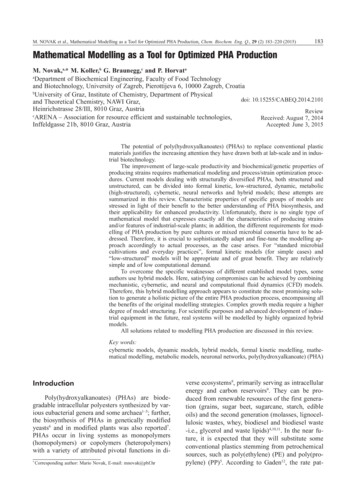
Transcription
M. NOVAK et al., Mathematical Modelling as a Tool for Optimized PHA Production, Chem. Biochem. Eng. Q., 29 (2) 183–220 (2015)183Mathematical Modelling as a Tool for Optimized PHA ProductionM. Novak,a,* M. Koller,b G. Braunegg,c and P. HorvataDepartment of Biochemical Engineering, Faculty of Food Technologyand Biotechnology, University of Zagreb, Pierottijeva 6, 10000 Zagreb, CroatiabUniversity of Graz, Institute of Chemistry, Department of Physicaldoi: 10.15255/CABEQ.2014.2101and Theoretical Chemistry, NAWI Graz,Heinrichstrasse 28/III, 8010 Graz, AustriaReviewcARENA – Association for resource efficient and sustainable technologies,Received: August 7, 2014Inffeldgasse 21b, 8010 Graz, AustriaAccepted: June 3, 2015aThe potential of poly(hydroxyalkanoates) (PHAs) to replace conventional plasticmaterials justifies the increasing attention they have drawn both at lab-scale and in industrial biotechnology.The improvement of large-scale productivity and biochemical/genetic properties ofproducing strains requires mathematical modeling and process/strain optimization procedures. Current models dealing with structurally diversified PHAs, both structured andunstructured, can be divided into formal kinetic, low-structured, dynamic, metabolic(high-structured), cybernetic, neural networks and hybrid models; these attempts aresummarized in this review. Characteristic properties of specific groups of models arestressed in light of their benefit to the better understanding of PHA biosynthesis, andtheir applicability for enhanced productivity. Unfortunately, there is no single type ofmathematical model that expresses exactly all the characteristics of producing strainsand/or features of industrial-scale plants; in addition, the different requirements for modelling of PHA production by pure cultures or mixed microbial consortia have to be addressed. Therefore, it is crucial to sophisticatedly adapt and fine-tune the modelling approach accordingly to actual processes, as the case arises. For “standard microbialcultivations and everyday practices”, formal kinetic models (for simple cases) and“low-structured” models will be appropriate and of great benefit. They are relativelysimple and of low computational demand.To overcome the specific weaknesses of different established model types, someauthors use hybrid models. Here, satisfying compromises can be achieved by combiningmechanistic, cybernetic, and neural and computational fluid dynamics (CFD) models.Therefore, this hybrid modelling approach appears to constitute the most promising solution to generate a holistic picture of the entire PHA production process, encompassing allthe benefits of the original modelling strategies. Complex growth media require a higherdegree of model structuring. For scientific purposes and advanced development of industrial equipment in the future, real systems will be modelled by highly organized hybridmodels.All solutions related to modelling PHA production are discussed in this review.Key words:cybernetic models, dynamic models, hybrid models, formal kinetic modelling, mathematical modelling, metabolic models, neuronal networks, poly(hydroxyalkanoate) (PHA)IntroductionPoly(hydroxyalkanoates) (PHAs) are biodegradable intracellular polyesters synthesized by various eubacterial genera and some archaea1–5; further,the biosynthesis of PHAs in genetically modifiedyeasts6 and in modified plants was also reported7.PHAs occur in living systems as monopolymers(homopolymers) or copolymers (heteropolymers)with a variety of attributed pivotal functions in di*Corresponding author: Mario Novak, E-mail: mnovak@pbf.hrverse ecosystems8, primarily serving as intracellularenergy and carbon reservoirs9. They can be produced from renewable resources of the first generation (grains, sugar beet, sugarcane, starch, edibleoils) and the second generation (molasses, lignocellulosic wastes, whey, biodiesel and biodiesel waste-i.e., glycerol and waste lipids)4,10,11. In the near future, it is expected that they will substitute someconventional plastics stemming from petrochemicalsources, such as poly(ethylene) (PE) and poly(propylene) (PP)5. According to Gaden12, the rate pat-
184M. NOVAK et al., Mathematical Modelling as a Tool for Optimized PHA Production, Chem. Biochem. Eng. Q., 29 (2) 183–220 (2015)terns of product formation enclose three basic typesin various bioprocesses:(1) growth-associated products arising directlyfrom the energy metabolism of carbohydrates;(2) indirect products of carbohydrate metabolism;(3) products apparently unrelated to carbohydrate oxidation.The aforementioned was studied for excretableproducts produced from carbohydrates. This wassubsequently confirmed as valid for other types ofC-sources (substrates related to the energy production and cell components synthesis). AlthoughPHAs are intracellular (insoluble in cytosol) storagepolymers, Gaden’s types of kinetic behaviours can,under certain assumptions (circumstances) be applied to these compounds.Regarding the kinetics of microbial growth andPHA production separately, three different types ofmicrobial producers of PHAs can be distinguished,as follows:a) Strains displaying strict separation betweenthe biomass growth phase and the PHA productionphase normally provoked by N or P limitation (prototype organisms: Pseudomonas sp. 2F, Methylomonas extorquens)13.b) Strains that accumulate certain quantities ofPHA already under balanced nutritional conditions;also here, in the non-growth phase (usually provoked by N or P limitation), amplified PHA accumulation is observed (prototype organism: Cupriavidus necator).c) Strains displaying high PHA formation ratesduring growth phase without limitation of an essential growth component2,14–16 (prototype organisms:Azohydromonas lata DSM 1122, or Pseudomonasputida GPo1 ATTC 29347).Based on the different kinetic properties of theproduction strains, different cultivation techniqueshave been applied in the past for their cultivation:batch, fed batch, repeated batch, repeated fed batch,or one-, two-, and multi-stage continuous processes17; both pure and mixed microbial cultures(MMCs) where used. For example, microorganismsbelonging to the mentioned groups (a) and (b) arewell suited for two-stage continuous cultivation thatrequires extensive biomass growth in the first stage,followed by non-growth-associated PHA synthesisin the second stage18–24. As a novel approach, Atlićet al.25 have recently tested a five-stage continuousprocess in a bioreactor cascade, specially designedto achieve different process conditions (concentrations of substrates and co-substrates, temperature,pH-value, dissolved oxygen [DO]) in each step ofthe cascade. From the point of view of such application, this cascade set-up was designed to act as atool for the designing of novel biopolymers, e.g.,blocky structured polymers with alternating “soft”and “hard” segments, or polymers displaying controlled molecular mass and polydispersity of molecular mass distribution.Regarding the type of polymers, substrates,strains (wild types or genetically modified organisms), cultivation procedures and techniques, aswell as the downstream processing systems forproduct recovery, numerous experiments were performed in the past in order to optimize PHA production both quantitatively and qualitatively.Mathematical models are useful tools for optimizing and controlling microbial product formationand microbial metabolism, encompassing the modeling of cultivation techniques, the design of singlecell metabolic models, or modelling of whole cellpopulations26–29. The segregated nature of biologicalsystems and the complexity of cell reactions arecumbersome for mathematical treatment of processes in the bioengineering field. In addition, linkingexperimental data with mathematical modeling canreveal new aspects of microbial physiology, providing reasonable interpretations of results from experimental work. This way, the improvement of knowledge as well as the designing of new, morecall-oriented experiments, can be achieved30,31.Model theory classifies models into severalclasses and its antipodes: verbal/non-verbal, descrip tive/explanatory, black-/grey-/white-box, unstructured/structured, non-mathematical /mathematical,deterministic/stochastic, discrete/continuous, anddistributed (non-segregated)/segregated. The derived combinations of the mentioned model classescan be further grouped into logistic, kinetic, dynamic and cybernetic models. Usually, a specific modeldeveloped from a biological situation observed inliving systems can belong to more than one class.For example, dynamic models can at the same timebe structured, mathematical, continuous, and segregated. Kinetic models include two basic subcategories: They can be unstructured or structured. In literature, unstructured kinetic models are known asformal kinetic (or mechanistic) models; structuredkinetic models can be divided into subclasses: morphological, compartmental, metabolic, and chemically related. Due to the plurality of microbial producers, the vast number of cultivation strategies,substrates, and products, and the existing broadspectrum of available models, a great number of interconnections (combinations) is conceivable.Therefore, very different model types have been applied to describe miscellaneous biotechnologicalsituations regarding cultivation techniques, masstransfer, microbial growth kinetics, and metabolicnetwork reaction kinetics. In the light of the aforementioned, it is practically impossible to discover a
M. NOVAK et al., Mathematical Modelling as a Tool for Optimized PHA Production, Chem. Biochem. Eng. Q., 29 (2) 183–220 (2015)conceivable model type in the literature that has notyet been applied to describe PHA biosynthesis.Such highly extensive, comprehensive, and sophisticated material is difficult to systematize in order toachieve the desired and urgently needed overviewfor the relevant scientific community, and reachdeeper insight into the essence of matter. Therefore,the aim of this work was to provide the respectedreaders with a comprehensive and critical synopsisof mathematical models that were applied for themicrobial synthesis of PHA, together with the related cultivation strategies. Thereby, special attentionis dedicated to the usefulness of applied models forimprovement of processes, product yields, downstream processing, strains, and knowledge of themetabolic network.Modeling approachesFormal kinetic (unstructuredand low-structured) modelsIn the early phase of mathematical modeling ofPHA biosynthesis, mainly formal kinetic unstructured, so-called “black box type”, models were introduced32–36. These early works focused on the kinetic relationships between substrate (S), product(P) and biomass (X); hence, at the beginning, theoptimization of the bioprocess itself was not themain purpose of modelling. At that time, microorganisms were cultivated by well-established standard microbiological techniques: Microbial brothusually contained the C-source as the growth-limiting substrate, whereas all other constituents werepresent in excess. For this type of cultivation, thestandard differential mass balance equations forbiomass (X), limiting substrate (S) and product (P;here: PHA) were established by applying the Monodequation for linking the substrate concentration tothe specific growth rate. An application of appropriate yield and/or stoichiometric coefficients (biomassto substrate and product to substrate) was the common tool in these works. Such approaches were especially applicable for the growth-associated „(c)“type of PHA producers as described above. Thereader can find an overview of these early works inthe literature37–38. Soon after, these early mathematical models for PHA synthesis were corrected thanksto the works of Sonnleitner et al.32 and Heinzle andLafferty34, who developed the “two main compartments” strategy, which is applicable and still validtoday. As the PHA mass fraction can account for thepredominant part with mass fractions exceeding 0.9of the whole biomass (X), the latter was structuredby the mentioned authors into two components:(1) The catalytically active fraction consistingof membrane- and cytosolic proteins (structural185proteins and catalytic enzymes), glycolipids, phospholipids, glycoproteins and nucleic acids (jointlyknown in literature as “residual biomass”, i.e., thebiologically active part Xr).(2) The biopolymeric storage material (PHA)as the intracellular, catalytically inert fraction ofbiomass.In addition, for some organisms it was observedthat both the nitrogen (N) and phosphorus (P) sources act as limiting substrates, mainly triggeringgrowth and product kinetics. So, N- and/or P-sourcelimitation in the late phase of cultivation was postulated as the inductive triggering factor that provokes(or enhances) PHA synthesis; this is especially validfor the abovementioned producer types “(a)” and“(b)”. By assuming that the rate of product formation is related both to the rate of growth and to theconcentration of the biologically active part of biomass (Xr), a reasonably good correlation was obtained between experimental data and the modelvalues. For this purpose, the Luedeking-Piret equation 39 was applied to describe product formation.Introducing growth-limiting substrates other thanC-source (i.e., N- and/or P-sources) had certain effect on the mathematical expressions for specificgrowth and specific PHA production rate. In suchcases, the specific growth rate of biomass (µ) wasexpressed using double-substrate Monod relationsaccording to Megee et al.40. Regarding broth composition, this was extended to the multi-substrateMonod relation for more complicated mediums(containing complex substrates or mixture of different C- or N-sources). In some cases, the logisticequation was applied for the mathematical description of cell growth41. Unfortunately, these improvements were not the only changes needed in the kinetic modelling to ach
PHA production separately, three different types of microbial producers of PHAs can be distinguished, as follows: a) Strains displaying strict separation between the biomass growth phase and the PHA production phase normally provoked by N or P limitation (pro-totype organisms: Pseudomonas sp. 2F, Methylo-monas extorquens)13.