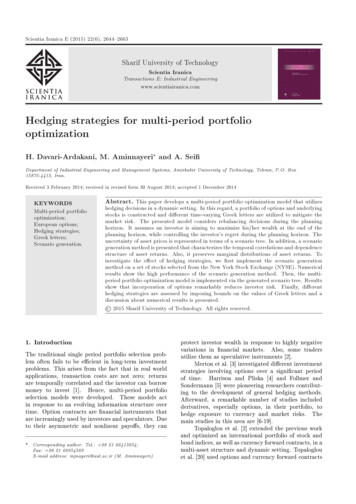
Transcription
Scientia Iranica E (2015) 22(6), 2644{2663Sharif University of TechnologyScientia IranicaTransactions E: Industrial Engineeringwww.scientiairanica.comHedging strategies for multi-period portfoliooptimizationH. Davari-Ardakani, M. Aminnayeri and A. SeiDepartment of Industrial Engineering and Management Systems, Amirkabir University of Technology, Tehran, P.O. Box15875-4413, Iran.Received 3 February 2014; received in revised form 30 August 2014; accepted 1 December 2014KEYWORDSAbstract. This paper develops a multi-period portfolio optimization model that utilizes1. Introductionprotect investor wealth in response to highly negativevariations in nancial markets. Also, some tradersutilize them as speculative instruments [2].Merton et al. [3] investigated di erent investmentstrategies involving options over a signi cant periodof time. Harrison and Pliska [4] and Follmer andSondermann [5] were pioneering researchers contributing to the development of general hedging methods.Afterward, a remarkable number of studies includedderivatives, especially options, in their portfolio, tohedge exposure to currency and market risks. Themain studies in this area are [6-19].Topaloglou et al. [2] extended the previous workand optimized an international portfolio of stock andbond indices, as well as currency forward contracts, in amulti-asset structure and dynamic setting. Topaloglouet al. [20] used options and currency forward contractsMulti-period portfoliooptimization;European options;Hedging strategies;Greek letters;Scenario generation.hedging decisions in a dynamic setting. In this regard, a portfolio of options and underlyingstocks is constructed and di erent time-varying Greek letters are utilized to mitigate themarket risk. The presented model considers rebalancing decisions during the planninghorizon. It assumes an investor is aiming to maximize his/her wealth at the end of theplanning horizon, while controlling the investor's regret during the planning horizon. Theuncertainty of asset prices is represented in terms of a scenario tree. In addition, a scenariogeneration method is presented that characterizes the temporal correlations and dependencestructure of asset returns. Also, it preserves marginal distributions of asset returns. Toinvestigate the e ect of hedging strategies, we rst implement the scenario generationmethod on a set of stocks selected from the New York Stock Exchange (NYSE). Numericalresults show the high performance of the scenario generation method. Then, the multiperiod portfolio optimization model is implemented via the generated scenario tree. Resultsshow that incorporation of options remarkably reduces investor risk. Finally, di erenthedging strategies are assessed by imposing bounds on the values of Greek letters and adiscussion about numerical results is presented. 2015 Sharif University of Technology. All rights reserved.The traditional single period portfolio selection problem often fails to be e cient in long-term investmentproblems. This arises from the fact that in real worldapplications, transaction costs are not zero; returnsare temporally correlated and the investor can borrowmoney to invest [1]. Hence, multi-period portfolioselection models were developed. These models actin response to an evolving information structure overtime. Option contracts are nancial instruments thatare increasingly used by investors and speculators. Dueto their asymmetric and nonlinear payo s, they can*. Corresponding author. Tel.: 98 21 66413034;Fax: 98 21 66954569E-mail address: mjnayeri@aut.ac.ir (M. Aminnayeri)
H. Davari-Ardakani et al./Scientia Iranica, Transactions E: Industrial Engineering 22 (2015) 2644{2663to hedge exposures to market and currency risks in astatic manner.Yin and Han [21] generalized the work ofTopaloglou et al [20] through adaptation of theirmethod in a dynamic setting.There are alternative hedging approaches, referred to as Greek letters, which measure di erentdimensions to the risk in an option position. The tradercan manage the Greek letters (Greeks), while ensuringthat all aspects of risk are acceptable. These hedgingstrategies make the investor more exible in hedgingexposure to di erent aspects of risk.Wu and Sen [22] proposed a stochastic programming approach to develop currency option hedgingstrategies to address multiple random factors in animperfect market. Their study incorporated constraints on sensitivity measures, such as Delta andGamma. Papahristodoulou [23] formulated a linearprogramming model and devised hedging strategies onall Greek letters.Horasanli [24] extended Papahristodoulou [23] toa multi-asset structure to deal with a portfolio ofoptions and underlying assets. Gao [25] proposeda linear programming model with some exible riskbounds on all Greek letters. These bounds can beadjusted by the investor to suit the needs of marketchange.In a recent study, Yin and Han [26] presented astochastic programming model for international portfolio management. Moreover, they used stock optionswith overall control on time-varying Greek letters toachieve an e cient hedging level. Compared to previous studies that used Greeks for hedging exposuresto di erent aspects of risk, their improvement wastwofold. First, they constructed an international portfolio with a multi-period and multi-currency structure.Second, they extended previous studies on optimaloptions strategies into a dynamic and nondeterministicframework.Although Yin and Han [26] incorporated hedgingdecisions in the multi-period portfolio selection problem, there exist some important issues that shouldbe necessarily addressed. Their study assumed thatoptions have a one-period time to maturity. Thus,when the investor takes a long position in a callor put option, at the next period, he/she has onlythe opportunity to exercise the option. Nevertheless,in real world nancial markets, derivatives, such asoptions, are issued with di erent maturities that areoften more than one time period. Thus, they canbe traded during their lifetime. Since the time tomaturity of an option a ects its value, in each time ofrebalancing, the pricing process for all options shouldbe performed. This makes the problem more complex.These important features of derivatives were ignored inprevious studies.2645In addition, options may impose two types oftransaction cost to investors that are charged whenany option is traded. These types of transaction costare xed and proportional. While the former does notdepend on the price of traded options, the latter does.To consider both xed and proportional transactioncosts is a matter of particular importance and stronglya ects the obtained results. This strong e ect will bediscussed later. The xed transaction cost was ignoredin [26].Moreover, although Yin and Han [26] used Greeksin a dynamic and nondeterministic setting, they considered that the volatility of each asset returns to be aconstant parameter in all scenarios. However, volatilityshould be considered a stochastic parameter that isdependent on scenarios.In this paper, all these important issues areaddressed in a multi-period portfolio selection setting.In addition, di erent from [2,20,21,26] that dealt withinternational portfolios, a portfolio with domestic diversi cation is considered. This approach seems tobe more appropriate for risk taking investors, sinceit enables them to invest in a wider variety of assets,rather than a limited set of indices. In addition, thoserisks associated with currency exchange rates are notimposed on investor wealth. Of course, the investorcan assign a part of his/her capital to less risky assetsthat have a relatively guaranteed payo .In addition, the most recent work in thisarea [2,20,21,26] utilized moment matching [27] togenerate scenarios of asset returns. This may have twomain drawbacks. First, the temporal correlations ofdata series, e.g. asset returns, are ignored. Second, thedependence structure of di erent data series is modelled via a covariance matrix that illustrates the lineardependence of the data series. This assumption holdswhen the time series data are normally distributed.However, as these studies mentioned, in most cases, thedistributions of nancial parameters, e.g. asset returns,are not normal. In this paper, these important issuesof scenario generation are addressed.Brie y speaking, this paper considers the dynamichedging of options in a multi-period portfolio selectionproblem. In fact, we incorporate options with di erentmaturities into a portfolio that is regularly rebalancedduring the planning horizon. Moreover, in additionto transaction costs pertaining to trading nancialassets, those pertaining to trading, as well as exercisingoptions, are carefully considered. Furthermore, thetemporal correlations and dependence structure ofreturn series are modelled in a careful manner.The remainder of this paper is organized asfollows. Section 2 presents a stochastic programmingmodel for multi-period portfolio selection with optionsand bounds on Greek letters. Section 3 discusses thepresented scenario generation method. In Section 4, a
2646H. Davari-Ardakani et al./Scientia Iranica, Transactions E: Industrial Engineering 22 (2015) 2644{2663practical application of the proposed framework, thepresented model, as well as the scenario generationmethod, on the New York Stock Exchange (NYSE)is provided, and computational results are discussed.Finally, Section 5 concludes the paper.2. Multi-period portfolio modelTo incorporate hedging decisions in the multi-periodportfolio selection problem, we use a stochastic programming paradigm. In addition to determining theoptimal decisions for rebalancing nancial assets, thismodel can make optimal hedging decisions againstfuture uncertainties to provide overall risk management. Greek letters play an important role in hedgingexposure to di erent aspects of risk. Each Greekletter measures the sensitivity of an option to a speci cvariable.2.1. Model assumptionsWe consider an investor that has an initial wealthand wants to maximize his/her wealth at the endof a speci ed period through investment in nancialmarkets. Moreover, he/she is going to control thebankruptcy risk during the planning horizon. In fact,this problem has a dynamic structure that involvesportfolio rebalancing decisions at periodic intervals inresponse to new information on market conditions. Weassume that borrowing and short selling are not allowedduring the lifetime of the investment. Also, the investorwants to exploit European style options to mitigatemarket risk. We assume that options are issued withdi erent maturities that are often more than a one timeperiod. Hence, they can be traded during their lifetime.Note that when the clock advances one period, the timeto maturity of options decreases one period. Therefore,the process of option pricing should be performed at thebeginning of each period.Since transaction costs have a key role in rebalancing decisions, proportional transaction costs arecharged for trading assets and taking long positionsin call and put options. Also, xed transaction costsare charged for taking long positions in call and putoptions. The uncertainty of asset returns is representedin terms of a scenario tree.In Section 3, we elaborate on the proposed scenario generation method and related concepts.2.2. Greek letters for call and put optionsGenerally, Greek letters measure the sensitivity ofoption prices to di erent parameters. Di erent hedgingstrategies can be designed by controlling di erentaspects of risk with Greek letters. For a detailed discussion about Greek letters, one can refer to Hull [28].We adapt the de nitions to the problem under consideration.For a European call option, the ve basic Greekletters are de ned as follows:s N (ds it k1;it k );sit kPits is N 0 (ds1;it k )p2s it k sit k k ep N 0 (ds1;it k )p ;Pits isr N (ds2;it k );rker N (ds2;it k ); sit k Pits N 0 (ds1;it k ):Also, they can be de ned for a European put option asfollows:0 s )0 s N (d1;it kp ; 0 sit k N (ds1;it k ) 1;it kPits isP s s N 0 (ds ) 0 sit k it i p 1;it k rke r N ( ds2;it k );2 0 s k e rT N (ds );it k 0 sit kp P s N 0 (ds2;it k1;it k );and is denoteitsPit ,where ,k, rtime to maturity,price of asset i at time t under scenario s, exercise priceof option, interest rate, and volatility of asset i underscenario s, respectively. Also:N (x) Z xp1 e2 z22 dz;1denotes the cumulative probability distribution function for a standard normal variable:d1;it k ln s Pitkand:d2;it k d1;it k s 2 r ( 2i )p is;p is :ss , s , s , sMoreover, it kit k it k it k and it k denoteDelta, Gamma, Theta, Rho and Vega of a call optionon underlying asset i at time t, with time to maturityand exercise price, k, respectively. Also, 0 sit k , 0 sit k , 0 sit k , 0 sit k and 0 sit k denote Delta, Gamma, Theta,Rho and Vega of a put option on underlying asseti at time t, with time to maturity , and exerciseprice, k, respectively. We can derive the values ofall Greek letters for the portfolio, as described laterin the mathematical programming formulation. Asmentioned above, in this study, we do not assumevolatility to be constant for all scenarios. In otherwords, for each asset in any scenario, a di erentvolatility, according to the asset prices in that scenario,is considered. We replace with is , which denotes thevolatility of asset i under scenario s.
H. Davari-Ardakani et al./Scientia Iranica, Transactions E: Industrial Engineering 22 (2015) 2644{26632.3. NotationsLet I and S be the set of available assets and scenarios,respectively. We use the following notations:Deterministic input data:W0Investor initial wealth; Proportional transaction cost for salesor purchases of asset i 2 I ;0 Proportional transaction cost fortaking long positions in options issuedon asset i 2 I ;00 Fixed transaction cost for taking longpositions in options issued on asseti 2 I;Initial market price of asset i 2 I ;i0 tInvestor level of risk aversion at time t(t 1; ; T ); tInvestor target wealth at time t(t 1; ; T );rRisk free interest rate; i0 kPrice of call option on asset i 2 Iat t 0, with time maturity andexercise price k 2 Ki ;0 i0 kPrice of put option on asset i 2 Iat t 0, with time maturity andexercise price k 2 Ki ; uUpper risk bound for Delta of theportfolio; lLower risk bound for Delta of theportfolio;Upper risk bound for Gamma of theuportfolio;Lower risk bound for Gamma of thelportfolio; uUpper risk bound for Theta of theportfolio; lLower risk bound for Theta of theportfolio;PuUpper risk bound for Rho of theportfolio;PlLower risk bound for Rho of theportfolio;KuUpper risk bound for Kappa of theportfolio;KlLower risk bound for Kappa of theportfolio.Scenario dependent parameters:sMarket price of asset i 2 I at period tit(t 1; ; T ) under scenario s;Price of call option on asset i 2 I ,with time maturity and exerciseprice k 2 Ki at time period t(t 1; ; T 1 and t 1; ; T )under scenario s 2 S ;s0 i;t; ;kPrice of put option on asset i 2 I ,with time maturity and exerciseprice k 2 Ki at time period t(t 1; ; T 1 and t 1; ; T )under scenario s 2 S .Decision variables:Amounts of asset i 2 I purchased atxsitt (t 0; 1; ; T 1) under scenarios 2 S;sxftAmounts of investment in the risk freeasset at t (t 0; 1; ; T 1) underscenario s 2 S ;sAmounts of asset i 2 I sold at tyit(t 1; ; T 1) under scenario s 2 S ;hsitAmounts of asset i 2 I held at t(t 0; 1; ; T 1) under scenarios 2 S;sAmounts of call option on asset i 2 I ,vit kwith time maturity and exerciseprice k 2 Ki held at time period t(t 0; 1; ; T 1 and t) underscenario s 2 S ;swit kAmounts of put option on asset i 2 I ,with time maturity and exerciseprice k 2 Ki held at time period t(t 0; 1; ; T and t) underscenario s 2 S ;usit kNumber of long positions in calloptions on asset i 2 I taken at periodt (t 0; 1; ; T 1), with timematurity ( t) and exercise pricek 2 Ki under scenario s 2 S ;scit kNumber of long positions in putoptions on asset i 2 I taken at periodt (t 0; 1; ; T 1), with timematurity ( t) and exercise pricek 2 Ki under scenario s 2 S ;smitkNumber of long positions in calloptions on asset i 2 I , with exerciseprice k 2 Ki exercised at time period t(t 1; ; T ) under scenario s 2 S ;sqitkNumber of long positions in putoptions on asset i 2 I , with exerciseprice k 2 Ki exercised at time period t(t 1; ; T ) under scenario s 2 S ;VtsInvestor wealth at time t (t 1; ; T )under scenario s 2 S ;s i;t; ;k2647
2648H. Davari-Ardakani et al./Scientia Iranica, Transactions E: Industrial Engineering 22 (2015) 2644{2663 stDelta of portfolio at period t(t 1; ; T 1) under scenario s 2 S ;sGamma of portfolio at period tt(t 1; ; T 1) under scenario s 2 S ;s tTheta of portfolio at period t(t 1; ; T 1) under scenario s 2 S ;sPtRho of portfolio at period t(t 1; ; T 1) under scenario s 2 S ;sKappa of portfolio at period tKt(t 1; ; T 1) under scenario s 2 S .Auxiliary variables:CtAuxiliary variable to make theobjective function linear (t 1; ; T ).Kts i 1 t 1 k2Ki i 1 t 1 k2Kis 2 S;st n XT XXi 1 t 1 k2Ki st i 1 t 1 k2Kii 1 t 1 k2KiPts 1:(5)In this paper, we are going to maximize investorexpected wealth at the end of the planning horizon,while controlling the risk of investor bankruptcy duringthe planning horizon. Zhu et al. [29] emphasized thenecessity of controlling investor bankruptcy risk inintermediate periods of a long-term investment, sinceit reduces the possibility that due to uctuations ininvestor wealth, he/she becomes bankrupt. To controlthe risk of investor bankruptcy, we use the expectedregret of investor wealth. A comparative discussionbetween expected regret and Conditional Value-at-Risk(CVaR) [30,31], a coherent and most popular riskmeasure, was made by Testuri and Uryasev [32]. Theyprove that an optimal portfolio in the CVaR sense isalso optimal in the expected regret sense for a giventarget, and vice versa. In addition, expected regret canbe well adapted to dynamic stochastic programmingmodels. Ji et al. [33] minimized the expected regret ofinvestor wealth during the planning horizon. However,the main objective of the investor, i.e. maximizingterminal wealth, was neglected. In this paper, we usea combination of expected nal wealth and expectedregret of wealth, not only during the end-of-horizonperiod, but also over the entire planning horizon. Theobjective function of the proposed model is as follows:01;(2)SPps VTsBB s 1max B T@Q(1 rp )p 1ps maxf t1;tQ(3)s svit kit kn XT XXi 1 t 1 k2Kis 2 S;sit ks 0 s ;wit kit kt 1; ; Ti 1 t 1 k2Kit 1; ; TS XTXs 1 t 1 t1i 1 t 1 k2Kin XT XX(1)s svit kit kn XT XXs 2 S;1;s0s ;wit kit kt 1; ; Tn XT XX svit kn XT XXs 2 S;s 0 s ;wit kit kt 1; ; Ti 1 t 1 k2Kis 0 s ;wit kit k2.5. Model formulations svit kit kn XT XXs svit kit kn XT XXs 2 S;Eqs. (1) to (5) can be used to compute the values ofGreek letters:n XT XXi 1 t 1 k2Ki 2.4. Computing values of Greek letters for theportfolio st n XT XXs 0 s ;wit kit kt 1; ; T1;(4)Vts ; 0g CC(1 rp )C:A(6)p 1In fact, the objective function makes a tradeo betweenthe present value of the expected terminal wealth ( rstpart) discounted by the risk free interest rate, and thepresent value of investor expected regret, with a targetlevel of t (second part) during the planning horizon.The objective function is in line with the de nition ofrobust optimization introduced by Mulvey et al. [34].The \max" operator in the second part of the objectivefunction will be linearized with an auxiliary variable,Ct .
H. Davari-Ardakani et al./Scientia Iranica, Transactions E: Industrial Engineering 22 (2015) 2644{2663The set of constraints for the proposed model areas follows:xsf 0 nXi 1xsi0 i0 (1 )n XT XXi 1 1 k2Kin XT XXi 1 1 k2Kiusi0 k i0 k (1 0 ) (7)i 1; ; n;Xk2KiXmsitkk2Kit 1; ; T;1;s 1; ; S; t 0; 1; ; T i 1(11)k 2 Ki ; t;!) n XXi 1 k2Kis sit yit (1n XT XXi 1 t 1 k2Kin XT XXi 1 t 1 k2Kin XT XXi 1 t 1 k2Kin XT XXi 1 t 1 k2Ki i ) s kqitknXi 1i 1(12)s (1 0 )usit k it kcsit k 0 sit k (1 0 )csit k 00Qsik; 0)(1 rp )p t 1s max(kwit kQi 1 t 1 k2Kisi ; 0)(1 rp )p t 1t 0; 1; ; T; l st u ;l st (14)u;P l Pts P u ;K l Kts K u ;s 1; ; S;0t 1; ; T8s; s0 for whichi 1; ; n;1;(15)Bts Btst 0; ; T01;s; s0 1; ; S;(16)8s; s0 for whichyits yitsi 1; ; n;Bts Btst 0; ; T01;s; s0 1; ; S;0usit k 00s max(vit kn XT XXs 1; ; S;(13)siti 1 t 1 k2Ki0s sit xit (1 )hsitn XT XXxsit xsiti 1; ; n; s 1; ; S;t 1; ; T; 1; k 2 Ki ; (10)k 2 Ki ; t;nXt 1; ; T; l st u ;i 1; ; n; s 1; ; S;s wsqitki;t 1; ;knXk 2 Ki ; (9)i 1; ; n;s wsswit ki;t 1; ;k cit kxsf;t 1 (1 rt hsits 1; ; S; (8)t 0; 1; ; Tsmsitk vi;t1; ;ksqitki 1; ; n;s vssvit ki;t 1; ;k uit ks 1; ; S;msitk k xsft ;i 1 k2KiVts xsft csi0 k i00 k (1 0 ) W0yits t 1; ; T;n XXs 1; ; S;s 1; ; S;hsi;t 1 xsit 2649(17)8s; s0 for whichxsft xsftt 0; ; T0usit k usit k1;Bts Btss; s0 1; ; S;8s; s0 for whichi 1; ; n;s; s0 1; ; S;0t 0; ; Tk 2 Ki ;(18)Bts Bts1;0 t;(19)
2650H. Davari-Ardakani et al./Scientia Iranica, Transactions E: Industrial Engineering 22 (2015) 2644{266308s; s0 for whichcsit k csit ki 1; ; n;t 0; ; Ts; s0 1; ; S;0Bts Bts1;i 1; ; n;(20)Bts Btst 0; ; Ts; s0 1; ; S;01;i 1; ; n;t 0; ; Ts; s0 1; ; S;0(21)Bts Bts1; t;Bts Btst 1; ; T;s; s0 1; ; S;k 2 Ki ;08s; s0 for which0(22)i 1; ; n;s qsqitkitk t;k 2 Ki ;8s; s0 for whichmsitk msitk0k 2 Ki ;8s; s0 for whichs wswit kit k t;k 2 Ki ;8s; s0 for whichs vsvit kit k00(23)Bts Btsi 1; ; n;t 1; ; T;s; s0 1; ; S;k 2 Ki :0(24)First, the investor's initial wealth is utilized to construct an initial portfolio. The construction of theinitial portfolio is performed regarding Eq. (7). Weassume that the initial wealth can be invested in riskfree and risky assets, as well as call and put options onrisky assets.Also, we have an inventory equation for all assets.Eq. (8) ensures the balance for quantities of all riskyassets during the planning horizon. The amount ofexercised call and put options at the current period arealso considered in Eq. (8).Since we assume that the lifetime of each optioncan be more than one period, information aboutamounts of call and put options held at all periodsis required. Eqs. (9) and (10) calculate these holdingamounts for di erent call and put options, respectively.The investor is able to take long positions on di erentcall and put options. In other words, di erent optionscan be purchased during their lifetime. This assumption, considered in Eqs. (9) and (10), is in conformancewith real conditions in nancial markets.Eqs. (11) and (12) ensure that at each time period,the amounts of call and put options exercised do notexceed the holding amounts of these options in theprevious time period.In the cash balance Eq. (13), the principal andpro ts of risk free investment and the funds provided byexercising put options on hand and selling risky assetsare used to cover the expenditure for the purchase ofrisky assets, taking long positions on call and put options, exercising call options on hand, and investmentin risk free assets. Linear transaction costs are chargedfor purchases and sales of risky assets, as well as calland put options. In addition, xed transaction costsare charged for purchases of call and put options.Eq. (14) computes the portfolio value at the endof time period t (t 1; ; T ) under scenario s 2 S .The portfolio value represents the value of the risk freeasset, the market value of all risky asset holdings, aswell as the value of call and put option holdings.Eq. (15) sets lower and upper bounds on valuesof all Greek letters of the portfolio at time period t(t 1; ; T ) under scenario s 2 S . The risk takinginvestor can relax bounds on Greek letters and, if thebounds approach in nity, the associated constraintswill be redundant. Contrarily, if the bounds approachzero, the investor will be risk neutral in terms of theassociated Greek letter.Nonanticipativity constraints are important partsof the stochastic programming models that should benecessarily addressed. If two scenarios, s and s0 ,are indistinguishable from the beginning to time t,on the basis of information available at time t, thenthe decision rendered for the two scenarios must beidentical from the beginning to time t. Let Bts denotesa decision part of scenario s 2 S from the beginning totime t. Eqs. (16) to (24) represent constraints that areused to satisfy the nonanticipative condition.In addition, all decision variables are consideredto be nonnegative. The constraints associated with thisassumption, as well as those pertaining to the amountof holdings in the initial investment, are not mentionedfor reasons of brevity.2.6. Pricing options on the scenario treeA key part of incorporating option decisions intomulti-period portfolio optimization problems is theappropriate valuation of call and put options. We usethe Black-Scholes model for pricing European call andput options. The option pricing is done according tothe following formulas:s it kke r( t) N (a2 );sit N (a1 ) 0 sit k ke r( t) N ( a2 )a1 a2 ln(ln(sit N (ss 2it k ) (r ( i ) 2)(n i tp(25)a2 );t)(26);(27)s 2p ( i )t 2)( t) a1 in t;(28)sit k ) (r itsp
H. Davari-Ardakani et al./Scientia Iranica, Transactions E: Industrial Engineering 22 (2015) 2644{2663swhere, it kand 0 sit k denote the prices of call andput options issued on asset i (i 1; ; n), with timeto maturity ( t), and strike price k 2 Ki attime period t (t 0; 1; ; T 1), under scenario s(s 1; ; S ), respectively. In addition, N (:) denotesthe cumulative distribution function of the standardnormal distribution.3. Scenario generationScenario generation is a key step in dealing with theuncertainty of parameters in stochastic programmingmodels. A good scenario set provides the investorwith realistic future information about the market,with which he/she can make better investment andhedging decisions. Although we do not intend to makea detailed discussion about scenario generation techniques, some explanation regarding previous studies,at least in the area of portfolio optimization, seem tobe unavoidable.One popular scenario generation method usedin nancial applications is moment matching, whichminimizes a distance measure between speci ed statistical moments and statistical moments of generatedscenarios. This method was proposed by H yland andWallace [35] and used widely in di erent studies in thearea of nance [27,36,37]. Also, some studies used themoment matching in combination with other methods.For instance, we can mention vector autoregression(VAR) [33], K -means clustering [38] and scenarioreduction [39]. Regardless of its advantages, momentmatching has the important drawback of utilizing acovariance matrix for modelling the dependence structure of returns. The covariance matrix is a useful toolfor modelling the dependence structure of normallydistributed data, since it only measures the lineardependence of the data series. Thus, when the dataseries are not normally distributed, there is not anyguarantee of appropriately capturing the dependencestructure of the data series with the moment matchingmethod. This issue is more important for nancial dataseries, since experience shows that nancial data haveoften fatter tails than normal distribution. This featureis referred to as leptokurtic behavior.Regardless of the important property of the nancial data series, some studies either explicitly assumethat they are normally distributed [33] or, without anydiscussion about normality, use the special propertiesof normal distributions for the data set under consideration [40].Temporal correlation is another important featureof the data series that should be modelled properly.This task is usually performed with time series models. Numbers of research work on applications oftime series in scenario generation can be found inthe literature, e.g. the VAR method [33,41] and the2651hidden Markov model [42]. Generally, in nancial timeseries, a large change tends to be followed by largechanges, and vice versa. This characteristic is referredto as volatility clustering or heteroskedasticity [43].Thus, classic time series models often fail to modeltemporal correlations of nancial time series. To dealwith this speci c feature of nancial time series data,the autoregressive conditional heteroskedastic (ARCH)model of volatility was presented by Engle [44] andfurther developed to the Generalized ARCH (GARCH)model by Bollerslev [45]. A detailed discussion aboutconditional heteroskedastic models and their extensionscan be found in Tsay [46]. A limited number ofstudies use heteroskedastic time series models. Chenand Yuen [47], Chen et al. [48] and Chen [49] usedthe GARCH-type process and conditional samplingto generate scenarios of risky asset returns. Yet,most nancial scenario generation procedures eitherdo not consider the temporal correlations of dataseries [27,39,50] or use traditional homoskedastic timeseries models to capture the temporal correlations ofdata series [33,41]. In addition, there are some studiesthat use reduction methods to provide an accurateand computationally tractable set of scenarios. Beraldiand Bruni [51] presented a reduction method basedon cluster an
strategies make the investor more exible in hedging exposure to di erent aspects of risk. Wu and Sen [22] proposed a stochastic program-ming approach to develop currency option hedging strategies to address multiple random factors in an imperfect market. Their study incorporated con-straints on sensitivity measures, such as Delta and Gamma.