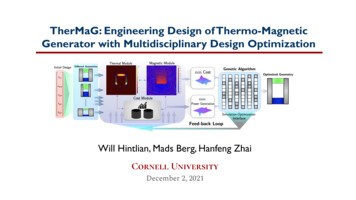
Transcription
TherMaG: Engineering Design of Thermo-MagneticGenerator with Multidisciplinary Design OptimizationWill Hintlian, Mads Berg, Hanfeng ZhaiCornell UniversityDecember 2, 2021
CONTENT Background & Motivation Single-objective Optimization Project Description Multi-objective Optimization Problem Formulation Recommendations Modeling & Simulation Summary & Future works
Background & MotivationSource: NBC NewsSource: WikipediaKishore and Priya, Renew. Sust. Ener. Rev., 2021Ahmed et al., Int. J. Ener. Res., 2021Source: ForbesSource: Google Patent
Project Description PROBLEM: Design of Thermo-Magnetic Generator Consists of active materials, yoke, permanent magnet Generate energy from temperature induced magnetic fieldchangeGOAL: Provide insights for NextGen clean energy Numerous research addressed on electrochemical, hydrogen,nuclear, and other forms of clean energiesImage acquired and reproduced from Wikimedia commons and comsol.com Very few tackles possible applications of TMGMETHOD: Utilize the powerof numerical simulation Black box code Platform for connectingcommercial softwares Optimization toolbox in MATLABImage acquired and reproduced from Waske et al., Nat. Ener., 2018
Problem Formulation Objective Design vectorConstraintsParametersDesign VariablesModulesDescriptionLower BoundsNominalUpper BoundsTherm., Magn., CostYoke Width0.010.050.5Therm., Magn., CostYoke Height0.010.40.5Therm., Magn., CostActive Material Height0.010.10.5Therm., Magn., CostPermanent Magnet Height0.010.10.5Therm., Magn., CostGap Width0.010.150.5
Problem Formulation Constraints-Effect of ConstraintsTypeMaximum device heightInequality ConstraintMaximum device widthInequality ConstraintMaximum device volumeInequality ConstraintNo overlapInequality ConstraintNo equality constraintsBoundParametersItemPhysical propertiesUnitValueMaterial of Active MaterialMagnetic permeabilities, thermal diffusivity, heat capacity, price[H/m], [m 2/s], [J/(kg*K)],USD/m 2(4 Pi 10 -7, 80 Pi 10 -7),built-in,1.7e5Material of Permanent MagnetMagnetic permeabilities, thermal diffusivity, heat capacity, rem. flux density,price[H/m], [m 2/s], [J/(kg*K)],[T], USD/m 2built-in, built-in,built-in, 1.3,1.4e3Material of YokeMagnetic permeabilities, thermal diffusivity, heat capacity, price[H/m], [m 2/s], [J/(kg*K)],[T], USD/m 2built-in, built-in, built-in, 1.63e5Ambient conditionsTemperature, magnetic permeability[K], [H/m]300, 4 Pi, 10 -7
Physical Modeling Total power outputProportionality constantTimeMagnetic flux Energy efficiency of the TMG systemProportionality constantHeat capacityTemperature
Modeling & SimulationImage acquired and reproduced from Waske et al., Nat. Ener., 2018Disciplines: 3Run time: 10s-5min
Model ValidationCredit: Waske et al., Nat. Ener., 2018
Single-objective Optimization: Design of ExperimentsLower bounds: 0.05 mUpper bounds: 0.45 m
Single-objective Optimization: Design of Experiments
Single-objective Optimization: Gradient AlgorithmsUpper bounds: 0.01 mLower bounds: 0.5 m
Single-objective Optimization: Gradient AlgorithmsOptimization completed: The relative first-order optimality measure, 1.426149e-10,is less than options.OptimalityTolerance 1.000000e-06, and the relative maximum constraintviolation, 0.000000e 00, is less than options.ConstraintTolerance 1.000000e-06.
Single-objective Optimization: Genetic Algorithms% set optimization optionsfuncTol 1e-4;conTol 1e-4;popSize 50;eliteCount ceil(.05*popSize);crossoverRatio 1.2;crossoverFraction .8;maxStallGens 4;Lower bounds: 0.01 mUpper bounds: 0.5 m
Single-objective Optimization: Genetic Algorithms
Comparing the DoE and Full Optimizations:
Multiobjective OptimizationUtopia pointCostPower outputEfficiency 166.35209220.71531650.3051% set optimization optionsfuncTol 1e-4;conTol 1e-5;popSize 100;crossoverRatio 1.2;crossoverFraction .8;maxStallGenerations 2;
Multiobjective Optimization: Walking the Pareto Front
Final Recommendations: 3 Objective OptimizationUtopia pointFinal suggested designNon-dominated point
Final Recommendations: 3 Objective Optimization
Summary & Future WorksSummary & Takeaways Gradient-based methods aremathematically more rigorous andconsumes less computational resourcesHeuristic methods are handy andpowerful for some black box simulationsand general engineering applicationsEach of us has gotten a taste of applyingMDO algorithms to engineeringproblems and hopes to use them moreWe leave the course armed with toolsand knowledge to begin applying MDOtechniques after graduation!Next Steps Implementing meshing of the TMG asdesign variables will provide a morecomprehensive geometric designInclude modules for fluid mechanics, wirecoils, pumps, etc. for a morecomprehensive system modelBuild up 3D simulation model for TMGdesign and optimizationConsideration of different materialspropertiesManufacture the TMG in a lab
Q &A
TherMaG: Engineering Design of Thermo-Magnetic Generator with Multidisciplinary Design Optimization Will Hintlian, Mads Berg, Hanfeng Zhai Cornell University December 2, 2021. . Design Variables Modules Description Lower Bounds Nominal Upper Bounds Therm., Magn., Cost Yoke Width 0.01 0.05 0.5 Therm., Magn., Cost Yoke Height 0.01 0.4 0.5 .