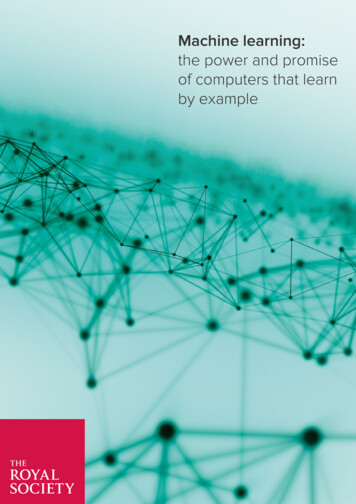
Transcription
Machine learning:the power and promiseof computers that learnby exampleMACHINE LEARNING: THE POWER AND PROMISE OF COMPUTERS THAT LEARN BY EXAMPLE1
Machine learning: the power and promiseof computers that learn by exampleIssued: April 2017 DES4702ISBN: 978-1-78252-259-1The text of this work is licensed under the termsof the Creative Commons Attribution Licensewhich permits unrestricted use, provided theoriginal author and source are credited.The license is available at:creativecommons.org/licenses/by/4.0Images are not covered by this license.This report can be viewed online atroyalsociety.org/machine-learningCover image shulz.2MACHINE LEARNING: THE POWER AND PROMISE OF COMPUTERS THAT LEARN BY EXAMPLE
ContentsExecutive summary5Recommendations8Chapter one – Machine learning151.116Systems that learn from data1.2 The Royal Society’s machine learning project181.3 What is machine learning?191.4 Machine learning in daily life211.5 Machine learning, statistics, data science, robotics, and AI241.6 Origins and evolution of machine learning251.7 Canonical problems in machine learning29Chapter two – Emerging applications of machine learning332.1 Potential near-term applications in the public and private sectors342.2 Machine learning in research412.3 Increasing the UK’s absorptive capacity for machine learning45Chapter three – Extracting value from data473.1 Machine learning helps extract value from ‘big data’483.2 Creating a data environment to support machine learning493.3 Extending the lifecycle of open data requires open standards553.4 Technical alternatives to open data: simulations and synthetic data57Chapter four – Creating value from machine learning614.1 Human capital, and building skills at every level624.2 Machine learning and the Industrial Strategy74MACHINE LEARNING: THE POWER AND PROMISE OF COMPUTERS THAT LEARN BY EXAMPLE3
4Chapter five – Machine learning in society835.1 Machine learning and the public845.2 Social issues associated with machine learning applications905.3 The implications of machine learning for governance of data use985.4 Machine learning and the future of work100Chapter six – A new wave of machine learning research1096.1 Machine learning in society: key scientific and technical challenges1106.2 Interpretability and transparency1106.3 Verification and robustness1126.4 Privacy and sensitive data1136.5 Dealing with real-world data: fairness and the full analytics pipeline1146.6 Causality1156.7 Human-machine interaction1156.8 Security and control1166.9 Supporting a new wave of machine learning research117Annex / Glossary / Appendices119Canonical problems in machine learning120Glossary122Appendix124MACHINE LEARNING: THE POWER AND PROMISE OF COMPUTERS THAT LEARN BY EXAMPLE
EXECUTIVE SUMMARYExecutive summaryMachine learning is a branch of artificialintelligence that allows computer systemsto learn directly from examples, data, andexperience. Through enabling computers toperform specific tasks intelligently, machinelearning systems can carry out complexprocesses by learning from data, ratherthan following pre-programmed rules.Recent years have seen exciting advancesin machine learning, which have raised itscapabilities across a suite of applications.Increasing data availability has allowedmachine learning systems to be trained ona large pool of examples, while increasingcomputer processing power has supported theanalytical capabilities of these systems. Withinthe field itself there have also been algorithmicadvances, which have given machine learninggreater power. As a result of these advances,systems which only a few years ago performedat noticeably below-human levels can nowoutperform humans at some specific tasks.Many people now interact with systems basedon machine learning every day, for examplein image recognition systems, such as thoseused on social media; voice recognitionsystems, used by virtual personal assistants;and recommender systems, such as thoseused by online retailers. As the field developsfurther, machine learning shows promiseof supporting potentially transformativeadvances in a range of areas, and the socialand economic opportunities which follow aresignificant. In healthcare, machine learning iscreating systems that can help doctors givemore accurate or effective diagnoses forcertain conditions. In transport, it is supportingthe development of autonomous vehicles, andhelping to make existing transport networksmore efficient. For public services it has thepotential to target support more effectively tothose in need, or to tailor services to users.And in science, machine learning is helpingto make sense of the vast amount of dataavailable to researchers today, offering newinsights into biology, physics, medicine, thesocial sciences, and more.The UK has a strong history of leadershipin machine learning. From early thinkersin the field, through to recent commercialsuccesses, the UK has supported excellencein research, which has contributed to therecent advances in machine learning thatpromise such potential. These strengths inresearch and development mean that theUK is well placed to take a leading role inthe future development of machine learning.Ensuring the best possible environment forthe safe and rapid deployment of machinelearning will be essential for enhancingthe UK’s economic growth, wellbeing, andsecurity, and for unlocking the value of ‘bigdata’. Action in key areas – shaping the datalandscape, building skills, supporting business,and advancing research – can help createthis environment.MACHINE LEARNING: THE POWER AND PROMISE OF COMPUTERS THAT LEARN BY EXAMPLE5
EXECUTIVE SUMMARYThe recent success of machine learning owesno small part to the explosion of data that isavailable in some areas, such as image orspeech recognition. This data has provideda vast number of examples, which machinelearning systems can use to improve theirperformance. In turn, machine learningcan help address the social and economicbenefits expected from so-called ‘big data’,by extracting valuable information throughadvanced data analytics. Supporting thedevelopment of this function for machinelearning requires an amenable dataenvironment, based on open standardsand frameworks or behaviours to ensuredata availability across sectors.As machine learning systems become moreubiquitous, or significant in certain fields, threeskills needs follow. Firstly, as daily interactionswith machine learning become the norm formost people, a basic understanding of theuse of data and these systems will become animportant tool required by people of all agesand backgrounds. Introducing key conceptsin machine learning at school can help ensurethis. Secondly, to ensure that a range ofsectors and professions have the absorptivecapacity to use machine learning in ways thatare useful for them, new mechanisms areneeded to create a pool of informed users orpractitioners. Thirdly, further support is neededto build advanced skills in machine learning.6There is already high demand for peoplewith advanced skills, with specialists in thefield being highly sought after, and additionalresources to increase this talent pool arecritically needed. ‘No regrets’ steps in buildingdigital literacy and informed users will alsohelp prepare the UK for possible changes inthe employment landscape, as the fields ofmachine learning, artificial intelligence, androbotics develop.There is a vast range of potential benefitsfrom further uptake of machine learning acrossindustry sectors, and the economic effectsof this technology could play a central role inhelping to address the UK’s productivity gap.Businesses of all sizes across sectors needto have access to appropriate support thathelps them to understand the value of dataand machine learning to their operations.To meet the demand for machine learningacross industry sectors, the UK will need tosupport an active machine learning sector,which capitalises on the UK’s strength in thisarea, and its relative international competitiveadvantages. The UK’s start-up environmenthas nurtured a number of high-profile successstories in machine learning, and strategicconsideration should be given to how tomaximise the value of entrepreneurialactivity in this space.MACHINE LEARNING: THE POWER AND PROMISE OF COMPUTERS THAT LEARN BY EXAMPLE
EXECUTIVE SUMMARYThe Royal Society conducted research tounderstand the views of members of thepublic towards machine learning. Whilemost people were not aware of the term,they did know of some of its applications.There was not a single common view, withattitudes, both positive and negative, varyingdepending on the circumstances in whichmachine learning was being used. Ongoingengagement with the public will be importantas the field develops.Machine learning applications can perform wellat specific tasks. In many cases it can be usedto augment human roles. Although it is clearthat developments in machine learning willchange the world of work, predicting how thiswill unfold is not straightforward, and existingstudies differ substantially in their projections.While offering potential for new businessesor areas of the UK economy to thrive, thedisruptive potential of machine learning bringswith it challenges for society, and questionsabout its social consequences. Some of thesechallenges relate to the way in which newuses of data reframe traditional concepts of,for example, privacy or consent, while othersrelate to how people interact with machinelearning systems. Careful stewardship willbe needed to ensure that the productivitydividend from machine learning benefits allin society.Machine learning is a vibrant field ofresearch, with a range of exciting areasfor further development across differentmethods and applications. In addition tothose areas of research that address purelytechnical questions, there is a collectionof specific research questions whereprogress would directly address areas ofpublic concern around machine learning,or constraints on its wider use. Supportfor research in these areas can thereforehelp ensure continued public confidencein the deployment of machine learningsystems. These areas include algorithmicinterpretability, robustness, privacy, fairness,inference of causality, human-machineinteraction, and security.MACHINE LEARNING: THE POWER AND PROMISE OF COMPUTERS THAT LEARN BY EXAMPLE7
RECOMMENDATIONSRecommendationsEXTRACTING VALUE FROM DATACreating a data environmentto support machine learningGood progress in increasing the accessibilityof public sector data has positioned the UKas a leader in this area; continued effortsare needed in a new wave of ‘open data formachine learning’ by Government to enhancethe availability and usability of public sectordata, while recognising the value of strategicdatasets.In areas where there are datasets unsuitable forgeneral release, further progress in supportingaccess to public sector data could be drivenby creating policy frameworks or agreementswhich make data available to specific usersunder clear and binding legal constraints tosafeguard its use, and set out acceptableuses. The UK Biobank demonstrates how sucha framework can work. Government shouldfurther consider the form and function of suchnew models of data sharing.Continuing to ensure that data generated bycharity- and publicly-funded research is openby default and curated in a way that facilitatesmachine driven analysis will be critical insupporting wider use of research data. Whereappropriate, journals should insist on this databeing made available to other researchers inits original form, or via appropriate summarystatistics where sensitive personal informationis involved.8In designing their studies, researchers shouldconsider future potential uses of their data,and build in the broadest consents that areethically acceptable, and acceptable to researchparticipants.Research funders should ensure that datahandling, including the cost of preparing dataand metadata, and associated costs, such asstaff, is supported as a key part of researchfunding, and that researchers are activelyencouraged across subject areas to apply forfunds to cover this. Research funders shouldensure that reviewers and panels assessinggrants appreciate the value of such datamanagement.Extending the lifecycle of opendata requires open standardsNew open standards are needed for data,which reflect the needs of machine-drivenanalytical approaches.The Government has a key role to play in thecreation of new open standards, for examplefor metadata. Government should exploreways of catalysing the safe and rapid deliveryof these to support machine learning in the UK.MACHINE LEARNING: THE POWER AND PROMISE OF COMPUTERS THAT LEARN BY EXAMPLE
RECOMMENDATIONSCREATING VALUE FROM MACHINE LEARNINGHuman capital, and buildingskills at every levelSchools need to ensure that key concepts inmachine learning are taught to those who willbe users, developers, and citizens.Government, mathematics and computingcommunities, businesses, and educationprofessionals should help ensure that relevantinsights into machine learning are built into thecurrent education curriculum and associatedenrichment activity in schools over the nextfive years, and that teachers are supported indelivering these activities.In addition to the relevant areas ofmathematics, computer science, and dataliteracy, the ethical and social implications ofmachine learning should be included withinteaching activities in related fields, such asPersonal, Social, and Health Education.The next curriculum reform needs to considerthe educational needs of young peoplethrough the lens of the implications of machinelearning and associated technologies for thefuture of work.An analysis of the future data science needsof students, industry, and academia shouldbe undertaken to inform future curriculumdevelopments.To equip students with the skills to work withmachine learning systems across professionaldisciplines, universities will need to ensurethat course provision reflects the skills whichwill be needed by professionals in fieldssuch as law, healthcare, and finance in thefuture. Some exposure to machine learningtechniques will also be useful in many scientificactivities. Professional bodies should workwith universities to adjust course provisionaccordingly, and to ensure accreditationschemes take these future skills needsinto account.In the short term, the most effective mechanismto support a strong pipeline of practitioners inmachine learning is likely to be governmentsupport for advanced courses – namely mastersdegrees – which those working across a rangeof sectors could use to pick up machine learningskills at a high level. Government shouldconsider introducing a new funded programmeof masters courses in machine learning,potentially in parallel with encouragement forapproaches to training in machine learning viaMassive Open Online Courses (MOOCs), withthe aim of increasing the pool of informed usersof machine learning.MACHINE LEARNING: THE POWER AND PROMISE OF COMPUTERS THAT LEARN BY EXAMPLE9
RECOMMENDATIONSCREATING VALUE FROM MACHINE LEARNING (CONTINUED)Universities and funders should give urgentattention to mechanisms which will help recruitand retain outstanding research leaders inmachine learning in the academic sector. Thisacademic leadership is critical to inspiringand training the next generation of researchleaders in machine learning.In considering the allocation of additionalPhD places, as announced in the Spring 2017budget, and new fellowships across subjectareas, machine learning should be considereda priority area for investment.Because of the substantial skills shortagein this area, near-term funding should bemade available so that the capacity to trainUK PhD students in machine learning is ableto increase with the level of demand forcandidates of a sufficiently high quality. Thiscould be supported through allocation ofthe expected 1000 extra PhD places, or mayrequire additional resources.Machine learning and theIndustrial StrategyAs it considers its future approach toimmigration policy, the UK must ensure thatresearch and innovation systems continue tobe able to access the skills they need. TheUK’s approach to immigration should supportthe UK’s aim to be one of the best placesin the world to research and innovate, andmachine learning is an area of opportunity insupport of this aim.Government’s proposal that robotics and AIcould be an area for early attention by theIndustrial Strategy Challenge Fund is welcome.Machine learning should be considered a keytechnology in this field, and one which holdssignificant promise for a range of industrysectors.UK Research and Innovation (UKRI) shouldensure machine learning is noted as akey technology in the Robotics and AIChallenge area.In determining the shape and nature ofDARPA-style challenge funding for research,Government should have regard to facilitatingthe spread and uptake of machine learningacross sectors.10MACHINE LEARNING: THE POWER AND PROMISE OF COMPUTERS THAT LEARN BY EXAMPLE
RECOMMENDATIONSKey sectors of UK industry – as outlinedin this report – have the potential to adoptmachine learning and create value fromits use. However, uptake across sectors ispatchy, and many areas of UK industry arenot yet making use of this technology. Forexample, in manufacturing, pharmaceuticals,the legal sector, energy, cities, and transportthere are challenges suitable for intervention,and potential for machine learning to disruptcurrent activities. Increasing the absorptivecapacity of these sectors through the IndustrialStrategy Challenge Fund could help deliverthe benefits of machine learning more quickly,and Government should design challengesin these areas to push forward the use ofmachine learning accordingly.Government needs to provide mechanismsto support people seeking to make use ofmachine learning, through public supportfor entrepreneurism, small business, andenterprise.Businesses need to understand the valueof data analytics as a key part of businessinfrastructure. Government support forbusiness should be able to provide adviceand guidance about how to make best use ofdata, and organisations such as Growth Hubsor the Knowledge Transfer Network shouldensure their business advisers are sufficientlyinformed about the value of data as businessinfrastructure to be able to provide guidancefor businesses about, for example, the value ofmachine learning.The Department for Business, Energy andIndustrial Strategy (BEIS) should review supportnetworks for small businesses to ensure theyare able to provide advice and guidanceabout how to make use of machine learning,or to effectively support businesses offeringmachine learning products. This includespublic-sector procurement processes, and theeffectiveness of support for businesses usingmachine learning should be considered aspart of the Government’s review of the SmallBusiness Research Initiative.MACHINE LEARNING: THE POWER AND PROMISE OF COMPUTERS THAT LEARN BY EXAMPLE11
RECOMMENDATIONSMACHINE LEARNING IN SOCIETYMachine learning and the publicContinued engagement between machinelearning researchers and the public isneeded: those working in machine learningshould be aware of public attitudes to thetechnology they are advancing, and largescale programmes in this area should includefunding for public engagement activities byresearchers. Government could further supportthis through its public engagement frameworkprogrammes.To help ensure those working in machinelearning are given strong grounding in thebroader societal implications of their work,postgraduate students in machine learningshould pursue relevant training in ethics aspart of their studies.It is not appropriate to set up governancestructures for machine learning per se. Whilethere may be specific questions about the useof machine learning in specific circumstances,these should be handled in a sector-specificway, rather than via an overarching frameworkfor all uses of machine learning; some sectorsmay have existing regulatory mechanisms thatcan manage, while in others there may not bethese existing systems.Machine learning and the futureof workSociety needs to give urgent consideration tothe ways in which the benefits from machinelearning can be shared across society.The implications of machinelearning for governance ofdata useThere are governance issues surroundingthe use of data, including those concerningthe sources of data, and the purposes forwhich it is used. For this, a new frameworkfor data governance – one that can keeppace with the challenge of data governancein the 21st century – is necessary to addressthe novel questions arising in the new digitalenvironment. The form and function of such aframework is the basis of a study by the RoyalSociety and British Academy.12MACHINE LEARNING: THE POWER AND PROMISE OF COMPUTERS THAT LEARN BY EXAMPLE
RECOMMENDATIONSA NEW WAVE OF MACHINE LEARNING RESEARCHProgress in some areas of machinelearning research will impact directly on thesocial acceptability of machine learning inapplications and hence on public confidenceand trust. Funding bodies should encourageand support research applications in theseareas, though not to the exclusion of otherareas of machine learning research. Theseareas include algorithm interpretability,robustness, privacy, fairness, inference ofcausality, human-machine interactions, andsecurity.MACHINE LEARNING: THE POWER AND PROMISE OF COMPUTERS THAT LEARN BY EXAMPLE13
14MACHINE LEARNING: THE POWER AND PROMISE OF COMPUTERS THAT LEARN BY EXAMPLE
Chapter oneMachine learningLeftMany people alreadyinteract with machinelearning systems on adaily basis, for examplethrough virtual personalassistants on smartphones. martin-dm.MACHINE LEARNING: THE POWER AND PROMISE OF COMPUTERS THAT LEARN BY EXAMPLE15
CHAPTER ONEMachine learningMachine learningis the technologythat allowssystems to learndirectly fromexamples, data,and experience.1.1 Systems that learn from dataRecent years have seen much discussionof machine intelligence, and what this meansfor our health, productivity, and wellbeing.In such discussion, machine learningapparently promises to save lives, addressglobal challenges such as climate change,and add trillions of dollars to the globaleconomy through increasing productivity;while doing so it also fundamentally changesthe nature of work, and shapes, or defines,the choices people make in everydaylife. Between these extremes, there liesa potentially transformative technology,which brings with it both opportunities andchallenges, and whose risks and benefitsneed to be navigated as its use becomesmore central to everyday activities.Machine learning is the technology thatallows systems to learn directly fromexamples, data, and experience.If the broad field of artificial intelligence (AI)is the science of making machines smart,then machine learning is a technology thatallows computers to perform specific tasksintelligently, by learning from examples.These systems can therefore carry outcomplex processes by learning from data,rather than following pre-programmed rules.1.16Recent years have seen significant advancesin the capabilities of machine learning, as aresult of technical developments in the field,increased availability of data, and increasedcomputing power. As a result of theseadvances, systems which only a few yearsago struggled to achieve accurate resultscan now outperform humans at specific tasks.There now exist voice and object recognitionsystems that can perform better than humansat certain tasks, though these benchmark tasksare constrained in nature. For example, in2015, researchers created a machine learningsystem that surpassed human capabilities ina narrow range of vision-related tasks, whichfocused on recognising individual handwritten digits1.Many people now interact with machinelearning-driven systems on a daily basis: inimage recognition systems, such as thoseused to tag photos on social media; invoice recognition systems, such as thoseused by virtual personal assistants; and inrecommender systems, such as those usedby online retailers.In addition to these current applications, thefield also holds significant future potential;further applications of machine learning arealready in development in a diverse rangeof fields, including healthcare, education,transport, and more. Machine learning couldprovide more accurate health diagnosticsor personalised treatments, tailor classroomactivities to enhance student learning, andsupport intelligent transport systems. It couldalso support scientific advances, by drawinginsights from large datasets, and driveoperational efficiencies across a range ofindustry sectors.Markoff J. 2015 A learning advance in artificial intelligence rivals human abilities. New York Times. 10 December 2015.See -abilities.html(accessed 22 March 2017).MACHINE LEARNING: THE POWER AND PROMISE OF COMPUTERS THAT LEARN BY EXAMPLE
CHAPTER ONEBy increasing our ability to extract insightsfrom ever-increasing volumes of data,machine learning could increase productivity,provide more effective public services, andcreate new products or services tailored toindividual needs. However, in doing so it raisesquestions about new uses of data, and the roleof intelligent computer systems in society.Given the scale of the potential benefitsfrom this technology, and its increasingpervasiveness, now is the time to ensure thatit is developed in a way that engenders publicconfidence and addresses key concernsor challenges. This is not only to managethe potential risks associated with machinelearning, but also to ensure that the full rangeof potential benefits is realised.There is an opportunity now – where the fieldof machine learning is sufficiently nascent –to both shape how this technology develops,and to ensure that the UK is at the forefrontof driving this development.The UK has a strong history of researchand development in AI and machine learning.In the 1950s, it was home to early thinkersin the field, with Alan Turing posing thequestion “can machines think?2” and famouslyestablishing the Turing Test – whether aperson could distinguish between answersgiven by a machine and a human – as amarker of machine intelligence. The UK’sworld-leading research centres continueto drive the development of the field. Inrecent years, the UK’s machine learningcommunity has also demonstrated its strengthin supporting start-ups, with high-profilecompanies including: DeepMind, an artificialintelligence start-up acquired by Googlein 2014; VocalIQ, which develops speechrecognition systems and was bought byApple in 2015; Swiftkey, a text predictionsystem bought by Microsoft in 2016; and MagicPony, whose software enables processing ofvisual data, which was sold to Twitter in 20163.The UK is therefore well placed to continueto play a leading role in the development ofmachine learning, and in doing so both toenjoy the economic benefits it can deliver,and to help shape the field so that it advancesin ways that deliver the greatest benefits tosociety as a whole.2.Turing A. 1950 Can machines think? Mind 59, 433–460.3.Digital firms making use of machine learning – amongst a suite of tools to provide new services – are alsoattracting similar interest. For example, in November 2016 Skyscanner, a travel search business based in Edinburgh,was acquired by Chinese travel company Ctrip in a 1.4billion deal. See, for example: BBC News. 2016 Skyscannersold to China travel firm Ctrip in 1.4bn deal. See http://www.bbc.co.uk/news/business-38088016 (accessed 24November 2016).MACHINE LEARNING: THE POWER AND PROMISE OF COMPUTERS THAT LEARN BY EXAMPLE17
CHAPTER ONE1.2 The Royal Society’s machinelearning projectEngagement with, Recognising the promise of this technology,in November 2015 the Royal Society launchedand contributionsa policy project on machine learning. Thisto, the project.sought to investigate the potential of machinelearning over the next 5 – 10 years, and theDigital interactions:4barriers to realising that potential. In doingso, the project sought to engage with keyaudiences – in policy communities, industry,academia, and the public – to raise awarenessFace-to-face encounters:of machine learning, understand views held5by the public and contribute to public debateabout this technology, and identify the keysocial, ethical, scientific, and technical issuesthat machine learning presents.Wider ipation:500718Overseen by the project’s Working Group,and in pursuit of these goals, the Royal Societyconvened leading thinkers and practitionersto consider the ethical, legal, scientific, andindustry issues associated with machinelearning. The project also supported a publicdialogue exercise to investigate the public’sattitudes towards this technology, using theresults of this exercise to inform its policywork and future engagement.This process of evidence gathering hasidentified key areas in which action isneeded to help the UK reap the full benefitsof machine learning: E nabling the use of machine learning inextracting value from data, through a dataenvironment that draws on open standardsand open data principles; B uilding a skills base and researchenvironment that can provide the humanand technical capital to both apply andfurther develop machine learning; and C reating governance systems to addressthe key social and ethical challenges raisedby data in the 21st century.Making progress in each of these areasnow will help ensure that the benefits ofmachine learning are shared across society,thereby helping to avoid a potentiallysubstantial backlash or negative reactionto this technology.This report outlines the significance ofaddressing these areas in order to ensurethe UK remains at the forefront of developingmachine learning, sets out the actionsrequir
Chapter one - Machine learning 15 1.1 Systems that learn from data 16 1.2 The Royal Society's machine learning project 18 1.3 What is machine learning? 19 1.4 Machine learning in daily life 21 1.5 Machine learning, statistics, data science, robotics, and AI 24 1.6 Origins and evolution of machine learning 25