may depend on risk factors known at the timeof transplantation, such as patient and/or donor age and sex, the stageof initial disease, the time from diagnosis to transplantation, etc. Thefinal prognosis may change as the patient’s posttransplantation historydevelops with the occurrence of events at random times during therecovery process, such as development of acute or chronic graft-versushost disease (GVHD), return of the platelet count to normal levels,return of granulocytes to normal levels, or development of infections.Transplantation can be considered a failure when a patient’s leukemiareturns (relapse) or when he or she dies while in remission (treatmentrelated death).Figure 1.1 shows a simplified diagram of a patient’s recovery processbased on two intermediate events that may occur in the recovery process. These intermediate events are the possible development of acuteGVHD that typically occurs within the first 100 days following transplantation and the recovery of the platelet count to a self-sustaininglevel ⱖ 40 109 l (called platelet recovery in the sequel). Immediatelyfollowing transplantation, patients have depressed platelet counts andare free of acute GVHD. At some point, they may develop acute GVHDor have their platelets recover at which time their prognosis (probabilities of treatment related death or relapse at some future time) maychange. These events may occur in any order, or a patient may dieor relapse without any of these events occurring. Patients may, then,experience the other event, which again modifies their prognosis, orthey may die or relapse.To illustrate this process we consider a multicenter trial of patientsprepared for transplantation with a radiation-free conditioning regimen.
4Chapter 1 Examples of Survival Dataplateletrecoveryacute gvhdtransplantrelapsedeathacute gvhdplateletrecoveryFigure 1.1 Recovery Process from a Bone Marrow TransplantDetails of the study are found in Copelan et al. (1991). The preparativeregimen used in this study of allogeneic marrow transplants for patientswith acute myeloctic leukemia (AML) and acute lymphoblastic leukemia(ALL) was a combination of 16 mg/kg of oral Busulfan (BU) and 120mg/kg of intravenous cyclophosphamide (Cy). A total of 137 patients(99 AML, 38 ALL) were treated at one of four hospitals: 76 at TheOhio State University Hospitals (OSU) in Columbus; 21 at HahnemannUniversity (HU) in Philadelphia; 23 at St. Vincent’s Hospital (SVH) inSydney Australia; and 17 at Alfred Hospital (AH) in Melbourne. Thestudy consists of transplants conducted at these institutions from March1, 1984, to June 30, 1989. The maximum follow-up was 7 years. Therewere 42 patients who relapsed and 41 who died while in remission.Twenty-six patients had an episode of acute GVHD, and 17 patients
1.3 Bone Marrow Transplantation for Leukemia5either relapsed or died in remission without their platelets returning tonormal levels.Several potential risk factors were measured at the time of transplantation. For each disease, patients were grouped into risk categoriesbased on their status at the time of transplantation. These categorieswere as follows: ALL (38 patients), AML low-risk first remission (54 patients), and AML high-risk second remission or untreated first relapse(15 patients) or second or greater relapse or never in remission (30patients). Other risk factors measured at the time of transplantationincluded recipient and donor gender (80 and 88 males respectively),recipient and donor cytomegalovirus immune status (CMV) status (68and 58 positive, respectively), recipient and donor age (ranges 7–52and 2–56, respectively), waiting time from diagnosis to transplantation(range 0.8–87.2 months, mean 19.7 months), and, for AML patients,their French-American-British (FAB) classification based on standardmorphological criteria. AML patients with an FAB classification of M4 orM5 (45/99 patients) were considered to have a possible elevated risk ofrelapse or treatment-related death. Finally, patients at the two hospitalsin Australia (SVH and AH) were given a graft-versus-host prophylacticcombining methotrexate (MTX) with cyclosporine and possibly methylprednisolone. Patients at the other hospitals were not given methotrexate but rather a combination of cyclosporine and methylprednisolone.The data is presented in Table D.1 of Appendix D.This data set is used throughout the book to illustrate the methodspresented. In Chapter 4, it is used to illustrate the product-limit estimator of the survival function and the Nelson–Aalen estimator of thecumulative hazard rate of treatment failure. Based on these statistics,pointwise confidence intervals and confidence bands for the survivalfunction are constructed. The data is also used to illustrate point andinterval estimation of summary survival parameters, such as the meanand median time to treatment failure in this chapter.This data set is also used in Chapter 4 to illustrate summary probabilities for competing risks. The competing risks, where the occurrence ofone event precludes the occurrence of the other event, in this example,are relapse and death.In section 6.2, the data set is used to illustrate the construction ofestimates of the hazard rate. These estimates are based on smoothingthe crude estimates of the hazard rate obtained from the jumps of theNelson–Aalen estimator found in Chapter 4 using a weighted averageof these estimates in a small interval about the time of interest. Theweights are chosen using a kernel weighting function.In Chapter 7, this data is used to illustrate tests for the equality of Ksurvival curves. Both stratified and unstratified tests are discussed.In Chapter 8, the data is used to illustrate tests of the equality of Khazard rates adjusted for possible fixed-time confounders. A proportional hazards model is used to make this adjustment. Model buildingfor this problem is illustrated. In Chapter 9, the models found in Chap-
6Chapter 1 Examples of Survival Datater 8 are further refined to include covariates, whose values changeover time, and to allow for stratified regression models. In Chapter 11,regression diagnostics for these models are presented.1.4 Times to Infection of KidneyDialysis PatientsIn a study (Nahman et al., 1992) designed to assess the time to first exitsite infection (in months) in patients with renal insufficiency, 43 patientsutilized a surgically placed catheter (Group 1), and 76 patients utilizeda percutaneous placement of their catheter (Group 2). Cutaneous exitsite infection was defined as a painful cutaneous exit site and positivecultures, or peritonitis, defined as a presence of clinical symptoms,elevated peritoneal dialytic fluid, elevated white blood cell count (100white blood cells l with 50% neutrophils), and positive peritonealdialytic fluid cultures. The data appears in Table 1.2.TABLE 1.2Times to infection (in months) of kidney dialysis patients with different catheterization proceduresSurgically Placed CatheterInfection Times: 1.5, 3.5, 4.5, 4.5, 5.5, 8.5, 8.5, 9.5, 10.5, 11.5, 15.5, 16.5, 18.5, 23.5, 26.5Censored Observations: 2.5, 2.5, 3.5, 3.5, 3.5, 4.5, 5.5, 6.5, 6.5, 7.5, 7.5, 7.5, 7.5, 8.5, 9.5,10.5, 11.5, 12.5, 12.5, 13.5, 14.5, 14.5, 21.5, 21.5, 22.5, 22.5, 25.5, 27.5Percutaneous Placed CatheterInfection Times: 0.5, 0.5, 0.5, 0.5, 0.5, 0.5, 2.5, 2.5, 3.5, 6.5, 15.5Censored Observations: 0.5, 0.5, 0.5, 0.5, 0.5, 0.5, 0.5, 0.5, 0.5, 0.5, 1.5, 1.5, 1.5, 1.5, 2.5,2.5, 2.5, 2.5, 2.5, 3.5, 3.5, 3.5, 3.5, 3.5, 4.5, 4.5, 4.5, 5.5, 5.5, 5.5, 5.5, 5.5, 6.5, 7.5, 7.5,7.5, 8.5, 8.5, 8.5, 9.5, 9.5, 10.5, 10.5, 10.5, 11.5, 11.5, 12.5, 12.5, 12.5, 12.5, 14.5, 14.5,16.5, 16.5, 18.5, 19.5, 19.5, 19.5, 20.5, 22.5, 24.5, 25.5, 26.5, 26.5, 28.5The data is used in section 7.3 to illustrate how the inference about theequality of two survival curves, based on a two-sample weighted, logrank test, depends on the choice of the weight function. In section 7.7, itis used to illustrate the two-sample Cramer–von Mises test for censoreddata. In the context of the proportional hazards model, this data isused in Chapter 8 to illustrate the different methods of constructing thepartial likelihoods and the subsequent testing of equality of the survival
1.5 Times to Death for a Breast-Cancer Trial7curves when there are ties present. Testing for proportional hazards isillustrated in section 9.2. The test reveals that a proport
tics based on censored and/or truncated data. Chapter 4 discusses es-timation of the survival function, the cumulative hazard rate, and mea-suresofcentralitysuch asthemedianandthemean.Theconstruction of pointwise confidence intervals and confidence bands is presented. Here we focus on right censored as well as left truncated survival data since