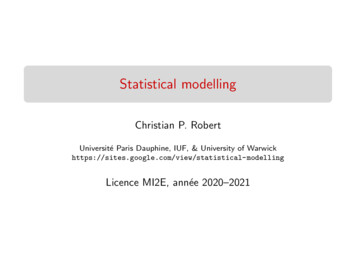
Transcription
Statistical modellingChristian P. RobertUniversité Paris Dauphine, IUF, & University of odellingLicence MI2E, année 2020–2021
g
Outline1the what and why of statistics2statistical models3bootstrap estimation4Likelihood function and inference5Decision theory and Bayesian analysis
Chapter 0 : the what and why of statistics1the what and why of statisticsWhat?ExamplesWhy?
What is statistics?Many notions and usages of statistics, from description to action:summarising datapredicting random eventsextracting significant patternsfrom huge datasetsselecting influential variatesexhibiting correlationsidentifying causessmoothing time seriesdetecting fraudulent datamaking decisions[xkcd]
What is statistics?Many approaches to the fieldalgebradata miningmathematical statisticsmachine learningcomputer scienceeconometricspsychometrics[xkcd]
Definition(s)Given data x1 , . . . , xn , possibly driven by a probability distributionF, the goal is to infer about the distribution F with theoreticalguarantees when n grows to infinity.data can be of arbitrary size and formatdriven means that the xi ’s are considered as realisations ofrandom variables related to Fsample size n indicates the number of [not alwaysexchangeable] replicationsdistribution F denotes a probability distribution of a known orunknown transform of x1inference may cover the parameters driving F or somefunctional of Fguarantees mean getting to the “truth” or as close as possibleto the “truth” with infinite data“truth” could be the entire F, some functional of F or somedecision involving F
Definition(s)Given data x1 , . . . , xn , possibly driven by a probability distributionF, the goal is to infer about the distribution F with theoreticalguarantees when n grows to infinity.data can be of arbitrary size and formatdriven means that the xi ’s are considered as realisations ofrandom variables related to Fsample size n indicates the number of [not alwaysexchangeable] replicationsdistribution F denotes a probability distribution of a known orunknown transform of x1inference may cover the parameters driving F or somefunctional of Fguarantees mean getting to the “truth” or as close as possibleto the “truth” with infinite data“truth” could be the entire F, some functional of F or somedecision involving F
Warning: models are neither true nor realData most usually comes without a model, which is amathematical construct intended to bring regularity andreproducibility, in order to draw inference“All models are wrongbut some are more useful than others”—George Box—Usefulness is to be understood as having explanatory or predictiveabilities
Warning (2)“Model produces data. The data does not produce themodel.”—P. Westfall and K. Henning—Meaning thata single model cannot be associated with a given dataset, nomatter how precise the data getsbut models can be checked by opposing artificial data from amodel to observed data and spotting potential discrepanciesc Relevance of [computer] simulation tools relying on probabilisticmodels
Example 1: spatial patternMortality from oral cancer in Taiwan:Model chosen to beYi P(mi ) {z }log mi log Ei a iPoisson[Lin et al., 2014, Int. J. Envir. Res. Pub. Health](a) and (b) mortality in the 1st and 8threalizations; (c) mean mortality; (d)LISA map; (e) area covered by hotspots; (f) mortality distribution withhigh reliability
Example 1: spatial patternMortality from oral cancer in Taiwan:Model chosen to beYi P(mi ) log mi log Ei a iwhereYi and Ei are observed and age/sexstandardised expected counts in area ia is an intercept term representing thebaseline (log) relative risk across thestudy region(a) and (b) mortality in the 1st and 8threalizations; (c) mean mortality; (d)LISA map; (e) area covered by hotspots; (f) mortality distribution withhigh reliabilitynoise i spatially structured with zeromean[Lin et al., 2014, Int. J. Envir. Res. Pub. Health]
Example 2: World cup predictionsIf team i and team j are playing and score yi and yj goals, resp.,then the data point for this game isqyij sign(yi yj ) yi yj Corresponding data model is:yij N(ai aj , σy ),where ai and aj ability parameters and σyscale parameter estimated from the dataNate Silver’s prior scoresai N(b prior scorei , σa )Resulting confidence[A. Gelman, blog, 13 July 2014] intervals
Example 2: World cup predictionsIf team i and team j are playing and score yi and yj goals, resp.,then the data point for this game isqyij sign(yi yj ) yi yj Potential outliers led to fatter tail model:yij T7 (ai aj , σy ),Nate Silver’s prior scoresai N(b prior scorei , σa )[A. Gelman, blog, 13 July 2014] Resulting confidenceintervals
Example 3: American voting patterns“Within any education category, richer people vote moreRepublican. In contrast, the pattern of education and voting isnonlinear.”
Example 3: American voting patterns“Within any education category, richer people vote moreRepublican. In contrast, the pattern of education and voting isnonlinear.”“There is no plausible way based on these data in which elites canbe considered a Democratic voting bloc. To create a group ofstrongly Democratic-leaning elite whites using these graphs, youwould need to consider only postgraduates (.), and you have togo down to the below- 75,000 level of family income, which hardlyseems like the American elites to me.”[A. Gelman, blog, 23 March 2012]
Example 3: American voting patterns“Within any education category, richer people vote moreRepublican. In contrast, the pattern of education and voting isnonlinear.”
Example 4: Automatic number recognitionReading postcodes and cheque amounts by analysing images ofdigitsClassification problem: allocate a new image (1024x1024 binaryarray) to one of the classes 0,1,.,9Tools:linear discriminant analysiskernel discriminant analysisrandom forestssupport vector machinedeep learning
Example 5: Asian beetle invasionSeveral studies in recent years have shown the harlequin conquering other ladybirds across Europe.In the UK scientists found that seven of the eight native British species have declined. Similarproblems have been encountered in Belgium and Switzerland.[BBC News, 16 May 2013]How did the Asian Ladybird beetlearrive in Europe?Why do they swarm right now?What are the routes of invasion?How to get rid of them(biocontrol)?[Estoup et al., 2012, Molecular Ecology Res.]
Example 5: Asian beetle invasionFor each outbreak, the arrow indicates the most likely invasionpathway and the associated posterior probability, with 95% credibleintervals in brackets[Lombaert & al., 2010, PLoS ONE]
Example 5: Asian beetle invasionMost likely scenario of evolution, based on data:samples from five populations (18 to 35 diploid individuals persample), genotyped at 18 autosomal microsatellite loci,summarised into 130 statistics[Lombaert & al., 2010, PLoS ONE]
Example 6: Are more babies born on Valentine’s day thanon Halloween?Uneven pattern of birth rate across the calendar yearwith large variations on heavily significant dates (Halloween,Valentine’s day, April fool’s day, Christmas, .)
Example 6: Are more babies born on Valentine’s day thanon Halloween?Uneven pattern of birth rate across the calendar year with largevariations on heavily significant dates (Halloween, Valentine’s day,April fool’s day, Christmas, .)The data could be cleaned even further. Here’s how I’dstart: go back to the data for all the years and fit a regression with day-of-week indicators (Monday, Tuesday, etc),then take the residuals from that regression and pipe themback into [my] program to make a cleaned-up graph. It’swell known that births are less frequent on the weekends,and unless your data happen to be an exact 28-year period, you’ll get imbalance, which I’m guessing is driving alot of the zigzagging in the graph above.
Example 6: Are more babies born on Valentine’s day thanon Halloween?I modeled the data with a Gaussianprocess with six components:1slowly changing trend27 day periodical componentcapturing day of week effect3365.25 day periodical componentcapturing day of year effect4component to take into accountthe special days and interactionwith weekends5small time scale correlating noise6independent Gaussian noise[A. Gelman, blog, 12 June 2012]
Example 6: Are more babies born on Valentine’s day thanon Halloween?Day of the week effect has beenincreasing in 80’sDay of year effect has changed onlya little during years22nd to 31st December is strangetime[A. Gelman, blog, 12 June 2012]
Example 6: Are more babies born on Valentine’s day thanon Halloween?Day of the week effect has beenincreasing in 80’sDay of year effect has changed onlya little during years22nd to 31st December is strangetime[A. Gelman, blog, 12 June 2012]
Example 7: Were the 2009 Iranian elections rigged?Presidential elections of 2009 in Iran saw Mahmoud Ahmadinejadre-elected, amidst considerable protests against rigging.We’ll concentrate on vote counts–the number of votesreceived by different candidates in different provinces–andin particular the last and second-to-last digits of thesenumbers. For example, if a candidate received 14,579votes in a province (.), we’ll focus on digits 7 and 9.[B. Beber & A. Scacco, The Washington Post, June 20, 2009]Similar analyses in other countries like Russia (2018)
Example 7: Were the 2009 Iranian elections rigged?Presidential elections of 2009 in Iran saw Mahmoud Ahmadinejadre-elected, amidst considerable protests against rigging.The ministry provided data for 29 provinces, and we examined the number of votes each of the four main candidates–Ahmadinejad, Mousavi, Karroubi and Mohsen Rezai–is reported to have received in each of the provinces–a total of116 numbers.[B. Beber & A. Scacco, The Washington Post, June 20, 2009]Similar analyses in other countries like Russia (2018)
Example 7: Were the 2009 Iranian elections rigged?Presidential elections of 2009 in Iran saw Mahmoud Ahmadinejadre-elected, amidst considerable protests against rigging.The numbers look suspicious. We find too many 7s andnot enough 5s in the last digit. We expect each digit (0,1, 2, and so on) to appear at the end of 10 percent of thevote counts. But in Iran’s provincial results, the digit 7appears 17 percent of the time, and only 4 percent of theresults end in the number 5. Two such departures fromthe average–a spike of 17 percent or more in one digitand a drop to 4 percent or less in another–are extremelyunlikely. Fewer than four in a hundred non-fraudulent elections would produce such numbers.[B. Beber & A. Scacco, The Washington Post, June 20, 2009]Similar analyses in other countries like Russia (2018)
Why modelling?Transforming (potentially deterministic) observations of aphenomenon “into” a model allows fordetection of recurrent or rare patterns (outliers)identification of homogeneous groups (classification) and ofchangesselection of the most adequate scientific model or theoryassessment of the significance of an effect (statistical test)comparison of treatments, populations, regimes, trainings, .estimation of non-linear regression functionsconstruction of dependence graphs and evaluation ofconditional independence
AssumptionsStatistical analysis is always conditional to some mathematicalassumptions on the underlying data like, e.g.,random samplingindependent and identically distributed (i.i.d.) observationsexchangeabilitystationaryweakly stationaryhomocedasticitydata missing at randomWhen those assumptions fail to hold, statistical procedures mayprove unreliableWarning: This does not mean statistical methodology only applieswhen the model is correct
Role of mathematics wrt statisticsWarning: This does not mean statistical methodology only applieswhen the model is correctStatistics is not [solely] a branch of mathematics, but relies onmathematics tobuild probabilistic modelsconstruct procedures as optimising criteriavalidate procedures as asymptotically correctprovide a measure of confidence in the reported resultsc This is a mathematical statistics course
Role of mathematics wrt statisticsWarning: This does not mean statistical methodology only applieswhen the model is correctStatistics is not [solely] a branch of mathematics, but relies onmathematics tobuild probabilistic modelsconstruct procedures as optimising criteriavalidate procedures as asymptotically correctprovide a measure of confidence in the reported resultsc This is a mathematical statistics course
Six quotes from Kaiser FungYou may think you have all of the data. You don’t.One of the biggest myths of Big Data is that data aloneproduce complete answers.Their “data” have done no arguing; it is the humans who aremaking this claim.[Kaiser Fung, Big Data, Plainly Spoken blog]
Six quotes from Kaiser FungBefore getting into the methodological issues, one needs toask the most basic question. Did the researchers check thequality of the data or just take the data as is?We are not saying that statisticians should not tell stories.Story-telling is one of our responsibilities. What we want tosee is a clear delineation of what is data-driven and what istheory (i.e., assumptions).[Kaiser Fung, Big Data, Plainly Spoken blog]
Six quotes from Kaiser FungThe standard claim is that the observed effect is so large as toobviate the need for having a representative sample. Sorry —the bad news is that a huge effect for a tiny non-randomsegment of a large population can coexist with no effect forthe entire population.[Kaiser Fung, Big Data, Plainly Spoken blog]
Chapter 1 :statistical vs. real modelsStatistical modelsQuantities of interestExponential families
Statistical modelsFor most of the course, we assume that the data is a randomsample x1 , . . . , xn and thatX1 , . . . , Xn F(x)as i.i.d. variables or as transforms of i.i.d. variables[observations versus Random Variables]Motivation:Repetition of observations increases information about F, by virtueof probabilistic limit theorems (LLN, CLT)
Statistical modelsFor most of the course, we assume that the data is a randomsample x1 , . . . , xn and thatX1 , . . . , Xn F(x)as i.i.d. variables or as transforms of i.i.d. variablesMotivation:Repetition of observations increases information about F, by virtueof probabilistic limit theorems (LLN, CLT)Warning 1: Some aspects of F may ultimately remain unavailable
Statistical modelsFor most of the course, we assume that the data is a randomsample x1 , . . . , xn and thatX1 , . . . , Xn F(x)as i.i.d. variables or as transforms of i.i.d. variablesMotivation:Repetition of observations increases information about F, by virtueof probabilistic limit theorems (LLN, CLT)Warning 2: The model is always wrong, even though we behave asif.
Limit of averagesCase of an iid sequence X1 , . . . , Xn N(0, 1)Evolution of the range of X̄n across 1000 repetitions, along with one randomsequence and the theoretical 95% range
Limit theoremsLaw of Large Numbers (LLN)If X1 , . . . , Xn are i.i.d. random variables, with a well-definedexpectation E[X]X1 . . . Xn prob E[X]n[proof: see Terry Tao’s “What’s new”, 18 June 2008]
Limit theoremsLaw of Large Numbers (LLN)If X1 , . . . , Xn are i.i.d. random variables, with a well-definedexpectation E[X]X1 . . . Xn a.s. E[X]n[proof: see Terry Tao’s “What’s new”, 18 June 2008]
Limit theoremsLaw of Large Numbers (LLN)If X1 , . . . , Xn are i.i.d. random variables, with a well-definedexpectation E[X]X1 . . . Xn a.s. E[X]nCentral Limit Theorem (CLT)If X1 , . . . , Xn are i.i.d. random variables, with a well-definedexpectation E[X] and a finite variance σ2 var(X), nX1 . . . Xn E[X]ndist. N(0, σ2 )[proof: see Terry Tao’s “What’s new”, 5 January 2010]
Limit theoremsCentral Limit Theorem (CLT)If X1 , . . . , Xn are i.i.d. random variables, with a well-definedexpectation E[X] and a finite variance σ2 var(X), nX1 . . . Xn E[X]ndist. N(0, σ2 )[proof: see Terry Tao’s “What’s new”, 5 January 2010]Continuity TheoremIfdist.Xn aand g is continuous at a, thendist.g(Xn ) g(a)
Limit theoremsCentral Limit Theorem (CLT)If X1 , . . . , Xn are i.i.d. random variables, with a well-definedexpectation E[X] and a finite variance σ2 var(X), nX1 . . . Xn E[X]ndist. N(0, σ2 )[proof: see Terry Tao’s “What’s new”, 5 January 2010]Slutsky’s TheoremIf Xn , Yn , Zn converge in distribution to X, a, and b, respectively,thendist.Xn Yn Zn aX b
Limit theoremsCentral Limit Theorem (CLT)If X1 , . . . , Xn are i.i.d. random variables, with a well-definedexpectation E[X] and a finite variance σ2 var(X), nX1 . . . Xn E[X]ndist. N(0, σ2 )[proof: see Terry Tao’s “What’s new”, 5 January 2010]Delta method’s TheoremIf dist.n{Xn µ} Np (0, Ω)and g : Rp Rq is a continuously differentiable function on aneighbourhood of µ Rp , with a non-zero gradient g(µ), then dist.n {g(Xn ) g(µ)} Nq (0, g(µ)T Ω g(µ))
Entertaining read
Exemple 1: Binomial sampleCase # 1: Observation of i.i.d. Bernoulli variablesXi B(p)with unknown parameter p (e.g., opinion poll)Case # 2: Observation of independent Bernoulli variablesXi B(pi )with unknown and different parameters pi (e.g., opinion poll, fluepidemics)Transform of i.i.d. U1 , . . . , Un :Xi I(Ui 6 pi )
Exemple 1: Binomial sampleCase # 1: Observation of i.i.d. Bernoulli variablesXi B(p)with unknown parameter p (e.g., opinion poll)Case # 2: Observation of conditionally independent BernoullivariablesXi zi B(p(zi ))with covariate-driven parameters p(zi ) (e.g., opinion poll, fluepidemics)Transform of i.i.d. U1 , . . . , Un :Xi I(Ui 6 pi )
Parametric versus non-parametricTwo classes of statistical models:Parametric when F varies within a family of distributionsindexed by a parameter θ that belongs to a finite dimensionspace Θ:F {Fθ , θ Θ}and to “know” F is to know which θ it corresponds to(identifiability);Non-parametric all other cases, i.e. when F is not constrainedin a parametric way or when only some aspects of F are ofinterest for inferenceTrivia: Machine-learning does not draw such a strict distinctionbetween classes
Parametric versus non-parametricTwo classes of statistical models:Parametric when F varies within a family of distributionsindexed by a parameter θ that belongs to a finite dimensionspace Θ:F {Fθ , θ Θ}and to “know” F is to know which θ it corresponds to(identifiability);Non-parametric all other cases, i.e. when F is not constrainedin a parametric way or when only some aspects of F are ofinterest for inferenceTrivia: Machine-learning does not draw such a strict distinctionbetween classes
Non-parametric modelsIn non-parametric models, there may still be constraints on therange of F‘s as for instanceEF [Y X x] Ψ(βT x), varF (Y X x) σ2in which case the statistical inference only deals with estimating ortesting the constrained aspects or providing prediction.Note: Estimating a density or a regression function like Ψ(βT x) isonly of interest in a restricted number of cases
Parametric modelsWhen F Fθ , inference usually covers the whole of the parameterθ and providespoint estimates of θ, i.e. values substituting for the unknown“true” θconfidence intervals (or regions) on θ as regions likely tocontain the “true” θtesting specific features of θ (true or not?) or of the wholefamily (goodness-of-fit)predicting some other variable whose distribution depends onθz1 , . . . , zm Gθ (z)Inference: all those procedures depend on the sample (x1 , . . . , xn )
Parametric modelsWhen F Fθ , inference usually covers the whole of the parameterθ and providespoint estimates of θ, i.e. values substituting for the unknown“true” θconfidence intervals (or regions) on θ as regions likely tocontain the “true” θtesting specific features of θ (true or not?) or of the wholefamily (goodness-of-fit)predicting some other variable whose distribution depends onθz1 , . . . , zm Gθ (z)Inference: all those procedures depend on the sample (x1 , . . . , xn )
Example 1: Binomial experiment againModel: Observation of i.i.d. Bernoulli variablesXi B(p)with unknown parameter p (e.g., opinion poll)Questions of interest:1likely value of p or range thereof2whether or not p exceeds a level p03how many more observations are needed to get an estimationof p precise within two decimals4what is the average length of a “lucky streak” (1’s in a row)
Exemple 2: Normal sampleModel: Observation of i.i.d. Normal variatesXi N(µ, σ2 )with unknown parameters µ and σ 0 (e.g., blood pressure)Questions of interest:1likely value of µ or range thereof2whether or not µ is above the mean η of another sampley1 , . . . , ym3percentage of extreme values in the next batch of m xi ’s4how many more observations to exclude µ 0 from likelyvalues5which of the xi ’s are outliers
Quantities of interestStatistical distributions (incompletely) characterised by (1-D)moments:central momentsZhikµ1 E [X] xdF(x) µk E (X µ1 ) k 1non-central moments ξk E X k k 1α quantileP(X ζα ) αand (2-D) momentsiZjcov(X , X ) (xi E[Xi ])(xj E[Xj ])dF(xi , xj )Note: For parametric models, those quantities are transforms ofthe parameter θ
Example 1: Binomial experiment againModel: Observation of i.i.d. Bernoulli variablesXi B(p)Single parameter p withE[X] p var(X) p(1 p)[somewhat boring.]Median and mode
Example 1: Binomial experiment againModel: Observation of i.i.d. Binomial variables n kXi B(n, p) P(X k) p (1 p)n kkSingle parameter p withE[X] np var(X) np(1 p)[somewhat less boring!]Median and mode
Example 2: Normal experiment againModel: Observation of i.i.d. Normal variatesXi N(µ, σ2 )i 1, . . . , n ,with unknown parameters µ and σ 0 (e.g., blood pressure)µ1 E[X] µ var(X) σ2 µ3 0 µ4 3σ4Median and mode equal to µ
Exponential familiesClass of parametric densities with nice analytic propertiesStart from the normal density: 1ϕ(x; θ) exp xθ x2/2 θ2/22π exp{ θ2/2} exp {xθ} exp x2/2 {z}2πx meets θwhere θ and x only interact through single exponential product
Exponential familiesClass of parametric densities with nice analytic propertiesDefinitionA parametric family of distributions on X is an exponential familyif its density with respect to a measure ν satisfiesf(x θ) c(θ)h(x) exp{T (x)T τ(θ)} , θ Θ, {z}scalar productwhere T (·) and τ(·) are k-dimensional functions and c(·) and h(·)are positive unidimensional functions.Function c(·) is redundant, being defined by normalising constraint:Z 1c(θ) h(x) exp{T (x)T τ(θ)}dν(x)X
Exponential families (examples)Example 1: Binomial experiment againBinomial variableX B(n, p) n kP(X k) p (1 p)n kkcan be expressed as nexp{k log(p/(1 p))}P(X k) (1 p)knhence nc(p) (1 p) , h(x) , T (x) x , τ(p) log(p/(1 p))xn
Exponential families (examples)Example 1: Binomial experiment againBinomial variableX B(n, p) n kP(X k) p (1 p)n kkcan be expressed as nP(X k) (1 p)exp{k log(p/(1 p))}knhence nc(p) (1 p) , h(x) , T (x) x , τ(p) log(p/(1 p))xn
Exponential families (examples)Example 2: Normal experiment againNormal variateX N(µ, σ2 )with parameter θ (µ, σ2 ) and density1f(x θ) exp{ (x µ)2 /2σ2 }2πσ21exp{ x2 /2σ2 xµ/σ2 µ2 /2σ2 } 22πσexp{ µ2 /2σ2 } exp{ x2 /2σ2 xµ/σ2 }2πσ2henceexp{ µ2 /2σ2 } c(θ) , T (x) 2πσ2 2 x 1/2σ2, τ(θ) xµ/σ2
natural exponential familiesreparameterisation induced by the shape of the density:DefinitionIn an exponential family, the natural parameter is τ(θ) and thenatural parameter space isZkΘ τ R ; h(x) exp{T (x)T τ}dν(x) XExample For the B(m, p) distribution, the natural parameter isθ log{p/(1 p)}and the natural parameter space is R
natural exponential familiesreparameterisation induced by the shape of the density:DefinitionIn an exponential family, the natural parameter is τ(θ) and thenatural parameter space isZkΘ τ R ; h(x) exp{T (x)T τ}dν(x) XExample For the B(m, p) distribution, the natural parameter isθ log{p/(1 p)}and the natural parameter space is R
regular and minimal exponential familiesPossible to add and (better!) delete useless components of T :DefinitionA regular exponential family corresponds to the case where Θ is anopen set.A minimal exponential family corresponds to the case when theTi (X)’s are linearly independent, i.e.Pθ (αT T (X) const.) 0 for α 6 0θ ΘAlso called non-degenerate exponential familyUsual assumption when working with exponential families
regular and minimal exponential familiesPossible to add and (better!) delete useless components of T :DefinitionA regular exponential family corresponds to the case where Θ is anopen set.A minimal exponential family corresponds to the case when theTi (X)’s are linearly independent, i.e.Pθ (αT T (X) const.) 0 for α 6 0θ ΘAlso called non-degenerate exponential familyUsual assumption when working with exponential families
IllustrationsFor a Normal N(µ, σ2 ) distribution,1 1exp{ x2/2σ2 µ/σ2 x f(x µ, σ) σ2πµ2/2σ2 }means this is a two-dimensional minimal exponential familyFor a fourth-power distribution4f(x µ) C(θ) exp{ (x θ)4 }} e x e4θ3 x 6θ2 x2 4θx3 θ4implies this is a three-dimensional minimal exponential family[Exercise: find C]
convexity propertiesHighly regular densitiesTheoremThe natural parameter space Θ of an exponential family is convexand the inverse normalising constant c 1 (θ) is a convex function.Example For B(n, p), the natural parameter space is R and theinverse normalising constant (1 exp(θ))n is convex
convexity propertiesHighly regular densitiesTheoremThe natural parameter space Θ of an exponential family is convexand the inverse normalising constant c 1 (θ) is a convex function.Example For B(n, p), the natural parameter space is R and theinverse normalising constant (1 exp(θ))n is convex
analytic propertiesLemmaIf the density of X has the minimal representationf(x θ) c(θ)h(x) exp{T (x)T θ}then the natural statistic Z T (X) is also distributed from anexponential family and there exists a measure νT such that thedensity of Z [ T (X)] against νT isf(z; θ) c(θ) exp{zT θ}
analytic propertiesTheoremIf the density of Z T (X) against νT is c(θ) exp{zT θ}, if the realvalue function ϕ is measurable, withZ ϕ(z) exp{zT θ} dνT (z) on the interior of Θ, thenZf : θ ϕ(z) exp{zT θ} dνT (z)is an analytic function on the interior of Θ andZ f(θ) zϕ(z) exp{zT θ} dνT (z)
moments of exponential familiesNormalising constant c(·) generating all momentsPropositionIf T (·) : X Rd and the density of Z T (X) is exp{zT θ ψ(θ)},then Eθ exp{T (x)T u} exp{ψ(θ u) ψ(θ)}and ψ(·) is the cumulant generating function.[Laplace transform]
moments of exponential familiesNormalising constant c(·) generating all momentsPropositionIf T (·) : X Rd and the density of Z T (X) is exp{zT θ ψ(θ)},then ψ(θ)Eθ [Ti (X)] i 1, . . . , d, θiand 2 ψ(θ)Eθ Ti (X) Tj (X) θi θji, j 1, . . . , dSort of integration by part in parameter space:Z Ti (x) log c(θ) c(θ)h(x) exp{T (x)T θ}dν(x) 1 0 θi θi
Sample from exponential familiesTake an exponential family f(x θ) h(x) exp τ(θ)T T (x) ψ(θ)and id sample x1 , . . . , xn from f(x θ).Then nnYXf(x1 , . . . , xn θ) h(xi ) exp τ(θ)TT (xi ) nψ(θ)i 1i 1RemarkFor an exponential family with summary statistic T (·), the statisticS(X1 , . . . , Xn ) nXT (Xi )i 1is sufficient for describing the joint density
Sample from exponential familiesTake an exponential family f(x θ) h(x) exp τ(θ)T T (x) ψ(θ)and id sample x1 , . . . , xn from f(x θ).Then nnYXf(x1 , . . . , xn θ) h(xi ) exp τ(θ)TT (xi ) nψ(θ)i 1i 1RemarkFor an exponential family with summary statistic T (·), the statisticS(X1 , . . . , Xn ) nXT (Xi )i 1is sufficient for describing the joint density
connected examples of exponential familiesExampleChi-square χ2k distribution corresponding to distribution ofX21 . . . X2k when Xi N(0, 1), with densityzk/2 1 exp{ z/2}fk (z) z R 2k/2 Γ (k/2)
connected examples of exponential familiesCounter-ExampleNon-central chi-square χ2k (λ) distribution corresponding todistribution of X21 . . . X2k when Xi N(µ, 1), with density k1fk,λ (z) 1/2 (z/λ) /4 /2 exp{ (z λ)/2}Ik/2 1 ( zλ) z R where λ kµ2 and Iν Bessel function of second order
connected examples of exponential familiesCounter-ExampleFisher Fn,m distributioncorresponding to the ratioZ Yn /nYn χ2n , Ym χ2m ,Ym /mwith densityfm,n (z) (n/m)n/2 n/2 1n m(1 n/mz) /2 z R znmB( /2, /2)
connected examples of exponential familiesExampleIsing Be(n/2, m/2) distribution corresponding to the distribution ofZ nYwhen Y Fn,mnY mhas densityfm,n (z) 1nmz /2 1 (1 z) /2 1 z (0, 1)B(n/2, m/2)
connected examples of exponential familiesCounter-ExampleLaplace double-exponential L(µ, σ) distribution corresponding tothe rescaled difference of two exponential E(σ 1 ) random variables, Z µ X1 X2 when X1 , X2 iid E(σ 1 )has densityf(z; µ, σ) 1exp{ σ 1 x µ }σ
chapter 2 :the bootstrap methodIntroductionGlivenko-Cantelli TheoremThe Monte Carlo methodBootstrapParametric Bootstrap
Motivating exampleCase of a random event with binary (Bernoulli) outcome Z {0, 1}such that P(Z 1) pObservations z1 , . . . , zn (iid) put to use to approximate p byp p (z1 , . . . , zn ) 1/nnXzii 1Illustration of a (moment/unbiased/maximum likelihood) estimatorof p
intrinsic statistical randomnessinference based on a random sample implies uncertaintySince it depends on a random sample, an estimatorδ(X1 , . . . , Xn )also is a random variableHence “error” in the reply: an estimator produces a
Why modelling? Transforming (potentially deterministic) observations of a phenomenon \into" a model allows for detection of recurrent or rare patterns (outliers) identi cation of homogeneous groups (classi cation) and of changes selection of the most adequate scienti c model or theory ass