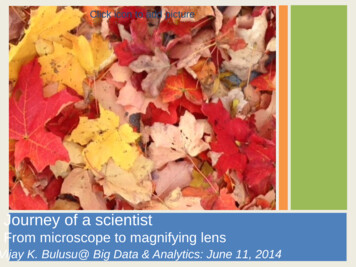
Transcription
Click icon to add pictureJourney of a scientistFrom microscope to magnifying lensVijay K. Bulusu@ Big Data & Analytics: June 11, 2014
Vijay K. BulusuDirector, Informatics & InnovationPharmaceutical SciencesWorldwide Research & .linkedin.com/in/vijaybulusu/
BIG DataorLots of SMALL Data(What?)
Drivers of change Deep understanding of pathwaysPrecision MedicineIncreased Patient StratificationBreakthrough TherapiesAdaptive clinical trialsChanging workforceEmerging Markets
Strategic Inflection Point: The inverted pyramidCollect dataPrepare reportsData drivenDecision Making20%Decision Making50%30%Analysis & Insight30%50%Data Collection & Reporting20%From Information Collection to DecisionMaking
Data growth in Pharma R&D4000 GBVELOCITYPer dayVARIETY 1000 instrument typesSCALABILITY DifficultVOLUME
Contextual Information Lots of data being collected Lower than the scale of other industries‘Volume’ and ‘Velocity’ are manageable‘Variety’ and ‘Scalability’ are applicableBIG Data is not necessarily our problemUsers need to visualize information inan intuitive way specific to the contextof their questions
Information is knowledge End-to-end linking of data across theProduct ostLaunchCommon Information Access LayerDon’t boil the ocean; Develop Use Cases
What is the solution? Semantic technologiesCloudBig DataAnalyticsEnterprise Service BusData Warehouse / Data MartText Mining / NLPThese are just tools. Before any of thesecan work, something more fundamentalis needed.
Fundamental pre-requisite: Data Standards
Benefits Level playing field between informationproducers and information consumers Common understanding and languageacross multiple groups Rapid identification of gaps in data quality
But, there are challenges Technical– Automated collection of scientific data– Preserve data for reuse– What identifiers should we use acrosssystems?– How do we move data between systems? Cultural– Enforce top-down adherence to standards– “What’s in it for me?”– Reward adoption of standards
Make every employeea data scientist(Who?)
Lab: Materials and NotebooksSource: commons.wikimedia.org
Experimentation: More materials; More techniques
Analysis: Becoming more complicated anddiversified
Observations: Electronic Notebooks
The changing role of a scientist Moving from a paper based environmentto a completely digital environment thatrequires new skills– Data processing– Statistics– Analysis
The changing role of a scientistLab ScientistData ScientistFROMTO
Problem & Response Problem– Lab scientists need to do science; notmanage data– Mixed response to the changing role Response– Create dedicated data scientists in theorganization who can work with lab scientists– Develop point solutions based onrequirements
Challenges Lead time between identification of needsand solution deployment Needs change over time; solutions notflexible enough Distinction between lab scientist and datascientist
Information to Insights(How?)
Data on tapMake data and integrated analysis toolsavailable to every employee in a selfservice mode
Triangle of InformationDATAePEOPLErib Who has worked onthis kind of problem? Who are the expertsin this space? Where is the data stored? Is the data computationallyaccessible?scdegenerateTypical informationaggregation solutions donot cover all threeAdd then there isdata external tothe organizationcreate What documentswere used to solvethis problem? Where can I findthese documents?UMCDOTSNE
Why is Data Access challenging? In order to search and explore our growing pool of information, usersneed to know ALL of the following:1. What system contains the data on the entity of interest?2. What is required for access to the system?3. How do I navigate in the system to find the data?4. How do I search accurately in the system?5. Do I have the correct name or ID used for that "entity" in thesystem?6. Can I trust the results from the system (Data Quality)? Information gathering activities tend to fail at #1It is hard to keep up with all of the systems and databases both insideand outside of an organizationWe revert to our people network for findinganswers
Intelligent Data FrameworkIDF aims to build a robust, foundational information architecture and deliver access tointernal and external information in an intuitive, self-service manner allowing efficientknowledge sharing. Data CaptureDataCapture Current: Raw data in many different places Desired: Data readily available for reuse withaligned metadata Data aAccess Current: Data called many different things Desired: Data becomes searchable as entitiesand are called by the same name across allsystems Data Access Current: Searching data across multiple systems;labor intensive manual process Desired: Tools are developed that provide intuitiveaccess to information, while hiding the underlyingcomplexity of assembling it across many sources
Intelligent Data Framework Data Capture– Lab systems and software Data Quality– J2EE– Vocabulary Services Data Access– HTML 5– Linked Data– Hadoop– Text Mining
Intelligent Data FrameworkAn easy-to-use, intuitive, semantic browserthat can bridge data standards acrossinternal and external partners to allow anyemployee to view and link data, documentsand people across internal and externalsources in a self-service mode
Intelligent Data Framework An industry-leading, best-in-class, dataframework where the end user canaccess and aggregate information fromany of the following data sources– Document Repositories (SharePoint,Documentum etc.)– Relational Databases (Oracle etc.)– Web Services– External sources on the Web (PubMed etc.)
Intelligent Data Framework Define and implement data standardsacross systems Built-in hypothesis generation Ability to overlay “new” contextualinformation over existing empiricalinformation
Intelligent Data Framework Ability to seamlessly navigate from onedataset and/or data source (documentrepositories, relational databases etc.) toanother without needing any specializedskills or previous knowledge of the data Collaborative development andexploration of entity relationships
Intelligent Data Framework Ability to save and share results ofexploration of entity relationships Ability to find connections between anytwo random datasets with no knowledgeof the underlying data structures Ability to run interface from multipleaccess devices (web browser, smartphones, iPads, tablet PCs etc.)
How IDF is helping Lab scientists can find historical dataeasily and not have to repeat work donepreviously Self-service model for data access andanalysis toolkit is driving increasedengagement Users identifying opportunities for dataintegration Breaking down ‘old’ silos Identifying patterns from linked datasets
Getting closer
Questions?
Vijay K. Bulusu@ Big Data & Analytics: June 11, 2014 . Vijay K. Bulusu . Product lifecycle Don't boil the ocean; Develop Use Cases Common Information Access Layer. What is the solution? Semantic technologies Cloud Big Data Analytics Enterprise Service Bus Data Warehouse / Data Mart Text Mining / NLP These are just tools