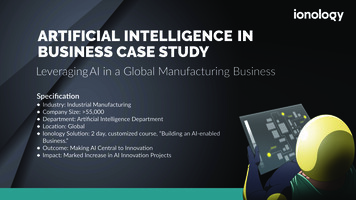
Transcription
ARTIFICIAL INTELLIGENCE INBUSINESS CASE STUDYLeveraging AI in a Global Manufacturing BusinessSpecifica(on Industry: Industrial Manufacturing Company Size: 55,000 Department: Ar@ficial Intelligence Department Loca@on: Global Ionology Solu@on: 2 day, customized course, “Building an AI-enabled Business.”Outcome: Making AI Central to Innova@onImpact: Marked Increase in AI Innova@on Projects
THE SITUATIONTechnology doesn’t Transform a Business;People do Leveraging TechnologyBeing a global market leader, the heavy machinerymanufacturer in this case study is constrained bydepartmental poli@cs, policies, silos, regula@on andfabrica@on challenges. A very capable AI and Data Science division was created tomodernise the business The company data was siloed and required extensive prepara@on,but no more than typically found in large enterprises The business owners claimed they want to ‘transform’ leveragingThese challenges prevent it from crea@ng new businessAI, but when asked what that means, they all give differentmodels, products and services that are only made possibleanswersby Machine Learning.The materials department were reluctant to move awayfrom aluminium as their preferred material, to compositematerials. They claim there’s no global study directlycomparing both, even though the advantages tocomposites are obvious. The transi@on would require acomplete transforma@on of engineering, design, legal, andcompliance prac@ces. The business wants to transform just not that much! AI projects were sporadic and ad hoc and relied on self-educatedbusiness innovators The innova@on, design and business unit heads, had yet to receiveformal training on the business capabili@es of AI.
THE PROBLEMNew Business Models are Needed toEnhance Opera(onal EfficienciesThere are two opportuni@es to create compe@@veadvantage and build new business revenue streams.1. Move from the use of aluminium to composite materials.The problem was that there was no accepted science peer-CHALLENGES If the business wanted to move part of its manufacturing awayfrom aluminium to composite materials it needed to create ame@culous, peer-reviewed materials science review. The current servicing model of the business was financed on therou@ne swapping of mechanical parts by highly trained engineers.Much of the swapping was considered ‘preventa@ve maintenance’(PM). That is, the swapping out of parts before they break. Machine Learning could predict the likely failure of parts andreviewed comparison of the materials in real-world use.could replace PM. It would result in reduced parts acquisi@onsThis review would give legal cover for moving from theand enhance the maintenance model. Addi@onally, it would alsotried and tested aluminium to a lighter weight composite.open a new second-hand market for parts.There was, therefore, a reluctance to move materialsdespite the obvious benefits of doing so.2. Parts used in the machines sold by the manufacturerrequired maintenance. For safety reasons, many wereswapped out long before they reached their natural end oflife. This was expensive for the machine owner and anecologically unsound prac@ce as the used parts were simplydiscarded.
THE INSIGHTU(lise AI Advocates to First Ini(ate Change,the Rest will Follow . Businesses that have studied digital transforma@on, haveSTART WITH THE FEW, NOT THE MANYNo one stands up in the boardroom and says “I object to change”. Inreality, this is what happens when they leave the boardroom andreturn to their desk.Don’t try and change those that are not willing to embrace the newdefined it to be the complete reposi@oning of theiropportuni@es presented by AI. Seek first a coali@on of the willingbusiness in the digital economy. They have preparedand demonstrate the success before moving onto the new project.their staff for wholesale change and are much more likelyto leverage the business poten@al of AI than businessesSeek to create short, interes@ng communica@ons that gives kudos tothat have overplayed ‘digital/technology.”those that par@cipated in the change rather than the technology orthe outcome. The use of Machine Learning pushes the business intoareas of research, development and engineering that it isnot comfortable with. Machine Learning has the ability to radically improve thecustomer experience in maintenance. This could offerhuge compe@@ve advantage, however, the businessneeded to change radically and quickly.
THE SOLUTIONStart Small and Manage the Change Create a semi-supervised ML solu@on that takesTHE OUTCOME In this case study, the success was as much down to thecommunica@ons as it was to the technology People, when brought on a journey of change were much moreunstructured data and compares materials. Then build anaccep@ng when they could see simula@ons and understood theinterface that will allow for the query of key proper@es ofpersonal impactaluminium compared to composite materials, with par@culara en@on to areas that are most scru@nised by industryregulators Build a coali@on of those willing to change using the tool Demonstrate the speed at which accurate, unbiased andcomprehensible insights can be a ained Create a very visual simula@on of the business case forchanging the maintenance business model from‘preventa@ve maintenance’ to ‘predic@ve maintenance’ andhow it will greatly increase compe@@ve advantage and opena new world of second-hand parts that have a validated lifestory. Once educated on the business poten@al of AI most leaders weremuch more accessible and open to assis@ng.
IONOLOGY ASSISTANCEIonology Executive Education and AdvisoryServicesPRACTICAL STEPS Educate business owners and decision-makers using nontechnical experiments that relate to their business problems Do it in a classroom - shared learning environmentIonology started with a comprehensive educa@on programfor the senior leaders and decisions makers. We helped Seek a collec@ve of willing par@cipantsthem understand the business poten@al of AI withoutgebng technical. By the end of the program, they had allmade Machine Learning models and built applica@ons usingthem.There’s a tendency with AI projects to be either tooambi@ous, too technically focused or they are designed tosolve a problem that is not important. Through our advisoryservices we also helped with the deep diagnosis,established the right level of communica@ons with keystakeholders that needed convincing and consulted on thecrea@on of the ML models, placorms and user experience. Find a problem to solve that will have a strong human story to tellat the end and is in-line with the strategic impera@ve.
Create a very visual simulaon of the business case for changing the maintenance business model from ‘preventave maintenance’ to ‘predicve maintenance’ and how it will greatly increase compeve advantage and open a new world of second-hand parts that have a validated li