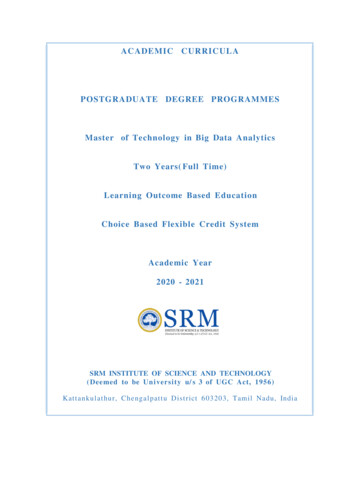
Transcription
ACADEMIC CURRICULAPOSTGRADUATE DEGREE PROGRAMMESMaster of Technology in Big Data AnalyticsTwo Years( Full Time)Learning Outcome Based EducationChoice Based Flexible Credit SystemAcademic Year2020 - 2021SRM INSTITUTE OF SCIENCE AND TECHNOLOGY( Deemed to be Uni versi ty u/ s 3 of UGC Act, 1956)Kat tankul at hur, Chengal pat tu District 603203, Tamil Nadu, India
M.Tech in Information TechnologyBig Data Analytics1.Department Vision StatementStmt - 1To develop the skills and knowledge to excel in their professional career in Information Technology and related disciplines.Stmt - 2To contribute and communicate effectively with the team to grow into leader.Stmt - 3To practice lifelong learning for continuing professional development.2.Department Mission StatementTo develop the ability to use and apply current technical concepts, skills, tools and practices in the core information technologyStmt - 1areas.Stmt - 2To develop the ability to identify and analyze user needs and take them into account in the selection, creation, evaluation andadministration of computer-based system.Stmt - 3To develop the ability to effectively integrate IT-based solutions into the user environment.3.Program Education Objectives (PEO)PEO - 1 Graduates involve in qualitative as well as quantitative techniques to improve business productivity and profits.PEO - 2Graduates will analyze the data efficiently with the current data analytics tools used by researchers, analysts, and engineers forbusiness organizations.PEO - 3Graduates will practice lifelong learning for continuing professional development.PEO - 4Graduates exploit the power of big data analytics those are in high demand as organizations are looking for.4.Consistency of PEO’s with Mission of the DepartmentMission Stmt. - 1Mission Stmt. - 2Mission Stmt. - 3Mission Stmt. - 4Mission Stmt. - 5PEO - 1HHHHHPEO - 2HHHHHPEO - 3HHMHHPEO - 4HHMHHHHHPEO - 5HHH – High Correlation, M – Medium Correlation, L – Low Correlation5.Consistency of PEO’s with Program Learning Outcomes (PLO)Program Learning Outcomes (PLO)Life Long Learning15.Leadership Skills14.ICT Skills13.Community Engagement12.Ethical Reasoning11.Multicultural Competence10.Self-Directed Learning9.Reflective Thinking8.Scientific Reasoning7.Team Work6.Research Skills5.Analytical Reasoning4.Problem Solving3.Critical Thinking2.Disciplinary Knowledge1.PEO - 1HHHHMMMHMMMLHMMPEO - 2HHHHMMMHMMMLHMMPEO - 3MLMMHLMHHMMLMLHPEO - 4HHHHMHMH – High Correlation, M – Medium Correlation, L – Low CorrelationHHHHHHHHSRM Institute of Science and Technology - Academic Curricula – (M.Tech Regulations 2020)1
6.Programme Structure (70 Total Credits)Professional Core Courses (C)(4 Courses)CourseCourseCodeTitleMultivariate techniques for data20ITC501Janalytics20ITC502J Computing for data analytics20ITC503J Big Data Technology20ITC504J Machine Learning for data analyticsTotal Learning CreditsOpen Elective Courses (O)(Any 1 Course)Hours/WeekL T PC3 0 243 0 23 0 23 0 0MBO602T20NTO601T20CEO531T20GNO620TTitleBusiness AnalyticsIndustrial SafetyOperations ResearchCost ManagementComposite MaterialsWaste to EnergyMOOCTotal Learning CreditsProfessional Elective Courses (E)(5 Courses)CourseCourseCodeTitle20ITE505J Cloud Computing for data analytics20ITE506J Advanced Algorithms AnalysisPython Programming for Data20ITE507JAnalyticsFunctional Programming for data20ITE508J analytics20ITE509J Marketing Analytics20ITE514T Risk Analytics20ITE510J Applied Social Network AnalysisNatural Language processing20ITE511Jtechniques20ITE611J Streaming Analytics20ITE612J Deep Learning for data analyticsTotal Learning CreditsHours/WeekL T PC3 0 2443 0 23 1 0443 0 243 0 2CodeCourseCourseHours/WeekL T PTitleResearch Publishing and Presenting20GNS501J Skills1 0 2Research Methods in Computer20CSS503J2 0 2SciencesTotal Learning CreditsTitleInternship (4-6 weeks during 2ndsem20ITP601Lvacation)20ITP602L Minor Project20ITP603L Project Work Phase I20ITP604L Project Work Phase IITotal Learning CreditsCourse2CourseCourse5---40 0 80 0 12 60 0 32 1626Hours/WeekL T P1 0 11 0 11 0 11 0 1C0000Hours/WeekL T PC1 0 101 0 101 0 10Mandatory Courses (M)(3 Courses)C3Hours/WeekL T P CAudit Courses (A)(Any 2 Courses)CodeTitle20CEA531J Disaster Management20GNA511T Constitution of India20GNA513J Value Education20GNA512L Physical and Mental Health using YogaSkill Enhancement Courses (S)(2 Courses)CourseCourse420C33333333Project Work, Internship InIndustry / Higher Technical Institutions(P)Code3 0 2Hours/WeekL T P3 0 03 0 03 0 03 0 03 0 03 0 0- - -CourseCodeTitleCareer Advancement Course for20PDM501TEngineers – ICareer Advancement Course for20PDM502TEngineers – IICareer Advancement Course for20PDM601TEngineers – III# Replace as appropriate i.e., Research Methods in Electrical Sciences / Mechanical Sciences etc.,SRM Institute of Science and Technology - Academic Curricula – (M.Tech Regulations 2020)2
8. Program Articulation MatrixSemester - 20ITE508J20GNS501J20PDM501TCourse TitleMultivariate techniques for dataanalyticsComputing for data analyticsCloud Computing for data analyticsAdvanced Algorithms AnalysisPython Programming for DataAnalyticsFunctional Programming for dataanalyticsResearch Publishing and PresentingSkillsCareer Advancement Course forEngineers – IAudit Course - ITotal Learning CreditsSemester - IIHours/WeekL T PC3 0 243 0 23 0 2443 0 241 0 221 0 101 0 10JBig Data TechnologyMachine Learning for data analyticsMarketing AnalyticsRisk AnalyticsApplied Social Network AnalysisNatural Language processing20ITE511JtechniquesResearch Methods in Computer20CSS503JSciencesCareer Advancement Course for20PDM502TEngineers – IIAudit Course - IITotal Learning CreditsSemester - IIICodeCourse TitleCourse TitleHours/WeekL T P3 0 23 0 23 0 23 1 0C44443 0 242 0 231 0 101 0 1019Semester - IVHours/WeekL T P3 0 220ITE611J Streaming Analytics20ITE612J Deep Learning for data analyticsOpen Elective3 0 020GNO620T MOOC- - Internship (4-6 weeks during 2ndSem20ITP601L- - vacation)20ITP602L Minor Project0 0 820ITP603L Project Work Phase I0 0 12Career Advancement Course for20PDM601T1 0 1Engineers – IIITotal Learning CreditsH – High Correlation, M – Medium Correlation, L – Low CorrelationC4Hours/WeekCL T P20ITP604L Project Work Phase II0 0 32 16Total Learning Credits16CodeCourse Title346017SRM Institute of Science and Technology - Academic Curricula – (M.Tech Regulations 2020)3
8. Program Articulation MatrixHHHHHH-LLLHHHHHHHHH-HHHH HH HHHHHHSRM Institute of Science and Technology - Academic Curricula – (M.Tech Regulations 2020)---Life Long LearningHHLeadership SkillsHHICT Skills- - - - - ML - H - - L - H - - - - - - - LL - H - - M- - - - - HL - - - - ML - - - - MH M M - M MH M M - M MM - - H - H- - - - - HM - - H - HL - H - - HEthical ReasoningM - L - L - - - MH - - - MH - H - HM - - - MM - - M M - - - MM - - - MM H - M M H - M M - M M HH - M H MM - M M HH - - - MMulticulturalHMMHMHMMHHHHHHSelf-Directed LearningHLMHLHHHHHMMMMReflective ThinkingHHHHHHHHMMHMHHScientific ReasoningResearch SkillsHMMHMHHHHHHHHMTeam WorkAnalytical ReasoningMultivariate techniques for data analyticsComputing for data analyticsBig Data TechnologyMachine Learning for data analyticsCloud Computing for data analyticsAdvanced Algorithms AnalysisPython Programming for Data AnalyticsFunctional Programming for data analyticsMarketing AnalyticsRisk AnalyticsApplied Social Network AnalysisNatural Language processing techniquesStreaming AnalyticsDeep Learning for data analyticsResearch Publishing and Presenting SkillsResearch Methods in Computer SciencesBusiness AnalyticsIndustrial SafetyOperations ResearchCost ManagementComposite MaterialsWaste to EnergyMOOCInternship (4-6 weeks during 2ndsem vacation)Minor ProjectProject Work Phase IProject Work Phase IIDisaster ManagementConstitution of IndiaValue EducationPhysical and Mental Health using YogaCareer Advancement Course for Engineers – ICareer Advancement Course for Engineers – IICareer Advancement Course for Engineers – IIIProgram AverageProblem 502T20PDM601TCritical ThinkingCourse NameDisciiplinary KnowledgeCourse CodeCommunity EngagementProgramme Learning Outcomes-HHHHH M HHHH4
O-2S 4- SLO-15 SLO-2151515Life Long Learning-Leadership SkillsMHMMMICT SkillsH HH HM MM MH HCommunity EngagementMHHHMEthical ReasoningHHHHHMulticultural Competence75757570809 10 11 12 13 14 15Self-Directed Learning80758075808Reflective ThinkingTeam Work112237Scientific ReasoningResearch Skills6Analytical Reasoning5Problem Solving4Critical Thinking3Disciplinary KnowledgeUnderstand the characteristics of data and its propertiesEffectively select and use the data reduction techniquesDeploy the multivariate techniques to solve the real world problemsAcquire information and inferences from data to predict future outputAchieve optimal solutions that maximize returns2Expected Attainment (%)CLO-1 :CLO-2 :CLO-3 :CLO-4 :CLO-5 :Program Learning Outcomes (PLO)Expected Proficiency (%)Course Learning OutcomesAt the end of this course, learners will be able to:(CLO):L T P C3 0 2 4Professional CoreLevel of Thinking (Bloom)CourseCourseCourse20ITC501JMULTIVARIATE TECHNIQUES FOR DATA eProgressiveNilNilCoursesCoursesCoursesCourse Offering Department Information TechnologyData Book / Codes/Standards NilCourse Learning RationaleThe purpose of learning this course is to:Learning(CLR):CLR-1 :Utlize data characteristics in form of distribution of the data structures1 2 31CLR-2 :Utilize the statistical data reduction techniquesUnderstand the usage of multivariate techniques for the problem under theCLR-3 :consideration.CLR-4 :Draw valid inferences and to plan for future investigationsCLR-5 :Optimize objectives and get most select resultsMM-------MMHHM1515Meaning of Multivariate AnalysisFactor Analysis IntroductionCluster Analysis IntroductionDiscriminant AnalysisIntroduction and Purpose withexamplesLinear Programming problemIntroductionMeasurements ScalesMeanings, ObjectivesObjectives and AssumptionsDiscriminant Analysisconcept,objectiveLinear Programming problemApplicationsMetric measurement scales andNon-metric measurement scalesAssumptionsResearch design in clusteranalysisDiscriminant AnalysisapplicationsFormulation of LPPLab 1: Exploration of data setsand characteristics in RLab4 :Implementation of factoranalysis in RLab 7 :Implementation of clusteranalysis in RLab10: Implementation ofdiscriminant analysis in RLab 13: Formulating a LPP in Rfrom a data set
Designing a factor analysisS-6 SLO-1SLO-2S-7S-8SLO-1S-12S-13Graphical methodProcedure for conductingdiscriminant analysisProcedure for conductingdiscriminant analysis - DemoDesigning a factor analysis Exampleassessing overall fitInter-dependence TechniquesDesigning a factor analysis –DemoDeriving clusters – Demo andexamplesProcedure for conductingdiscriminant analysis - ExamplesLab 2: Implementation ofdependent and interdependence techniquesApplications of multivariatetechniquesLab 5: Implementation of factoranalysis in RLab 8: Implementation of clusteranalysis in RLab 11: Implementation ofdiscriminant analysis in RGraphical and simplex methods– Problems, examples anddemoLab 14: Solving LPP in R –Graphical and SimplexDeriving factors and assessingoverall factorsHierarchical methodsStepwise discriminate analysisInteger ProgrammingApplications of multivariatetechniques -ExamplesInterpreting the factors andvalidation of factor analysisNon Hierarchical MethodsMahalanobis procedureTransportation problemApplications of multivariatetechniques - DemoInterpreting the factors andvalidation of factor analysis –Demo and ExamplesCombinationsLogit modelAssignment problemLab 6: Interpreting factoranalysisLab9: Implementation of clusteranalysis in RLab 12: Implementation ofdiscriminant analysis in RLab 15 :Implementation oftransportation of assignmentproblem in RSLO-2SLO-1SLO-2SLO-1S 910 SLO-2S-11Classification of multivariatetechniquesDependence TechniquesDeriving 215Lab 3: Explore scope ofmultivariate analytics in differentapplications1.LearningResources2.3.Joseph F Hair, William C Black etal , “Multivariate Data Analysis” , PearsonEducation, 7th edition, 2013.T. W. Anderson , “An Introduction to Multivariate Statistical Analysis, 3rdEdition”, Wiley, 2003.William r Dillon, John Wiley & sons, “Multivariate Analysis methods andapplications”, Wiley, 1984.4.5.6.Simplex methodNaresh K Malhotra, Satyabhusan Dash, “Marketing Research AnappliedOrientation”, Pearson, 2011.Hamdy A Taha, “Operations Research”, Pearson, 2012.6. S R Yaday, A K Malik, “Operations Research”, Oxford, 2014.
Continuous Learning Assessment (CLA) (60% weightage)Bloom’s Level ofThinkingCLA-1 (20%)CLA-2 15%Level 1UnderstandApply20%20%15%15%Level 2AnalyzeEvaluate10%10%20%20%Level 3CreateTotal100 %100 %#CLA-3 will be a Self-Learning Component and is generally a combination from among one or more of these options:AssignmentsTech. TalksMini-ProjectsPresentationsSurprise TestsField VisitsCase-StudyDebatesCourse DesignersExperts from Industry1. Dr. Hari Sekharan ,Freelance Software consultancy on Big data, analyticsSeminarsSelf-StudyGroup ActivitiesConference PapersFinal Examination (40% weightage)#CLA-3 (15%)TheoryPractice20%15%10%40%20%20%40%15%20%100 %100 %Multiple Choice QuizzesNPTEL/MOOC/SwayamOnline CertificationsGroup DiscussionsExperts from Higher Technical Institutions1. Dr.JeyaShree, Professor, Rajalakshmi Institute ofTechnologyInternal Experts1. Ms. K. Sornalakshmi, SRMIST2. Ms.D.Hemavathi SRMIST
527570238585807538070Life Long Learning2Leadership Skills1ICT Skills3Community Engagement2Ethical Reasoning1Program Learning Outcomes (PLO)Multicultural CompetenceCLO-6 :4Self-Directed LearningCLO-4 :CLO-5 :Identification of role of different professionalsApplying visualization techniquesApplying fundamental Statistics in different casestudiesApplying Interval estimation and hypothesis testingApplying various supervised techniquesApplying various unsupervised techniques andensemble techniques2Reflective ThinkingCLO-3 :At the end of this course, learners will be able to:0Scientific ReasoningCourseLearningOutcomes(CLO):CLO-1 :CLO-2 :3Team WorkCLR-5 :CLR-6 :CResearch SkillsCLR-4 :PAnalytical ReasoningCLR-3 :LearningExpected Attainment (%)CLR-2 :TData Book / Codes/Standards NilThe purpose of learning this course is to:Understanding the Role of Data Science Engineer,Big data analystUnderstanding the Role of Business IntelligencePracticing the foundations of statistics required forAnalyticsPracticing Interval estimation and understandinghypothesis testing strategyUtilizing Supervised learning algorithmsUtilizing Unsupervised learning algorithmsLProfessional CoreProgressiveCoursesExpected Proficiency (%)CLR-1 :CProblem SolvingCourse Offering Department Information oryCOMPUTING FOR DATA ANALYTICSCritical ary Knowledge20ITC502JLevel of Thinking HHHMM-HHHLLL---MMLLLL-HHH---
Duration(hour)SLO-115Overview of Data science - dataengineering,15Descriptive Statistics: DataSummarization15Sampling distributions: Basicterminologies1515Introduction to MachineLearningUnsupervised learning ClusteringSupervised learning Regression AnalysisK-means algorithmS-1SLO-2SLO-1S-2Introduction to Data engineering- DB,ETLMeasure of Location, skewnessand shapeCentral limit theoremTrue versus Fitted RegressionLine, f1score, over fitting andunder fittinghierarchical and DimensionalityreductionIntroduction to Big dataanalytics, Data in Data analyticsMeasure of dispersion- Range,VarainceApplicability of Central limittheoremLeast Square method, Measureof quality of FitPrinciple component analysis(PCA)SLO-2SLO-1S-3Standard DeviationSLO-2S 4- SLO-15 SLO-2SLO-1S-6Lab. 1: Role of data analyst innetflix applicationLab. 4: Understanding R- DatatypesLab.7: problems on ProbabilityLab. 10: Implementation ofLinear Regression in RLab. 13: Implementation of Kmeans algorithmNOIR classification, State of thepractice in analytics, role of datascientistMean absolute Deviation,Absolute Average variationChi squared DistributionClassification techniques Logistic Regression :Introduction to classification ,confusion matrix 2*2 and 3*3Ensemble techniques introduction to ensembletechniqueKey roles for successful analyticprojectInter Quartile Range, FiveNumber summaryHypothesis testing procedurGradient descent, Maximumlikelihood EstimationBaggingErrors in Hypothesis testingModel evaluation metrics,Multiple Linear RegressionBagging -ContinuedInferential Statistics :Approaches- Hypothesis,Confidence IntervalmeasurementSLO-2SLO-1Potential problems in LinearregressionS-7Other measures (Arithmetic,Geometric, Harmonic mean)SLO-2SLO-1S-8SLO-2Main phases of life cycleBivaraint - correlationCovarianceIntroduction to Probabilitydistribution: Discrete andcontinuous
S 9- SLO-110 SLO-2SLO-1Lab. 2: Role of Big data analystin Health care DomaiLab. 5: UnderstandingFunctions, Import, ExportLab. 8: Problems on HypothesisLab. 11: Implementation ofMultiple Regression in RLab. 14: Implementation ofLogistic Regression in Roverview about BI toolDiscrete : BinomialRejection region, Two tailed test,Naive Bayes' classifier, MEstimate approachBoostingOne tailed testS-11SLO-2Parametric Tests (Z-test, t-test)SLO-1S-12SLO-2SLO-1S-13Application of BI andadvantages of BIPoissonParametric Tests (Chi squaretest, F-test)Decision tree: Induction,Information GainBoosting-ContinuedDeveloping core deliverables forstakeholdersContinuous : Normal,ExponentialRelationship analysis :Correlation analysis- KarlPearson coefficient correlationCART algorithmStackingLab 3: Role of Data EngineerFraud management andPreventionLab.6: problems on DataSummarizationLab.9: problems on CorrelationLab. 12: Implementation ofLogistic Regression in RLab. 15: Implementation ofLogistic Regression in RSLO-2SSLO-114SLO-215LearningResources1. Chris Eaton, Dirk Deroos, Tom Deutsch et al., “Understanding Big Data”,McGrawHIll,2012.2. Alberto Cordoba, “Understanding the Predictive Analytics Lifecycle”, Wiley,2014.3. S M Ross, “Introduction to Probability and Statistics for Engineers andScientists”, Academic Foundation, 2011.4. Gareth James, Daniela Witten, Trevor Hastie , Robert Tibshirani, An Introduction toStatistical Learning: with Applications in R, Springer 20175. John Chambers , Software for Data Analysis: Programming with R,20086. Joseph Adler , R in a Nutshell, O’Reilly, Sebastopol, 2009Continuous Learning Assessment (CLA) (60% weightage)Bloom’sLevel of ThinkingCLA-1 (20%)CLA-2 (25%)TheoryPracticeTheoryPracticeRememberLevel 120%20%15%15%UnderstandApplyLevel 220%20%15%15%AnalyzeEvaluateLevel 310%10%20%20%CreateTotal100 %100 %#CLA-3 will be a Self-Learning Component and is generally a combination from among one or more of these options:Final Examination (40% weightage)#CLA-3 (15%)TheoryPractice20%15%10%40%20%20%40%15%20%100 %100 %
AssignmentsTech. TalksMini-ProjectsPresentationsSurprise TestsField VisitsCase-StudyDebatesSeminarsSelf-StudyGroup ActivitiesConference PapersMultiple Choice QuizzesNPTEL/MOOC/SwayamOnline CertificationsGroup DiscussionsCourse DesignersExperts from Industry1. Dr. Deepan raj, Visteon,ChennaiExperts from Higher Technical Institutions1. Dr. Sushama M.Bendre, Professor, Indian StatisticalInstitute,Applied Statistical Unit, Chennai.2. Dr. C.K. Chandrasekhar, Data Science Consultant2. Dr. R.Srinivasan, Professor, SSN college of Engineering,KalavakkamInternal Experts1.Dr.M.Thenmozhi
CourseCourseCourse20ITC503JBIG DATA teProgressiveNilNilCoursesCoursesCoursesCourse Offering Department Information TechnologyData Book / Codes/Standards NilDuration(hour)S-1S-2S-3Self-Directed LearningMulticultural CompetenceEthical ReasoningCommunity EngagementICT SkillsLeadership SkillsLife Long Learning10 11 12 13 14 15Reflective Thinking9Scientific Reasoning8Team Work7Research Skills6Analytical Reasoning5Problem Solving4Critical Thinking3Disciplinary MHHHLHMMH---LMMMMLLLLL-HHHHH---Understand Hadoop architecture and its Business ImplicationsBuild reliable, scalable distributed system with Apache HadoopImport and export data into Hadoop Distributed File systemInterpret MongoDB design goals and setup MongoDB environmentDevelop Big Data Solutions using Hadoop Eco System tools151Expected Attainment (%)CLO-1 :CLO-2 :CLO-3 :CLO-4 :CLO-5 :3C4Expected Proficiency (%)Course Learning OutcomesAt the end of this course, learners will be able to:(CLO):2P2Program Learning Outcomes (PLO)Learning1T0Level of Thinking (Bloom)Course Learning RationaleThe purpose of learning this course is to:(CLR):CLR-1 :Ulitize the Hadoop architecture and its use casesCLR-2 :Create mapper and reducer functions to build Hadoop applicationsCLR-3 :Understand key design considerations for data ingress and egress tools in HadoopCLR-4 :Review about MongoDB Aggregation frameworkCLR-5 :Infer about different kind of ecosystem tools in HadoopL3Professional Core15151515SLO-1Introduction to Big Data and itsimportanceHadoop Map Reduce paradigmAPIs used to Write/Read filesinto/from HadoopHistory of NoSQL DatabasesIntroduction to Ecosystem toolsSLO-2Basics of Distributed File SystemMap and reduce tasksNeed for Flume and SqoopFeatures of NoSQLHive ArchitectureFour Vs, Drivers for Big dataJob Tracker and task trackerFlume ArchitectureNOSQL VS RDBMSSLO-2Big data applicationsMap reduce execution pipelineThe HDFS SinkTypes of NoSQL DatabasesComparison with TraditionalDatabaseHiveQLKey value pair, Shuffle and sortPartitioning and and quasi structured dataHistory of Hadoop-Hadoop use casesKey-value stores-DocumentdatabasesQuerying DataSLO-1Combiner and PartitionerFile FormatsWide-column storesLab10: HBase-CommandsSorting And AggregatingSLO-1SLO-2SLO-1
S4SLO-25SLO-1S-6SLO-2SLO-1S-7SLO-2LAB 1:HDFS Shell Commands – Filesand FoldersThe Design of HDFSLab4: Implementing word countprogram using map reduceMap reduce exampleLAB 7:Write/Read filesinto/from Hadoop using APIFan OutBlocks and replication managementUnderstanding input textformats in HadoopUnderstanding output formatsin HadoopMap reduce AlgorithmsData transport using FLUMEeventsIntegrating Flume withApplicationsIntroduction to SQOOPSQOOP featuresRack AwarenessHDFS architectureBenefits of NOSQLJoins & Sub queriesIntroduction to MongoDBPIGExecution TypesLAB 8: Installing EcosystemtoolsMongoDB document modeland basic schema designThe key MongoDBcharacteristicsUnderstanding the MongoDBEcosystemLab 11: Hive Create, Alter andDrop tablesSqoop Import All TablesDiving into create operationsPig Latin EditorsSLO-1S9SLO-210LAB 2:HDFS Shell Commands ManagementS- SLO-111 SLO-2Name node High e Coordination andTask ManagementLab 5: Finding out Number ofProducts Sold in Each Countryusing map reduce with sampledatasetHadoop 2.0 featuresBasic Hadoop Shell commandsYARN ArchitectureSqoop Export All TablesRead operationsGenerating ExamplesS- SLO-112 SLO-2Anatomy of File WriteMRV1 Vs MRV2Sqoop ConnectorsUpdate operationsHBase ArchitectureAnatomy of File readIntroduction to SchedulersA Sample ImportDelete operationsComponents, and Use CasesYARN scheduler policiesSqoop Import from MySQL toHDFSSqoop vs flumeUnderstanding the Basics andCRUD operationsUpdate and delete operationLAB 9: Scoop – Move Data intoHadoopLab 12:Pig Latin Scripts inthree modesComparison of HBase withRDBMSHBase Create Table withExampleLab 15:Twitter Data Analyticsfor understanding big datatechnologies.SLO-1S-8SLO-2S13SLO-1SLO-2S SLO-114SLO-215LearningResourcesName node and Data nodeGraph storesLab 13:Cluster Managementusing ZookeeperMap Reduce ScriptsHDFS FederationData serializationSerialization in JAVA and HadoopLab 3: Steps to run map reduceprogramFIFO, Fair And CapacityschedulerLab 6: Find matrixmultiplication using map reduceSqoop Architecture1. Tom White, ―HADOOP: The definitive Guide , O Reilly 2012.2. Chris Eaton, Dirk deroos et al. , ―Understanding Big data McGraw Hill, 2012.3. Vignesh Prajapati, ―Big Data Analytics with R and Hadoop Packet Publishing2013.4. http://www.bigdatauniversity.com/Running Pig ProgramsGruntLab 14: Execute pig Latincommands-To query datasetstored in HDFS
Continuous Learning Assessment (CLA) (60% weightage)Bloom’sLevel of acticeRemember20%20%15%15%Level 1UnderstandApply20%20%15%15%Level 2AnalyzeEvaluate10%10%20%20%Level 3CreateTotal100 %100 %#CLA-3 will be a Self-Learning Component and is generally a combination from among one or more of these options:AssignmentsTech. TalksMini-ProjectsPresentationsCourse DesignersExperts from IndustrySurprise TestsField VisitsCase-StudyDebatesSeminarsSelf-StudyGroup ActivitiesConference PapersFinal Examination(40% weightage)#CLA-3 (15%)TheoryPractice20%15%10%40%20%20%40%15%20%100 %100 %Multiple Choice QuizzesNPTEL/MOOC/SwayamOnline CertificationsGroup DiscussionsExperts from Higher Technical Institutions1. Dr.S Muthurajkumar, Asst. Professor, Department of1.Dr.R. SivaKumar,Sr. Consultant,rsivakoumar@gmail.com A2O Integrated services Pvt.,Computer Technology, muthuraj@annauniv.edu, MITLtd., ChennaiCampus, Anna University, Chromepet, Chennai-600044.Internal Experts1.Ms.S.Sindhu2.Dr.G.Maragatham
CourseCourseCourseMACHINE LEARNING FOR DATA urse Offering Department Information TechnologyData Book / Codes/Standards NilCourse Learning RationaleThe purpose of learning this course is to:(CLR):To introduce the Concept of data , characteristics of data and PreprocessingCLR-1 :TechniquesTo introduce Classification Techniques Basic methods – Supervised MachineCLR-2 :learningTo introduce Classification Techniques Advanced methods – Supervised MachineCLR-3 :learningCLR-4 :To introduce Clustering Techniques – Un Supervised Machine learningCLR-5 :To introduce Reinforcement Learning TechniquesLearningDuration(hour)SLO-1S-1SLO-2S-2 SLO-115Basics - Data Objects andAttribute typesTypes : Nominal, Binary,Ordinal, Numeric, Discrete VsContinuous AttributesBasic Statistical Descriptions ofData15Classification : BasicsIntroduction to Classification ,General Approach toClassificationDecision Tree InductionProgram Learning Outcomes (PLO)Ethical ReasoningCommunity EngagementICT SkillsLeadership SkillsLife Long Learning9 10 11 12 13 14 15Multicultural Competence8Self-Directed Learning7Reflective Thinking6Scientific Reasoning5Team Work4Research Skills3Analytical Reasoning2Problem Solving1Critical Thinking3Disciplinary KnowledgeUnderstanding the Pre-processing concepts in Machine LearningUnderstanding the Basic level Supervised learning Techniques with working knowledgeUnderstanding the Advanced Supervised learning Techniques with working knowledgeUnderstanding the Un Supervised learning Techniques with working knowledgeUnderstanding the concept of Reinforcement learning and its applicationsC4Expected Attainment (%)CLO-1 :CLO-2 :CLO-3 :CLO-4 :CLO-5 :P2Expected Proficiency (%)Course Learning OutcomesAt the end of this course, learners will be able to:(CLO):2T0Level of Thinking (Bloom)1L3Professional ification- AdvancedMethodsConcepts and MechanismsCluster Analysis : Basic conceptsand MethodsIntroduction to Cluster AnalysisReinforcement learning :BasicsIntroduction : Definition andpurposeBayseian Belief NetworksCluster AnalysisReinforcement learning:Basics
SLO-2SLO-1S-3SLO-2S SLO-14-5 SLO-2SLO-1S-6SLO-2SLO-1Measuring the CentralTendency, Measuring theDispersion of Data.Data Pre processingBasics of Decision TreeInduction, Attribute SelectionMeasuresDecision Tree InductionData QualityLab 1: Data Pre processingTechniques using PythonData Pre processingTree Pruning, Scalability &Decision Tree InductionLab4 :Decision TreeImplementation using PythonBayes’ Classification MethodsMajor Tasks in Data PreprocessingData CleaningBayes’ Theorem , NaïveBayesian ClassificationRule Based ClassificationMissing ValuesUsing IF-THEN Rules forClassification, Rule Extractionfrom Decision Tree, RuleInduction using a sequentialCovering AlgorithmModel Evaluation and SelectionS-7SLO-2SLO-1S-8SLO-2SLO-1S9-10 SLO-2SLO-1S-11SLO-2SLO-1S-12Data CleaningNoisy data, Data cleaning as aProcessLab 2: Lab 1: Data Preprocessing Techniques usingPythonData IntegrationEntity Identification Problem ,Redundancy and CorrelationAnalysisData ReductionSLO-2Overview of Data ReductionStrategies, wavelet TransformsSLO-1Data ReductionSLO-2Principal Components Analysis,Attribute Subset SelectionS-13Training Bayesian BeliefNetworksClassification by BackPropagationA Multilayer Feed-ForwardNeural NetworksLab 7 : BPN Implementation pythonClassification by BackPropagationDefining a Network TopologyClassification by BackPropagationBack PropagationRequirements for Cluster Analysis,Overview of Basic ClusteringMethodsPartitioning MethodsK-Means : A Centroid BasedTechniqueLab10: K – Means Implementation pythonPartitioning MethodsK-Medoids : A RepresentatviveObject-Based TechniqueHierarchical MethodsAgglomerative Vs DivisiveHierarchical Cluste
SRM Institute of Science and Technology - Academic Curricula - (M.Tech Regulations 2020) 1 M.Tech in Information Technology Big Data Analytics . PEO - 4 Graduates exploit the power of big data analytics those are in high demand as organizations are looking for. 4. Consistency of PEO's with Mission of the Department Mission Stmt. - 1 .